Abstract
Vulnerable atherosclerotic plaque is a type of plaque that poses a significant risk of high mortality in patients with cardiovascular disease. Ultrasound has long been used for carotid atherosclerosis screening and plaque assessment due to its safety, low cost and non-invasive nature. However, conventional ultrasound techniques have limitations such as subjectivity, operator dependence, and low inter-observer agreement, leading to inconsistent and possibly inaccurate diagnoses. In recent years, a promising approach to address these limitations has emerged through the integration of artificial intelligence (AI) into ultrasound imaging. It was found that by training AI algorithms with large data sets of ultrasound images, the technology can learn to recognize specific characteristics and patterns associated with vulnerable plaques. This allows for a more objective and consistent assessment, leading to improved diagnostic accuracy. This article reviews the application of AI in the field of diagnostic ultrasound, with a particular focus on carotid vulnerable plaques, and discusses the limitations and prospects of AI-assisted ultrasound. This review also provides a deeper understanding of the role of AI in diagnostic ultrasound and promotes more research in the field.
Introduction
Cardio-cerebrovascular disease is one of the leading causes of disability and death worldwide [ ]. Atherosclerosis (AS) is the leading cause of cardio-cerebrovascular disease [ ]. Atherosclerotic plaques are the hallmark lesions of AS [ ], and plaques with a high risk of rupture and prone to thrombosis are called vulnerable plaques [ ]. The core features of vulnerable plaques include thin caps with large lipid cores, active inflammation, endothelial detachment with superficial platelet aggregation, fissured plaques and stenosis >90%. Additionally, features such as superficial calcified nodules, glistening yellow, intra-plaque hemorrhage, endothelial dysfunction and outward (positive) remodeling are also important in the diagnosis of vulnerable plaques [ ]. These plaques are susceptible to rupture due to fibrous cap thinning in response to mechanical stresses and inflammatory responses within the vessel wall. When rupture occurs, the exposed lipid core contacts with the blood, leading to rapid platelet aggregation to form a thrombus, potentially resulting in acute vascular obstruction, the primary pathological basis for myocardial infarction and stroke [ ]. Therefore, early diagnosis and treatment of vulnerable plaques is an essential way to prevent cardio-cerebrovascular disease.
Due to its non-invasive and safe nature, ultrasound has been widely used for the diagnosis of AS and assessment of carotid vulnerable plaques [ ]. However, ultrasound has some limitations as it is dependent on the operator’s technical skills, is more subjective at identifying vulnerable plaques, and has low inter-observer agreement. Artificial intelligence (AI) empowers machines with problem-solving and decision-making capabilities through algorithms [ ], surpassing humans in some respects [ , ]. AI has also been applied in the medical field, ranging from imaging diagnosis of diseases [ , ] to interventional surgical treatment [ ]. Medical imaging data are more suitable for training AI algorithms due to their large amount of relevant data and high availability to improve qualitative assessment and reduce visual dependency [ ].
This article reviews advances in the use of AI in the ultrasound diagnosis of different diseases, particularly AS-prone plaques. This article also aims to deepen the understanding of AI in conjunction with ultrasound for the diagnosis of atherosclerotic plaques and to encourage further research in this field.
Application of AI in ultrasound
Overview of AI
The term AI was first coined in 1956 as a broad term for the study of how computer systems can simulate certain human thought processes and intelligent behavior through the development and application of algorithms [ ]. Continuous training enables computer systems to learn from input or past data [ ], enhancing efficiency and reducing errors, and is applicable across various fields such as finance, autonomous driving and health care. However, AI’s reliance on vast data and computational resources often leads to algorithmic complexities and low interpretability. AI includes machine learning (ML) and deep learning (DL). Figure 1 shows the hierarchy between these terms. ML algorithms learn from large data sets that traditional software struggles to analyze [ ]. ML can be broadly classified into four learning modes: supervised learning, un-supervised learning, semi-supervised learning and reinforcement learning. Supervised learning can make accurate predictions and classifications based on pre-labeled data but requires extensive time for data collection. In un-supervised learning, the data set is un-labeled in advance. However, it may be difficult to determine the accuracy. Semi-supervised learning combines the features of supervised and un-supervised learning by using a small amount of labeled data and a large amount of un-labeled data for training. Whereas reinforcement learning can be seen as a mixture of supervised and un-supervised learning, it is a type of pattern recognition learning [ ]. The training process of reinforcement learning is often more time-consuming and need more trials than other AI algorithms. DL, based on artificial neural networks (ANNs), processes layers to analyze data [ , ], excelling at handling complex, unstructured data for tasks such as facial and voice recognition. It automatically extracts features from large data sets but demands significant computational resources and time, complicating de-bugging. ANNs mimic the human brain’s neural structure to process information, offering strong adaptability and optimizing outputs, and are widely used in biometric identification and fault detection; however, training complexities and over-fitting risks in small data sets are notable. The convolutional neural network (CNN) is the most common DL architecture [ ] using convolutional layers to extract local features, reducing network parameters and enhancing training efficiency. CNNs out-perform traditional neural networks in image processing, capturing spatial features effectively for tasks such as image classification and segmentation but struggle with small data sets.
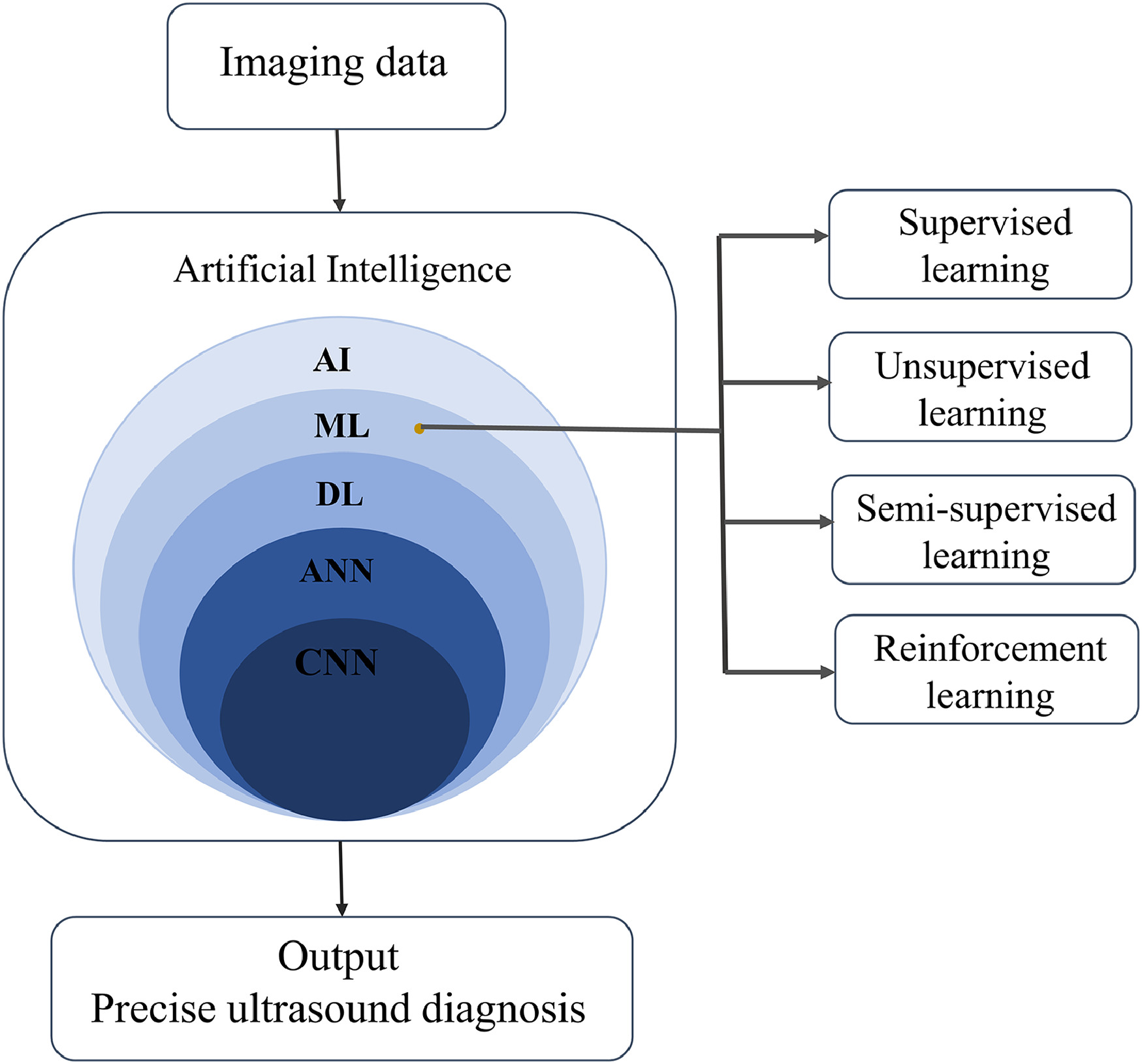
Overview of the application of AI in ultrasound
With the development of AI, ML and DL have made great strides in the field of medical imaging with their excellent image recognition and raw data-processing capabilities [ , ]. AI-assisted ultrasound has greatly improved variability factors such as operator and scanner dependency, increased the accuracy of diagnostic results and significantly reduced the time required for each image reader [ ]. Figure 2 depicts the general workflow of AI-assisted ultrasound. Table 1 demonstrates the application of AI in ultrasound imaging for lesion detection, disease classification, image segmentation and prognostic assessment of different organ systems, covering thyroid, breast, liver, kidney, heart and other systems.
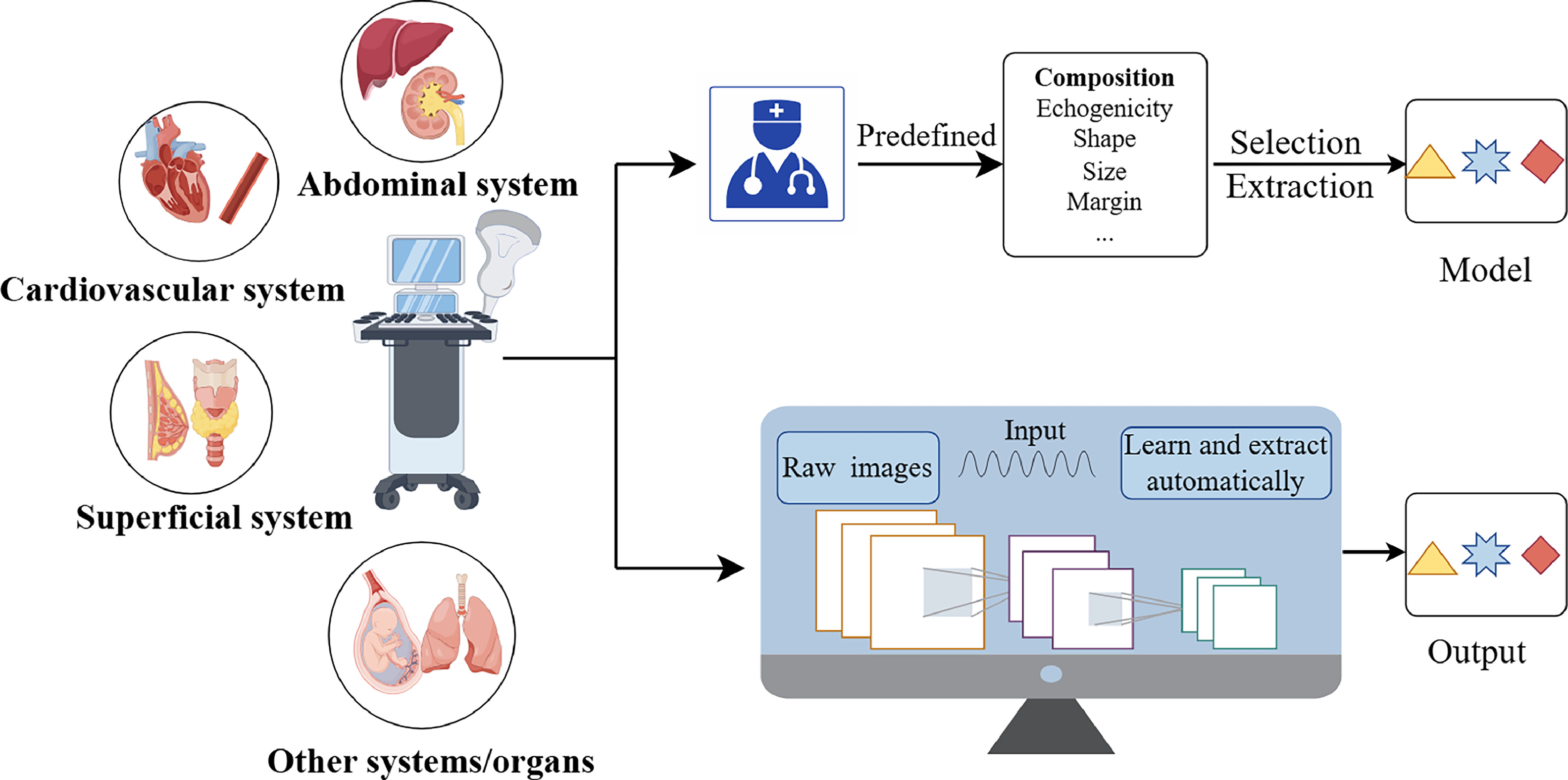
Organ system | Lesion detection | Disease classification | Image segmentation | Prognosis evaluation or clinical decision |
---|---|---|---|---|
Thyroid | To detect thyroid papillary cancer [ ] and thyroid nodules [ ] | Enhance the specificity of TI-RADS [ ] and distinct benign and malignant nodules | Segmentation of thyroid nodules [ ] | Based on DL to decide whether a thyroid nodule should undergo a biopsy [ ] |
Breast | Detect breast lesions [ ] and diagnose breast cancer [ ] | Distinct benign and malignant breast lesions [ , ] | Segment the breast tumor automatically [ , ] | Reduce unnecessary biopsy [ ] and predict lymph node metastasis [ ] |
Liver | Detect fatty liver disease [ ] and liver fibrosis [ ] | Identify benign and malignant focal liver lesions [ ] and classify lesions automatically [ ] | Segment liver lesions [ ] and in liver ablation [ ] | Predict responses to TACE for hepatocellular carcinoma patients [ ] |
Kidney | Detect and measure renal cysts [ ] and renal lesions [ ] | Predict and classify kidney function [ ] | Kidney segmentation to detect CKD [ ] and segmentation of ultrasound kidney [ ] | Assessment of kidney function after allograft transplantation [ ] |
Heart | Detect diseases [ ] and regional wall motion abnormalities [ ] | Quantification of cardiac function [ ] | Segmentation of the left ventricle of the heart [ ] | |
Other systems | Diagnosis of abnormal fetal brain [ ] | Distinguish myopathic conditions [ ] | Segmentation of fetal lung [ ] |
Advances in the application of AI in the ultrasound diagnosis of carotid AS plaques
Through advances in imaging technology intra-vascular ultrasound (IVUS) and contrast-enhanced ultrasound (CEUS) techniques are able to assess intra-vascular and plaque conditions more accurately [ ]. However, IVUS is an invasive technique and relatively expensive. Additionally, whether CEUS can adequately assess vulnerable plaques remains controversial. Some scholars have argued that plaque vulnerability cannot be assessed based on the enhanced intensity of CEUS [ ]. Huang et al. also argued that the quantitative assessment of carotid plaque by ultrasonography in clinical practice is limited [ ]. The rapid development of AI technology has opened up new approaches for its application in medical image analysis, especially in the diagnosis and assessment of atherosclerotic plaques, which shows great potential. AI is able to automate and intelligently process ultrasound images to provide fast and accurate diagnostic support through DL and big data analysis. Table 2 further summarizes the specific applications of AI in plaque diagnosis and assessment in the following aspects: (ⅰ) AI can efficiently and automatically measure intima-media thickness (IMT) and plaque area, reducing human error and improving diagnostic accuracy; (ⅱ) AI technology can identify and segment different components of plaques such as lipid cores and fibrous caps, which can help to assess plaque stability and vulnerability; (ⅲ) predicting risk to assess prognosis: based on detailed image analysis by AI, doctors are able to predict the risk of plaques and thus assess the disease prognosis for patients. The results of these studies illustrate the significant advancements in AI in ultrasound imaging, particularly in enhancing diagnostic precision, minimizing errors and accelerating analysis. Figure 3 illustrates the workflow of AI-assisted ultrasound in the diagnosis of AS.
Study | Algorithm | Data type (number of patients or images) | Objective | Performance |
---|---|---|---|---|
Savaş S, et al. [ ] | CNN | Ultrasound images (n = 501) | IMT measurement and classification | Achieved accuracy of 89.1% in IMT classification with 89% sensitivity and 88% specificity |
Sudha S, et al. [ ] | CNN | Ultrasound images (n = 220) | IMT measurement | Mean IMT difference of 0.08 mm is observed, with difference ranging from 0.07 mm to 0.14 mm |
Biswas M et al. [ ] | Combination of two DL systems (AtheroPoint/ AtheroEdge) | Ultrasound images (n = 407) | Lumen diameter measurement to estimate the risk of stroke | Improvement in lumen diameter error by 24%; AUROC showed an accuracy above 90% |
Biswas M et al. [ ] | DL (AtheroEdge system) | Ultrasound images (n = 250) | In the form of carotid IMT and total plaque area to joint automated detection and measurement of wall thickness and carotid plaque | Carotid IMT error using the AI model is 0.0935 ± 0.0637 mm, which is lower than those of all previous methods |
Wei, et al. [ ] | CNN (automatic recognition system, BETU) | Ultrasound images (n = 3259) | Real-time plaque recognition from ultrasound videos | Diagnostic accuracy of BETU (98.5%); consistent with the radiologist’s (κ = 0.967; p < 0.001) |
Lekadir K, et al. [ ] | CNN | Ultrasound images (n = 56) | Identify characterization of plaque composition | Results showed a correlation of ∼0.9 with clinical assessment |
Su, et al. [ ] | Two transfer DL models (Inception V3 and VGG-16) | Doppler ultrasound images (n = 230) | Classify color Doppler images of carotid plaques automatically into two categories to predict stroke risk | Achieved accuracy of 93.81% in classification |
Saba L, et al. [ ] | DL | Ultrasound images (n = 346) | Classify symptomatic and asymptomatic plaques | DL accuracy (89.7%) is superior to previously published ML [ ] (83.7%) |
Skandha SS, et al. [ ] | Three types of DL (deep CNN, VGG-16 and tCNN) and ML | Ultrasound images (n = 346) | Plaque tissue classification and characterization | Accuracy of DCNN and tCNN are 95.66% and 83.33%, respectively. DCNN was superior to ML by 7.01% |
Jain PK, et al. [ ] | UNet | Ultrasound images (n = 660) | Plaque segmentation | Mean accuracy was 98.55 ( p < 0.0001) |
Jain PK, et al. [ ] | SegNet-UNet | Ultrasound images (n = 970) | Plaque segmentation | System performs segmentation within 1s |
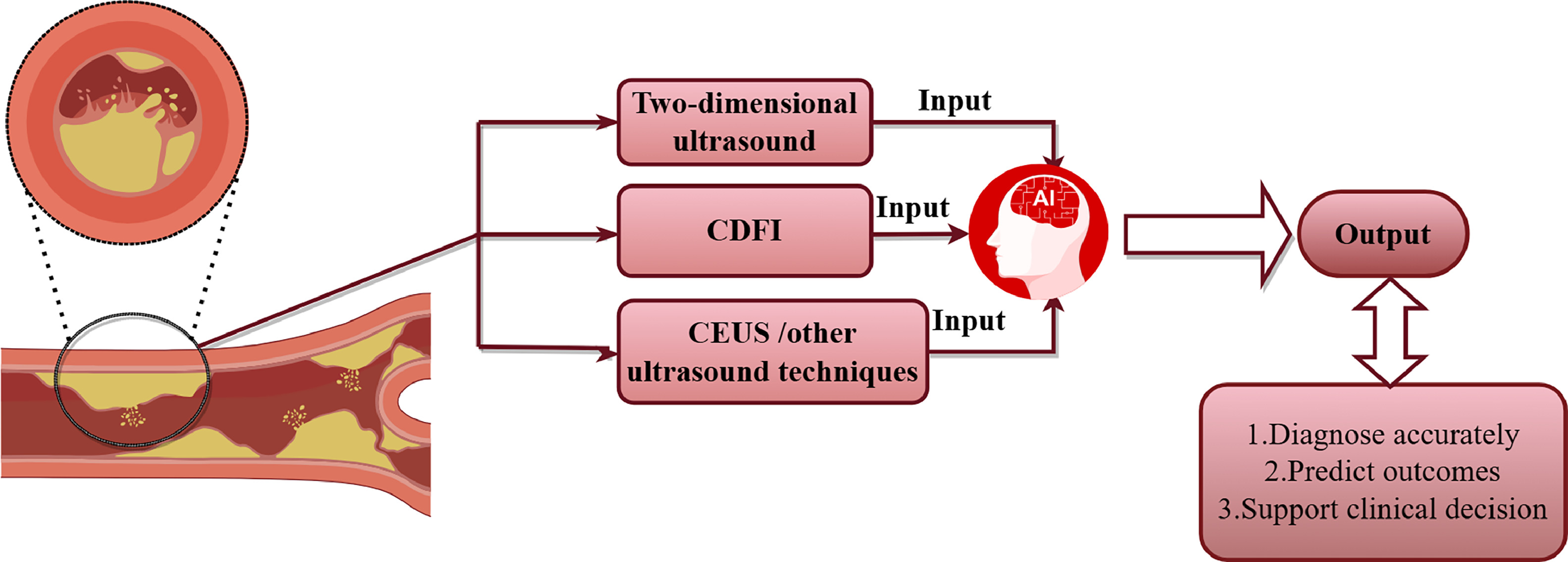
Application to carotid IMT and plaque measurement
Measurement of intima and plaque area. IMT is important for the early diagnosis of AS. Several researchers developed a classification model for IMT measurements using a CNN algorithm and validated the model on the carotid ultrasound images of 153 patients, which ultimately resulted in 89.1% accuracy in the classification of IMT [ ]. This demonstrates the efficiency and reliability of AI at identifying and measuring IMT. AI also enables automated and accurate measurements of IMT, reducing human error and improving measurement consistency. For example, Suda and colleagues selected bi-lateral carotid ultrasound images of 110 patients and used a CNN algorithm to identify and measure IMT in distal arteries, which showed an average difference of only 0.08mm [ ]. Yang et al. proposed automated segmentation based on DL of the lumen and media-adventitia [ ], as well as the use of AI-assisted ultrasound to evaluate vascular lumen measurements [ , ], which could be valuable for monitoring AS.
Measurement and delineation of plaque area is considered an important predictor of stroke risk [ ]. Initially, manual delineation of measurement boundaries was both time-consuming and error-prone. Therefore, Suri’s team designed CALEXia , an algorithm for automated plaque measurement [ ]. Later, Suri’s team commercialized their algorithms as AtheroEdge ( AtheroPoint, CA, USA) and deployed in clinical studies. Based on this system, a new solution was proposed by Biswas et al. They created an AI model using DL for the joint assessment of carotid artery wall thickness and plaque area measurements, which showed higher performance compared with previous techniques [ ]. On the other hand, Mainak and colleagues investigated how better measurement of IMT/plaque area with the help of ML and DL could potentially become a recommended parameter for ultrasound assessment of AS [ ]. AI can quickly process a large number of carotid ultrasound images and automate the measurement of IMT and plaque areas, significantly reducing time and labor costs for physicians, especially when mass screening is required. Additionally, AI-assisted ultrasound can provide physicians with more accurate data to support more scientific decisions in the early diagnosis of AS and stroke risk assessment.
Plaque component identification and image segmentation
Plaque composition is complex, and composition identification is significant for disease diagnosis and treatment. Lekadir et al. constructed a CNN model to automatically extract information from ultrasound images that is most suitable for identifying different plaque components. The correlation between model assessment and clinical assessment was reportedly found to be 0.90 after training and validation [ ]. The application of CNN models has demonstrated significant advantages in plaque component identification, enabling automatic extraction of key features and improving diagnostic accuracy and consistency. Yao et al. established Be Easy to Use (BETU), a CNN model for automatic plaque identification based on ultrasound dynamic images, and tested the application remotely in a 5G environment. The results showed that the diagnostic accuracy of BETU was 98.5%, with diagnostic compliance with radiologists (κ = 0.967, p < 0.001) [ ]. Therefore, combining 5G technology and AI makes remote medicine more convenient and efficient.
Plaque segmentation is essential for plaque feature extraction and identification; manual segmentation is inefficient and lacks precision. Accurate and efficient segmentation of vessel and plaque images is crucial for better plaque risk assessment. In 2015, some researchers used 2-D ultrasound and CEUS to automatically segment plaques but did not use artificial intelligence [ , ]. Commonly used end-to-end segmentation network architectures are UNet, SegNet and UNet variants, which are based on DL models and achieve direct prediction from the input image to the segmentation result, with high efficiency and accuracy, and are often applied to medical image segmentation. UNet performs well in small-sample learning compared with other DL methods, and the network structure is straightforward and easy to use. SegNet is based on the CNN model, which can maintain the integrity of high-frequency details in segmentation on the basis of UNet with lower storage cost but high computational complexity and limited segmentation ability on small-size objects.
Researchers used the UNet framework to segment images of early carotid plaques between different ethnic groups with an AUROC of 0.95 ( p < 0.001) [ ]. Zhou et al. proposed a DL-based UNet++ integration algorithm to segment carotid plaques in ultrasound images and to measure the total plaque area [ ]. Furthermore, on this basis researchers used the SegNet-UNet architecture for automatic plaque segmentation, training and prediction of plaque regions, and quantification of total plaque area. It was finally verified that the architecture could complete plaque segmentation in less than 1 s [ ]. Vila et al. segmented and verified ultrasound images based on densely connected CNNs with 96.45% accuracy [ ]. These improvements in levels of automatic segmentation [ , ] are significant for accurate clinical diagnosis and timely treatment. Despite the superior performance of UNet and SegNet architectures in image segmentation, the application of these architectures in medical image segmentation needs to be further standardized and optimized, especially in terms of applicability to different devices and imaging conditions.
In summary, AI significantly improves measurement accuracy and diagnostic efficiency in carotid IMT and plaque area, reduces human error, and enhances diagnostic consistency. AI automatically identifies plaque components and accurately segments them, providing support for large-scale screening and personalized treatment, and promoting the development of precision medicine.
Risk assessment and classification of plaques
To better assess plaque risk, in 2013 Suri’s team presented a data mining framework based on echoes of plaque ultrasound images for automated classification to stroke risk assessment [ ]. From the histological features and ultrasound characteristics of the plaques, the researchers hypothesized that symptomatic plaques would have a lower greyscale median due to larger lipid cores, less calcification and a more chaotic representation due to the frequent presence of neovascularization. In contrast, asymptomatic plaques typically appear hyperechoic on ultrasound and have a higher greyscale median. Based on this hypothesis, the authors designed and developed a DL model to identify and classify carotid plaques as symptomatic or asymptomatic, with classification accuracy and an area under the curve of 89.7% and 0.91, respectively ( p < 0.0001) [ ]. However, the results of this study were based on the assumption that symptomatic plaques are heterogeneous and have a more chaotic representation on ultrasound images. It is worth noting that symptomatic or asymptomatic symptoms still need to be dependent on detailed clinical assessment and the source of patient symptoms needs to be combined with new cerebral magnetic ischemic lesions location to make a comprehensive judgment. The ability of the DL model to accurately distinguish between stable and vulnerable plaques is valuable for the early identification of high-risk patients and prevention of cardiovascular events. Araki et al. proposed an effective system for stroke risk assessment. The system was analyzed based on ML-trained proximal and distal plaques in grayscale carotid ultrasound and used lumen diameters to create labels for stroke risk stratification. The final accuracy of distal and proximal plaques was 98.05% and 97.53%, respectively, and the classification accuracy was 95.08% and 93.47%, respectively [ ]. Likewise, Mainak’s team proposed a method to measure IMT/plaque area for stroke monitoring [ ]. Su and colleagues mainly used ultrasound features such as plaque thickness, plaque echo and fibrous cap thickness to classify and evaluate the plaques of stable and vulnerable plaques using two pre-trained DL models, both of which achieved more than 90% accuracy [ ]. AI can effectively enhance risk assessment and patient classification for carotid plaque, supporting early intervention and personalized treatment.
Limitations, challenges and prospects
Taken together, AI-assisted ultrasound could improve plaque diagnosis accuracy, assess patient prognosis and predict risk of stroke. However, there are no uniform standards for ultrasound assessment of vulnerable plaques. We hope that further research will establish uniform scoring criteria and combine them with AI for more objective, comprehensive and diagnostic results.
Despite the considerable promise of AI as a tool to enhance ultrasound diagnosis of carotid AS plaques, it still has a long way to go. The preceding paragraph of this review provided a brief overview of the advantages and disadvantages associated with various types of AI algorithms. Furthermore, there are some limitations to applications of AI the diagnosis of carotid AS plaques that should be carefully considered. First, it should be noted that AI is reliant upon the availability of high-quality and diverse data. The application of AI-assisted ultrasound may be hindered by complex, noisy or poor-quality ultrasound images, which may potentially lead to a reduction in diagnostic accuracy. It is important to distinguish whether the AI analysis is based on static or dynamic images. Most algorithms mentioned in this review use static images, and while static images provide detailed section feature parameters, they have the limitation of lacking temporal information. In cardiovascular ultrasound in particular, images of different cross-sections may show different feature parameters, and relying only on static images of a single cross-section may miss some key dynamic changes, thus limiting the generalization ability of the model. On the other hand, dynamic images may suffer from inaccurate positioning due to motion artifacts, which could affect the diagnostic process. Second, the morphology and lesions of the carotid artery, including the shape, size and location of atherosclerotic plaques, exhibit inter-individual variability. It is essential for AI to accurately adapt to these variations, but current algorithms may fail to capture all possible heterogeneous patterns. Additionally, some scientists believe that AI’s easy access to data may pose ethical dilemmas. Data privacy and security issues should not be ignored, and the highly sensitive nature of medical data requires that it be fully utilized in a way that ensures privacy. Any lack of transparency of AI algorithms, that is, medical problems such as errors in the hidden layers between the input and output layers [ ], are difficult to track and solve. Moreover, the performance and generalization capabilities of AI algorithms require improvements to handle diverse and large-scale data, and their scalability must be enhanced to adapt to complex clinical cases. Current studies are mainly based on specific data sets. The stability and consistency of models under different hospital and equipment conditions remain a challenge. Finally, the risk of junior doctors over-relying on AI, potentially leading to skill degradation, and the need for clear accountability in algorithmic decision-making are critical issues that need attention. Although AI shows great potential in ultrasound diagnosis, its application still faces multiple challenges. Therefore, future research should aim to address these issues in order to fully realize the potential of AI in medical diagnosis.
Looking ahead, we believe that the application of AI combined with ultrasound has the following key development directions. First, multi-modal AI used both in imaging biomarkers and diverse data sources for comprehensive and accurate diagnostic information can improve diagnostic capabilities. This includes addressing the inadequacy of static and dynamic images; for example, through multi-modal data fusion techniques that combine multiple imaging data with non-imaging data to provide more comprehensive information. Second, joint imaging technology improves the diagnostic ability of complex diseases by integrating different imaging data, which can effectively compensate for the limitations of a single imaging modality and provide more accurate treatment plans. Third, deep reinforcement learning has great potential in ultrasound image segmentation, lesion detection and classification. The development of adaptive AI systems that automatically adjust parameters based on case characteristics can then improve diagnostic personalization. Such systems could provide more personalized diagnostic solutions by learning from a patient’s historical data, enabling optimal processing of both static and dynamic images. Similarly, real-time AI-assisted diagnostic systems provide real-time information on lesions by processing dynamic images in real time, and also offer immediate lesion assessment during ultrasound examination, aiding rapid decision-making. Last, the combination of AI with clinical data (such as patient history, laboratory results and other data) is also an important direction, providing more comprehensive and personalized diagnosis and treatment plans. These advancements are expected to bring significant innovations to clinical practice.
Conclusion
The combination of AI and imaging represents a transformative advancement in the field of disease diagnosis, with the potential to significantly enhance diagnostic accuracy and efficiency. The use of AI-assisted ultrasound diagnosis in particular can effectively reduce inter-observer variability. The ability of AI to learn from vast data sets and apply consistent criteria to image analysis can effectively reduce this variability and thus improve diagnostic reliability. This review emphasizes AI’s role in ultrasound diagnosis of carotid plaque, highlighting its ability to improve plaque detection accuracy and evaluate characteristics such as size, composition and vulnerability. AI-assisted ultrasound shows higher sensitivity and specificity at identifying high-risk plaques, which is crucial for preventing stroke and cardiovascular complications. AI’s advanced pattern recognition identifies subtle plaque differences that humans might miss, aiding in distinguishing between stable and vulnerable plaques. Despite these benefits, this review also acknowledges the challenges and limitations inherent in AI-assisted ultrasound diagnostics, including the need for diverse data sets, algorithm transparency and ethical considerations of AI-driven decisions. Addressing these concerns is essential to unlocking the full potential of AI in medical diagnostics.
In conclusion, AI-assisted ultrasound holds great potential in carotid plaque assessment, promising more accurate, consistent and timely diagnoses. However, the full integration of AI into clinical practice still requires continued research and validation, particularly in the evaluation of vulnerable plaques. We sincerely hope that our review will inspire further studies to explore the assessment of AI combined with ultrasound for analyzing vulnerable carotid plaques. With ongoing research and technological advancements, we are optimistic that AI-assisted ultrasound diagnostics will eventually be translated and disseminated into routine clinical practice, offering substantial benefits to both doctors and patients.
Conflict of interest
The authors declare that the research was conducted in the absence of any commercial or financial relationships that could be construed as a potential conflict of interest.
Acknowledgments
The authors would like to thank Figdraw ( www.figdraw.com ) for providing sources to draw Figures 2 and 3 of this manuscript. This work was supported by Science and Technology Bureau of Quanzhou (grant no. 2020CT003 ) and Special fund project of the Second Affiliated Hospital of Fujian Medical University (grant no. 2024BD1102 ).
Data availability statement
No data sets were generated or analyzed during the current study.
References
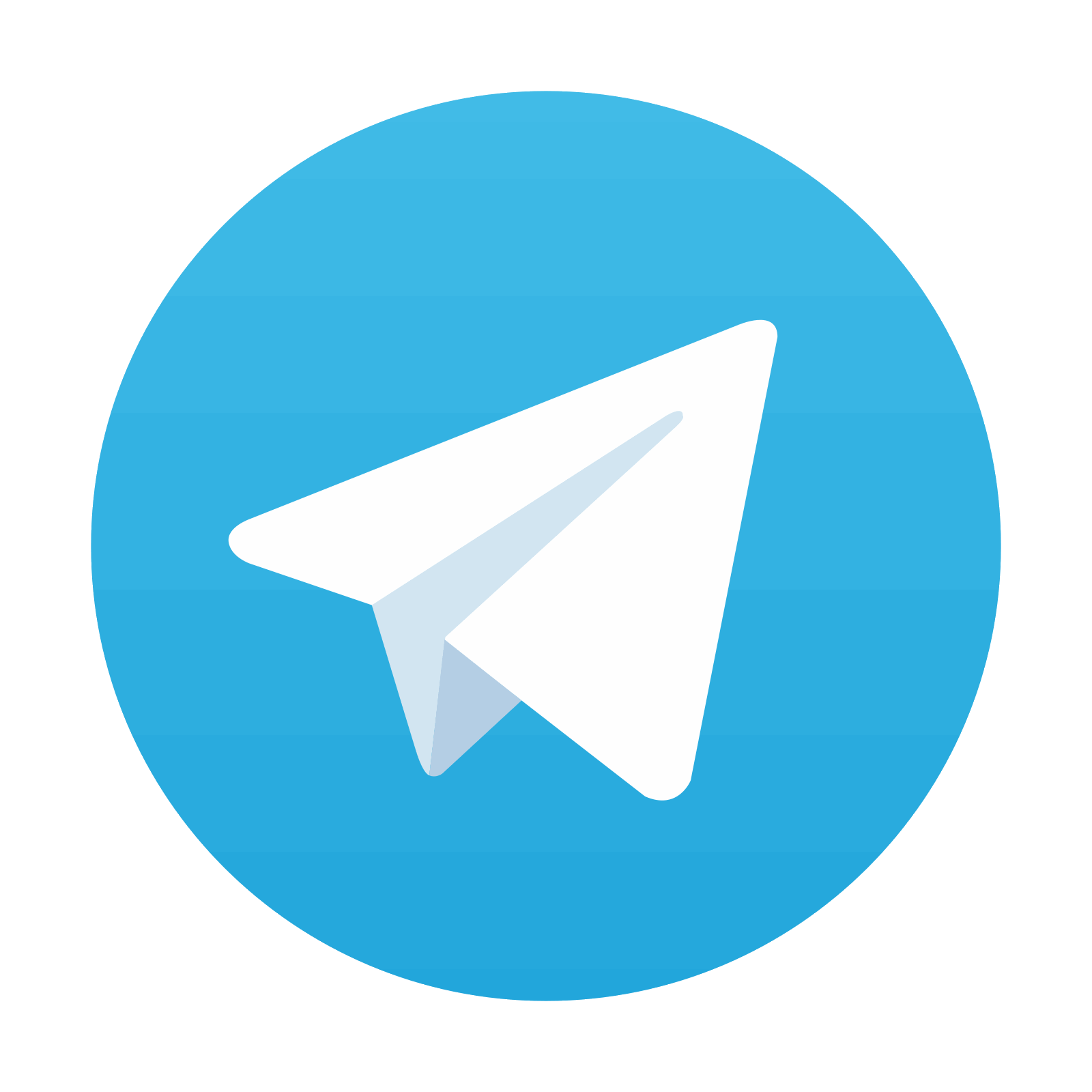
Stay updated, free articles. Join our Telegram channel

Full access? Get Clinical Tree
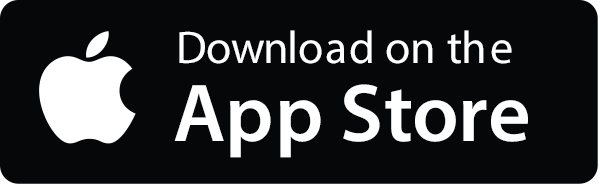

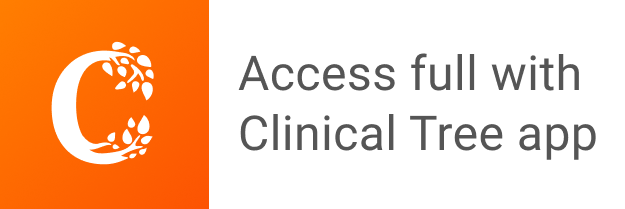