ABSTRACT
Objective
To develop a comprehensive nomogram to predict the histological grading of breast cancer and further examine its clinical significance by integrating both intra-tumoral and peri-tumoral ultrasound radiomics features.
Methods
In a retrospective study 468 female breast cancer patients were analyzed from 2017 to 2020 at the Second Affiliated Hospital of Harbin Medical University. Patients were grouped into high-grade (n = 215) and low-grade (n = 253) categories based on pathological evaluation. Tumor regions of interest were defined and expanded automatically to peri-tumor regions of interest. Ultrasound radiomics features were extracted independently. To ensure rigor, cases were randomly divided into 80% training and 20% test sets. Optimal features were selected using statistical and machine learning methods. Intra-tumor, peri-tumor, and combined radiomics models were constructed. To determine the best predictors of breast cancer histological grading, we screened the features using single- and multi-factor logistic regression analyses. Finally, a nomogram was developed and evaluated for its predictive value in this context.
Results
By applying logistic regression, we integrated ultrasound, clinicopathologic, and radiomics features to generate a nomogram. The combined model outperformed others, achieving areas under the curve of 0.934 and 0.812 in training and test sets. Calibration curves also showed high accuracy and reliability.
Conclusion
A nomogram constructed through the integration of combined intra-tumor–peri-tumor ultrasound radiomics features along with clinicopathologic characteristics exhibited remarkable performance in distinguishing the histologic grades of invasive breast cancer.
Introduction
Based on data from the International Agency for Research on Cancer, a subsidiary of the World Health Organization, breast cancer stands as the most prevalent malignant tumor among women globally, accounting for 11.7% of all cancer cases and posing a substantial threat to women’s overall health [ ]. Prompt diagnosis and treatment are critical in breast cancer management. Histological grading through pathological examination remains the definitive diagnostic method, significantly influencing treatment planning and prognosis assessment in invasive breast cancer [ , ]. Notably, histological grading has been shown to more accurately capture the tumor’s biological behavior and proliferation status compared with other time-dependent factors such as age, tumor size, or lymph node status [ , ]. This prognostic significance has led to its widespread adoption in clinical decision-making. Specifically, higher histological grades correlate with increased neovascularization, poorer differentiation and a higher degree of malignancy. Therefore, pre-operative prediction of tumor histological grade based on imaging features offers significant clinical benefits, enabling the development of personalized treatment plans and improving prognosis [ ].
Ultrasound serves as the preferred modality for breast cancer screening among women in China due to its high resolving power, non-invasive nature and lack of radiation exposure. This approach effectively mitigates the potential oversight of breast masses in patients with dense breast tissue [ ]. Clinical observations have revealed certain similarities in the internal ultrasound images of masses across different grades. Recent research further indicates that alterations in the tumor micro-environment are also present in peri-tumoral tissues, encompassing changes in vascular endothelial growth factor, vascular infiltration, lymphocyte infiltration, mesenchymal reaction and peri-tumoral edema [ , ]. These findings suggest that the peri-tumoral area is influenced by the tumor and potentially harbors valuable diagnostic information.
The peri-tumoral area refers to the interface where the tumor directly interfaces with adjacent normal tissues of the body. It serves as a gateway for nutrients and channels necessary for tumor growth and metastasis, encompassing blood vessels, lymphatic vessels, nerves, fat and various connective tissues. Both the peri-tumor and the tumor influence and constrain the occurrence and progression of the tumor through metabolic, secretory, immune, structural and functional alterations. They exhibit a complex interplay of inter-dependence, mutual promotion, as well as antagonism and conflict [ ]. In the case of invasive breast cancer, the central region of the cancer foci often undergoes atrophy and degeneration due to rapid tumor growth, ischemia and hypoxia, ultimately leading to the formation of fibrotic scars [ ]. This region typically exhibits a low density of cancer cells and reduced proliferation activity. Conversely, the peripheral regions of the cancer foci frequently demonstrate high proliferative activity. This particular growth pattern is now recognized as a pivotal factor in the development and progression of breast cancer.
Current research has delved into the peri-tumor region of various tumor types, including its predictive value in assessing the efficacy of adjuvant chemotherapy in lung adenocarcinoma [ ], reflecting radiotherapy response in predicting metastatic cervical lymph nodes in nasopharyngeal carcinoma [ ] and determining the likelihood of pathological complete response (pCR) following neoadjuvant chemotherapy in esophageal carcinoma [ ]. These studies highlight the significance of the peri-tumor region’s information in evaluating tumor outcomes. However, current research on breast cancer ultrasound primarily emphasizes tumor internals, overlooking the mass margins. Scarce reports address this niche, indicating that imaging radiomics from peri-tumoral tissues may reveal intricate tumor micro-environment heterogeneity and complexity [ ]. This approach may enhance biological tumor behavior assessment, enabling earlier interventions [ ].
The objective of this study was to devise a predictive nomogram for histologic grading of invasive breast cancer, leveraging the intra-tumoral and peri-tumoral imaging radiomics characteristics of breast masses. This nomogram aims to serve as a reliable technical tool for precise pre-operative assessment of histologic grading, thereby offering invaluable imaging insights to facilitate individualized clinical diagnosis and treatment.
Materials and methods
Patient selection
The Medical Ethics Committee of Harbin Medical University’s Second Hospital approved the retrospective study protocol, exempting informed consent. This study included 713 female patients diagnosed with invasive breast cancer from January 2017 to January 2020. Inclusion criteria were histopathologically confirmed invasive ductal carcinoma and availability of complete clinicopathologic data and ultrasound images. Exclusion criteria comprised: (i) prior radiotherapy/hormone therapy or other tumor history, (ii) non-massive breast cancer, (iii) superficial mass location impeding accurate marginal zone delineation and (iv) insufficient image clarity for mass margin determination. Applying the criteria for inclusion and exclusion, 468 patients were ultimately selected. These patients were randomly allocated to two groups in an 8:2 ratio, comprising a training set of 374 patients (175 high-grade, 199 low-grade) and a validation set of 94 patients (40 high-grade, 54 low-grade). The low-grade group encompassed cases with histologic grading of 1–2, while the high-grade group comprised cases with histologic grading of 3 ( Fig. 1 a).
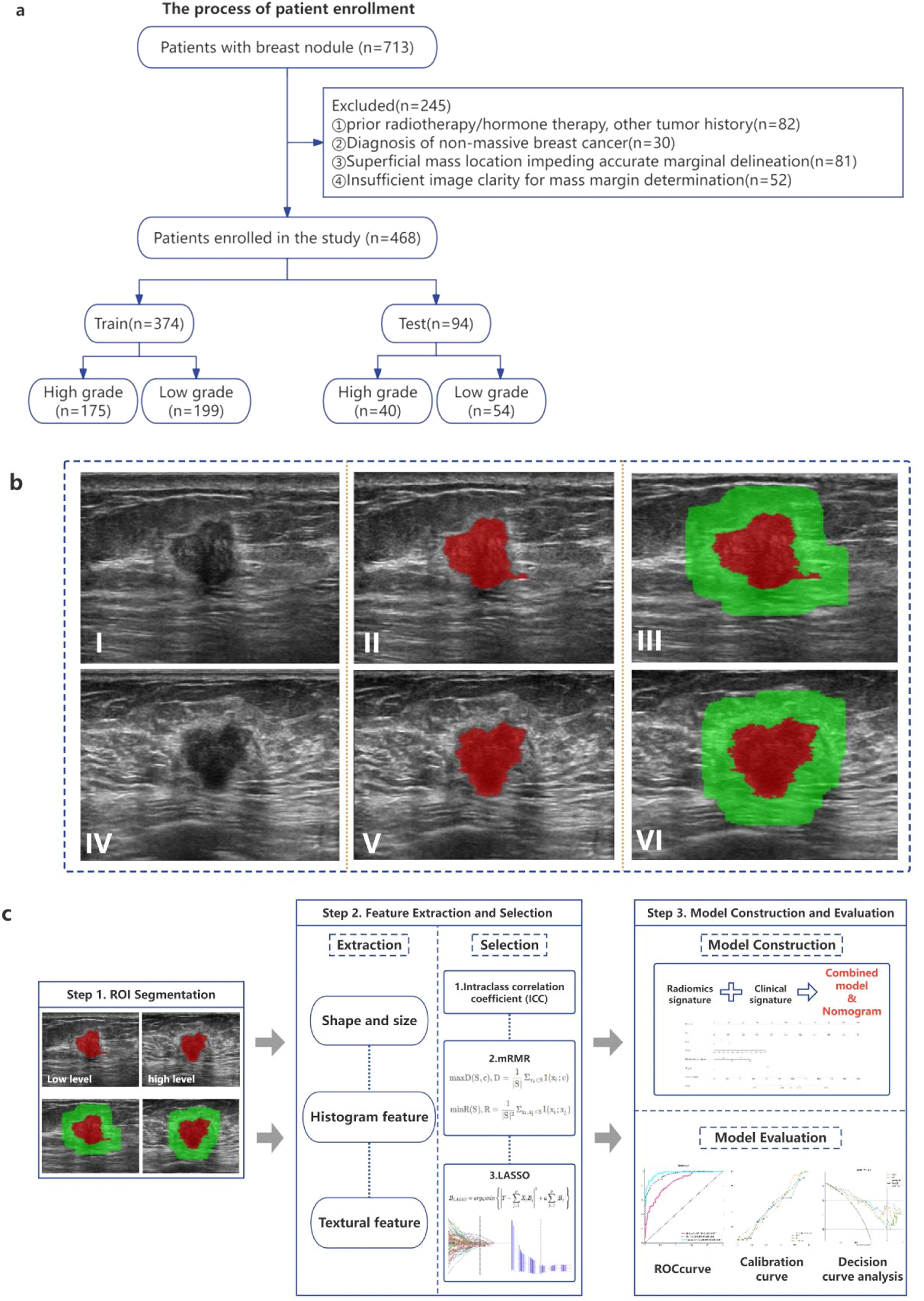
Clinical data and US characteristics
In the context of our study, we carefully assessed age, the maximum diameter of the mass, the presence or absence of nipple and margin infiltration, lymph node metastatic status, and the expression status of key biomarkers such as estrogen receptor (ER), progesterone receptor (PR) and human epidermal growth factor receptor 2 (HER-2). Additionally, we evaluated the Ki-67 proliferation index, which serves as a vital indicator of cellular proliferation in breast cancer and molecular typing, including Luminal A, Luminal B, HER-2 overexpressing, and triple-positive and triple-negative sub-types [ ]. The Ki-67 proliferation index was calculated based on the percentage of malignant cells staining positive for Ki-67, which was categorized according to the St. Gallen International Consensus of Experts, with a Ki-67 score of ≥14% considered as high expression and scores <14% considered low expression. HER-2 immunohistochemical assay results were specifically classified as (3+), (2+), (1+) and (0) [ , ]. Patients with IHC (2+) results underwent HER-2 fluorescence in situ hybridization analysis. HER-2 positivity was confirmed by IHC (3+) or (2+) with HER-2 fluorescence in situ hybridization amplification [ ].
All patients included in the study underwent independent analysis of the mass images by two experienced sonographers, each having over 5 years of diagnostic breast ultrasound experience. Any discrepancies were resolved through consensus. Notably, both sonographers were blinded to the patients’ clinical details and pathological outcomes. Ultrasound characterization adhered to Breast Imaging Reporting and Data System (BI-RADS) criteria [ ]. We retrospectively examined nine routine ultrasound features: maximum mass diameter, shape (regular vs. irregular), orientation (parallel vs. not parallel), margin (indistinct, angular/spiculated, micro-lobulated), boundary (abrupt, halo), echo pattern (complex, hypo-echoic, iso-echoic, very low echo), posterior acoustic features (none, enhancement, shadowing, combined pattern), calcification (present vs. absent) and surrounding tissue changes (none, architectural distortion/duct or skin changes).
For color Doppler evaluation, we adopted the Alder grading method [ ]. Specifically, grade 0 (no blood flow signal within the lesion) and grade 1 (minimal blood flow with one to two punctate or short strip vessels, <1 mm diameter) were considered indicative of minimal flow. Grade 2 signified moderate flow, characterized by one to two branched blood vessels within and around the lesion, exceeding the lesion’s radius. Lastly, grade 3 denoted ample flow, manifesting as more than four blood vessels interconnected or interwoven into a mesh pattern within and around the lesion tissue.
Region of interest outlining
The 2-D ultrasound image’s section with the tumor’s largest diameter was identified, and intra-tumoral regions of interest (ROIs) were precisely delineated along the contours of breast masses on ultrasound images by an experienced physician with over 10 years of diagnostic breast ultrasound expertise using ITK-SNAP software (version 3.8.0). Meanwhile, peri-tumoral ROIs were automatically generated as annular regions by identifying a 50-pixel extension of the intra-tumoral ROI boundaries using Python (version 2.7.13) [ ]. Subsequently, rigorous image normalization and grayscale standardization procedures were implemented to guarantee the consistency and comparability of images captured across diverse ultrasound devices ( Fig. 1 b) [ ].
Feature extraction and selection
Using the Pyradiomics software suite ( http://pyradiomics.readthedocs.io/en/latest/ ), which is compatible with Python 3.0.1, we extracted a range of ultrasound imaging–derived radiomics properties from unprocessed ultrasound images. Central to this work was the extraction of first-order statistics, shape and texture features. The current study identified 3122 distinct features that spanned both intra-tumoral and peri-tumoral domains of each patient’s data.
Subsequent refinement of the extracted ultrasound imaging radiomics features was conducted in a rigorous manner. Initially, a Mann-Whitney U test was implemented to filter out features, retaining only those with statistical significance ( p < 0.05). To mitigate feature redundancy, we computed the Spearman rank correlation coefficient between features, selecting only one feature from any pair exhibiting a correlation coefficient above 0.9. To maximize the relevance between features and categorical variables while minimizing feature-to-feature correlations, we employed the maximum relevance minimum redundancy (mRMR) criterion. This approach calculates the relevance of each feature to the label and the redundancy to the already selected features. Based on these metrics, features were ranked and selected accordingly. To further identify the most influential subset of features, greedy recursive feature elimination was utilized. This technique iteratively eliminates features with the highest redundancy within the current feature set. Subsequently, a least absolute shrinkage and selection operator (LASSO) regression model was constructed on the discovery dataset. LASSO, depending on the modulation weight λ, shrinks regression coefficients, setting those of uncorrelated features to zero. The optimal λ was identified through 20-fold cross-validation, minimizing the cross-validation error. Features with non-zero coefficients were then incorporated into the radiomics features. Employing linear combinations, the retained features were weighted to generate a radiomics score for each patient. The Python scikit-learn package facilitated the implementation of LASSO regression modeling.
Model construction and test
Following LASSO feature selection, the refined features were incorporated into various machine learning models, including logistic regression, support vector machine and other relevant algorithms, to formulate a comprehensive risk assessment model. To ensure robustness and generalization, we employed fivefold cross-validation in determining the final radiomics signature. Furthermore, to provide a visual and efficient evaluation of the incremental prognostic value that ultrasound radiomics features impart on clinical risk factors, we crafted a corresponding nomogram in both the training and validation sets. The graph integrates ultrasound radiomics signatures with clinicopathologic risk factors. To verify the congruency between the nomogram’s predictive outcomes for histologic grading of invasive breast cancer and the actual observations, we evaluated the calibration effect through a calibration curve and assessed the nomogram’s calibration capability using Hosmer-Lemeshow analysis. Additionally, this study employed receiver operating characteristic (ROC) curves and decision curve analysis (DCA) to assess the diagnostic efficacy and clinical practicality, respectively, of the nomogram ( Fig. 1 c).
Statistical analysis
The χ 2 test and independent sample t -test were employed to assess the normality of distributions. For non-normally distributed continuous variables, the Mann-Whitney U test was utilized for comparison, while the χ 2 test was adopted for categorical variables. Predictive models were subsequently developed and ROC curves were plotted to determine the accuracy, area under the curve (AUC) values, 95% confidence intervals (CIs), sensitivity, specificity, precision, recall and F1 scores of the models. Additionally, calibration curves were plotted to evaluate the model’s predictions. Statistical significance was set at a p value of less than 0.05.
Results
Clinical and ultrasound characteristics
In a study examining the clinicopathologic characteristics of 468 cases of invasive breast cancer, 215 cases were classified as high grade, while 253 cases were classified as low grade. Univariate logistic regression analysis revealed statistically significant associations between age ( p = 0.018), maximum diameter ( p < 0.001), HER-2 status ( p < 0.001), ER status ( p < 0.001), PR status ( p < 0.001), Ki-67 index ( p < 0.001) and molecular sub-type ( p < 0.001) in the training set, comparing patients with high- and low-grade invasive breast cancer. Similarly, in the test set, significant differences were observed in maximum diameter ( p = 0.014), HER-2 status ( p = 0.002), ER status ( p < 0.001), PR status ( p = 0.019), Ki-67 index ( p = 0.002) and molecular sub-type ( p < 0.001) between the two groups ( Table 1 ).
Train | Test | |||||
---|---|---|---|---|---|---|
Feature | Low level | High level | p value | Low level | High level | p value |
Age | 53.99 ± 10.66 | 51.28 ± 9.90 | 0.018 | 53.65 ± 9.80 | 49.60 ± 10.26 | 0.055 |
Maximum diameter | 74.42 ± 53.09 | 94.60 ± 52.71 | <0.001 | 88.70 ± 52.76 | 114.67 ± 46.21 | 0.014 |
Nipple | 0.218 | 0.791 | ||||
Negative | 192 (96.48) | 163 (93.14) | 53 (98.15) | 38 (95.00) | ||
Positive | 7 (3.52) | 12 (6.86) | 1 (1.85) | 2 (5.00) | ||
Superficial fascia | 1.000 | 1.000 | ||||
Negative | 198 (99.50) | 174 (99.43) | 54 (100.00) | 40 (100.00) | ||
Positive | 1 (0.50) | 1 (0.57) | Null | Null | ||
Incisal margin | 0.078 | 0.630 | ||||
Negative | 25 (12.56) | 26 (14.86) | 7 (12.96) | 8 (20.00) | ||
Positive | 4 (2.01) | 11 (6.29) | 1 (1.85) | 1 (2.50) | ||
Unknown | 170 (85.43) | 138 (78.86) | 46 (85.19) | 31 (77.50) | ||
Lymph node | 0.384 | 0.548 | ||||
Negative | 138 (69.35) | 113 (64.57) | 32 (59.26) | 27 (67.50) | ||
Positive | 61 (30.65) | 62 (35.43) | 22 (40.74) | 13 (32.50) | ||
HER-2 | <0.001 | 0.002 | ||||
Negative | 99 (49.75) | 69 (39.43) | 27 (50.00) | 14 (35.00) | ||
Positive | 29 (14.57) | 75 (42.86) | 7 (12.96) | 18 (45.00) | ||
Unknown | 71 (35.68) | 31 (17.71) | 20 (37.04) | 8 (20.00) | ||
ER | <0.001 | <0.001 | ||||
Negative | 21 (10.55) | 83 (47.43) | 3 (5.56) | 15 (37.50) | ||
Positive | 178 (89.45) | 92 (52.57) | 51 (94.44) | 25 (62.50) | ||
PR | <0.001 | 0.019 | ||||
Negative | 30 (15.08) | 95 (54.29) | 10 (18.52) | 17 (42.50) | ||
Low (≤20%) | 21 (10.55) | 21 (12.00) | 6 (11.11) | 6 (15.00) | ||
High (>20%) | 148 (74.37) | 59 (33.71) | 38 (70.37) | 17 (42.50) | ||
Ki-67 | <0.001 | 0.002 | ||||
Low (<14%) | 81 (40.70) | 1 (0.57) | 16 (29.63) | 1 (2.50) | ||
High (≥14%) | 118 (59.30) | 174 (99.43) | 38 (70.37) | 39 (97.50) | ||
Molecular sub-type | <0.001 | <0.001 | ||||
Luminal A | 62 (31.16) | 1 (0.57) | 11 (20.37) | 1 (2.50) | ||
Luminal B | 101 (50.75) | 60 (34.29) | 34 (62.96) | 12 (30.00) | ||
Triple-positive | 15 (7.54) | 31 (17.71) | 6 (11.11) | 12 (30.00) | ||
HER-2 overexpressing | 18 (9.05) | 44 (25.14) | 2 (3.70) | 6 (15.00) | ||
TNBC | 3 (1.51) | 39 (22.29) | 1 (1.85) | 9 (22.50) |
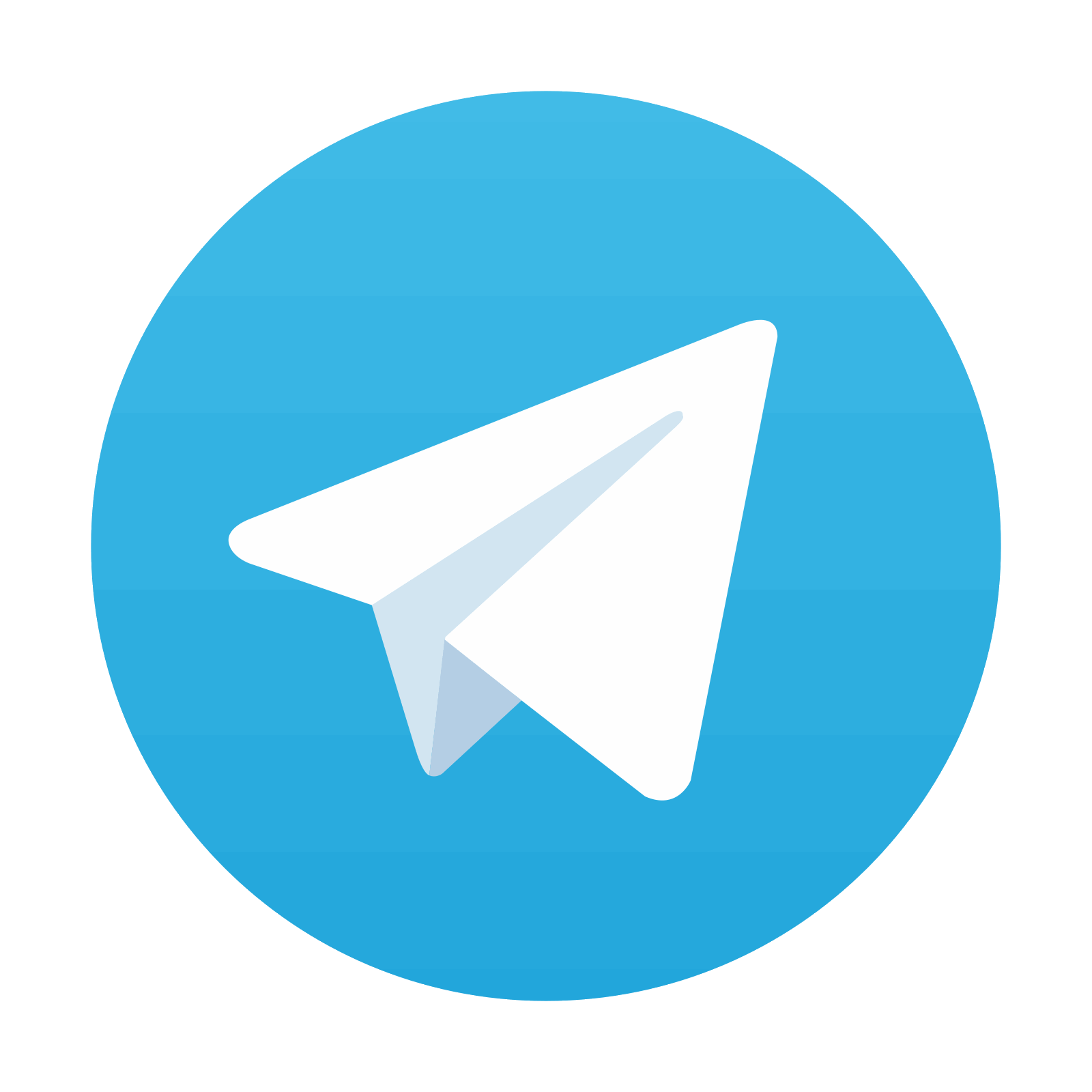
Stay updated, free articles. Join our Telegram channel

Full access? Get Clinical Tree
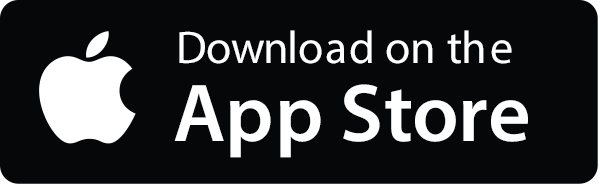

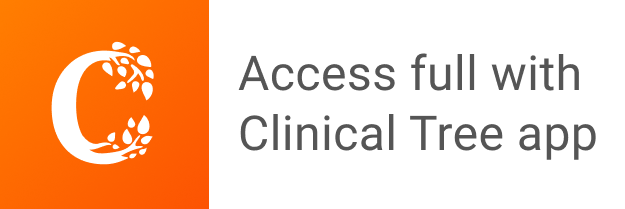