Rapid advances in neuroimaging and cyberinfrastructure technologies have brought explosive growth in the Web-based warehousing, availability, and accessibility of imaging data on a variety of neurodegenerative and neuropsychiatric disorders and conditions. There has been a prolific development and emergence of complex computational infrastructures that serve as repositories of databases and provide critical functionalities such as sophisticated image analysis algorithm pipelines and powerful three-dimensional visualization and statistical tools. The statistical and operational advantages of collaborative, distributed team science in the form of multisite consortia push this approach in a diverse range of population-based investigations.
Rapid advances over the past several decades in neuroimaging and cyberinfrastructure technologies have brought explosive growth in the Web-based warehousing, availability, and accessibility of imaging data on a broad array of neurodegenerative and neuropsychiatric disorders and conditions. This growth has been driven largely by the demand for multiscale data in the investigation of fundamental disease processes; the need for interdisciplinary cooperation to integrate, question, and interpret the data; and the movement of science in general toward freely available and openly accessible information. In response to this substantial need for capacity to store and exchange data online in meaningful ways in support of data analysis, hypothesis testing, and future reanalysis or even repurposing, the electronic collection, organization, annotation, storage, and distribution of clinical, genetic, and imaging data are essential activities in the contemporary biomedical and translational discovery process. The result has been the prolific development and emergence of complex computational infrastructures that serve as repositories of databases and provide critical functionalities such as sophisticated image analysis algorithm pipelines and powerful three-dimensional (3D) visualization and statistical tools. The statistical and operational advantages of collaborative, distributed team science in the form of multisite consortia continue to push this approach in a diverse range of population-based investigations.
New era of collaborative, interdisciplinary team science
The ongoing convergence and integration of neuroscientific infrastructures worldwide is heading to the creation of a global virtual imaging laboratory. Through ordinary Web browsers, large-scale image data sets and related clinical data and biospecimens, algorithm pipelines, computational resources, and visualization and statistical toolkits are easily accessible to users regardless of their physical location or disciplinary orientation. The promise of this investigatory environment-without-walls, and its incipient marshalling of scientific talent and facilitation of collaboration across multiple disciplines, are accelerating various translational initiatives with high societal impact, such as early or presymptomatic diagnosis and prevention of Alzheimer’s disease (AD).
Neuroimaging is now a major focus for multi-institutional research on progressive changes in brain architecture, biomarkers of treatment response, and the differential effects of disease on patterns of cognitive activation and connectivity. Prominent research consortia and multisite clinical trials have focused on AD, pediatric brain cancer, and fetal alcohol syndrome, in addition to multi-institutional collaborative programs for mapping the normal brain. Current leading-edge mapping consortia are focusing on the human brain as a complex network of connectivity and aim for a comprehensive structural description of the network architecture of the brain. This collaborative effort, the human connectome ( http://www.humanconnectomeproject.org/ ), is exploring and generating new insights in to the organization of the structural connections of the brain and their role in shaping functional dynamics and brain plasticity. Such large-scale efforts necessitate close coordination of image data collection protocols, ontology development, computational requirements, and sharing.
Impact of key areas of e-science on neuroscience and neurology
Multisite neuroimaging studies are dramatically accelerating the pace and volume of discoveries regarding major brain disease and the contrasts between normal and abnormal brain structure and function. The large-scale, purpose-driven data sets generated by these consortia can then be used by the broader community to model and predict clinical outcomes as well as guide clinicians in selecting treatment options for various neurologic diseases. Multisite trials are an important element in the study of a disease or the process of evaluating an intervention. Linking together multiple sites facilitates the recruitment of large samples that yield high statistical power for both main analyses as well as secondary analyses of subgroups. Generalizability of results to the level of the population is also maximized. Because data come from multiple sites, investigators can explore how the effects of a treatment vary across geographically diverse sites and how such variation relates to site characteristics, and to cultural and socioeconomic characteristics of the patients who participated in the studies. Such information can directly inform clinical decision-making at the level of the patient and guide the selection of treatment options. These research efforts are imperative for guiding treatment recommendations for neurologic disorders domestically and internationally as well as at the level of the individual patient. Multicenter collaborations strengthen understanding of brain diseases that affect all walks of life, all ages, and all cultures, thus enabling accelerated translation of neuroimaging trial outcomes directly into clinical applications.
Large archives of neuroimaging data are also creating innumerable opportunities for reanalysis and mining that can lead to new findings of use in basic research or in the characterization of clinical syndromes. Access to databases of neuroanatomic morphology has led to the development of content-driven approaches for exploration of brains that are anatomically similar, revealing patterns embedded within entire (sub)sets of neuroimaging data.
Provenance, or the description of the history of a set of data, has grown more important with the proliferation of research consortia-related efforts in neuroimaging. Knowledge about the origin and history of an image is crucial for establishing the quality of data and results; detailed information about how it was processed, including the specific software routines and operating systems that were used, is necessary for proper interpretation, high-fidelity replication, and reuse and repurposing of the data. New mechanisms have emerged for describing provenance in a simple and easy-to-use environment, alleviating the burden of documentation from the user and still providing a rich description of the source history of an image. This combination of ease of use and highly descriptive metadata is greatly facilitating the collection of provenance and subsequent sharing of large data sets.
Multimodal classification of images has advanced the usefulness of atlases of neuropathology through standardized 3D coordinate systems that integrate data across patients, techniques, and acquisitions. Atlases with a well-defined coordinate space, together with algorithms to align data with them, have enabled the pooling of brain mapping data from multiple subjects and sources, including large patient populations, and facilitated reconstruction of the trajectories of neurodegenerative diseases like AD as they progress in the living brain. Automated algorithms can then use atlas descriptions of anatomic variance to guide image segmentation, tissue classification, functional analysis, and detection of disease. Statistical representations of anatomy resulting from the application of atlasing strategies to specific subgroups of diseased individuals have revealed characteristics of structural brain differences in many diseases, including AD, human immunodeficiency virus/AIDS, unipolar depression, Tourette syndrome, and autism.
Atlas-based descriptions of variance offer statistics on degenerative rates and can elucidate clinically relevant features at the systems level. Atlases have identified differences in atrophic patterns between AD and Lewy body dementia, and differences between atrophy rates across clinically defined subtypes of psychosis. Atlases have also revealed the association between genes and brain structure. Based on well-characterized patient groups, population-based atlases contain composite maps and visualizations of structural variability, asymmetry, and group-specific differences. Pathologic change can be tracked over time, and generic features resolved, enabling these atlases to offer biomarkers for a variety of pathologic conditions as well as morphometric measures for genetic studies or drug trials.
Brain atlases can now accommodate observations from multiple modalities and from populations of individuals collected at different laboratories around the world. These probabilistic systems show promise for identifying patterns of structural, functional, and molecular variation in large imaging databases, for disease detection in individuals and groups, and for determining the effects of age, gender, handedness, and other demographic or genetic factors on brain structures in space and time. Integrating these observations to enable statistical comparison has already provided a deeper understanding of the relationship between brain structure and function.
This article considers and assesses the clinical implications of enabling large numbers of scientists to work in tandem with the same large data sets in the context of one such effort, the Alzheimer’s Disease Neuroimaging Initiative (ADNI). Two facets, in particular, of this project exemplify the clinical value of large-scale neuroimaging databases in research and in patient care: (1) disease diagnosis and progression tracking, including the diagnostic value of image databases in demarcating abnormal and normal ranges of biospecimens; and (2) role of neuroimages in statistical powering, subject stratification, and incisive end points and outcomes of clinical trials.
ADNI
ADNI exemplifies a remarkably successful, open, shared, and efficient database. ADNI brings together geographically distributed investigators with diverse scientific capabilities for the intensive study of biomarkers that signify and track the progression of AD. The quantity of imaging, clinical, cognitive, biochemical, and genetic data acquired and generated throughout the project have required powerful informatics systems and mechanisms for processing, integrating, and disseminating these data not only to support the research needs of the investigators who make up the ADNI cores but also to provide widespread data access to the greater scientific community. At the junction of this collaborative endeavor, the University of California at Los Angeles Laboratory of Neuro Imaging (LONI) has provided an infrastructure to facilitate data integration, access, and sharing across a diverse and growing community of multidisciplinary scientists.
ADNI is composed of 8 cores responsible for conducting the study along with external investigators authorized to use ADNI data. The various information systems used by the cores result in an intricate flow of data in to, out of, and among information systems and institutions. The data flow into the ADNI data repository ( http://adni.loni.ucla.edu/ ), where they are made available to the community. This well-curated scientific data repository enables data to be accessed by researchers across the globe and to be preserved over time. More than 1300 investigators have been granted access to ADNI data, resulting in extensive download activity that exceeds 1 million downloads of imaging, clinical, biomarker, and genetic data.
Image Data Workflow
The ADNI informatics core provides a user-friendly, Web-based environment for storing, searching, and sharing data acquired and generated by the ADNI community. In the process, the LONI image and data archive (IDA) has grown to meet the evolving needs of the ADNI community through continuing development of an increasingly interactive environment for data discovery and visualization. The automated systems developed to date include components for deidentification and secure archiving of imaging data from the 57 ADNI sites; management of the image workflow whereby raw images move from quarantine status to general availability and then proceed through preprocessing and postprocessing stages; integration of nonimaging data from other cores; management of data access and data sharing activities; and provision of a central, secure repository for disseminating data and related information to the ADNI community.
The imaging cores perform quality control and preprocessing of the magnetic resonance (MR) and positron emission tomography (PET) images; the ADNI image analysts perform postprocessing and analysis of the preprocessed images and related data; the biochemical samples are processed and the results compiled; and investigators download and analyze data as best fits their individual research needs.
Raw image data
In keeping with the objectives of the ADNI project to make data available to the scientific community, without embargo, and meet the needs of the core investigators, the IDA developed the image data workflow shown in Fig. 1 . Initially, each acquisition site uploads image data to the repository via the IDA, a Web-based application that incorporates several data validation and data deidentification operations, including validation of the subject identifier, validation of the dataset as human or phantom, validation of the file format, image file deidentification, encrypted data transmission, database population, secure storage of the image files and metadata, and tracking of data accesses. The image archiving portion of the system is both robust and simple, with new users requiring little, if any, training. Key system components supporting the process of archiving raw data are:
- 1.
The subject identifier is validated against a set of acceptable, site-specific identifiers.
- 2.
Potentially patient-identifying information is removed or replaced. Raw image data are encoded in the digital imaging and communications in medicine (DICOM), emission computer-assisted tomography (ECAT), high resolution research tomograph (HRRT) file formats, from different scanner manufacturers and models (eg, Siemens Symphony [Siemens Corporation, Oxfordshire, Oxford, UK], GE Signa Excite [GE Healthcare, Waukesha, WI, USA], Philips Intera [Philips Healthcare, Latham, NY, USA]). The Java applet deidentification engine is customized for each of the image file formats deemed acceptable by the ADNI imaging cores, and any files not of an acceptable format are bypassed. Because the applet is sent to the upload site, all deidentification takes place at the acquisition site and no identifying information is transmitted.
- 3.
Images are checked to see that they look appropriate for the type of upload. Phantom images uploaded under a patient identifier are flagged and removed from the upload set. This check is accomplished using a classifier that has been trained to identify human and phantom images.
- 4.
Image files are transferred encrypted (HTTPS [Hypertext Transfer Protocol Secure]) to the repository in compliance with patient-privacy regulations.
- 5.
Metadata elements are extracted from the image files and inserted into the database to support optimal storage and access. Customized database mappings were constructed for the various image file formats to provide consistency across scanners and image file formats.
- 6.
Newly received images are placed into quarantine status, and the images are queued for those charged with performing MR and PET quality assessment.
- 7.
Quality assessment results are imported from an external database and applied to the quarantined images. Images passing quality assessment are made available and images not passing quality control tagged as failing quality control.
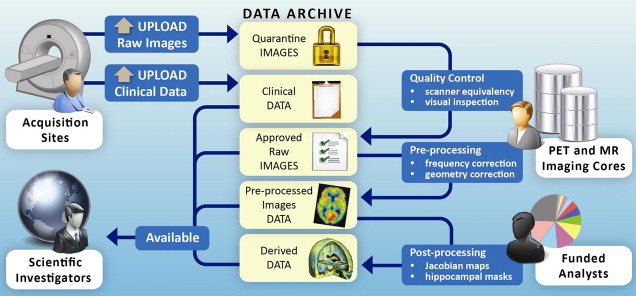
Once raw data undergo quality assessment and are released from quarantine, they become immediately available to authorized users.
Processed image data
Preprocessed images are the recommended common set for analysis. The goals of preprocessing are to produce data standardized across site and scanner and with certain image artifacts corrected. Usability of processed data for further analysis requires an understanding of the data provenance, or information about the origin and subsequent processing applied to a set of data. To provide almost immediate access to preprocessed data in a manner that preserved the relationship between the raw and preprocessed images and that captured processing provenance, we use an extended markup language (XML) schema that defines required metadata elements as well as standardized taxonomies. The system supports uploading large batches of preprocessed images in a single session with minimal interaction required by the person performing the upload. A key aspect of this process is agreement on the definitions of provenance metadata descriptors. Using standardized terms to describe processing minimizes variability and aids investigators in gaining an unambiguous interpretation of the data.
Preprocessed images are uploaded by the quality-control sites on a continuous basis. To minimize duplicate analyses, an automated data collection component was implemented whereby newly uploaded preprocessed scans are placed into predefined, shared data collections. These shared collections, organized by patient diagnostic group (normal control, mild cognitive impairment [MCI], AD) and visit (baseline, 6-month, and so forth), together with a redesigned user interface ( Fig. 2 ) that clearly indicates which images have not previously been downloaded, greatly reduce the time and effort needed to obtain new data. The same process may be used for postprocessed data, allowing analysts to share processing protocols via descriptive information contained in the XML metadata files.
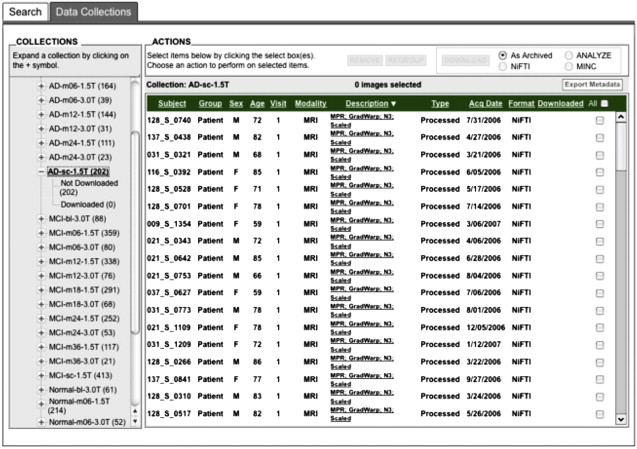
Impact of key areas of e-science on neuroscience and neurology
Multisite neuroimaging studies are dramatically accelerating the pace and volume of discoveries regarding major brain disease and the contrasts between normal and abnormal brain structure and function. The large-scale, purpose-driven data sets generated by these consortia can then be used by the broader community to model and predict clinical outcomes as well as guide clinicians in selecting treatment options for various neurologic diseases. Multisite trials are an important element in the study of a disease or the process of evaluating an intervention. Linking together multiple sites facilitates the recruitment of large samples that yield high statistical power for both main analyses as well as secondary analyses of subgroups. Generalizability of results to the level of the population is also maximized. Because data come from multiple sites, investigators can explore how the effects of a treatment vary across geographically diverse sites and how such variation relates to site characteristics, and to cultural and socioeconomic characteristics of the patients who participated in the studies. Such information can directly inform clinical decision-making at the level of the patient and guide the selection of treatment options. These research efforts are imperative for guiding treatment recommendations for neurologic disorders domestically and internationally as well as at the level of the individual patient. Multicenter collaborations strengthen understanding of brain diseases that affect all walks of life, all ages, and all cultures, thus enabling accelerated translation of neuroimaging trial outcomes directly into clinical applications.
Large archives of neuroimaging data are also creating innumerable opportunities for reanalysis and mining that can lead to new findings of use in basic research or in the characterization of clinical syndromes. Access to databases of neuroanatomic morphology has led to the development of content-driven approaches for exploration of brains that are anatomically similar, revealing patterns embedded within entire (sub)sets of neuroimaging data.
Provenance, or the description of the history of a set of data, has grown more important with the proliferation of research consortia-related efforts in neuroimaging. Knowledge about the origin and history of an image is crucial for establishing the quality of data and results; detailed information about how it was processed, including the specific software routines and operating systems that were used, is necessary for proper interpretation, high-fidelity replication, and reuse and repurposing of the data. New mechanisms have emerged for describing provenance in a simple and easy-to-use environment, alleviating the burden of documentation from the user and still providing a rich description of the source history of an image. This combination of ease of use and highly descriptive metadata is greatly facilitating the collection of provenance and subsequent sharing of large data sets.
Multimodal classification of images has advanced the usefulness of atlases of neuropathology through standardized 3D coordinate systems that integrate data across patients, techniques, and acquisitions. Atlases with a well-defined coordinate space, together with algorithms to align data with them, have enabled the pooling of brain mapping data from multiple subjects and sources, including large patient populations, and facilitated reconstruction of the trajectories of neurodegenerative diseases like AD as they progress in the living brain. Automated algorithms can then use atlas descriptions of anatomic variance to guide image segmentation, tissue classification, functional analysis, and detection of disease. Statistical representations of anatomy resulting from the application of atlasing strategies to specific subgroups of diseased individuals have revealed characteristics of structural brain differences in many diseases, including AD, human immunodeficiency virus/AIDS, unipolar depression, Tourette syndrome, and autism.
Atlas-based descriptions of variance offer statistics on degenerative rates and can elucidate clinically relevant features at the systems level. Atlases have identified differences in atrophic patterns between AD and Lewy body dementia, and differences between atrophy rates across clinically defined subtypes of psychosis. Atlases have also revealed the association between genes and brain structure. Based on well-characterized patient groups, population-based atlases contain composite maps and visualizations of structural variability, asymmetry, and group-specific differences. Pathologic change can be tracked over time, and generic features resolved, enabling these atlases to offer biomarkers for a variety of pathologic conditions as well as morphometric measures for genetic studies or drug trials.
Brain atlases can now accommodate observations from multiple modalities and from populations of individuals collected at different laboratories around the world. These probabilistic systems show promise for identifying patterns of structural, functional, and molecular variation in large imaging databases, for disease detection in individuals and groups, and for determining the effects of age, gender, handedness, and other demographic or genetic factors on brain structures in space and time. Integrating these observations to enable statistical comparison has already provided a deeper understanding of the relationship between brain structure and function.
This article considers and assesses the clinical implications of enabling large numbers of scientists to work in tandem with the same large data sets in the context of one such effort, the Alzheimer’s Disease Neuroimaging Initiative (ADNI). Two facets, in particular, of this project exemplify the clinical value of large-scale neuroimaging databases in research and in patient care: (1) disease diagnosis and progression tracking, including the diagnostic value of image databases in demarcating abnormal and normal ranges of biospecimens; and (2) role of neuroimages in statistical powering, subject stratification, and incisive end points and outcomes of clinical trials.
ADNI
ADNI exemplifies a remarkably successful, open, shared, and efficient database. ADNI brings together geographically distributed investigators with diverse scientific capabilities for the intensive study of biomarkers that signify and track the progression of AD. The quantity of imaging, clinical, cognitive, biochemical, and genetic data acquired and generated throughout the project have required powerful informatics systems and mechanisms for processing, integrating, and disseminating these data not only to support the research needs of the investigators who make up the ADNI cores but also to provide widespread data access to the greater scientific community. At the junction of this collaborative endeavor, the University of California at Los Angeles Laboratory of Neuro Imaging (LONI) has provided an infrastructure to facilitate data integration, access, and sharing across a diverse and growing community of multidisciplinary scientists.
ADNI is composed of 8 cores responsible for conducting the study along with external investigators authorized to use ADNI data. The various information systems used by the cores result in an intricate flow of data in to, out of, and among information systems and institutions. The data flow into the ADNI data repository ( http://adni.loni.ucla.edu/ ), where they are made available to the community. This well-curated scientific data repository enables data to be accessed by researchers across the globe and to be preserved over time. More than 1300 investigators have been granted access to ADNI data, resulting in extensive download activity that exceeds 1 million downloads of imaging, clinical, biomarker, and genetic data.
Image Data Workflow
The ADNI informatics core provides a user-friendly, Web-based environment for storing, searching, and sharing data acquired and generated by the ADNI community. In the process, the LONI image and data archive (IDA) has grown to meet the evolving needs of the ADNI community through continuing development of an increasingly interactive environment for data discovery and visualization. The automated systems developed to date include components for deidentification and secure archiving of imaging data from the 57 ADNI sites; management of the image workflow whereby raw images move from quarantine status to general availability and then proceed through preprocessing and postprocessing stages; integration of nonimaging data from other cores; management of data access and data sharing activities; and provision of a central, secure repository for disseminating data and related information to the ADNI community.
The imaging cores perform quality control and preprocessing of the magnetic resonance (MR) and positron emission tomography (PET) images; the ADNI image analysts perform postprocessing and analysis of the preprocessed images and related data; the biochemical samples are processed and the results compiled; and investigators download and analyze data as best fits their individual research needs.
Raw image data
In keeping with the objectives of the ADNI project to make data available to the scientific community, without embargo, and meet the needs of the core investigators, the IDA developed the image data workflow shown in Fig. 1 . Initially, each acquisition site uploads image data to the repository via the IDA, a Web-based application that incorporates several data validation and data deidentification operations, including validation of the subject identifier, validation of the dataset as human or phantom, validation of the file format, image file deidentification, encrypted data transmission, database population, secure storage of the image files and metadata, and tracking of data accesses. The image archiving portion of the system is both robust and simple, with new users requiring little, if any, training. Key system components supporting the process of archiving raw data are:
- 1.
The subject identifier is validated against a set of acceptable, site-specific identifiers.
- 2.
Potentially patient-identifying information is removed or replaced. Raw image data are encoded in the digital imaging and communications in medicine (DICOM), emission computer-assisted tomography (ECAT), high resolution research tomograph (HRRT) file formats, from different scanner manufacturers and models (eg, Siemens Symphony [Siemens Corporation, Oxfordshire, Oxford, UK], GE Signa Excite [GE Healthcare, Waukesha, WI, USA], Philips Intera [Philips Healthcare, Latham, NY, USA]). The Java applet deidentification engine is customized for each of the image file formats deemed acceptable by the ADNI imaging cores, and any files not of an acceptable format are bypassed. Because the applet is sent to the upload site, all deidentification takes place at the acquisition site and no identifying information is transmitted.
- 3.
Images are checked to see that they look appropriate for the type of upload. Phantom images uploaded under a patient identifier are flagged and removed from the upload set. This check is accomplished using a classifier that has been trained to identify human and phantom images.
- 4.
Image files are transferred encrypted (HTTPS [Hypertext Transfer Protocol Secure]) to the repository in compliance with patient-privacy regulations.
- 5.
Metadata elements are extracted from the image files and inserted into the database to support optimal storage and access. Customized database mappings were constructed for the various image file formats to provide consistency across scanners and image file formats.
- 6.
Newly received images are placed into quarantine status, and the images are queued for those charged with performing MR and PET quality assessment.
- 7.
Quality assessment results are imported from an external database and applied to the quarantined images. Images passing quality assessment are made available and images not passing quality control tagged as failing quality control.
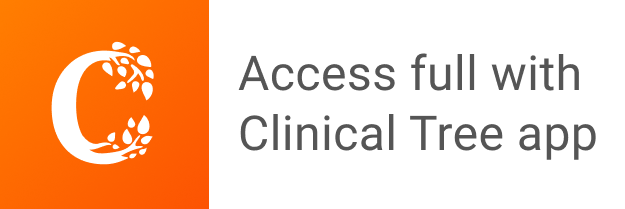