Abstract
Background
Dual-energy CT (DECT) is a non-invasive way to determine the presence of monosodium urate (MSU) crystals in the workup of gout. Color-coding distinguishes MSU from calcium following material decomposition and post-processing. Most software labels MSU as green and calcium as blue. There are limitations in the current image processing methods of segmenting green-encoded pixels. Additionally, identifying green foci is tedious, and automated detection would improve workflow. This study aimed to determine the optimal deep learning (DL) algorithm for segmenting green-encoded pixels of MSU crystals on DECTs.
Methods
DECT images of positive and negative gout cases were retrospectively collected. The dataset was split into train ( N = 28) and held-out test ( N = 30) sets. To perform cross-validation, the train set was split into seven folds. The images were presented to two musculoskeletal radiologists, who independently identified green-encoded voxels. Two 3D Unet-based DL models, Segresnet and SwinUNETR, were trained, and the Dice similarity coefficient (DSC), sensitivity, and specificity were reported as the segmentation metrics.
Results
Segresnet showed superior performance, achieving a DSC of 0.9999 for the background pixels, 0.7868 for the green pixels, and an average DSC of 0.8934 for both types of pixels, respectively. According to the post-processed results, the Segresnet reached voxel-level sensitivity and specificity of 98.72 % and 99.98 %, respectively.
Conclusion
In this study, we compared two DL-based segmentation approaches for detecting MSU deposits in a DECT dataset. The Segresnet resulted in superior performance metrics. The developed algorithm provides a potential fast, consistent, highly sensitive and specific computer-aided diagnosis tool. Ultimately, such an algorithm could be used by radiologists to streamline DECT workflow and improve accuracy in the detection of gout.
1
Introduction
Imaging is an integral component in the workup of gout. Specifically, dual-energy CT (DECT) is a non-invasive means of determining the presence of monosodium urate (MSU) crystals . DECT with material decomposition has a high sensitivity and specificity for MSU crystals [ , ], and a definitive result has been shown to correlate with a decrease in the use of confirmatory joint aspirations . Following material decomposition and with post-processing, pixels with x-ray attenuation consistent with MSU are differentiated from calcium using color-coding. For example, one commercial post-processing software (Syngo.via, Siemens Healthcare) labels MSU pixels as green and calcium as blue ( Fig. 1 ).
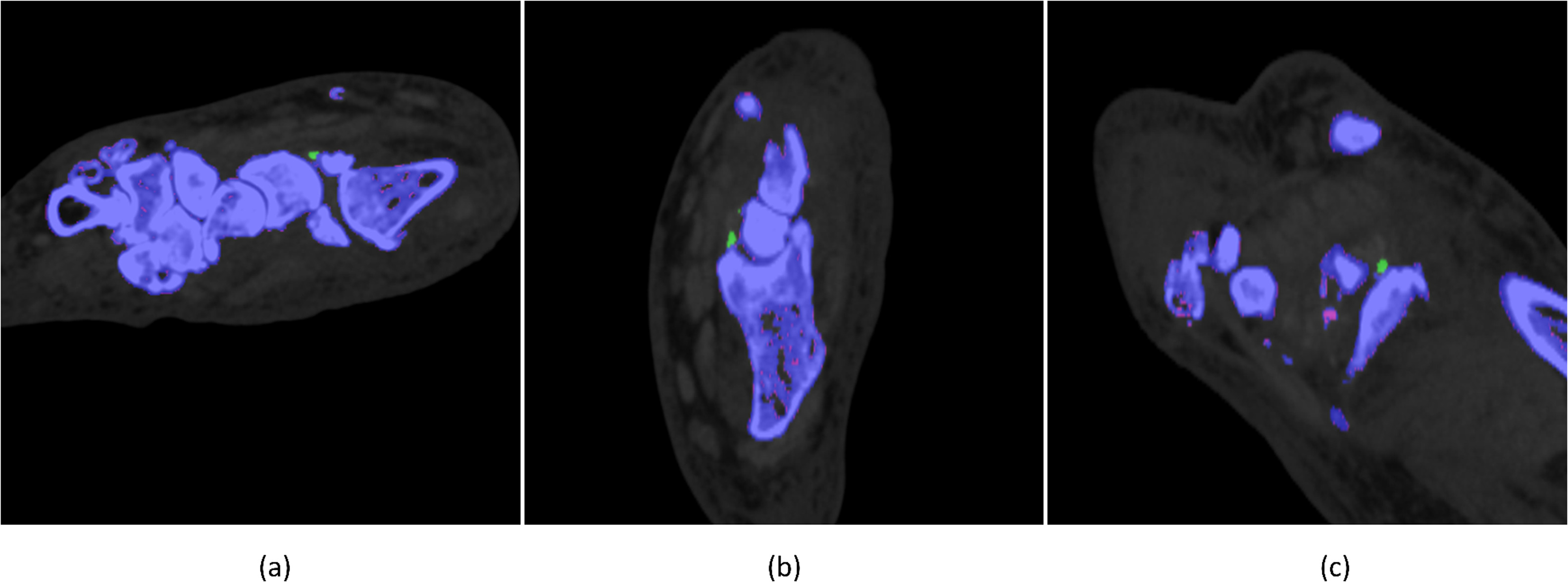
Diagnostic accuracy of DECT is high, however careful review and evaluation of the post-processed images is necessary, as there are well-known sources of true and false positive green pixelation using this method . Additionally, identifying and evaluating small foci of green pixelation in a large dataset is a tedious diagnostic task for which automated detection would improve workflow. Although it might initially seem that setting a threshold on the green channel in color-encoded images would result in quick segmentation of green-encoded pixels, employing image processing techniques actually leads to numerous false positives and negatives across various thresholds.
Deep learning (DL) algorithms can be utilized for semantic segmentation in medical imaging . However, developing DL algorithms for detection tasks in which the lesion site represents a minuscule fraction of the image set is challenging . For instance, identifying a single pixel of green on a post-processed DECT image for gout detection poses a challenge for DL algorithms given the tiny size of the pixel of interest relative to the large background volume.
Different convolutional neural networks (CNN) and vision transformers (ViT) based Unet architectures have been used for medical image segmentation. While the ViT-based models such as SwinUNETR have superior performance over CNN models such as Segresnet, ViT-based models require larger training sample sizes . Segresnet is an Unet-based DL model that benefits from a variational autoencoder branch and exerts a regularization effect on the encoder, which makes Segresnet a suitable segmentation model when training data is scarce [ , ]. SwinUNETR is another Unet variant that is designed to more completely capture spatial relation information due to its Swin transformers-based architecture. Since both of these architectures achieved benchmark performance in the Brain Tumor segmentation competition, we selected them as representatives of CNN-based and ViT-based models [ , ].
For this study, we sought to identify the optimal DL algorithm for segmentation of green-encoded pixels of MSU crystals on DECTs. This research represents the first step in developing an AI model that can quickly and accurately identify MSU deposits for the radiologist. The goal for such an algorithm is to improve identification of true monosodium urate deposition, potentially enhancing diagnostic confidence and saving time.
2
Methods
Institutional review board approval was obtained for this retrospective study. The requirement for informed consent was waived.
3
Subjects
DECTs acquired between 7/23/2019 and 7/13/2022 were included in this study. Cases were identified from a pre-existing dataset of DECT examinations, with a final diagnosis of gout determined based on imaging findings, joint aspiration, serum uric acid level, and/or clinical evaluation. The cohort of positive cases comprised consecutive participants with a positive diagnosis of gout. The cohort of negative cases comprised consecutive participants with a negative diagnosis of gout.
4
Dual-energy CT images
All patients were scanned on a third generation dual-source CT system (SOMATOM Force, Siemens Healthcare) with tube potentials of 80 kV(tube A) and 150 kV (tube B). Tin prefiltration was applied to the high-energy beam for improved spectral separation. The reconstructed images were analyzed using commercially available software (syngo.via VB30A, Siemens Healthcare). The software uses a material decomposition algorithm to identify uric acid and calcium voxels on the basis of their material-specific behavior under two different x-ray beam energy levels. No IV contrast material was used.
5
Dataset splitting
Participant data were split into training ( N = 28) and hold-out test ( N = 30) sets at the patient level to avoid potential data leakage. This split was determined by the need to ensure a comprehensive and reliable assessment of our model’s performance, particularly to ensure that the results were not influenced by overfitting or limited to specific subsets. Balancing the size of the training and test sets was a key consideration in the design of this study. We split the train set into seven folds (7 groups of 4 patients) using the GroupKfold module from scikit-learn package version 1.2.0 . Cross-validation of the final model was performed on all seven groups to determine robustness of the results .
6
Data preprocessing and annotation
Material decomposed DECT images were acquired in the axial (2-dimensional) plane and presented in a 3-color pixel image: green (MSU deposits), blue (calcium), and black (background). Using pydicom and nibabel packages [ , ], the three-channel color-coded DECT images were transformed from 2-dimensional Digital Imaging and Communications in Medicine (DICOM) format to a three-channel, 3-dimensional format, Neuroimaging Informatics Technology Initiative (NIfTI), a segmentation-compatible format. Three-dimensional color NIfTI images were presented to two fellowship-trained musculoskeletal radiologists (CT and NR) with 8 and 10 years of post-training subspecialty expertise in interpreting DECTs and an in-training fellowship musculoskeletal radiologist (SP). The radiologists independently identified green encoded voxels in each dataset. Annotations were made on image slices with a 3D isotropic brush using ITK-SNAP software version 3.8.0 ( Fig. 2 ).
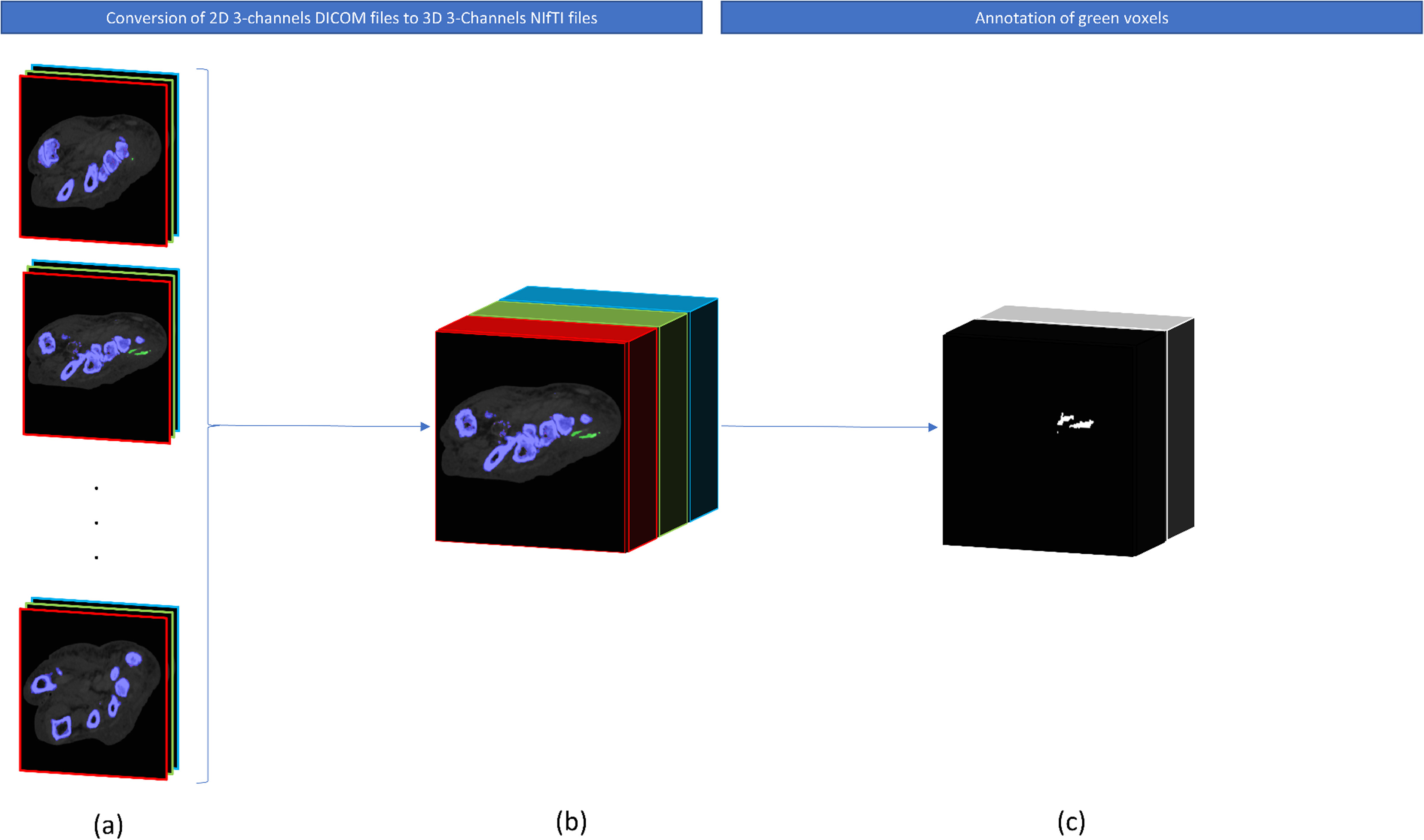
7
Model development for deposit detection
Two Unet-based DL models were trained to automatically segment the green voxels on DECT images: A 3D Segresnet and a SwinUNETR from the MONAI framework. Please see supplementary 1 for detailed model architecture. Both models were trained and the results were compared.
To enhance the model’s generalizability and prevent overfitting, we utilized AdamW as the optimizer with a weight decay of 0.1, which incorporates weight decay as a form of regularization . CosineAnnealing learning rate scheduler with an initial learning rate of 0.001 was used as the learning rate scheduler to avoid local minima . Since MSU deposits can be tiny, we used weighted FocalDiceLoss as the loss function, which is a variation of the Dice loss function that is designed to address the class imbalance in the dataset, with 1 and 100 as weights for the background and deposits, respectively . During training, to increase the number and diversity of training samples for each epoch, we randomly cropped images into four 64 × 64 × 64 patches with 3 to 1 ratios for patches containing deposits and patches without any deposits to reduce the false positive predictions ( Fig. 3 ). This approach generates a large number of diverse patches, which contributes to the model’s performance gain despite the limited number of patients, as the model “sees” different patches in each spot for each patient. Rotation and scaling were utilized as data augmentation techniques to reduce the risk of overfitting and increase model generalizability . During inference, sliding window inference with the size of 128 × 128 × 128 without any data augmentation was utilized (please see the supplementary Table 1 for a detailed description of hyperparameters).
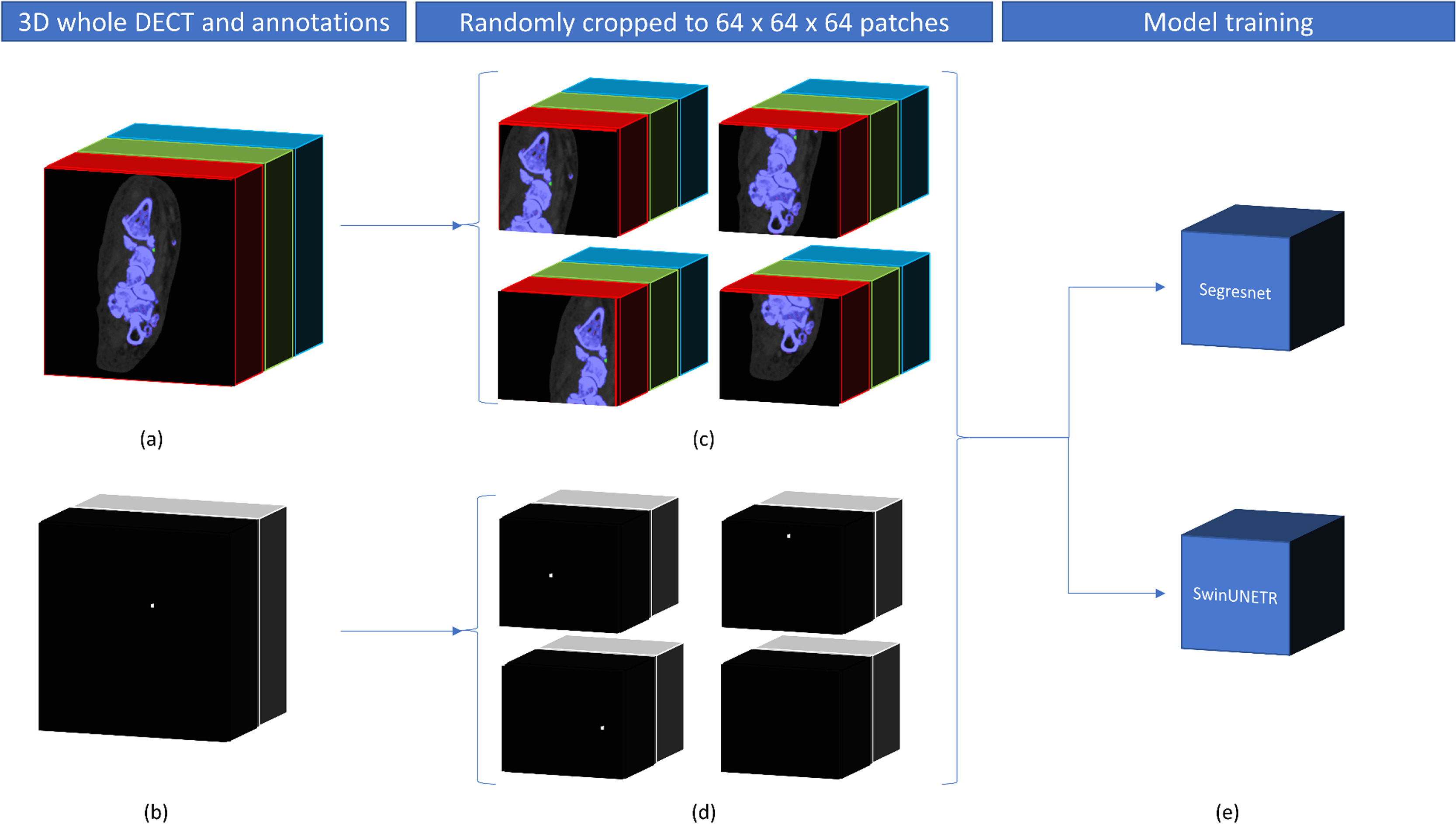
The mean Dice similarity coefficient (DSC) was used to measure the amount of overlap between the ground truth and the predicted masks ( Eq. (1) ) . The DSC of each channel and the average of all channels per fold were reported as the segmentation metric.
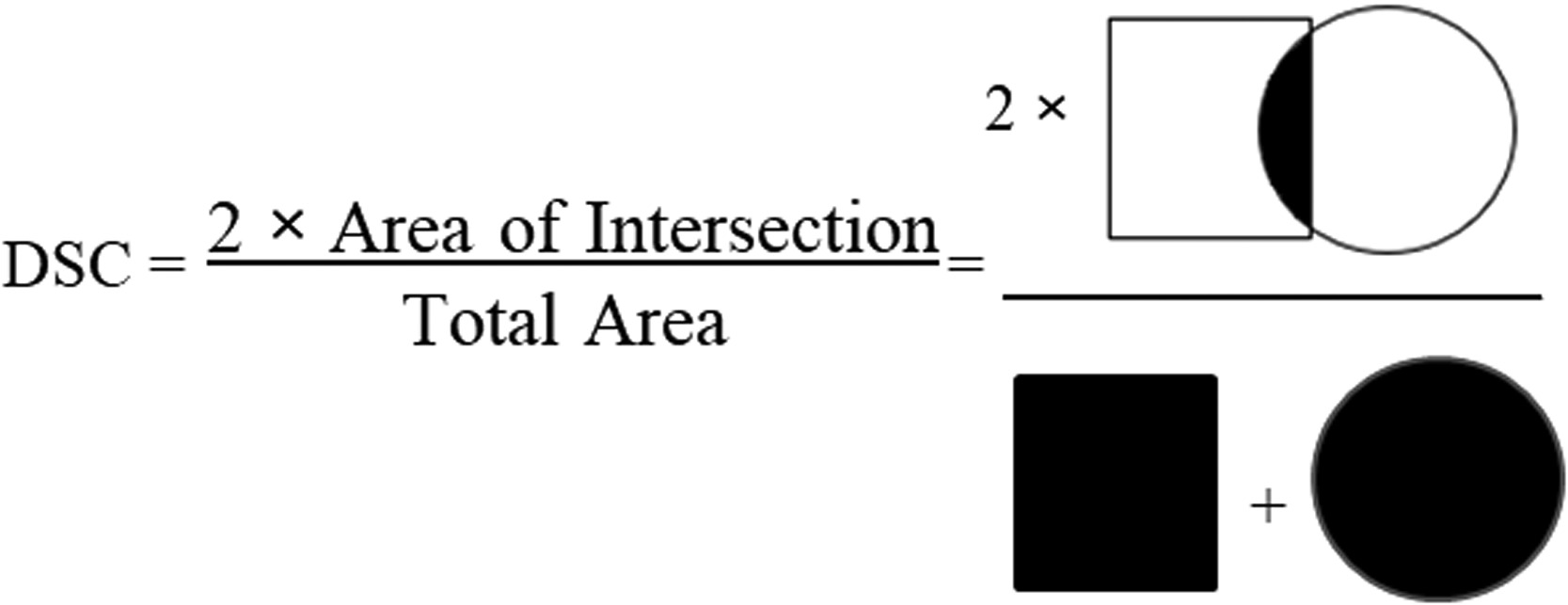
Mean DSC was used as the model selection criteria. To determine the performance of each segmentation model, the DSCs for green pixels and background pixels for each fold were calculated. To increase the model sensitivity and improve the visibility of deposits for radiologists, predicted masks of better-performing model based on cross-validation were expanded to encompass two voxels in each direction. Voxel-level sensitivity and specificity were reported for the model with the highest mean DSC.
The best-performing model on the validation set was selected and run on the hold-out test set. DSCs, pixel-level sensitivity, and specificity were reported.
Due to the paucity of literature on this topic, to set a baseline DSC, we measured the inter-rater DSC of the study MSK radiologists and their intra-rater DSC after a 1 month washout period.
All image processing and model development was performed using Pytorch 1.12.0 and MONAI 0.9.0 on Python 3.10.4 on a GPU cluster of 4 GPUs (NVIDIA A100). All statistical analyses were performed using scikit-learn 1.2.0 on Python version 3.10.4.
8
Results
Fifty-eight subjects who had a DECT exam were included in this study. The median age of subjects was 66.5 years (interquartile range = 32.75). The exams comprised 33 (56.90 %) feet and ankles, 18 (31.03 %) wrists and hands, 5 (8.62 %) knees, and 2 (3.45 %) elbows. Patient demographics are summarized in Table 1 .
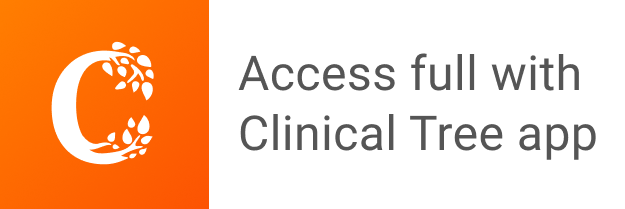