Abstract
Objective
To perform the first known investigation of differences between real-time and offline B-mode and short-lag spatial coherence (SLSC) images when evaluating fluid or solid content in 60 hypoechoic breast masses.
Methods
Real-time and retrospective ( i.e. , offline) reader studies were conducted with three board-certified breast radiologists, followed by objective, reader-independent discrimination using generalized contrast-to-noise ratio (gCNR).
Results
The content of 12 fluid, solid and mixed ( i.e. , containing fluid and solid components) masses were uncertain when reading real-time B-mode images. With real-time and offline SLSC images, 15 and 5, respectively, aggregated solid and mixed masses (and no fluid masses) were uncertain. Therefore, with real-time SLSC imaging, uncertainty about solid masses increased relative to offline SLSC imaging, while uncertainty about fluid masses decreased relative to real-time B-mode imaging. When assessing real-time SLSC reader results, 100% (11/11) of solid masses with uncertain content were correctly classified with a gCNR<0.73 threshold applied to real-time SLSC images. The areas under receiver operator characteristic curves characterizing gCNR as an objective metric to discriminate complicated cysts from solid masses were 0.963 and 0.998 with real-time and offline SLSC images, respectively, which are both considered excellent for diagnostic testing.
Conclusion
Results are promising to support real-time SLSC imaging and gCNR application to real-time SLSC images to enhance sensitivity and specificity, reduce reader variability, and mitigate uncertainty about fluid or solid content, particularly when distinguishing complicated cysts (which are benign) from hypoechoic solid masses (which could be cancerous).
Introduction
Ultrasound is an important supplement to mammography, which has long been the standard screening method for breast cancer [ ]. However, the effectiveness of mammography is compromised in women with dense breast tissue and ultrasound also has limitations [ ]. Although ultrasound helps to visualize lesions in mammographically dense breasts, its high false-positive rates lead to unnecessary biopsies and follow-up procedures for benign fluid-filled cysts [ , ]. In the American College of Radiology Imaging Network 6666 trial, more false positives were seen with ultrasound with increased breast density (e.g., 10.2% false positives with <25% density, increasing to 14.4% false positives with >80% breast density) [ ].
False positives with ultrasound B-mode imaging are often caused by image artifacts such as acoustic clutter, which is partially responsible for similarities in the appearance of complicated cysts and hypoechoic solid masses [ , ]. Acoustic clutter is caused by multi-path acoustic interactions, phase aberration and off-axis scattering [ ], and is particularly problematic in acoustically heterogeneous breast tissue.
Harmonic imaging reduces acoustic clutter by leveraging the non-linear propagation of acoustic waves in biological tissues to minimize acoustic reverberations, suppress side and grating lobes and produce narrower beamwidths, offering additional advantages over traditional fundamental B-mode imaging, such as improved organ visualization and enhanced lateral resolution [ ]. In breast imaging, harmonic imaging improves lesion characterization and margin assessment, particularly when differentiating fluid-filled from solid masses [ , ]. However, the effectiveness of harmonic imaging can be influenced by tissue composition, with its benefits diminished in dense (as opposed to fatty) tissue [ , ]. Additional challenges associated with harmonic imaging in dense breast tissue include increased shadowing and reduced penetration depths at higher frequencies [ ].
Alternatives to harmonic imaging include shear wave imaging [ ], strain imaging [ ], quantitative ultrasound [ ] and ultrasound tomography [ , ]. However, these approaches tend to minimize many of the historical benefits of ultrasound imaging. For example, the accuracy of shear wave and strain imaging depends on loading conditions introduced by the probe pressure to maintain acoustic coupling, and shear wave imaging additionally requires system power specifications that are capable of large acoustic outputs [ ]. Quantitative ultrasound requires careful calibration methods [ ], and ultrasound tomography requires dedicated hardware with high channel counts and with less portability than traditional ultrasound systems [ ].
Coherence-based imaging, particularly short-lag spatial coherence (SLSC) imaging [ ], is promising for ultrasound imaging of dense breasts [ , ]. This technique is known to reduce acoustic clutter while maintaining many of the traditional ultrasound imaging benefits. For example, SLSC imaging does not rely on loading conditions, necessitate custom calibration phantoms nor require custom hardware with reduced portability. In addition, the sensitivity and specificity of coherence-based imaging can outperform clinical elastography techniques [ ].
SLSC images are based on local measurements of the spatial coherence of backscattered echoes rather than the amplitude of backscattered echoes that is the fundamental basis for other ultrasound image formation methods ( e.g. , B-mode, harmonic imaging, shear wave imaging, quantitative ultrasound, ultrasound tomography). Initially demonstrated as a technique to reduce acoustic clutter and improve ultrasound image quality in clinical applications such as thyroid [ ], cardiac [ , ], fetal [ , ], liver [ ] and needle [ ] imaging, SLSC has clear potential to differentiate hypoechoic solid from fluid breast masses [ ]. This differentiation is possible because solid masses have similar spatial coherence relative to surrounding tissue, whereas fluid masses have lower spatial coherence, which assists with complicated cyst distinction [ , ].
In a reader study with five board-certified breast radiologists, a modification to SLSC imaging termed robust short-lag spatial coherence (R-SLSC) [ ] decreased uncertainty regarding fluid-filled mass content from 47.5% to 15.8%, which decreased the percentage of fluid-filled masses recommended for biopsy from 43.3% to 13.3% [ ]. However, there was inter-reader variability with moderate agreement among the five readers when assessing the sensitivity of detecting fluid-filled masses. To reduce subjectivity, objective metrics such as contrast, contrast difference, lag-one coherence, coherence length and generalized contrast-to-noise ratio (gCNR) were investigated [ , ]. Most recently, gCNR with a pre-defined threshold of 0.73 produced similar sensitivity and greater specificity compared to that of the previously prevailing lag-one coherence metric, when applied to SLSC and R-SLSC images [ ]. SLSC imaging was also superior to harmonic B-mode imaging when making this objective distinction between fluid and solid mass contents [ ]. Therefore, gCNR applied to SLSC images has the greatest potential to objectively improve the distinction between fluid and solid masses [ ].
The coherence-based breast imaging results summarized above [ ] were obtained through offline analyses conducted outside of the patient examination room. With the development of real-time GPU SLSC implementations [ ] and deep learning solutions [ ] clinical deployment is feasible, with R-SLSC imaging requiring more computational steps to implement in comparison to SLSC imaging. There are also known image quality trade-offs between real-time and offline SLSC implementations due to the signal-processing shortcuts required for GPU implementations [ ]. We are primarily interested in evaluating real-time SLSC approaches, with the understanding that findings can potentially be translated to more advanced SLSC techniques with more computing resources in future implementations.
A preliminary study from our group [ ] deployed real-time SLSC imaging on 47 hypoechoic breast masses, resulting in three radiologists correctly classifying four complicated cysts as Breast Imaging-Reporting and Data System (BI-RADS) category 2 in the patient examination room, whereas one was uncertain with B-mode imaging and two were recommended for follow-up (BI-RADS 3) or biopsy (BI-RADS 4) with B-mode imaging. Although we anticipate greater reader variability [ ] with more masses included, the previously promising results obtained with the objective gCNR metric applied to SLSC images created after patient exams were completed (i.e., offline) [ ] are expected to successfully translate to similarly positive outcomes when gCNR is applied to SLSC image screenshots acquired during real-time acquisitions. This improvement is expected despite known differences introduced by the real-time GPU signal-processing shortcuts.
The purpose of the study herein is to evaluate potential differences between gCNR results obtained with real-time and offline SLSC images with respect to reader classifications of hypoechoic masses. A secondary objective is to determine the extent to which real-time SLSC imaging and gCNR applied to either real-time B-mode or SLSC images can assist with uncertain B-mode evaluations of mass content. To achieve these objectives, we conducted a reader study comparing offline and real-time SLSC and B-mode images, and subsequently evaluated the performance of gCNR as an objective metric when applied to real-time screenshots and images that were beamformed offline.
Methods
Proposed process
We contrast the current real-time process in cases of uncertainty with our proposed process, which incorporates SLSC and gCNR, as summarized in Figure 1 . First, we acknowledge that traditional B-mode breast ultrasound imaging is expected to be sufficient in some cases ( e.g. , simple cysts [ , ] or malignant masses with highly suspicious imaging features such as irregular shape and spiculated margins [ ]). With our proposed process, viewing SLSC images may be sufficient to determine solid or fluid content in cases of traditional uncertainty ( e.g. , complicated cysts) [ , , , ]. If a reader remains uncertain about fluid or solid mass contents after viewing SLSC images, we propose a follow-up calculation of the gCNR of the SLSC images. With the outcome of this gCNR calculation provided, it is possible that there may be some residual uncertainty ( e.g. , due to borderline cases near the chosen gCNR threshold, lack of trust of new technology, auxiliary image features of concern, the clinical history of the patient, etc.). In these cases of uncertainty, it would be reasonable to proceed with the typical protocol that is already familiar to the radiologist (see last step in Fig. 1 ).
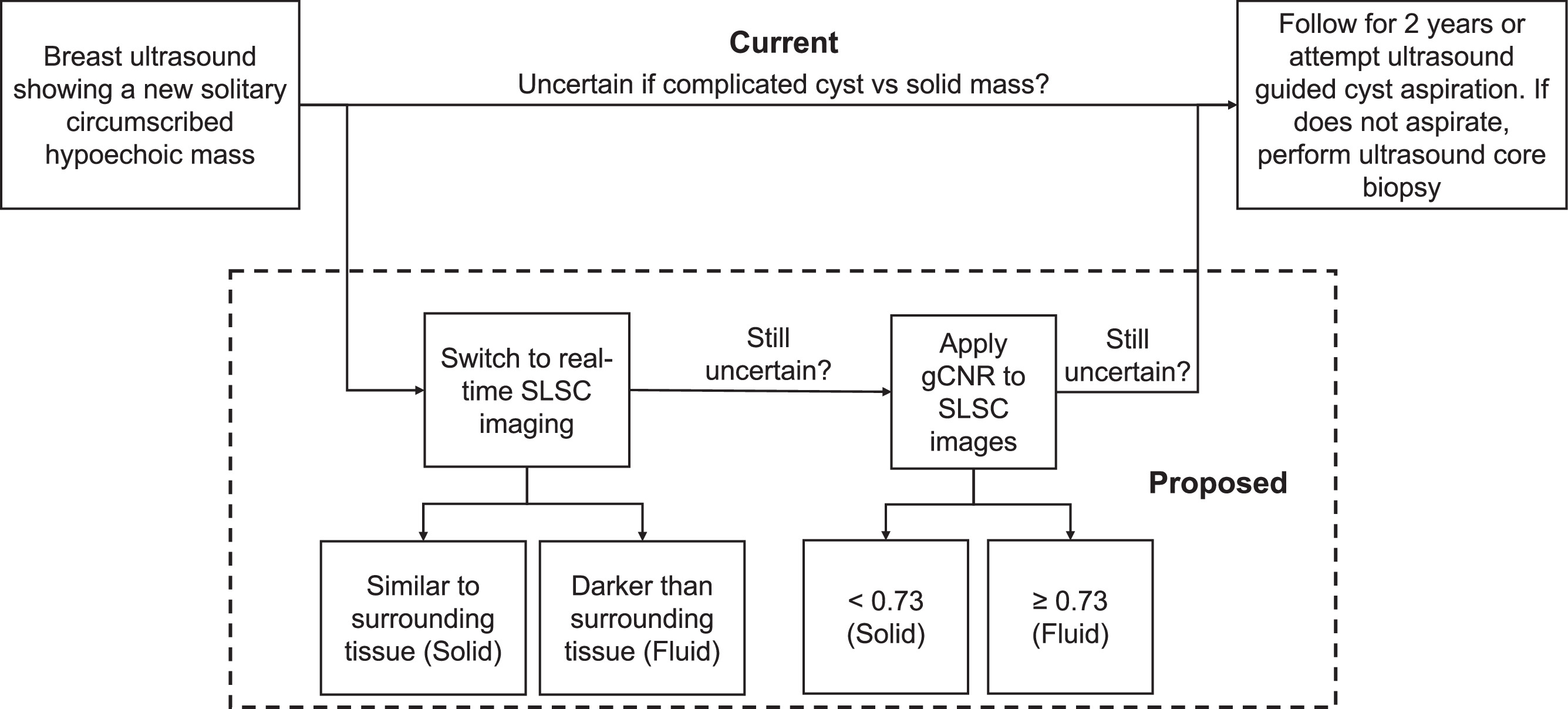
Study population
Patients who met the following four inclusion criteria were recruited: (i) adult females (>18 y), (ii) English speakers, (ii) not pregnant and (iv) scheduled for ultrasound-guided core-needle biopsy, aspiration or follow-up of at least one breast mass. A total of 44 patients (mean Β± standard deviation age: 51 Β± 17 y) with 82 masses in total were enrolled after obtaining written informed consent. Sixty of the 82 masses were eligible for analysis, including five simple cysts present in addition to the masses scheduled for aspiration or biopsy. As documented in Figure 2 , masses were excluded from analysis based on pathological, imaging or physical characteristics ( i.e. , BI-RADS 3 masses have no pathological results, real-time SLSC imaging was required, shallow masses are not suitable for SLSC imaging [ ]). When the differential diagnosis included a cyst, ultrasound-guided aspiration was initially attempted, resulting in a complicated cyst classification upon successful aspiration. Simple cysts were classified without aspiration based on clinical ultrasound B-mode features. Otherwise, the results of each core-needle biopsy served as the ground truth for mass classification. This study was approved by the Johns Hopkins Institutional Review Board (protocol no. IRB00127110).
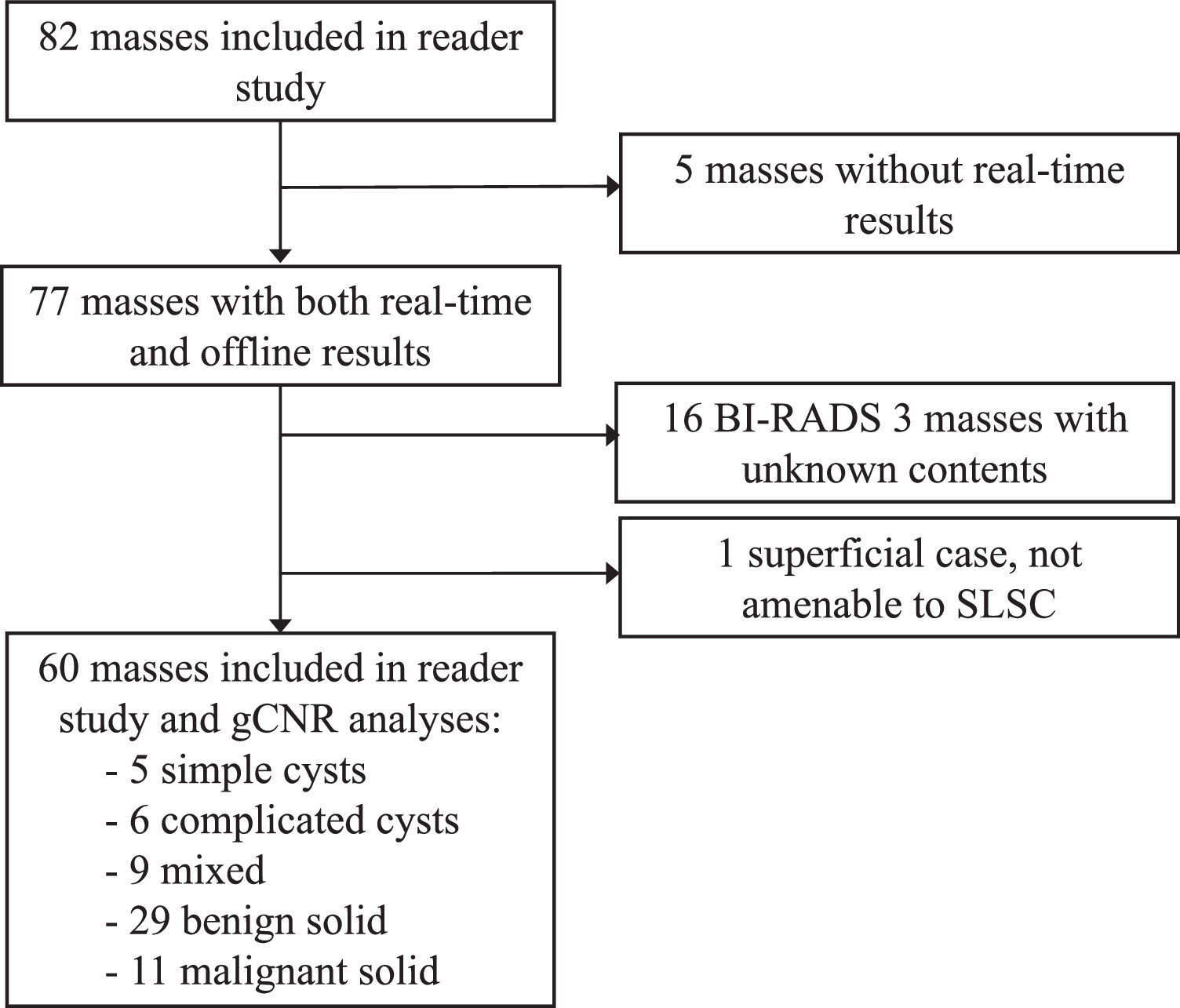
Data acquisition and processing
Each patient was imaged by a board-certified breast radiologist co-author (E.A., K.M. or E.O.). An Alpinion ECUBE12R research ultrasound scanner (Alpinion, Seoul, South Korea) connected to a 128-element, L8-17 probe with 64 receive elements, 12.5 MHz center frequency and 40 MHz sampling frequency was employed to acquire raw ultrasound radiofrequency (RF) channel data with 256 receive scan lines per image. The focus of transmitted beams was located within 0-1 cm of the mass center, as selected by the radiologist co-author performing the scan. Raw RF ultrasound data of two orthogonal views per mass were acquired and saved for post-procedure processing. In addition, clinical screenshots of real-time B-mode images (processed using proprietary information) and real-time SLSC images (processed using the details reported in [ , ], with additional proprietary processing information added to the real-time display), each located within the same buffer as the RF data, were concurrently acquired. These data were acquired from January 24, 2023 through October 5, 2023.
To process acquired RF data offline and create offline B-mode images, a standard delay-and-sum beamformer was implemented. To form offline SLSC images [ , , , ], delays were first applied to the acquired RF data to account for time-of-arrival differences. Then, data within correlation kernel lengths equal to one wavelength were cross-correlated to create coherence functions, <SPAN role=presentation tabIndex=0 id=MathJax-Element-1-Frame class=MathJax style="POSITION: relative" data-mathml='R^’>π
ΛR^
R ^
, for each lateral and axial position in the image [ ]:
R^(m)=1Nβmβi=1Nβmβn=n1n2si(n)si+m(n)βn=n1n2si2(n)βn=n1n2si+m2(n)
Rsl=β«1MR^(m)dmββm=1MR^[m]
Contrary to the offline processing method for SLSC images, eqns (1) and ( 2 ) were manipulated to fully utilize the benefits of the GPU embedded in the ultrasound system and form real-time SLSC images. In particular, as described in previous work [ , ], the GPU SLSC implementation first computes individual correlation ( C i,i ) and autocorrelation ( C i,i ; and ( C i,j ) terms for scanline x , axial sample z , and elements i and j separated by m , given by the following equations:
Ci,j(z,x,m)=βi=1Niβmsi(z,x)si+m(z,x)*,
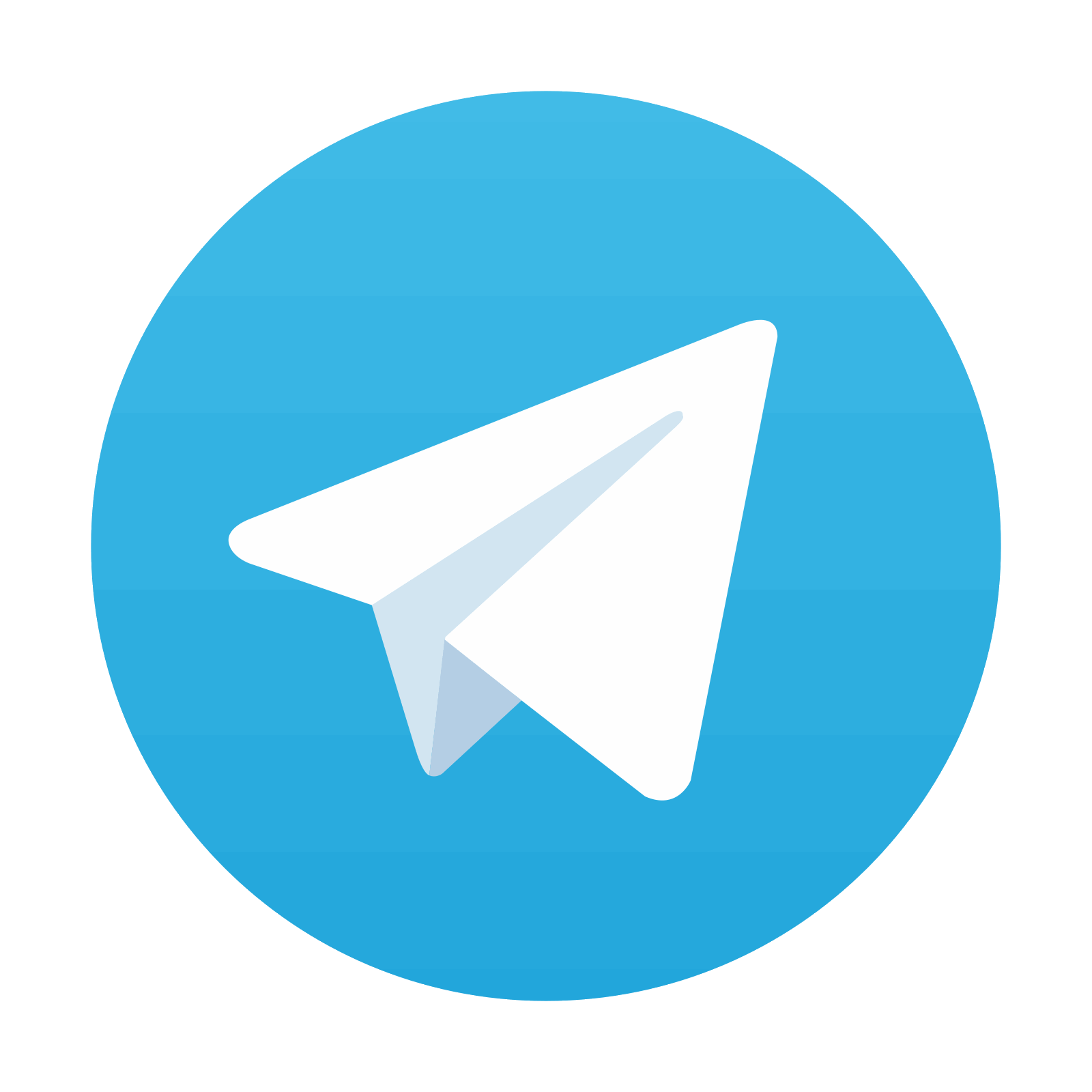
Stay updated, free articles. Join our Telegram channel

Full access? Get Clinical Tree
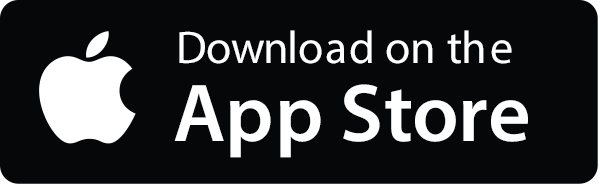

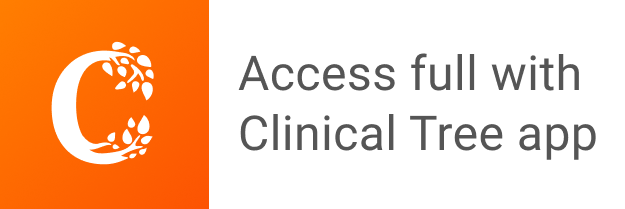