Musculoskeletal (MSK) pain leads to significant healthcare utilization, decreased productivity, and disability globally. Due to its complex etiology, MSK pain is often chronic and challenging to manage effectively. Disparities in pain management—influenced by provider implicit biases and patient race, gender, age, and socioeconomic status—contribute to inconsistent outcomes. Interventional radiology (IR) provides innovative solutions for MSK pain through minimally invasive procedures, which can alleviate symptoms and reduce reliance on opioids. However, IR services may be underutilized, especially due to current treatment paradigms, referral patterns, and in areas with limited access to care. Artificial intelligence (AI) presents a promising avenue to address these inequities by analyzing large datasets to identify disparities in pain management, recognizing implicit biases, improving cultural competence, and enhancing pain assessment through multimodal data analysis. Additionally, patients who may benefit from an IR pain procedure for their MSK pain may then receive more information through their providers after being identified as a candidate by AI sifting through the electronic medical record. By leveraging AI, healthcare providers can potentially mitigate their biases while ensuring more equitable pain management and better overall outcomes for patients.
Introduction
Musculoskeletal (MSK) pain afflicts nearly half of the general population and is a major contributor to healthcare utilization, productivity loss, and disability. , MSK pain most often presents as chronic low back pain, neck pain, and pain associated with arthritis, resulting in a substantial number of healthcare visits each year. For instance, low back pain (LBP), accounts for approximately 570 million cases worldwide and contributes to an estimated 42 million annual ambulatory care encounters in the US. , In the emergency setting, an annual 4 million U.S. emergency department visits were primarily attributed to MSK pain, not accounting for low back pain. The scale of this issue is further exacerbated by the chronic nature and difficulty in treating chronic MSK pain as causes may arise from a complex combination of overuse, degenerative, neuropathic, rheumatologic, and other unknown reasons. When provider biases in the perception of patient pain are included in the equation, disparities in management varying across different patient races, sex, age, and socioeconomic status have led to inconsistent outcomes. Thus, effective pain management remains a significant challenge and burden to the healthcare system.
The inadequacies of pain management options, interventions and otherwise, have been a contributor to the opioid crisis as millions of individuals experiencing chronic pain began to depend on opioids. While effective for acute pain, sustained long-term opioid usage can lead to tolerance, addiction, dependence, and overdose. The opioid crisis not only contributed to a staggering number of deaths each year but also created barriers for those that require opioids for appropriate use to acquire the medication. Furthermore, it is estimated that the total economic impact of opioid misuse and fatalities in the U.S. amounted to an astounding $1.0 trillion in 2017. The enormous strain placed on the economy and the healthcare system by this epidemic highlights the urgent need for alternative pain management strategies.
The field of interventional radiology (IR) has long demonstrated its acumen for innovative solutions to difficult healthcare problems, including the problem of MSK pain. The expanding repertoire of IR MSK pain procedures include vertebral augmentation, sacroplasty, osseous ablation, joint arterial embolization and ablation, among others. These minimally invasive procedures may offer longer-lasting pain management solutions to ultimately decrease opioid usage. In this review article, we discuss the emerging role of IR in the treatment of MSK pain and the potential of leveraging artificial intelligence (AI) to address pain perception bias, treatment disparities, and increasing the utilization of IR pain services.
Health inequities in pain management
Impact of age, race and sex
Disparities in pain severity perception and provider management pose significant concerns in healthcare systems. These inequities are often driven by factors such race, gender, and age. Moreover, there is evidence to suggest that patients’ perception of pain may differ across sociodemographic boundaries. For instance, African Americans and Hispanics have consistently experienced greater pain severity and worse quality of life when compared to non-Hispanic Whites. , There may be many factors accounting for these observed differences in pain perception amongst minorities, including stressors, insurance status, and education level. When considering patient sex, women were found be to 28% more likely to report pain as both “chronic” and “severe” in comparison to men. And likewise with increasing age, studies demonstrate increasing pain scores and greater suffering.
Not surprisingly, provider practices affect documented patient differences in pain severity, which may stem from implicit bias. Studies evaluating implicit bias through the Implicit Association Test (IAT), have found that it is highly prevalent amongst medical professionals, and often working against minority and marginalized groups such as African Americans and women. , A recent study by Epstein et al. found that physicians who practiced with substantial implicit bias ended up providing less effective care to African American patients in certain instances, particularly involving patient-centered pain communication and opioid prescription. These disparities have also been seen in the sector of pain management. It was evidenced that physicians are underdiagnosing African American patients’ level of pain perhaps because they are perceived as experiencing less pain, leading to racial and ethnic differences in epidural analgesia rates in recent years. In fact, Rust et al. reported that amongst 15,935 women that received epidural analgesia using 1998 Georgia Medicaid claims data, epidural analgesia rates were decreased for black (49.5%), Hispanic (35.3%), and Asian women (48.1%) in comparison to non-Hispanic white women (59.6%; P < 0.001).
Furthermore, these implicit biases translate to differential prescription of both opioid and non-opioid medications. For instance, Pletcher et al. concluded that white patients were more likely to be prescribed opioids than African American, Hispanic, and Asian patients in 2005. In a study of 156,729 pain-related emergency department visits, African American (OR: 0.66; 95% CI: 0.62-0.70), Hispanic (OR: 0.67; 95% CI: 0.63-0.72), and Asian patients (OR: 0.79; 95% CI: 0.67-0.93) were significantly less likely to receive an opioid prescription compared to white patients even after statistical adjustment for pain severity. This discrepancy in prescription rates was true for all pain visits but was larger with increasing pain severity. These disparities are currently thought to be due to biases in pain assessment and socioeconomic factors, as African Americans have been found to be prescribed fewer opioid prescriptions even though they experienced higher levels of pain compared to white patients. Interestingly, the pattern was slightly different in the case of nonopioid prescriptions. Thompson et al. conducted a study on 94,222 pain-related primary care visits and did not find an association between race (African American, White, Other Race) and the probability of being prescribed non-opioid analgesics. However, the authors did report that the proportion of non-opioid analgesic-only prescriptions was 15% higher for African American patients when compared to white patients (RR: 1.15; 95% CI: 1.07-1.24). The reasons for this divergence from opioid prescriptions remains unclear and warrants additional research.
Moreover, a lack of culturally competent care may be an additional culprit for the mismanagement of pain. Often, miscommunication between physicians and patients leads to inadequate comprehension of the patients’ experience with pain and the overall pain assessment. Consequently, the physician may not consider the patient’s beliefs, attitudes, and behaviors during their clinical decision making, leading to inappropriate and undertreatment of the patient’s pain. This notion is corroborated by a study which concluded that language barriers (ie, limited English proficiency) by patients was associated with higher pain scores. Additionally, Kapoor et al. examined language barriers and concluded that patients who utilized language assistance services had reduced opioid consumption (median: 5 vs 10; P = 0.021) and lower pain intensity (median: 2.3 vs 2.6; P = 0.046) during the PACU stay than the subjects not using translation. This example showcases the impact that bridging language barriers can make for equalizing access for all sectors of the population, an area that can be revolutionized by AI.
Additionally, gender-based biases in pain management have also been widely reported. There is evidence to suggest that the genders perceive pain differently, stemming down to biological, psychological, and sociocultural factors. For instance, women are more sensitive to pain during specific portions of their menstrual cycle. Additionally, gender role expectations play a role in pain perception as studies have demonstrated that men were less likely to report their pain compared to women due to potential feelings of embarrassment. However, there are also treatment biases based on the beliefs held by physicians. Evidence suggests that some physicians may consider the pain experienced by women to be more “emotional” in nature than men rather than the condition itself, leading to improper assessment of their true pain experience and therefore inadequate treatment. Beyond the assessment of pain, physicians also prescribe varying types of medications to either gender. For example, in the treatment of lower back pain, male doctors prescribed twice as much hydrocodone to male patients than females (426 mg vs 238 mg), whereas female doctors prescribed more hydrocodone to females rather than males (335 mg vs 161 mg, F 1,85 = 9.65, P = 0.003 ). This observation further corroborates the context-specific gender biases that are at play in pain management that may be potentially mediated through the implementation of AI.
Biased pain management can have widespread consequences for patient outcomes. For one, misconceptions surrounding pain perception and management can prolong diagnoses, resulting in worsening of the condition. , In a study examining patients with pain related to spondyloarthritis, the median diagnostic delay was 3 years for the early diagnosis group, whereas it was 15 years for the late diagnosis group, with patients in the later diagnosis group have longer disease durations and poorer outcomes when it came to activity, function, and spinal mobility. Development of chronic pain conditions due to under treatment has been noted, but it also has been shown to cause feelings of distress and mistrust in healthcare providers. , This may lead to further hesitance to seek out medical care in the future, thereby providing the opportunity for the condition to aggravate. To add, it was found that pain severity and feelings of depression were strongly correlated with functional impairment of patients dealing with chronic pain compared to those without depression symptoms (average pain interference score > 5, OR = 5.36, P < 0.0001; activity limitation days ≥ 30, OR = 4.05, P < 0.0001; unemployed due to health reasons, OR = 4.06, P < 0.0001). Consequently, these factors can compound to reduce the overall functionality of patients subject to biased pain management. Finally, the economic costs of chronic pain conditions are steep. One study estimated that 100 million citizens were experiencing chronic pain and estimated incremental costs were $6,349 per patient-year. These large economic costs may further compound the negative effects caused by biased pain management, especially on those of lower SES, leading to less optimal patient outcomes.
At both the systems and provider levels, AI offers significant potential to improve pain management practices. By analyzing large-scale data, AI can identify patterns and highlight disparities that might be overlooked by individual providers, making them aware of their implicit biases by detecting biased language and providing feedback on how to adjust their approach, ensuring a more equitable assessment of a patient’s pain. Technological advances, such as ambient AI listeners, which operate alongside traditional patient encounters, show promise in capturing patient conversations and constructing clinical notes, saving providers significant time. These systems can capture the nuanced ways patients discuss pain based on their cultural norms, providing support to providers who may lack cultural competency training. Furthermore, for patients limited by linguistic or cognitive barriers, emerging machine learning techniques can recognize pain through facial expressions, body language, behavior, and other nonverbal cues. These multimodal AI technologies could help bridge gaps in pain identification, decreasing disparities related to provider variance and improving overall pain management.
The impact of socioeconomic factors and access to care
The relationship between socioeconomic status (SES) and pain treatment is complex and underscored by differences in provider perceptions and the educational attainment associated with high or low SES. Individuals with lower SES or living in areas with lower education levels are significantly more likely to have prolonged opioid use. Specifically, patients eligible for Medicaid, a proxy for low income, were more than twice as likely to have prolonged opioid prescriptions compared to those not eligible (OR: 2.03 for schedule II opioids, OR: 2.22 for schedule III opioids, and OR: 2.24 for combined schedule II/III opioids). Additionally, patients residing in areas with the lowest quartile of education were more likely to have prolonged opioid use, with odds ratios of 1.05 for schedule II opioids, 1.11 for schedule III opioids, and 1.12 for combined schedule II/III opioids. In a study by Kuo et al., the impact of prolonged opioid use in low SES and low educational attainment is emphasized wherein analyses of Medicare Part D data, encompassing 800,664 individuals aged 66 and older with 2,720,343 person-years of observation, revealed that prolonged opioid prescription use increased the odds of opioid overdose-related emergency room visits or hospitalizations by 60% (OR: 1.60; 95% CI: 1.53-1.67). Kuo et al. found that factors independently associated with prolonged use included older age, female gender, white race, low income, living in a lower education area, and comorbidities such as drug abuse, rheumatoid arthritis, and depression. These findings highlight the significant impact of socioeconomic status and education on opioid prescription practices and prolonged use. The multifactorial nature of this issue makes it difficult to identify an underlying etiology; however, AI tools may be able to discern distortions in provider perception and assist in recognizing their own biases toward these vulnerable populations.
Related to SES, access to pain management care is not homogeneous across the population, with many factors responsible for the observed inequities. As previously mentioned, out-of-pocket costs for pain medications are often exceedingly high which poses major limitations to access in those who are socioeconomic unprivileged, leading to under management of chronic pain. , , These shortcomings may even lead to lack of access to other pain mediating options such as physical therapy or interventional procedures. Green et al. found that minority groups, such as women and non-Hispanic African Americans, tend to be undertreated for pain when compared to the majority, ranging from the prescription of analgesics to referrals to pain specialists. Though the rationale for this observed disparity is multifactorial, it may be due to implicit bias and preconceived notions held by physicians during the treatment of minority patients, which may affect their clinical judgement, pain assessment, and therefore treatment plan. Additionally, geographical and regional factors may restrict access to pain care. A recent paper reported that rural older adults faced major transportation-related issues while accessing pain and palliative care, likely due to distance and cost. As of 2022, only about 14% of US hospices were situated in rural areas, and the number of palliative care hospices was 827 in 2022 which was down from 871 in 2018. This poses a problem as pain care centers are often concentrated in urban hubs, thereby resulting in lack of access to trained professionals and poorly managed pain amongst these marginalized groups.
Looking towards the future, AI can utilize vast amounts of clinical records as a source of retrospective data to identify potentially undertreated pain or emerging pain-related disorders. Given the significant impact of socioeconomic status and education on opioid prescription practices and prolonged use, AI tools such as Natural Language Processing (NLP) and Large Language Models (LLMs) can mine electronic medical records (EMRs) for patients with undertreated pain or opioid use disorders who may benefit from interventional pain procedures such as nerve ablation, vertebroplasty, or arterial embolization, thereby improving pain management and reducing chronic opioid use. By analyzing data related to SES, implicit biases, and language barriers, AI can uncover patterns that highlight disparities and identify patients who might otherwise fall through the cracks. Particularly for those with low SES or infrequent primary care visits, AI can pinpoint candidates for targeted interventions. Interventional radiologists can then review these patients via an interface and provide further information to their primary care providers, ensuring a more equitable and effective pain management strategy. This comprehensive approach underscores the potential of AI to bridge gaps in pain management, addressing both clinical and socioeconomic factors to enhance patient outcomes.
AI bias
Despite its promise, AI model performance in real clinical care may be limited by its inherent biases which may perpetuate healthcare disparities. These biases, including age, sex, race, among other features, can affect disease diagnosis. Moreover, AI models exhibit behavior akin to taking “shortcuts” whereby their ability to detect features, such as pain, could be from associated findings rather than direct features. Furthermore, because most AI training data derives from high income countries, , AI models may fail to capture pain features or descriptions from patients from other countries. These biases in AI pain identification could mislead or sustain pain management disparities.
Conclusion
The promise of AI in revolutionizing healthcare has been rapidly accelerating, with implications in improving diagnostic accuracy, clinical workflows, and patient outcomes. With the iterative advancements in machine learning and NLP, and more recently, LLMs, the ability of AI to process large healthcare datasets- already existing in our EMRs- and extract or generate meaningful information about a patient can be transformative. Specifically, in the MSK pain management space, there is potential for AI to help IRs and providers identify pain when it is not readily apparent, uncover undertreated pain, and offer more personalized treatments.
However, there are inherent challenges to clinical deployment of AI in the MSK pain management space. The clinical use of AI, especially technology that is deployed in the cloud and is not fully understood, draws ethical concerns regarding patient safety and privacy. What AI companies do with the large amounts of patient data that will be fed to their system could potentially be misguided. Moreover, many patients may not truly understand how their data may be used and may not be subject to a true informed consent process. Thus, ensuring ethical AI use in healthcare requires addressing the implications of biases and safeguarding patient rights.
Declaration of competing interest
The authors report no potential conflicts of interest.
References
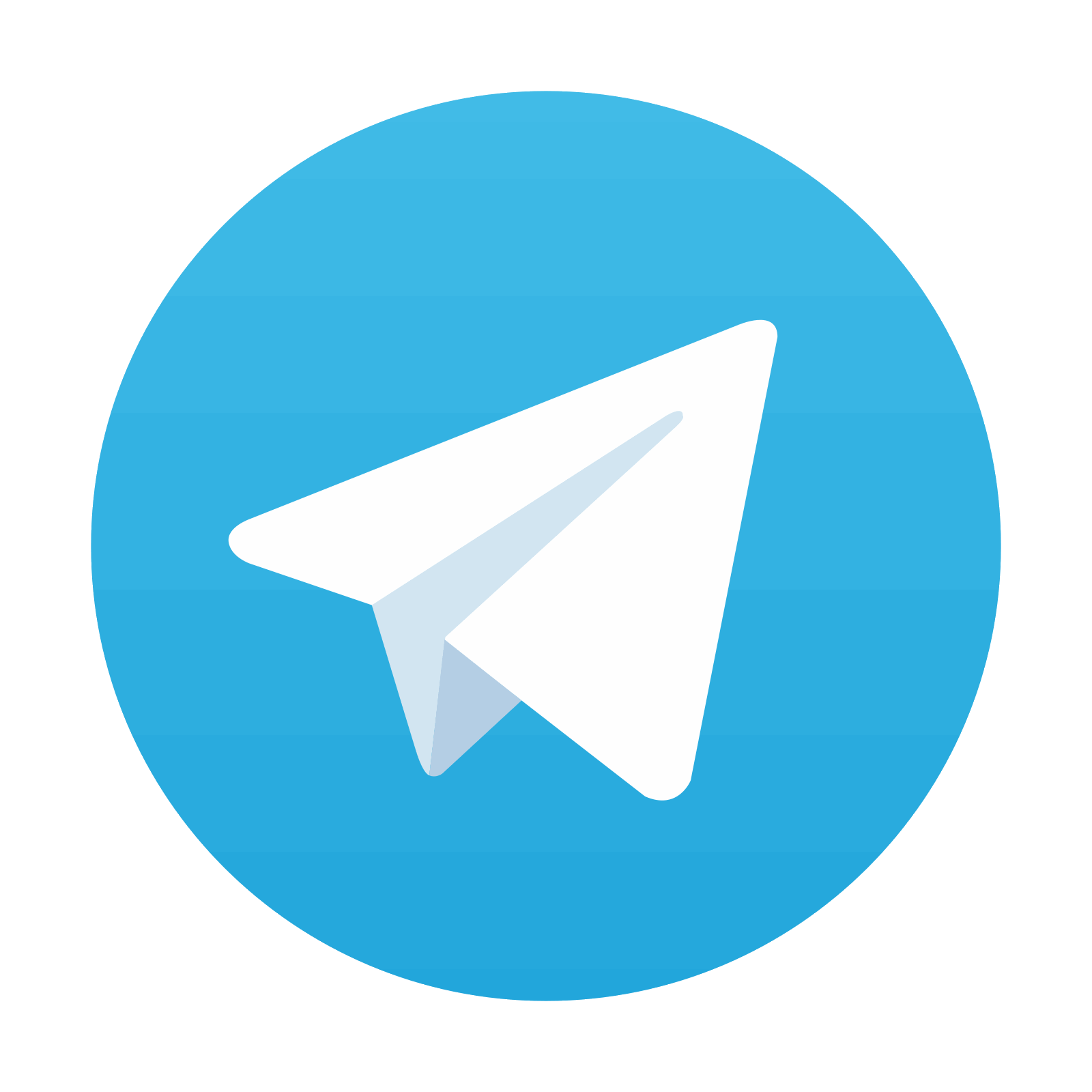
Stay updated, free articles. Join our Telegram channel

Full access? Get Clinical Tree
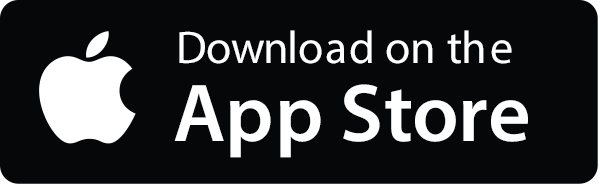

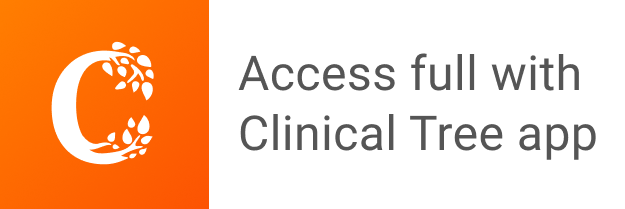