The radiology reporting process is beginning to incorporate structured, semantically labeled data. Tools based on artificial intelligence technologies using a structured reporting context can assist with internal report consistency and longitudinal tracking. To-do lists of relevant issues could be assembled by artificial intelligence tools, incorporating components of the patient’s history. Radiologists will review and select artificial intelligence-generated and other data to be transmitted to the electronic health record and generate feedback for ongoing improvement of artificial intelligence tools. These technologies should make reports more valuable by making reports more accessible and better able to integrate into care pathways.
Key points
- •
The radiology reporting process is beginning to incorporate structured data, including common data element identifiers for bringing a universal ontology to radiology reports.
- •
Artificial intelligence tools can assist with internal consistency, determine whether additional information is required, provide suggestions to make expert-like reports, and help with longitudinal tracking.
- •
A dynamic to-do list could be assembled by artificial intelligence tools to ensure that they are addressed by the current report.
- •
Radiologists will review and determine if artificial intelligence-generated data should be transmitted to the electronic health record, and generate feedback for monitoring and continuous tool improvement.
- •
Artificial intelligence has the potential to move the radiologists emphasis onto more advanced cognitive tasks while increasing the clinical relevance of reports for driving decision support and care pathways.
Introduction
The work product of radiology is the radiology report, which is still most typically a plain text, natural language description of the radiologists’ observations, synthesized impressions of the clinical situation, and possibly recommendations for further management of the patient. As artificial intelligence (AI) plays an increasing role throughout medicine, the data-oriented nature of imaging has made it an early target for application. Because the radiology report is the end-product of the interpretation process, the reporting process is a natural place for the application of AI technologies. This situation creates certain challenges to overcome, but also presents important opportunities for improving the reporting process. The challenges primarily center around the process of incorporating data into the reporting process, whereas the opportunities can relate to long-standing obstacles in creating high-quality radiology reports.
The radiology report has traditionally been natural language text generated by a radiologist via voice dictation. In recent years, the standard practice has moved toward structured reporting, but this practice has typically remained within the framework of plain text reports without associated structured data. This process presents a challenge to integrate results generated by AI tools into radiology results. Progress has been made in incorporating structured numeric and categorical data into the radiology reporting process and product. This process can manifest as a bilayered radiology report with a text layer resembling the current plain text radiology report along with a data layer, consisting of appropriately tagged structured data. The American College of Radiology (ACR) and the Radiological Society of North America have collaborated to create a registry of common data element identifiers for the purpose of bringing a universal ontology to this data layer. , Although these efforts are in an early stage, they will clearly form an important part of the foundation for incorporating AI tools into radiology reporting, and much of the further discussion will presuppose this kind of data structure underlying the radiology report.
Most of the progress toward implementing AI into radiology reporting in the recent past has been focused on leveraging simple sentences generated by AI imaging analysis tools and using natural language processing (NLP) tools for the annotation, summarization, or extraction of findings from reports. The creation of radiology reporting tools integrating convolutional neural networks for image analysis alongside recurrent neural networks for NLP to integrate structured data as part of the radiology result is an area of growing research interest. The new generation of AI-enabled NLP has made possible the extraction of structured data from the dictated text that radiologists are currently creating (or have previously created). This development could lead to a more sophisticated reporting system in which as a radiologist describes a finding using voice dictation, an NLP-based system automatically recognizes what has been described (eg, a hepatic lesion) and encodes the relevant attributes of that entity (eg, size, location, enhancement characteristics) with semantic labels. This process could help radiologists to evolve their practice of reporting to one underpinned by structured data.
This process leads to an opportunity for AI to be applied to several important problems in radiology reporting, such as that of internal report consistency. For example, a radiologist may describe a tumor in the left kidney in the body of their report, but then in their impression, misplace the lesion in the right kidney. Another common issue is the description of wrong-sex structures, such as the uterus in a man or a prostate in a woman. Once descriptions are captured as structured data, these descriptions can be analyzed for internal consistency and potential errors flagged for the radiologist to review before final signing. An even more common and dangerous scenario is radiologists describing the absence of a finding in the body of their report (or not describing a finding at all in the body of their report) and then, through a dictation error, seeming to indicate that such a finding is present in the impression. For example, a radiologist might say that the pleural spaces are clear in the body of the report, intend to say, “No evidence of pneumothorax” in the impression of their report, but owing to a voice recognition error, have the impression read “Evidence of pneumothorax.” With findings in both the body and the impression encoded by AI-enabled NLP using common data element-labeled data structures, separate AI-based tools could then assess for consistency and alert the radiologist to potential disagreements.
Specific report content may be expected in certain clinical scenarios. This content might include relevant characterizations or categorizations and possibly guideline-oriented recommendations for further work-up and management. AI tools can assess data-based descriptions of lesions and determine whether the descriptions would be considered complete or additional characterization or assessment is required. In addition, by encoding published guidelines for further management, these tools could lead to more guideline-compliant radiology reports. The open computer-assisted reporting/decision support (CAR/DS) framework promulgated by the ACR provides a foundation for early assistance with such suggestions, but must be encoded manually. AI tools could take this even further by helping to “discover” new guidelines to assist radiologists to improve their reporting. For example, AI tools may examine what the reporting patterns are for specific clinical entities used by experts (ie, how experts characterize and assess the lesion, and what recommendations they make for further characterization). These tools could be deployed for less expert radiologists; when the AI tool recognizes the relevant clinical scenario from the underlying data structures, it can provide suggestions to readers as to how to make their report more like reports generated by experts.
An additional challenge AI tools could help address is the longitudinal reporting of lesions, which is especially important in oncologic imaging. Specifically, many radiology examinations are ordered to reassess findings seen on prior imaging examinations. An important issue then is how to compare the findings being described in the current examination with the findings from prior examinations. Currently, radiologists have been responsible for examining the prior report text, determine which lesions require follow-up, correlating the current findings with previous ones, and describing their evolution (in many cases by redictating measurements from prior reports). For complex cases with many lesions, this process can be quite tedious and time consuming. An AI tool could examine a data structural description of a lesion and compare it with lesions described in multiple previous reports and try to construct the sequence of measurements and assessments of a lesion on serial studies over time. This information could then be presented to the radiologist in a format such that the appropriateness of the associations created by the AI algorithm can be established, each lesion’s current properties confirmed, and any time-based trend assessed. Such tables, automatically generated from the data associated with prior reports without the need for data reentry, could even then be included in the report and made available to ordering providers and other registries for automatic import into care management or research systems.
A recent challenge in radiology reporting has been to make radiology results more accessible to patients. Both recent trends and federal law changes have increased the availability of radiology reports to patients. Radiology reports have not traditionally been intended for patient consumption and tend to include language that is opaque to nonmedical professionals. Having radiologists manually create an additional work product exclusively intended for patient review is impractical. Thus, automated methods for making reports more comprehensible to laypeople would markedly improve the patient experience. AI tools could attack this problem in at least 2 ways. One would be direct natural language analysis of the report generated by the radiologist, providing links and definitions to annotate the original report that has been developed. As more sophisticated NLP becomes available, the sophistication of this annotation could improve. Such annotation could also be markedly improved by leveraging associated structured data to create specific patient-oriented descriptions of the radiologist’s findings.
Challenges and opportunities in artificial intelligence for report preparation, assembly, and clinical integration
Although radiology examinations are typically ordered to address a specific clinical question, every patient has numerous open questions at any given time. Each radiology examination presents an opportunity to address many of these questions. A tool integrated with the reporting system that can make the radiologist aware of the patient’s open questions at the time of reporting and suggest how the current examination might help to provide relevant information for those questions could allow radiologists to markedly increase the clinical value of radiology reports. Such a tool would cross-reference the patient’s given problem list against a knowledgebase of possible findings that might be seen on the given examination. That is, for each problem on the problem list, the tool would consult a list of potential imaging findings filtered by the type of examination being interpreted. Some of these findings might be flagged as potentially pertinent negative findings in a patient with a particular condition. The reporting system could then examination the data structures of the current report and prompt radiologists to note the specific absence of relevant findings that might be of concern. For example, when reporting on patients with genetic syndromes predisposing them to specific lesions, an AI-based system could recognize that the report did not mention those lesions in the current report and prompt the radiologist to consider describing their presence or absence. As another example, patients on immunotherapy-based oncology treatments are prone to agent-specific complications that might be seen on chest or abdominal computed tomography (CT) scans. When a radiologist reports an abdomen CT scan in such a patient, an AI system could recognize that the radiologist has not described the presence or absence of those complications and bring that to the radiologist’s attention (possibly including reference material on typical CT appearance of these complications). This means that, even though an examination was ordered for the specific condition, the resulting report can answer not only the main clinical question, but many more of the relevant clinical questions for the patient. A related phenomenon would be to help the radiologist by identifying findings and recommendations from prior radiology reports that are likely to be detected on the current examination. These could then be cross-referenced against the findings described in the current report to determine whether those issues had been addressed, and possibly point out to the radiologist the opportunity to describe them (or even insert an “unchanged” statement automatically). For example, if a patient with a history of an incidental pulmonary nodule has a chest CT scan to assess for pulmonary embolus in the emergency department, the radiologist might concentrate on the pulmonary vasculature and other acute findings and not comment on the previously seen pulmonary nodule. Such a tool could identify this opportunity for the radiologist, which might help to prevent future, unnecessary imaging. Extending this further, because NLP tools are applied to prior radiology reports, patterns can be identified that in aggregate provide insights that could be missed when seen in isolation. For example, intimate partner violence could be predicted with high specificity around 3 years before violence prevention program entry using such techniques on the basis of the distribution and imaging appearance of the patient’s current and past injuries. ,
Note that this interaction between the patient’s broad clinical history and the imaging findings could also be bidirectional. As a radiologist (or an image-analyzing AI tool) identifies a specific finding, this information may suggest additional diagnoses that are not currently attached to the patient. These new diagnoses could possibly be corroborated by other results (prior imaging results, previous laboratory results, physical examination findings, documented genetic alleles) that have not been connected previously. To accomplish this goal, a system would have to be designed that could cross-reference new findings to diagnoses, identify potentially new diagnoses, and know which related findings to query the electronic health record (EHR) for, and then bring these potentially new diagnoses to the radiologist’s attention. This can form a virtuous cycle, where EHR entities can prompt the radiologist to look for possibly related findings, and findings can suggest the possibility of new diagnoses.
In summary, as a radiologist works on crafting a report for an examination, a suite of AI tools can assemble for them a sort of to-do list of issues to be addressed. This list could include questions prompted by the specific question or history as contained in the order, but such a tool will become more useful the more it can incorporate other components of the clinical context. These components should include specific diagnoses that have been attached to the patient, as well as prior treatments a patient has received (eg, surgery, radiation therapy). In addition, many of the findings and recommendations described in prior radiology reports should be addressed in subsequent reports, even if only to note resolution. Finally, as the radiologist describes findings in their report or image analysis tools create putative new findings and encode them for the radiologist to consider including them in the report, further potential issues can be raised. Taken together, this process forms a sort of dynamic list of issues that the report should be addressing. As the radiologist adds content to the report, each new addition might address one of these open issues, or create new issues to address, or both. When a radiologist thinks their report is complete, they can see that all the relevant issues have been addressed and sign their report with confidence that their report is providing maximum value for patients and providers.
One new challenge that arises for radiologists as reporting transitions from pure text generation is the process of managing data flow from upstream sources into the patient’s clinical data as represented in the EHR. AI-based image analysis tools will create data structures based on the features automatically extracted from pixel data. In fact, such AI-generated data elements content can leverage existent standards such as the CAR/DS through the ACR Assist initiative also for integration into radiology reporting systems. , A new important role for the radiologist as they interpret images and generate report data will be to review these data structures and determine which should be included in the data to be transmitted to the EHR. This inclusion can take the form of either sub rosa inclusion in only the data layer, but also might be included as natural language (or part of a table) in the findings section of the text report. An additional important step for the radiologist to carry out is to provide feedback on the generated data, where appropriate. That is, a radiologist might reject the finding (eg, if the AI tool has recognized an artifact) or correct some of the data parameters (eg, changing a measurement). In addition, an AI tool might not be able to create complete descriptions of the findings it recognizes, and the radiologist must complete the description with additional data elements. These combined data could be used to automatically generate elements to be included in the text report or remain within the data layer to be transmitted to the EHR as structured data.
For example, consider the case of an AI tool that automatically detects and partially characterizes adrenal lesions. The generated data from the output of this tool (including descriptors for the side of the lesion, its size, and its radiodensity) would be automatically fed into a CAR/DS tool integrated into the reporting environment. As the radiologists reports on a case where such a lesion has been detected automatically, they can be alerted to the putative lesion. They can then confirm the lesion is a true lesion (rather than artifact) and be prompted to confirm the measurements and enter additional information about the lesion (eg, whether it has specifically benign features or has documented stability from prior examinations). Based on this additional information, the CAR/DS tool can then propose both report text for the radiologist to insert into the report and propose guideline-compliant recommendations for further management of the lesion ( Fig. 1 ). In addition, the complete structured data characterization of the lesion is stored, blending both AI-tool generated data and information elicited from the radiologist.
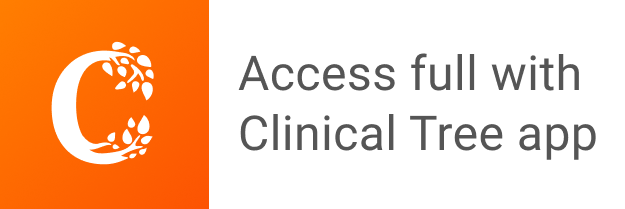