Recent advancements in artificial intelligence (AI) can significantly improve radiologists’ workflow, improving efficiency and diagnostic accuracy. Current AI applications within spine imaging are approved to accelerate image acquisition time, improve MR imaging quality, triage studies with urgent findings, and aid with image interpretation and report generation. Radiologists should stay up to date on the latest AI advancements and know how to use these tools to improve their own practice. We review current practical applications of AI as well as cutting edge research for future workflow integration.
Key points
- •
Review cutting-edge artificial intelligence (AI) research in spine imaging.
- •
Examine current practical applications of AI in image acquisition, computer aided diagnosis, and report generation.
- •
Explore the future directions of AI in spine imaging.
Introduction
Spinal imaging is a large point of interest for diagnostic imaging advancements, particularly given the prevalence and the disabling nature of spinal pathology. According to the World Health Organization, low back pain is the leading cause of disability worldwide. Recent advancements in artificial intelligence (AI) have allowed for improved workflow efficiency and diagnostic accuracy of spinal imaging. In the clinical setting, AI is a relatively broad term that encompasses any task performed by a computer, which typically requires human intelligence, such as image protocoling and interpretation. Two long standing forms of AI are computer aided detection (CADe) and computer aided diagnosis (CADx); both involve the use of computer software to assist the radiologist and improve accuracy. , These algorithms rely on the original dataset and can only be improved with the addition of more datasets; the more recent concept of true AI , which involves machine learning, deep learning, and neural networks, also use datasets but are less rigid and can incorporate autonomous learning and do not require explicit programming of each step. True AI has been increasingly incorporated into diagnostic imaging for a variety of purposes. In this article, we will discuss the cutting-edge applications of AI in spine imaging, including image acquisition, study triaging, report generation, and diagnosis.
Current clinical artificial intelligence applications
Imaging Protocoling
Image protocoling can take up a significant portion of a radiologist’s workflow. Interruptions by clinicians and technologists to clarify protocols can result in frequent task-switching and can increase the rate of diagnostic error. Machine learning has the potential to streamline the image protocol workflow, and in some cases bypass the need for radiologist approval for routine cases. By analyzing clinical indications and patient data, these tools can recommend appropriate imaging protocols, reducing the burden on radiologists and minimizing the risk of errors or suboptimal protocols.
One study used natural language processing and deep-learning algorithms to protocol neuroradiology MR imagings and showed a 95% concordance of AI protocols compared to those from a neuroradiologist. Another more generalized study used AI to categorize studies into high certainty cases that would not require a radiologist approval for protocoling, and a category for radiologist review. The AI categorized 69% of studies as high certainty, and auto protocolled these studies, while sending the rest to a radiologist for review. Within the high certainty cohort, 2 independent radiologists determined that the AI selected inferior protocols in 0.6% of cases, whereas the error rate of radiologists of selecting a clinically inferior protocol was 0.5%. Both studies used a combination of free text from the clinical indication and select data points from the patient’s chart to train the AI.
Studies thus far using AI to predict imaging protocols have been retrospective in nature. Algorithms were trained using data from a single health center. Generalizability remains a challenge as sequence selection within an MR imaging protocol can vary between practices, and the amount of information listed within the indication can vary greatly. However, these studies show promise for decreasing the burden of radiologists for straightforward protocols and directing their attention to more complicated cases.
Image Acquisition/Acceleration
One of the largest trade-offs in image acquisition is time (and radiation dose for computed tomography [CT] imaging) for image quality. Reduced scan time is associated with a reduced signal to noise ratio and reduced image quality subsequently reduces diagnostic accuracy. To improve efficiency, AI has been implemented to improve postacquisition image quality via deep-learning algorithms; this allows for reduction of scan time and increased patient throughput without appreciable losses in signal to noise ratio or diagnostic accuracy. Additionally, motion artifact is a common cause of image degradation and subsequently repeat imaging. Andre and colleagues estimated the cost of repeat imaging to be between $115,000 per scanner per year. Reduced imaging duration would improve patient comfort and subsequently motion artifact, allowing for cost savings and an overall improved patient experience.
Digital imaging and communications in medicine based postprocessing/K-space based reconstruction
Multiple commercially available deep-learning reconstruction algorithms have been introduced in order to optimize image acquisition by augmenting image quality. K-space based deep-learning algorithms are scanner dependent and are performed directly on the MR imaging scanner’s workflow; digital imaging and communications in medicine (DICOM)-based deep-learning algorithms are applied after image acquisition on an offsite server. Yang and colleagues tested 2 different methods, DICOM-based postprocessing and k-space based reconstruction, and observed reduced scan times for both methods in multiple clinical applications. In addition to reducing scan times, deep-learning image reconstruction algorithms can be applied to improve image quality in low-field MR imaging (below 1T), which has traditionally been limited by low signal to noise ratio and longer scan times. Schlict and colleagues used an advanced deep-learning based image reconstruction algorithm, along with a 0.55 T low-field MR imaging, to obtain a higher signal to noise ratio and up to a 44.4% reduction in scan time on lumbar spine MR imaging. Additionally, Almansour and colleagues demonstrated an approximately 70% reduction in acquisition time using a deep-learning algorithm, which augmented the turbo-spin-echo (TSE) acquisition; despite the drastic reduction in acquisition time, the deep-learning reconstructed images provided excellent image quality interchangeable with the standard TSE acquisition.
Synthetic computed tomography (CT)
Another point of interest for AI applications in spinal imaging involves the synthesis of CT-like MR images ( Fig. 1 A–C ). Bony spinal pathology is often better assessed via CT imaging; for example, axial spondyloarthritis involves bony changes and the presence of bony structural damage and formation of syndesmophytes is best assessed on CT imaging. Willesen and colleagues compared a synthetic AI-generated CT (obtained from MR imaging) sequences to the traditional low dose CT used for evaluation of bony changes and found synthetic CT to be of higher sensitivity and specificity compared to radiography. Additionally, the generation of CT-like images from spine MR imaging eliminates any potential ionizing radiation and has been shown to provide qualitatively accurate images that can be used for preoperative planning. Implementation of synthetic AI-generated CT sequences would additionally reduce time and cost from the preoperative planning standpoint, as 1 examination can ideally provide the same amount of information as 2 examinations.
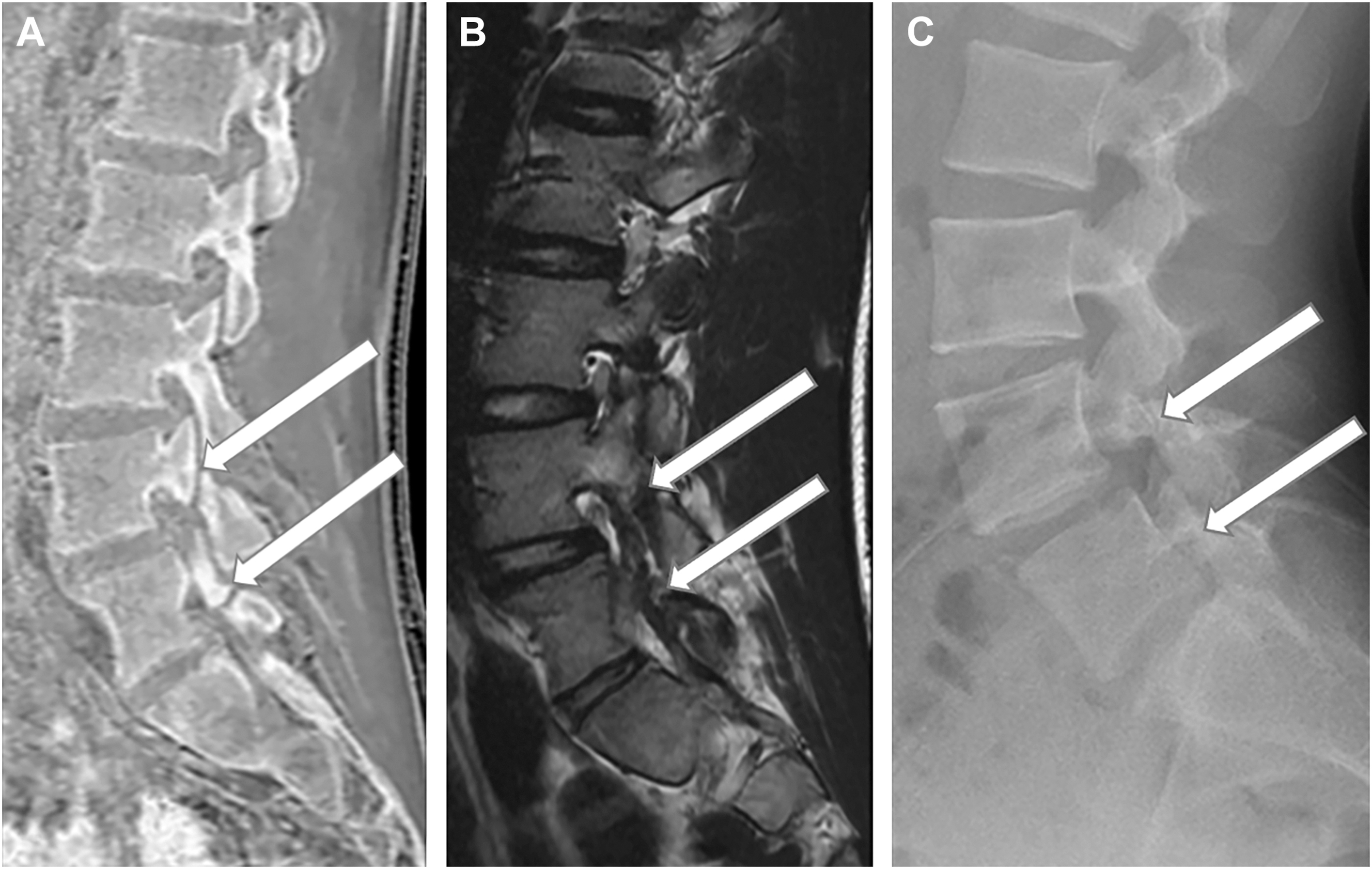
Synthetic short tau inversion recovery
In addition to generating synthetic CT images, AI can be used to generate short tau inversion recovery (STIR) sequences, which are often in the clinical protocol for spine imaging ( Figs. 2 and 3 ). STIR imaging highlights fluid-filled regions (ie, edema and inflammation) and suppresses fat signal, allowing for increased contrast resolution and improved diagnostic accuracy of bone pathology. Tanenbaum and colleagues analyzed the validity of DICOM-based deep-learning STIR synthesis and found that synthetic STIR spine MR images were diagnostically interchangeable with acquired STIR. The incorporation of synthetic STIR imaging can significantly improve imaging workflow and allow for faster and more comfortable spine MR imaging.
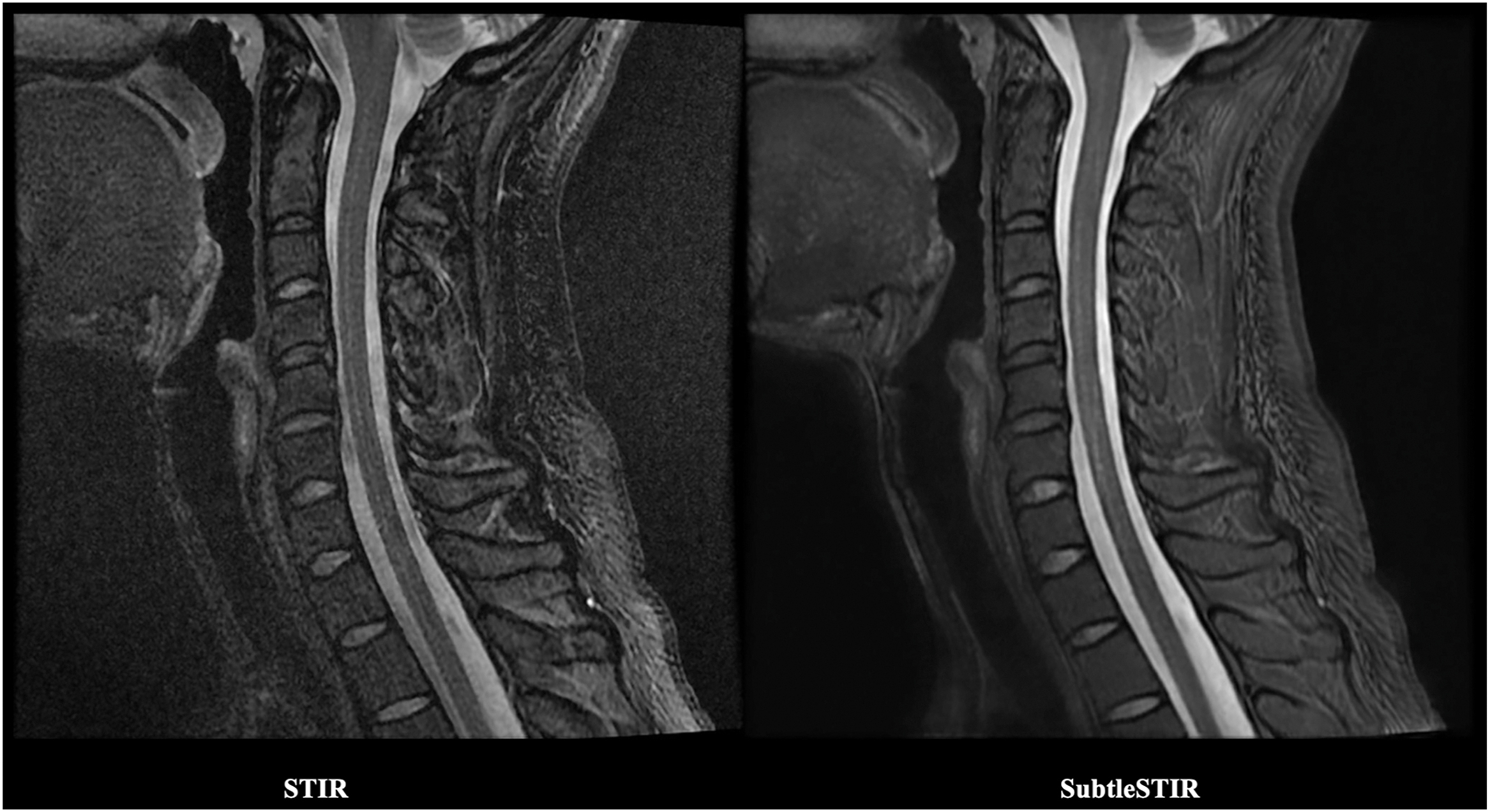
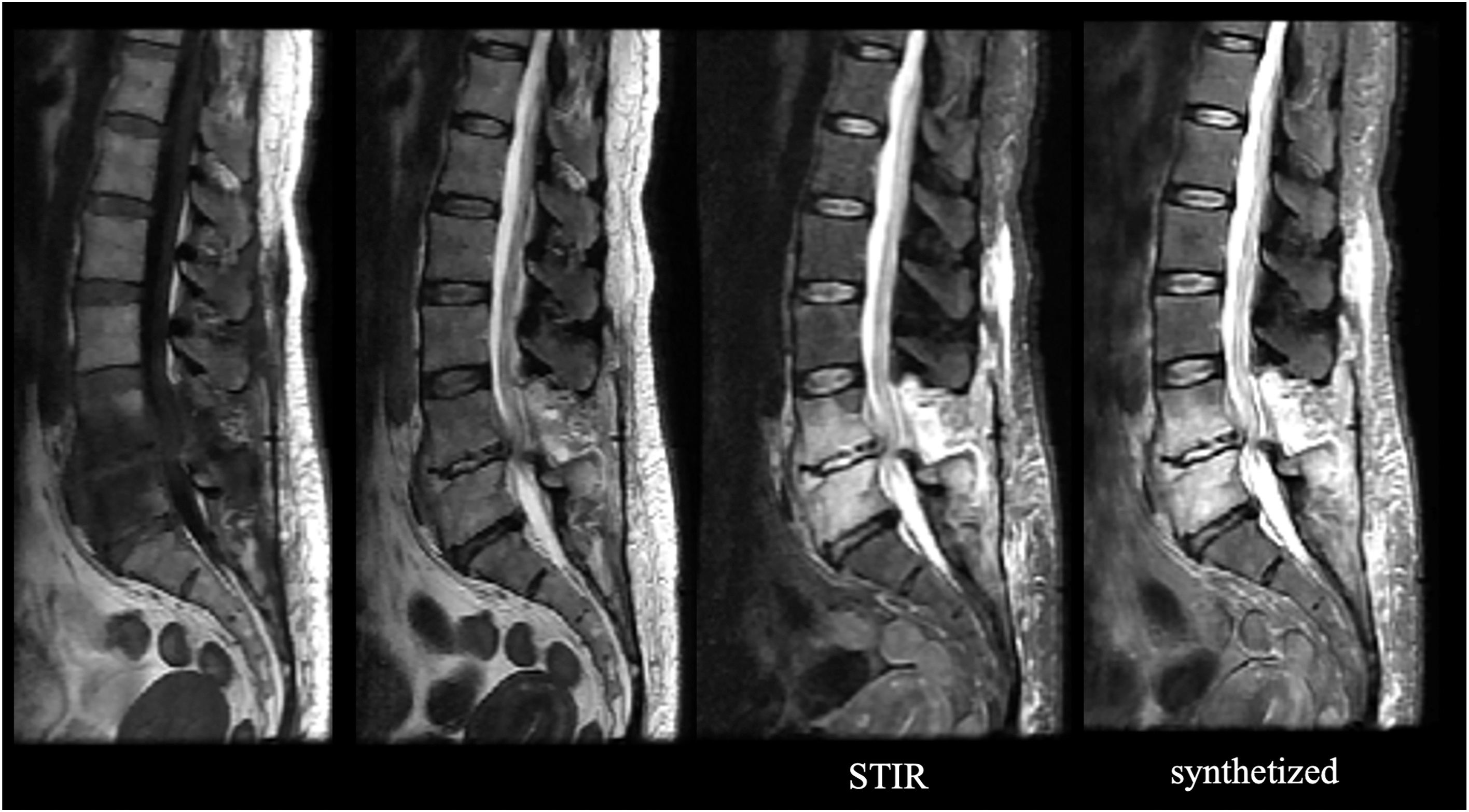
Image Interpretation
Image interpretation is one of the largest growing fields of research in spine AI; the development of pathology-specific algorithms allows for improved diagnostic accuracy. Qualitative and quantitative AI can serve different functions such as detection, diagnosis, and triaging. The previously mentioned CADe and CADx software has already had widespread incorporation into the radiologist workflow. The more recent application of machine learning, deep-learning, and neural networks has allowed for improved detection/diagnosis of spinal pathology.
Spine labeling and segmentation
Quantitative analysis of the spine often involves spine labeling and spine segmentation. Spine labeling identifies the various vertebral body levels and localizes them relative to each other, while spine segmentation involves identifying and delineating the boundaries of spinal structures (ie, vertebral bodies and the spinal canal). Both spine labeling and segmentation are critical steps to detect and diagnose underlying spinal pathology such as vertebral compression fractures, spinal canal stenosis, and scoliosis.
Although several different spine labeling and segmentation algorithms have already been introduced (some of which are commercially available), further developments using AI have demonstrated improved accuracy of labeling and segmentation. , Windsor and colleagues employed a neural-network-based detection and labeling pipeline, which demonstrated a 98.1% detection rate and a 96.5% identification rate with spinal labeling. The algorithm was further improved with the incorporation of a separate transformer-based neural network, which was capable of performing radiologic grading of disc degenerative changes, endplate defects, and herniation ( Fig. 4 A). , The latter development, in conjunction with a language model trained algorithm, can automate the process of grading spinal degenerative changes and producing a radiologic report ( Fig. 4 B).
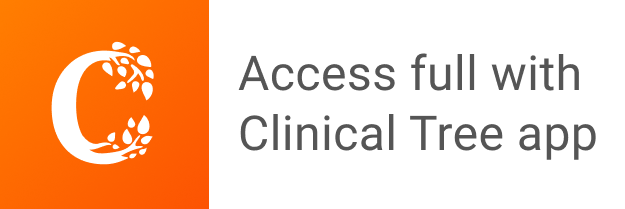