Abstract
Evidence-based imaging consists of identifying the relevant imaging literature for a specific clinical question, understanding the strengths and limitations of the existing evidence, and then incorporating that evidence into clinical care. Although the radiology literature is abundant, there may be biases that can affect the validity of the published information, which must be identified through critical analysis. The effectiveness of imaging can be considered on multiple successive levels, from technical adequacy, to diagnostic accuracy, to diagnostic certainty, to medical decision making, to patient outcome, and finally to societal cost-effectiveness. Radiologists must be able to translate measures of accuracy into the more clinically relevant levels of effectiveness, including medical decision making and patient outcomes. Imaging is not appropriate without the potential to change management based on the study results. In practice, evidence-based imaging consists of incorporating research regarding the value of imaging at different levels of effectiveness to address whether imaging is appropriate and, if so, which imaging is preferred. This approach balances the benefits of imaging with the costs, both in dollars and in patient outcomes, including complications from incorrect diagnosis, incidental findings, and radiation effects. As healthcare increasingly focuses on appropriate use of imaging to improve quality and contain costs, radiologists must use the best evidence to maximize the value of imaging for patients and society.
Keywords
Appropriateness, cost-effectiveness, effectiveness, evidence-based imaging, quality, outcomes
Introduction
Over the past 2 decades, evidence-based medicine has become a dominant paradigm for understanding best practices in medicine. Simply put, evidence-based medicine is the explicit incorporation of the best research evidence into the care decision-making process. More formally, evidence-based medicine has been defined by Sackett and others as the incorporation of the best available evidence with physician judgment and experience and patient values and preferences. Evidence-based medicine should be distinguished from eminence-based medicine, typified by the seasoned professional using his or her best judgment and knowledge, without explicit review and incorporation of medical evidence. Implicit within evidence-based medicine is a process of identifying relevant evidence, critically appraising the evidence to identify and weight most heavily that which is methodologically most valid, and incorporating the best evidence into clinical care.
In the therapeutic arena, evidence-based medicine is generally focused on the choice between competing drugs or procedures to treat specific diseases. In this realm, randomized clinical trials are supreme as the research approach most likely to lead to unbiased estimates of the effectiveness of the various treatments. However, in diagnostics, including imaging, the process is more complex. Diagnostic tests do not directly affect clinical outcome, but rather that effect is mediated by treatment. In addition, randomized clinical trials are an inefficient means of understanding the performance of diagnostic tests. More commonly, diagnostic tests are evaluated based on cohort studies, where all patients get one or more imaging studies. However, as discussed in this chapter, such cohort studies are susceptible to a number of different biases, emphasizing the need for critical analysis. Screening introduces an additional set of biases that are difficult to avoid, compelling the use of randomized clinical trials to evaluate screening studies despite their relative inefficiency and large sample size requirement. Accordingly, evidence-based medicine applied to imaging, known as evidence-based imaging, has particular challenges in its application. However, despite its limitations, incorporation of evidence into imaging practice remains essential for the highest-quality clinical care.
Evidence-based imaging consists of identifying the relevant imaging literature for a specific clinical question, understanding the strengths and limitations of the existing evidence, and then incorporating that evidence into clinical care. The strength of evidence is based on the quality of the published studies, including the study size and potential for bias. Grading schemes are often used to categorize the strength of evidence as low or high. Because most radiologists do not directly order imaging studies, evidence-based imaging is, by nature, a collaborative process between radiologists and referring clinicians, incorporating the best evidence with patient values, and the experience of radiologist and clinician alike.
In this chapter, we discuss the critical analysis of the radiology literature to understand the methodological rigor of the published information. Second, we define how to incorporate evidence from the literature into an understanding of whether imaging will have value. Finally, in the third section, we explore how to apply evidence-based imaging in clinical practice and answer the critical question of whether imaging should be performed.
Critical Analysis of Imaging Research
There is abundant literature evaluating radiology tests. As of 2015, there were over 100 journals devoted to imaging, with additional publication of imaging research in other nonimaging journals as well. This massive body of research is comprehensive in breadth, but unfortunately limited in depth, with most research pertaining only to new experimental imaging techniques and accuracy of existing imaging tests. Further, methodological flaws are common in the imaging literature (as in the rest of medicine). Intrinsic to evidence-based imaging is that medical evidence or research undergoes a critical evaluation process. In the radiology literature, there are several consistent pitfalls that decrease the validity of the published information ( Table 10.1 ). Often these biases cannot be completely excluded even with careful research design, but it is critical for the user of literature to understand the presence and magnitude of such concerns.
Biases in Inclusion of Subjects | |
Selection bias | Selection only of subjects in whom an imaging study will perform well, nonrandom, or nonconsecutive selection |
Spectrum bias | Selection of only subjects with severe disease |
Case-mix bias | Comparison subjects selected who are completely normal (rather than representing the clinical spectrum of those who would be imaged) |
Imaging-based selection | Including only those who undergo the two different tests being compared |
Biased Reference Standard | |
Indeterminate reference standard | Reference standard itself is not accurate in identifying the presence of disease |
Verification bias | Not all subjects undergo the same reference standard |
Differential verification bias | Different reference standards, and the choice of reference standard is determined by the imaging study |
Blinding | |
Unblinded interpretation | Interpreting radiologist has knowledge of the reference standard |
Unblinded reference standard | Individuals determining the reference standard have knowledge of the imaging test results |
Screening Biases | |
Lead time bias | The time of survival from diagnosis is increased by early detection even without a decrease in actual time of death. |
Length bias | Less aggressive lesions will have a longer time in the screen-detectable preclinical interval, causing screening to have a higher probability of detecting such less aggressive lesions. |
Overdiagnosis | Earlier detection leads to identification of some lesions that would never have been clinically known, leading to treatment without benefit. |
Selection bias occurs when a research study is conducted on only a portion of the population; such bias means that the results do not reflect the population as a whole. Selection bias can take on many forms. Restricting selection to study subjects in whom the imaging study will perform well will make the test look better than it actually is. For example, ultrasound is known to perform better in thinner subjects. So, an ultrasound study limited to those with low body mass index will have questionable relevance for the broader population. Similarly, imaging tends to be more accurate in more severe disease. Hence, including only advanced cases in an accuracy study will lead to overestimation of accuracy, also known as spectrum bias. For example, the accuracy of computed tomography (CT) for detection of hepatoma in patients with clinical symptoms and abnormal liver function tests might differ from the accuracy for screening in clinically normal but at-risk patients. Finally, an extreme form of spectrum bias occurs when accuracy is evaluated on a mix of clinically normal subjects and those with advanced disease. This design, sometimes erroneously referred to as a case-control study, may lead to the largest overestimation of both sensitivity and specificity. To avoid this bias, subjects should be selected from the clinically relevant group, representing both the spectrum of disease and normal that would be encountered in clinical practice. At a minimum, users of the imaging literature can often get a quick estimate of the potential for selection bias by closely examining the relative number of enrolled subjects versus the number of subjects who were not included in the study. Though not always clearly reported in many papers, readers can infer from the number of subjects recruited over the time frame of the study if the sample is consecutive or sporadic. Sporadic or convenience samples are obviously much more susceptible to selection bias.
A particularly insidious form of selection bias in imaging is imaging-based selection. Often in the comparison of competing imaging modalities, individuals are selected for inclusion because they have undergone both studies. This can be unbiased if all individuals are selected a priori to undergo both studies as part of the research protocol. However, more commonly, retrospective studies are performed selecting only those individuals who underwent both studies based on clinical indications rather than on a consecutive research protocol. In the latter design, it must be understood that individuals who undergo both imaging studies are unlikely to be representative of the more general population. Usually, individuals undergo both studies because the initial study is for some reason inadequate or not definitive. Obviously this creates a bias against the initial study. As a hypothetical example, one could compare the accuracy of ultrasound and CT scan for severe splenic injury in hemodynamically unstable trauma patients who underwent both studies. If the protocol was for patients to undergo ultrasound initially, with CT as a secondary study, the comparison would be biased because those patients with clearly positive ultrasound studies might be expected to go to the operating room or to angiography for definitive treatment without CT. Thus, the study would be composed of those in whom the ultrasound for some reason was not definitive.
This same example also highlights an additional challenge with imaging research, the selection of an appropriate reference standard. A reference standard should be definitive for the presence of pathology, should be the same for all individuals, and should be independent of the imaging studies being compared. In the ultrasound versus CT for splenic rupture example, pathology of the spleen in the operating room would be an appropriate reference standard as it is definitive and not affected by imaging modality. However, it is likely that not all patients will go to the operating room, so a secondary reference standard would also have to be employed. If CT is used as a reference standard for ultrasound, then the results will be biased in favor of CT. In addition, differential verification bias occurs when the imaging modality itself determines what reference standard will be performed. For example, if operative findings are used as a reference standard in some individuals, and imaging findings in others, then the imaging findings themselves will likely drive the determination of who goes to the operating room and will have the potential to affect the reference standard. For example, if ultrasound identifies some splenic ruptures but not others, the ones identified by ultrasound will be considered true positive diagnoses in the operating room. However, those that are missed by ultrasound might undergo conservative treatment, and because the patient may recover clinically, may not be considered as ruptured spleen based on the clinical reference standard (true negatives). In truth, these are missed diagnoses (false negatives) but with good clinical outcome because conservative treatment may sometimes be effective.
An additional bias in imaging is blinding of the imaging interpretation. This can be a particular problem with retrospective studies. The interpreting radiologists, if aware of the final diagnosis will, even without intending to, almost certainly change their subjective assessments to match the known diagnosis. This may be particularly problematic with new imaging tests in rare diseases, where the findings on imaging study may be remembered by the radiologist even for some time afterward. Blinding is also important for the reference standard. Though we tend to think of pathology and surgical findings as objective outcomes, they do have a subjective component that may be biased by knowledge of the imaging findings. For example, consider CT for the detection of ischemic bowel. We tend to think of bowel ischemia as a binary diagnosis. However, in reality, ischemia, like many diseases, runs a spectrum from mild (which may be hard to differentiate from normal) to severe, which is more unequivocal. Knowledge of the CT findings may certainly influence the surgeon’s decision to remove a segment of bowel and therefore determine the reference standard.
Screening carries an additional set of challenges. The premise underlying screening is that through performance of imaging, we will identify disease earlier and that earlier identification will enable more effective therapy and better outcomes. These are simple and appealing concepts, but in practice, measurement of the effectiveness of screening is challenged by several biases. The first of these is lead time. Lead time as a bias is an artifact of our reliance on survival as a metric for assessing screening. Survival is generally thought of as the time from diagnosis to death or some other adverse outcome, as in 5-year survival or mean survival. However, if a diagnosis is made earlier, then even if death occurs at the same time, the time from diagnosis to death is increased. The patient may not actually live longer, but he or she will live longer from the time of diagnosis and with knowledge of disease. Simply by detecting disease earlier, measured survival must be increased, even without any true effect on outcome. Increased survival in effect means earlier detection but provides no real information about clinical outcome.
A second challenge with screening is length bias. Length bias is due to the reality that the conditions for which screening is done (e.g., tumors or disease) are not homogeneous but will progress at different rates in different individuals. Screening only has potential value from the time that lesions reach a certain size threshold when they can be found by screening, known as the screen-detectable threshold, to the time when such lesions become clinically apparent. This time period is known as the preclinical screen-detectable interval. However, different tumors will exist in the preclinical screen-detectable interval for different lengths of time, depending on how aggressively they are growing. More aggressive tumors will rapidly grow through this screen-detectable preclinical interval, achieving clinical detectability after only a brief period of time. A more indolent tumor, on the other hand, because of its slow growth, will have a longer period of time in the screen-detectable preclinical interval. Thus, there is a longer time period when screening can detect slower growing lesions, and screening will be more likely to detect these less aggressive lesions. Screen-detected lesions will therefore be less aggressive than those detected on clinical grounds. Unfortunately, pathologic examination may not be able to differentiate these slower growing lesions from those that are more aggressive.
Finally, in screening, there is the construct of pseudo-disease, also called overdiagnosis. Simply put, early detection means that some tumors will be found years sooner than they would have become clinically manifest. If an individual dies after a tumor is detected by screening but before the tumor would have become clinically evident, then the screening has assigned that individual a diagnosis that he or she would never have known about otherwise. This individual would undergo all of the cancer treatment, with associated morbidity, but with no possibility of benefit, because death occurs before the disease would even have become clinically evident. This is particularly a problem when screening for relatively slow-growing disease in older patients. Overdiagnosis must be considered as a harm of screening and remains at the center of much of the ongoing controversy, particularly for breast and prostate cancer screening. Unfortunately, pseudo-disease can never be directly quantified because pathology cannot accurately predict growth rate and because time of death is never known in advance.
Understanding the Value of Imaging
Critically evaluating the literature is only the first step in evidence-based imaging. To be useful, the existing evidence must be applied to determine if an imaging test will have value in a specific patient. However, understanding the value of imaging in patient care and clinical outcomes is complex and requires more than simply knowing the accuracy of a diagnostic test. Fryback and Thornbury in the 1990s developed a tiered effectiveness model to enable better understanding of the value of imaging in context. Based on this model ( Table 10.2 ), imaging must obtain value at each successive level before achieving value at the highest levels, patient outcomes and societal value. At the most basic level, tests must have technical effectiveness. This means that an image must be obtainable, free from artifacts, and have sufficient signal-to-noise to generate meaningful information. It is at this level that core basic science radiology research occurs in trying to develop new or improved imaging tests. However, producing an image is not sufficient to provide value to the patient; the imaging study must be able to differentiate pathology from normal. This second level of effectiveness, accuracy, is dependent on the radiologist who interprets the imaging study and is generally measured as the sensitivity and specificity of the test. Sensitivity is the ability of a test to detect disease when it is present, and specificity is the ability of a test to identify subjects as normal when they do not have disease. There is a trade-off between these two because having a lower threshold to call a test abnormal will increase sensitivity at the expense of specificity and vice versa. This trade-off is captured in the receiver-operator characteristic (ROC) curve and can be summarized as the area under the curve. The diagnostic likelihood ratio is an alternate measure of the effectiveness of a diagnostic test that captures results in both diseased and nondiseased subjects as a single metric to compare tests.
Level of Effectiveness | Definition | Measures |
---|---|---|
Technical effectiveness | Ability to generate an image | Signal to noise, freedom from artifacts |
Accuracy | Ability for the image interpreter to distinguish between normal and abnormal | Sensitivity, specificity, receiver-operator characteristic curve |
Diagnostic certainty | Ability of the test to change the perceived probability of disease in a given patient | Pre- and posttest probability of disease, level of diagnostic certainty |
Therapeutic effectiveness | Ability of the test results to change choice of treatment in a given patient | Change in management |
Patient outcomes | Effect of use of test on patient outcomes, mediated through change in management or provision of prognostic information | Morbidity, mortality, patient satisfaction, quality of life |
Societal value | Opportunity cost of resources consumed to provide imaging when compared to other interventions in medicine | Cost-effectiveness analysis, cost-utility analysis, cost-benefit analysis |
Accuracy is core to our understanding of imaging and is foundational to radiology research. However, accuracy itself is not sufficient evidence to determine that an imaging study has overall value or indeed that it should be performed. Levels 3 and 4 of the Fryback and Thornbury hierarchy are diagnostic certainty and clinical decision making, which are related. In diagnostic certainty, the results of the diagnostic tests are not simply interpreted in a vacuum but are applied to an individual patient. Diagnostic certainty is the potential change in the likelihood of a particular disease(s) in a given patient. Sensitivity and specificity are useful metrics to compare diagnostic tests, but care is better driven by the probability of disease in a specific individual with a specific test result. This is known as the positive predictive value when the test is positive (the probability of disease in an individual with a positive test result) and the negative predictive value when the test is negative (the probability of an individual not having the disease when the test is negative). The positive and negative predictive values are based not only on the test result but also on the probability of disease in the population. The trade-off between sensitivity/specificity and positive/negative predictive value can be brought home through the use of Bayes theorem. In simplest terms, Bayes theorem is the precept whereby the probability of disease in a particular patient is based on a test result but also on the probability of disease based on the clinical findings before the test was performed, known as the pretest probability. In effect, Bayes theorem tells us that we cannot interpret imaging studies in isolation but, rather, that the results of an imaging study must be considered in the clinical context. More formally, Bayes theorem mathematically combines pretest probability with sensitivity and specificity to determine the probability of disease after the test results, through the equation:
p [ posttest ] = ( p [ pretest ] ) ( sensitivity ) { ( p [ pretest ] ) ( sensitivity ) } { ( 1 − p [ pretest ] ) ( 1 − specificity ) }
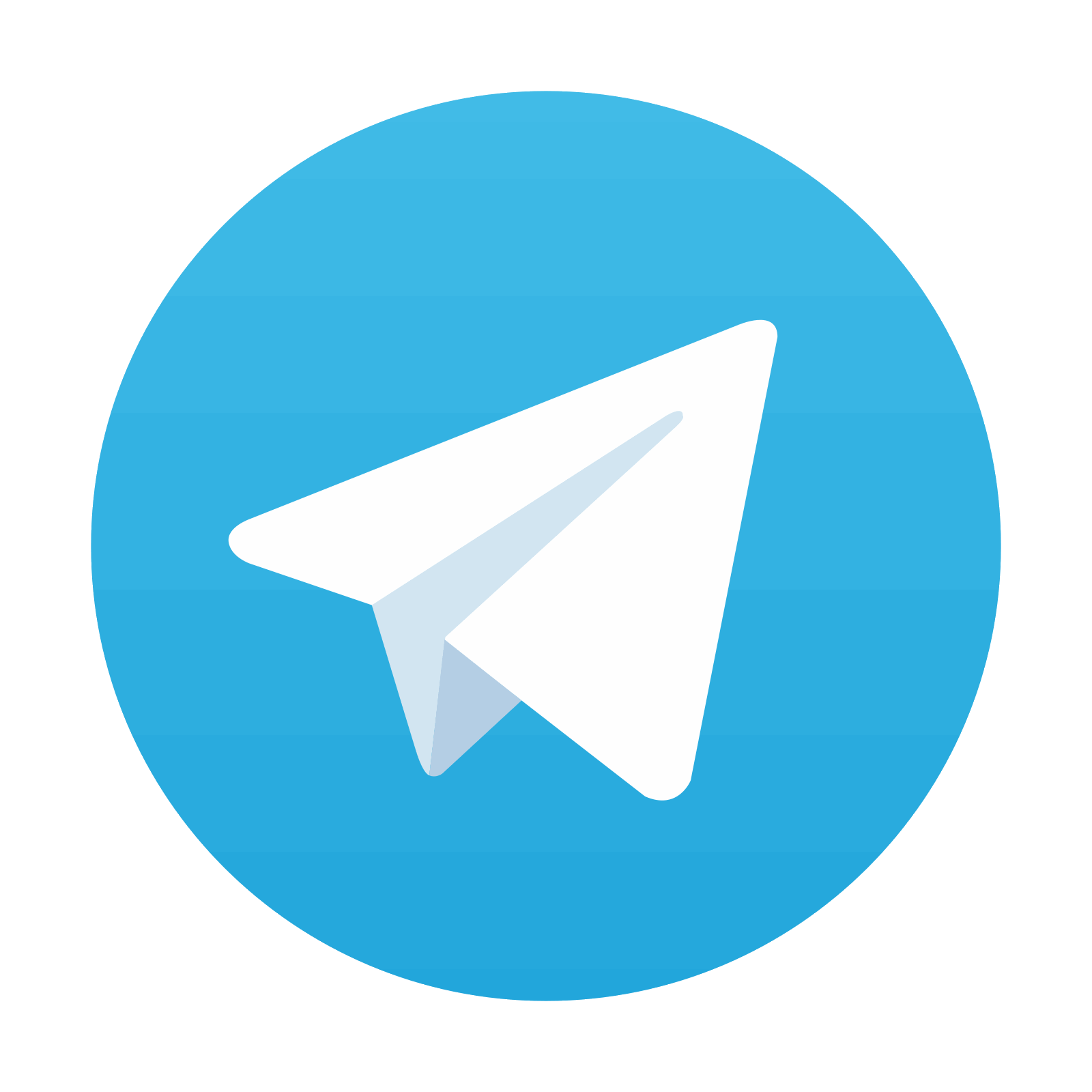
Stay updated, free articles. Join our Telegram channel

Full access? Get Clinical Tree
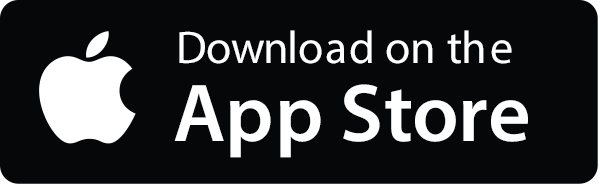
