While artificial intelligence (AI) has shown considerable progress in many areas of medical imaging, applications in abdominal imaging, particularly for the gastrointestinal (GI) system, have notably lagged behind advancements in other body regions. This article reviews foundational concepts in AI and highlights examples of AI applications in GI tract imaging. The discussion on AI applications includes acute & emergent GI imaging, inflammatory bowel disease, oncology, and other miscellaneous applications. It concludes with a discussion of important considerations for implementing AI tools in clinical practice, and steps we can take to accelerate future developments in the field.
Key points
- •
The development of artificial intelligence (AI) applications in gastrointestinal (GI) imaging is still in its early stages with most applications being limited to academic research.
- •
Many of the published studies are limited by single-center retrospective designs, small sample sizes, and insufficient external validation.
- •
Further advancements in GI AI applications require intensified efforts to generate high-quality representative datasets, overcoming challenges in GI tract segmentation, and external validation of models.
Introduction
The potential benefits of artificial intelligence (AI) in medical imaging include enhanced diagnostic accuracy, increased interpretive efficiency, greater consistency among radiologists, automation of repetitive tasks, and the creation of new imaging-based biomarkers. While AI has shown considerable progress in many areas of medical imaging, applications in abdominal imaging, particularly for the gastrointestinal (GI) system, have notably lagged behind advancements in other body regions. Several challenges have contributed to this slower rate of innovation including the scarcity of open-source imaging datasets and the considerable resource demands in curating and annotating datasets. There are also particular challenges in segmenting the GI tract compared to other abdominal structures due to its greater morphologic complexity and mobility. This difficulty is further exacerbated when the bowel wall is thin, intra-abdominal fat is limited, or bowel loops are closely opposed, making delineation particularly challenging. Due to these complexities, there are currently disproportionately few commercial AI systems that focus on GI applications, with most being vendor-specific endoscopy assistant solutions and computer-aided diagnosis for virtual colonoscopy/computed tomography (CT) colonography. Many of the published studies in this field are limited by single-center retrospective designs, small sample sizes, and insufficient external validation. Despite these limitations, current research shows significant potential for AI applications in GI imaging.
This article will review some foundational concepts in AI and highlight example AI applications in GI tract imaging. The discussion on AI applications will be organized into broad themes: acute & emergent GI imaging, inflammatory bowel disease, oncology, and miscellaneous applications. It will conclude with a discussion of important considerations for implementing AI tools in clinical practice, and steps we can take to accelerate future developments in the field.
Overview of Artificial Intelligence Concepts
This review is not meant to serve as an introduction to AI. For a more in-depth exploration, readers are encouraged to consult comprehensive reviews by others. However, the authors will outline some key concepts essential for understanding machine learning and radiomics, both of which fall under the umbrella of AI.
Machine learning (ML) systems are fundamentally pattern recognition systems that extract valuable information from a set of representative examples, known as a dataset, through a process called training. The output of the training process is called a model, which can then be used to predict previously unseen examples. Consequently, all ML systems are heavily dependent on the dataset used for training, which significantly influences a model’s capabilities and performance. If the training dataset is too small, incomplete, or contains biased data, the model may fail in its intended task. In AI terminology, this is called a failure to generalize. , The more representative and varied the training data is, by including diverse patient populations, health care institutions, scanners, and protocols, the more likely the resultant model will be able to generalize and be clinically useful.
External validation is a crucial step in the translation of models from the development laboratory to real-world clinical settings. Testing a model with a dataset that is temporally and geographically distinct from the training data can help demonstrate its capability to perform well in various settings. However, curating external validation datasets, particularly multi-center datasets, is a challenging and resource-intensive activity. A meta-analysis by Kim and colleagues found that out of 519 published radiology AI studies, only 31 studies (6.0%) performed external validation. A follow-up study showed that the vast majority of studies that perform external validation demonstrate some decrease in performance (70 of 86, 81.4%) with nearly a quarter reporting a substantial decrease. Unfortunately, a model’s failure to generalize is a common event in the translation of academic research to real-world clinical environments.
Radiomics
Many of the applications described in this review rely on a technique known as radiomics. This approach is based on AI techniques that require feature engineering and utilize statistical visual features such as intensity, shape, or texture ( Fig. 1 ). Most work in this field employs large, predefined libraries of imaging features that have demonstrated utility in other domains. These features can be extracted from segmented lesions and subsequently analyzed using a combination of dimensionality reduction techniques (eg, Least Absolute Shrinkage and Selection Operator [LASSO]) and an AI-based classifier. Although this technique shows promise, the resultant models often struggle with generalization.
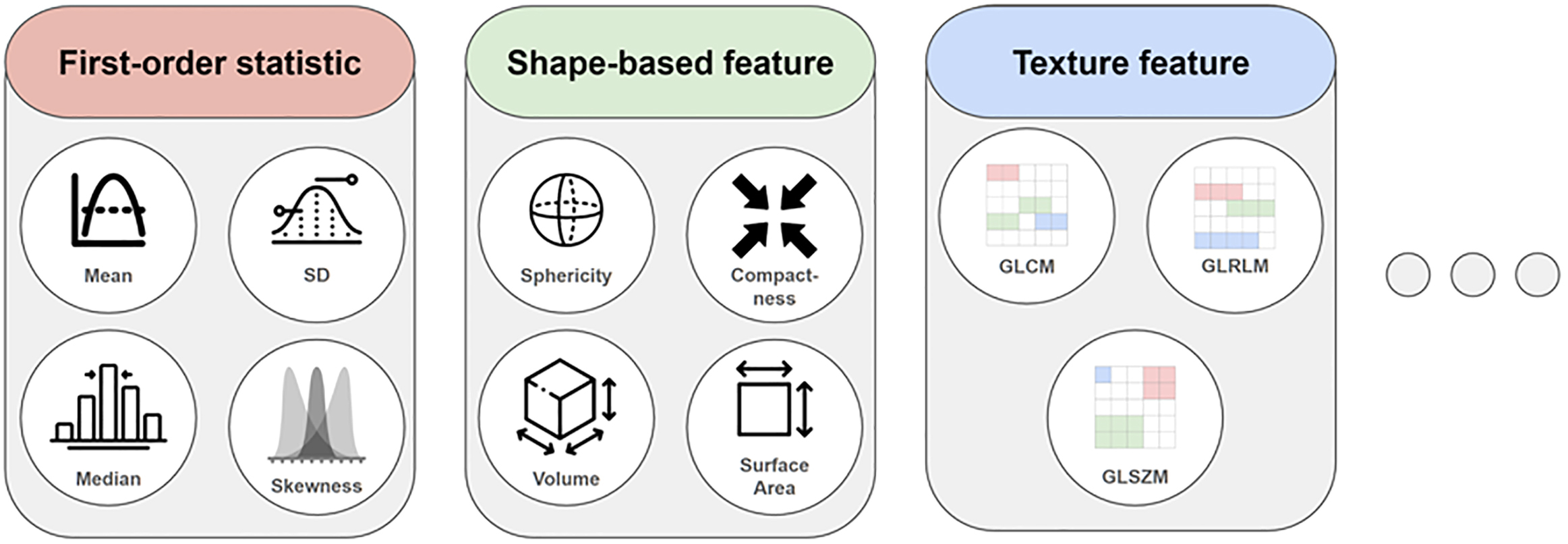
Applications in acute gastrointestinal conditions
Acute abdominal pain is a common emergency presentation with a wide range of potential causes, often involving the GI system. Since patient presentation and laboratory findings are often non-specific and many pathologies are potentially life-threatening, imaging is routinely used to aid in diagnosis. Although still in its infancy, AI systems are starting to emerge, helping to interpret these studies more rapidly and accurately, with the goal of accelerating patient treatment. However, most current ML systems used in acute GI diagnosis perform decision support rather than generating definitive diagnosis.
Acute Appendicitis
Acute appendicitis is a common surgical emergency with the potential for significant consequences in a missed or delayed identification. Since there is considerable overlap in the presentation between acute appendicitis and other pathologies, imaging plays a critical role in diagnosis with CT being considered the gold standard for evaluation, and ultrasound representing another important tool. Investigators have explored utilizing ML systems with both modalities showing some early success. For instance, Park and colleagues used a convolutional neural network (CNN) to diagnose acute appendicitis on CT demonstrating an overall sensitivity, specificity, and accuracy of 90.2%, 92.0%, and 91.5%, respectively. Using ultrasound imaging in a pediatric population, Marcinkevičs and colleagues utilized a multiview concept bottleneck model to detect acute appendicitis along with other related important supporting imaging characteristics.
The earliest AI acute appendicitis projects involved predicting the likelihood of acute appendicitis from clinical history, laboratory findings, and demographic data. For instance, Park and Kim showed significantly better accuracy than the commonly used Alvarado appendicitis scoring system when using a radial basis function neural network (72.2% vs 99.8%). Recent studies have combined imaging with other clinical factors as model inputs to enhance overall performance. Byun and colleagues utilized such a combination approach showing a sensitivity, specificity, and accuracy of 91.8%, 90.0%, and 90.9%, respectively, with improved performance over using CT alone. Akgül and colleagues used a similar approach merging physical examination findings, biomarkers, and ultrasound to create a superior classifier over any of these in isolation. These studies highlight the utility of moving beyond the more common ML approach of considering imaging studies in isolation.
Pneumoperitoneum
The detection of pneumoperitoneum is a common reason for performing abdominal radiographs (AXR). Park and colleagues developed an ML model to detect pneumoperitoneum with a sensitivity and specificity of 85.4% and 73.3%, respectively, on supine AXRs, and 91.1% and 95.0%, respectively, on erect studies. de Cea and colleagues used a combination of multiple ML models with pooling of results to detect pneumoperitoneum on chest radiographs with an area under the receiver operator curve (AUC) of 0.934. This latter approach demonstrates a key potential use of AI systems—the detection of important pathologies outside the usual scope of the imaging study.
Small Bowel Obstruction
Another common cause for emergent presentation with abdominal pain is small bowel obstruction, which is often investigated with both CT and AXR imaging. There are now emerging ML systems for decision support for both modalities.
Researchers have developed systems for both the detection and localization of small bowel obstruction (SBO) on CT. For instance, Vanderbecq and colleagues used a 3-dimensional (3D) mixed CNN as a binary classifier for bowel obstruction generating a sensitivity and specificity of 98% and 76%, respectively. Murphy used a 3D U-Net CNN to segment the small bowel and used this segmentation for classification based on standard reference values for dilation ( Fig. 2 ), generating a sensitivity and specificity for detecting dilated loops of 83% and 90%, respectively. Beyond detection alone, Vanderbecq and colleagues used a CNN and probabilistic mapping to detect the transition site in SBO with an AUC of 0.93.
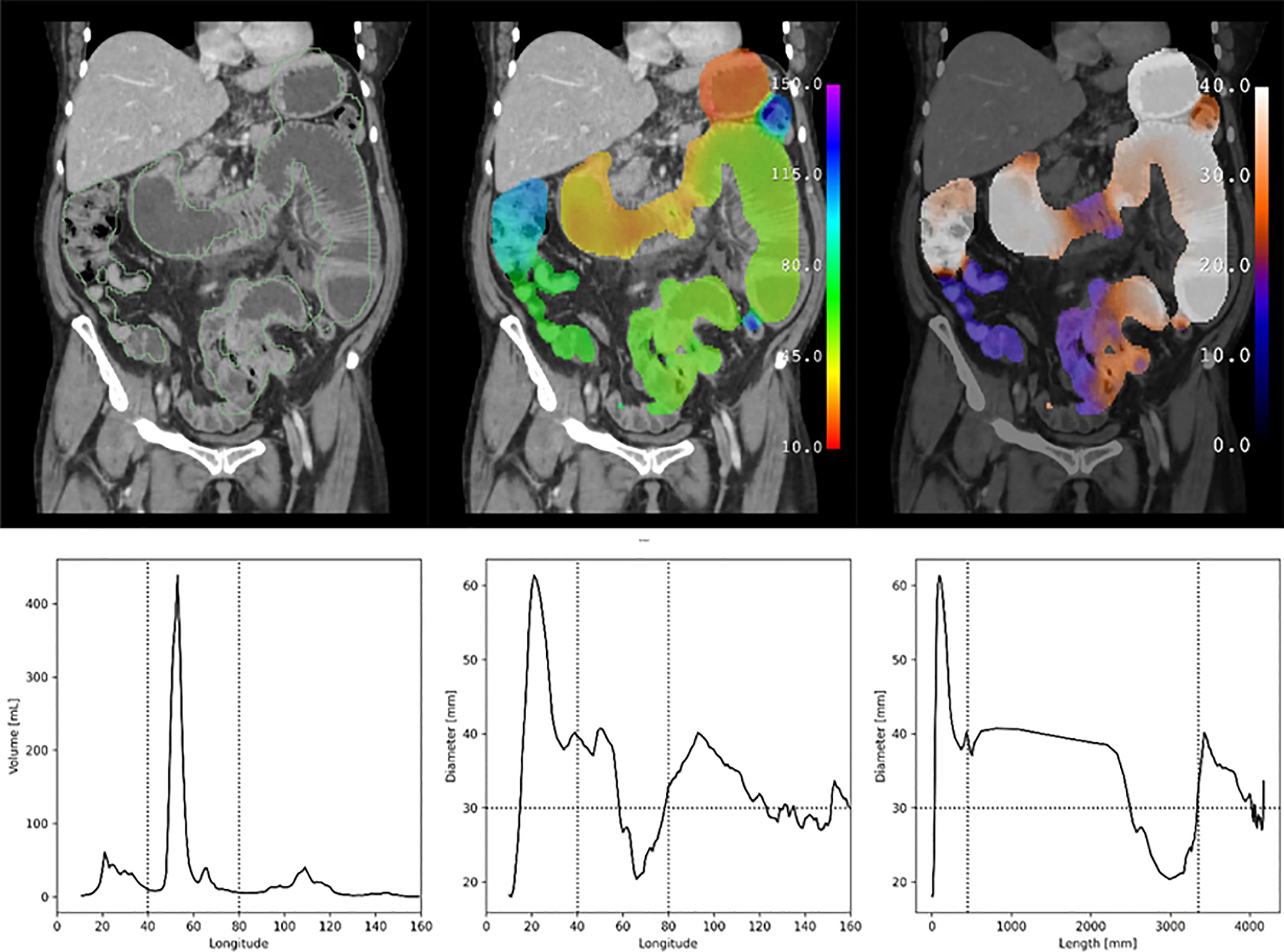
Developing tools that utilize AXR imaging is important as this remains a common screening tool in the emergency setting. Cheng and colleagues used a CNN for the detection of high-grade SBO with a sensitivity, specificity, and AUC of 83.8%, 68.1%, and 0.84, respectively. Similarly, Kim and colleagues used a CNN for the detection of bowel obstruction on AXR, demonstrating a sensitivity and specificity of 91% and 93%, respectively.
Traumatic Bowel Injury
There has been sparse ML research in traumatic bowel injuries in part due to a lack of available datasets. However, this has changed following the 2023 Radiological Society of North America (RSNA) Abdominal Trauma Detection AI Challenge which led to the release of a large expert annotated, multi-institutional CT dataset which includes bowel and mesenteric injuries. Using this dataset, Shen and colleagues published the first ML study for detecting bowel injuries on CT with a specificity of 94.3% and sensitivity of only 14.9%, providing a system capable of excluding bowel injury but a poor positive predictive value. Their study also included the detection of liver, splenic, and renal injuries, highlighting the potential of an ML system that can aid in the diagnosis of a wide range of pathologies in an integrated fashion.
Applications in inflammatory bowel disease
Crohn’s disease (CD) is a chronic immune-mediated transmural inflammatory bowel disease affecting the entire GI tract. Most commonly affecting the small bowel and particularly the terminal ileum (TI), its natural history is progressive and eventually leads to irreversible damage. Clinical assessment of CD is poor because symptoms do not correlate with disease severity and clinical assessment correlates poorly with endoscopic findings. CT enterography (CTE) and magnetic resonance (MR) enterography (MRE) play a significant role in the diagnostic workup and monitoring of CD. These imaging tests have been demonstrated to impact medical and surgical management.
Significant effort has led to the creation of international society-endorsed standardized reporting systems and multiple quantitative CD activity scoring systems, including the magnetic resonance index of activity (MaRIA), Crohn’s Disease Magnetic Resonance Imaging Index (CDMI), magnetic resonance enterography global score (MEGS), Nancy score, and simplified MaRIA. These efforts have built systems to measure disease activity and response to treatment on imaging studies and facilitated the rapid uptake of imaging studies as entry criteria and outcome measures in clinical trials. These scoring systems are based on assessment with MRE and thus can measure transmural healing, as opposed to older systems that are based on endoscopic or clinical findings only. All of these systems have seen limited adoption due to barriers such as the need to incorporate qualitative findings leading to poor observer agreement, the practical limit of visualized features that can plausibly be incorporated, and the requirement of complex calculations of scores.
Diagnostic Interpretation and Disease Assessment
There is value in building AI applications based on or adopting these quantitative and semi-quantitative reporting systems as AI assistance may help overcome adoption barriers. There is a growing body of literature applying ML to MRE-referenced disease activity scoring indices and the qualitative findings upon which they are based, in both pediatric and adult populations. Guez and colleagues assessed the performance of ML models whose inputs were MRE data of the TI based on MaRIA and sMaRIA scores, along with an additional pediatric-specific CD MRE-based scoring system incorporating diffusion-weighted imaging. The various ML models with inputs of 3 different MRE scoring systems had similar performances with AUC ranging between 0.80 and 0.81. The ML models in their study also improved correlation between all types of MRE scores and endoscopic-based scoring systems. Li and colleagues developed 3 ML models to evaluate the disease activity of CD undergoing dual energy CTE by assessing a combination of 9 quantitative and qualitative imaging findings, including contrast enhancement and iodine concentration, and comparing to a ground truth of inflammation of the TI on endoscopy using the Simplified Endoscopic Activity Score for Crohn’s Disease (SES-CD) score. Many of the imaging findings they selected are also used in enterography-based scoring systems. They found that all ML models performed well in evaluating CD activity based on CT findings with AUC ranging from 0.808 to 0.869. Wasnik and colleagues attempted to specifically address the limitation of including qualitative assessments of findings in the MRE-based scoring systems by using 2 ML models (Random Forest and CNNs) to assess the presence of qualitative CD findings and predict disease activity. They also incorporated spatial distribution of disease into the models, a feature of CD imaging assessment that is known to require human expertise ( Fig. 3 ). The performance of these models, based on 165 patients undergoing CTE assessed for the presence and spatial distribution of 5 CTE findings of CD yielding 29,895 individual finding assessments, ranged between AUC values of 0.869 and 0.948. ML models that evaluate cumulative ileal injury in CD using CTE and automated segmentation of diseased segments have demonstrated potential for improved prediction of future surgeries compared to standard enterography and clinical measures. Stidham and colleagues reported an AUC of 0.76 in a model that predicts the need for surgery within 3 years by combining spatial bowel severity from enterography with clinical data.
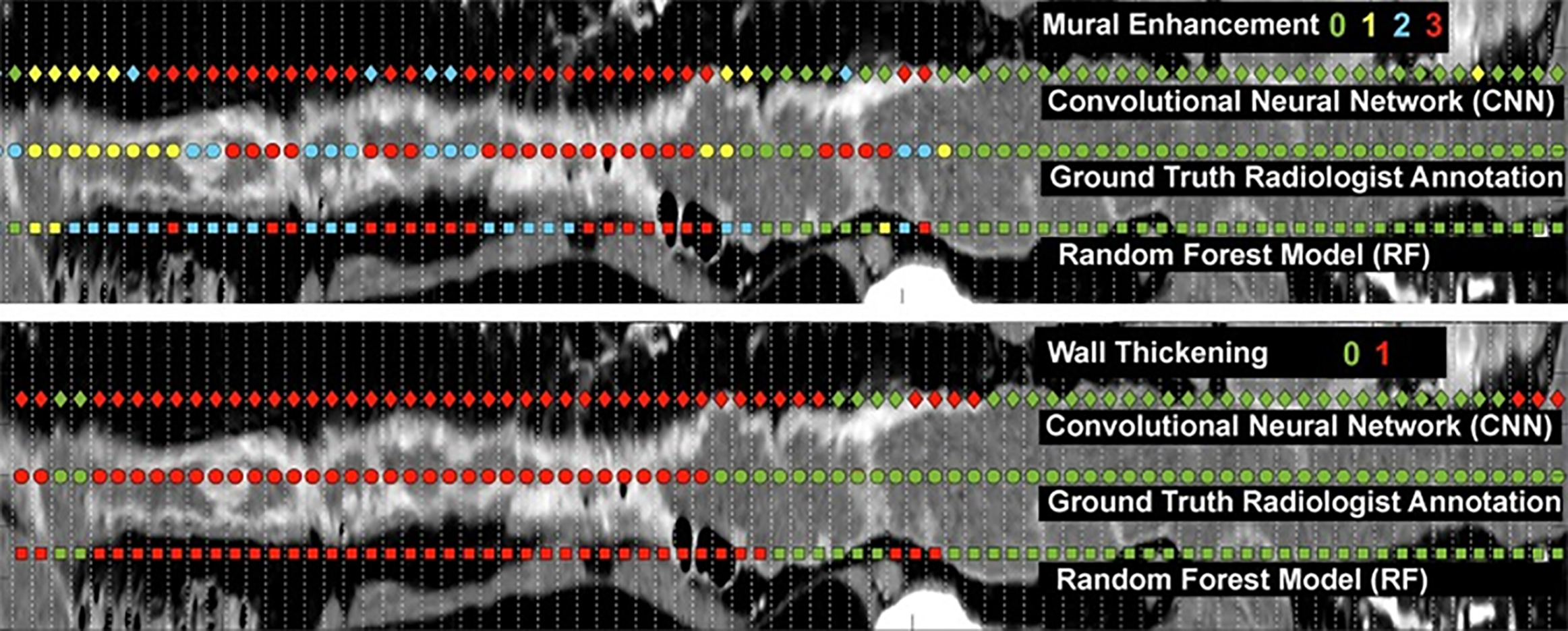
Radiomics has been used to assess and predict disease activity and to assess intestinal fibrosis in CD. Gao and colleagues used ML for segmentation, extraction of radiomics features from CD lesions, and multiple ML models to assess CD activity on CTE. Model accuracy ranged between an AUC of 0.729 and 0.862 relative to a gold standard of non-imaging-based SES-CD. Meng and colleagues developed both deep learning (DL) and radiomics models for the detection of intestinal fibrosis on CTE and showed that both approaches outperformed radiologists in detecting intestinal fibrosis (AUC 0.811 for DL and 0.813 for radiomics), but that image processing times for the DL model was much shorter than radiomics.
The use of contemporary ML and radiomics to assist CTE and MRE interpretation of CD activity assessment will grow and is also likely to increase both the adoption of existing and the creation of new standardized scoring systems. As such, this is a highly impactful application of ML to CD imaging.
Other Applications for Crohn’s Disease Patients
Other investigators have explored the use of ML in distinguishing CD from other diseases radiologically, improving image quality, and 3D image processing and reconstruction. Several studies have built radiomics models to distinguish CD from intestinal tuberculosis (ITB), given their similar appearance on CT. Zhu and colleagues used 9 radiomics and 2 clinical features to build a model analyzing inflammatory ileocecal lesions with CD or ITB and were able to achieve an AUC of 0.93. Lu and colleagues developed a multi-modal radiomics model to distinguish CD from ITB using 21 MRE, 3 clinical, 5 colonoscopy, and 4 pathology features, and found strong model performance with an AUC of 0.94.
Recent studies have shown that DL techniques can be used to improve the image quality of MRE. Son and colleagues showed an improved quality score and increased signal-to-noise ratio following the use of a DL reconstruction model.
ML models have also been shown to aid surgical planning through 3D image reconstruction. Jeri-McFarlane et al used CNNs on pelvic MR imaging examinations with complex perianal fistulas to provide a comprehensive visual representation of perianal disease. This shows the potential of AI to improve surgical planning and patient outcomes.
Barriers and Challenges in Crohn’s Disease Assessment
Two important technical challenges in advancing the use of ML in the imaging of CD with enterography are bowel segmentation and the wide spatial distribution of disease across the GI tract. Manual, semi-automated, and automated bowel segmentation techniques have been described. With a semi-automated approach, radiologists annotate center points along the course of the GI tract. These center points are used as a reference to perform a curved planar reformation to reconstruct the original bowel volume, and then followed by automated segmentation of bowel wall boundaries. Automatic segmentation is possible using ML methods such as U-Net neural networks. Gao and colleagues found high agreement between the automatic technique and experienced radiologists with a Dice similarity coefficient (DSC) of 82.4%. Lamash and colleagues found high agreement between CNN-based automatic and manual segmentation while assessing active inflammation in pediatric CD patients with the DSC ranging between 75% and 81%. Causes for error in automatic segmentation include heterogeneous patterns of CD lesions, patients with less abdominal fat creating less gap between adjacent intestinal walls, poor bowel distention, and non-intestinal structures mimicking bowel loops. Automatic segmentation should benefit radiologists by being able to identify CD lesions with greater speed and accuracy. Improvements in automatic segmentation will facilitate innovation in the application of AI not only for CD but also for other bowel applications.
Stidham and colleagues have highlighted the value of ML in improving the capture of the wide spatial distribution of CD on enterography studies. This aspect is readily appreciated by radiologists but often not considered in existing scoring systems and most existing AI tools. The incorporation of spatial distribution, in addition to CD lesion features, could possibly lead to novel biometrics and improved quantitative scoring of CD activity. An early pilot study of a spatially sensitive model of ileal CD on CTE showed better prediction of future surgery compared to traditional measurements of bowel wall thickness, luminal narrowing, proximal bowel dilation, and mural enhancement.
Applications in oncology—rectal cancer
Rectal cancer is the third most common malignancy in the United States. Medical imaging plays a crucial role in staging and treatment planning, with MR imaging as the reference standard for local staging while CT is used for distant staging. Despite its importance, we continue to face challenges such as differentiating T2 from T3 tumors, detecting lymph node metastases, and assessing treatment response.
Staging
MR imaging is considered a key imaging modality for the T staging of rectal cancer with a reported sensitivity and specificity of 87%, and 75%, respectively. Differentiating early-stage (T1-T2) from locally aggressive (T3-T4) tumors is crucial for treatment planning, as T3/T4 tumors typically require neoadjuvant chemoradiotherapy. However, distinguishing T2 from borderline T3 tumors remains a significant challenge, arising from the overlap in imaging findings between benign peritumoral desmoplastic reaction and tumor infiltration beyond the muscularis propria. Studies have shown that T2 tumors are often over-staged which places the patient at increased risk of treatment-related morbidity and mortality.
Many investigators have focused on using AI for the binary classification between T1/T2 and T3/T4 tumors. For example, Hou and colleagues employed an ML method that first enhances the z-axis resolution of T2-weighted imaging, followed by differentiating between T1/2 and T3/4 tumors. Their classification model demonstrated an AUC of 0.869, an accuracy of 83.3%, a sensitivity of 71.1%, and a specificity of 93.1%, and outperformed radiologists (AUC 0.685). You and colleagues used a radiomics approach with high-resolution T2-weighted imaging and apparent diffusion coefficient (ADC) maps to differentiate T1/T2 from T3/T4, which demonstrated a 0.910 AUC, 90.0% sensitivity, 88.6% specificity, and 87.7% accuracy.
Determining lymph node involvement in rectal cancer patients is crucial, as it serves as both a prognostic indicator and a key factor in clinical decision-making. Similar to T staging, it influences decisions regarding surgical strategy and the need for neoadjuvant therapy. Contemporary practice for assessing lymph nodes primarily relies on features such as size, signal intensity, morphology, border, and ADC value. However, determining lymph node involvement is challenging, as evidenced by the wide range of reported sensitivities (32%–77%) and specificities (76%–94%). , In a recent study, Xia and colleagues developed a lymph node diagnosis model that achieved an AUC of 0.81 with a 79% accuracy, 70% sensitivity, and 84% specificity for binary lymph node staging. The model’s performance was comparable to experienced radiologists (AUC 0.79), and improved radiologist performance when used as an aid. Their automated approach could detect and segment lymph nodes in less than 2 seconds and used heat maps to enhance interpretability by highlighting areas of concern in lymph nodes ( Fig. 4 ).

Full access? Get Clinical Tree
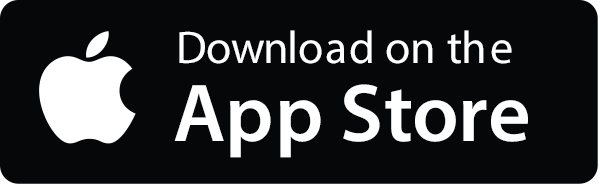
