Radiologists have been at the forefront of the digitization process in medicine. Artificial intelligence (AI) is a promising area of innovation, particularly in medical imaging. The number of applications of AI in neuroradiology has also grown. This article illustrates some of these applications. This article reviews machine learning challenges related to neuroradiology. The first approval of reimbursement for an AI algorithm by the Centers for Medicare and Medicaid Services, covering a stroke software for early detection of large vessel occlusion, is also discussed.
Key points
- •
The most recent advances of AI in neuroradiology are presented.
- •
These include applications related to differential diagnosis, image acquisition, prediction of genetic mutations, lesion quantification, identification of critical findings, prognostication, and others.
- •
A brief review of machine learning competitions in neuroradiology is given.
- •
The first case of reimbursement for an AI algorithm is described.
Introduction
Radiologists have been at the forefront of the digitization process in medicine. Artificial intelligence (AI) is a promising area of innovation, particularly in medical imaging. Thus, it is no surprise that the number of publications on this topic has increased more than 6-fold from 2007 to 2017. More than one-third of these articles relate to the central nervous system (CNS). The number of applications of AI in neuroradiology has also grown. This article illustrates some of these applications.
Machine learning (ML) competitions are a different approach to problem-solving in science. One of the advantages of ML challenges over classical hypothesis-driven research is that they encourage global collaboration. The result is often a set of out-of-the-box solutions that achieves state-of-the-art performance for the specified problem. Many societies have launched ML challenges across almost all radiology subspecialties. This article reviews those related to neuroradiology.
The first approval of reimbursement for an AI algorithm by the Centers for Medicare and Medicaid Services (CMS) was announced at the end of 2020, covering a stroke software for early detection of large vessel occlusion (LVO), which is discussed at the end of this article. ,
Neuroradiology examples
The number of AI applications in neuroradiology is increasing every day, which include differential diagnosis of diseases, improvements in image acquisition (both quality and time), prediction of genetic mutations from MR imaging, segmentation of anatomy to guide interventional procedures, segmentation to quantify CNS lesions, identification of critical findings to shorten notification time, prognostication of diseases, quality assurance of patient position during image acquisition, and many others. This section presents notable examples of these applications.
Differential Diagnosis
Some papers focus on diagnosis of a single disease, including psychiatric and behavioral disorders, such as attention-deficit/hyperactivity disorder (ADHD). In “A Multichannel Deep Neural Network Model Analyzing Multiscale Functional Brain Connectome Data for Attention Deficit Hyperactivity Disorder Detection,” the investigators use resting-state functional MR imaging to generate connectome maps in different scales, using these data to train a deep neural network to identify patients with ADHD, achieving an area under the curve (AUC) of 0.74 (95% confidence interval, 0.73, 0.76). There are also many articles about cognitive diseases, with “A Deep Learning Model to Predict a Diagnosis of Alzheimer Disease by Using 18 F-FDG PET of the Brain” as an example for Alzheimer disease (AD), in which an Inception-V3 convolutional neural network (CNN) was trained on nuclear medicine images, achieving 100% sensitivity and 82% specificity in predicting the final diagnosis of AD, an average of 75.8 months before the diagnosis, outperforming reader performance. Models like this may aid in the early diagnosis of these diseases, which may be difficult to pinpoint in the initial clinical stages, allowing patient selection for clinical trials looking for early treatment.
Algorithms used for differential diagnosis are also well known, including articles that compare their ability and performance to radiologists and neuroradiologists. In “Deep Learning for Pediatric Posterior Fossa Tumor Detection and Classification: A Multi-Institutional Study,” the investigators trained a ResNeXt-50 CNN model to detect and classify pediatric posterior fossa tumors, achieving an area under the receiver operating characteristic curve of 0.99 for tumor detection and an accuracy of 92% (F1-score of .80) for classification, which was higher than two of four radiologists in the study. A seminal paper “Neuroradiologist-level Differential Diagnosis Accuracy at Brain MRI” described an innovative way to use deep learning (DL) and Bayesian networks to diagnose 19 common and rare CNS diseases using only a few cases, comparing its performance to radiologists with different levels of training. The paper demonstrated a unique approach to creating models that make the differential diagnosis with just a few cases, breaking the established concept that AI requires large amounts of data. The follow-up to this study is the article “Subspecialty-Level Deep Gray Matter Differential Diagnoses with Deep Learning and Bayesian Networks on Clinical Brain MRI: A Pilot Study” in which they used a similar approach to diagnose deep gray matter disease, with the same performance as neuroradiologists. The investigators demonstrated how integrating DL and Bayesian networks can be applied to other diseases. These examples show us the capacity of a DL model to achieve a performance similar to neuroradiologists for some specific diseases, even complex and rare ones. The examples may aid the neuroradiologist in the differential diagnosis of some difficult cases and help radiology/neuroradiology fellows in their learning progress.
There are also some ML algorithms that may improve pathology detection, which may be used to increase radiologist’s detection rate and also for automated peer review or peer learning. One example is “Deep Learning for MR Angiography: Automated Detection of Cerebral Aneurysms,” where the investigators developed an aneurysm detection tool that improved the sensitivity in the external test set by 13% when compared with the initial reports, achieving 93% sensitivity at the cost of 6 false-positives per scan. One company has also developed a product that uses AI to help create a structured radiology report. Their product characterizes tumors in terms of localization, size, signal intensity in each MR imaging sequence, and other features; automatically populates editable combo boxes; and generates a structured report with the most likely differential diagnoses. If the radiologist disagrees with any of the features, they can be adjusted, and a new report is automatically generated. This approach could improve turnaround time and consistency of results for common diseases.
Image Acquisition
A separate article of this series was dedicated to improving image acquisition with AI. We briefly list some examples related to neuroimaging, such as techniques for imaging quality improvement. As shown in “Improving the Quality of Synthetic FLAIR Images with Deep Learning Using a Conditional Generative Adversarial Network for Pixel-by-Pixel Image Translation,” a conditional generative adversarial network was used to improve the quality of synthetic fluid-attenuated inversion recovery (FLAIR) images (SyMRI version 8.0; SyntheticMR, Linköping, Sweden), with increment in image contrast and fewer granular/swelling artifacts, while preserving lesion contrast. Another example is “Improving Arterial Spin Labeling by Using Deep Learning,” which leveraged a CNN to generate higher-quality perfusion images, with 40% lower mean squared error than the conventional method. The reconstructed images also had less noise and motion artifacts ( P <.001).
Gadolinium-based contrast media are part of the cost of some MR imaging examinations, and there is also an increasing concern regarding gadolinium deposition in the brain. In “Deep Learning Enables Reduced Gadolinium Dose for Contrast-Enhanced Brain MRI,” a DL model was trained to generate a full-dose T1-weighted sequence from the same sequence with only 10% of the conventional contrast dose. The results are promising, and the investigators continue to improve the model with more data.
Many researchers have focused on generating one MR imaging sequence from another or generating computed tomography (CT) from MR imaging, and vice versa. Although the feasibility and reliability of some of these applications remain uncertain, generating a CT scan from MR imaging to plan radiotherapy seems to hold promise; this happens because there is no need to perfectly characterize the texture of bone lesions, but only to map the electron density of the head of the patients, which is highly dependent on the shape and thickness of the skull, as shown on “Generation of Synthetic CT Images from MRI for Treatment Planning and Patient Positioning Using a 3-Channel U-Net Trained on Sagittal Images.”
In “3D Deep Learning Angiography (3D-DLA) from C-Arm Conebeam CT,” the model accurately generated the vasculature of 3D rotational angiography without a mask, reducing radiation exposure and misregistration artifacts. Also, in “A Deep Learning-Based Approach to Reduce Rescan and Recall Rates in Clinical MRI Examinations,” the DL model was able to identify MR imaging sequences with motion artifacts to avoid rescan and recall. The investigators estimate savings of $24,000 per scanner per year if the model is used in clinical practice.
All these examples show how these algorithms may aid in reducing both the physical exposure of the patients and the financial costs in radiology departments, which may improve the quality of the medical care.
Prediction of Genetic Features from MR Imaging
Recent discoveries have shown MR imaging contains information predictive of genetic mutation in CNS tumors, such as high specificity of the T2-FLAIR mismatch sign for isocitrate dehydrogenase-mutant astrocytomas, raising the possibility that DL could play a role in predicting tumor genomics. In “Predicting Deletion of Chromosomal Arms 1p/19q in Low-Grade Gliomas from MR Images Using Machine Intelligence,” the investigators show that a multiscale CNN architecture was trained on T2 and postcontrast T1-weighted images, achieving 87.7% accuracy in predicting 1p/19q codeletion in low-grade gliomas. In the paper “Residual Deep Convolutional Neural Network Predicts MGMT Methylation Status,” a ResNet50 was trained to predict O6-methylguanine methyltransferase (MGMT) methylation status from routine T2-weighted images, with simpler preprocessing steps, achieving an accuracy of 94.9% ± 3.92%. Chang and colleagues used T2, FLAIR and T1-weighted precontrast and postcontrast images of both high- and low-grade gliomas to train a CNN to predict IDH1 status, MGMT promoter methylation, and 1p/19q codeletion, achieving 94%, 83%, and 92% accuracy, respectively. The important clinical implication of all these examples is that image-based predictors of tumor genomics might offer prognostic information or even identify tumor subgroups amenable to targeted therapies or eligible for new drug therapy clinical trials, which may improve the clinical outcome of these patients.
Applications of Segmentation
Segmentation is a pixelwise classification. It is useful to delineate lesions and measure their area and volume. In the past years, many applications have been designed for segmentation in neuroradiology, some of them already assimilated in everyday use, whereas others only in the research field.
Some articles emphasize the time reduction of such algorithms. One of them is “Deep Learning-Based Automatic Segmentation of Lumbosacral Nerves on CT for Spinal Intervention: A Translational Study,” where the researchers developed and validated a segmentation model for the 3D reconstruction of the safe triangle and the Kambin triangle, which are targeted areas for transforaminal epidural steroid injection, primarily at the L5/S1 level. By automating this task, it could be feasibly applied in clinical practice because manual segmentation is time consuming. Another example is “Deep Learning for Automated Delineation of Pediatric Cerebral Arteries on Pre-operative Brain Magnetic Resonance Imaging,” in which a U-Net was modified to segment cerebral arteries in nonangiographic MR imaging sequences. Usually, manual delineation of critical structures is required by intraoperative navigation systems to avoid complications during surgery. This DL model achieved a Dice score of 0.75, and its inference time was around 8 seconds per patient, whereas manual segmentation took about 1 to 2 hours.
Many articles showed ML algorithms that could be useful tools to aid neuroradiologists in their clinical practice, such as “Three-Plane–Assembled Deep Learning Segmentation of Gliomas,” in which a U-Net was trained on the 2018 Multi-modal Brain Tumor Segmentation Challenge (BraTS) Dataset using the axial, sagittal, and coronal planes to segment the enhancing tumor, tumor core, and whole tumor, achieving mean Dice scores of 0.80, 0.84, and 0.91, respectively. This model also could be implemented in clinical practice to assist radiologists or neuro-oncologists to better characterize the tumors. In “Fully Automated Segmentation of Head CT Neuroanatomy Using Deep Learning,” a U-Net was trained to segment 11 intracranial structures, achieving Dice scores comparable to neuroradiologists, even in external test sets with idiopathic normal pressure hydrocephalus. Also, in “Artificial Intelligence for Automatic Cerebral Ventricle Segmentation and Volume Calculation: A Clinical Tool for the Evaluation of Pediatric Hydrocephalus,” a U-Net was modified to segment the ventricles in T2-weighted images, achieving a Dice score of 0.901, and generalized to an external dataset. This model could be used to provide real-time clinical comparison and improve workflow, because the ventricular volume estimation can be used to objectively compare serial imaging in patients with hydrocephalus.
The segmentation calculated by the AI model could also be used as a biomarker to estimate clinical symptoms, as shown in “Convolutional Neural Network-Based Automated Segmentation of the Spinal Cord and Contusion Injury: Deep Learning Biomarker Correlates of Motor Impairment in Acute Spinal Cord Injury.” In this article, the segmentation models were trained on T2-weighted images of patients with spinal cord injuries and the automated volume estimate had a correlation with motor impairment in the acute phase. Another well-known use of AI is to increase radiologist’s performance with the use of ML algorithms. In “Deep Learning-Assisted Diagnosis of Cerebral Aneurysms Using the HeadXNet Model,” a 3D CNN was trained to segment cerebral aneurysms and the performance of clinicians was compared with and without AI. There were statistically significant improvements in sensitivity, accuracy, and interrater agreement in the group augmented by AI.
In “Improved Segmentation and Detection Sensitivity of Diffusion-weighted Stroke Lesions with Synthetically Enhanced Deep Learning,” the investigators compared the performance of segmentation of stroke lesions on diffusion-weighted imaging when training with real and synthetic data. The best Dice was achieved with the training set comprising real and synthetic images. The idea of using synthetic data is not new, but this article proves it works for this use-case. Use of synthetic data paves the way to the improvement of other segmentation models where annotated datasets are scarce.
Identification of Critical Findings
A well-established application of DL in medical imaging is the prioritization of studies with critical findings. In this section, we describe 2 seminal papers in this area. The first is “Automated Critical Test Findings Identification and Online Notification System Using Artificial Intelligence in Imaging,” which may be the first use of DL to prioritize critical findings. Two CNNs were trained, one to identify suspected acute infarct (SAI) and the other to detect hemorrhage, mass effect, and hydrocephalus (HMH) at noncontrast head CT. The SAI model achieved 62% sensitivity and 96% specificity, whereas the HMH model reached 90% sensitivity and 85% specificity. The main conclusion of this article was that AI holds promise for detecting critical findings, supporting further investigation with a prospective trial. The second paper is “Deep Learning Algorithms for Detection of Critical Findings in Head CT Scans: A Retrospective Study.” The models created in this work are able to identify all types of intracranial hemorrhage, skull fractures, midline shift, and mass effect. The models used a large dataset from India comprising 313,318 CT scans, achieving AUCs greater than 0.90 in most of the test sets.
Prognostication
Some studies have shown that DL can aid in determining the prognosis in a variety of diseases. Many articles were published trying to predict the prognosis of patients with brain tumor. One example is the “Multi-Channel 3D Deep Feature Learning for Survival Time Prediction of Brain Tumor Patients Using Multi-Modal Neuroimages,” in which multiple MR imaging sequences and demographic data were used to train a DL model that achieved 90.66% accuracy in predicting survival time of patients with high-grade gliomas, which was better than the standard of care.
Another hot topic is related to stroke characterization, patient treatment, and prognosis. In “Automated Calculation of the Alberta Stroke Program Early CT score: Feasibility and Reliability,” the investigators compared an automated ASPECTS calculator to 2 neuroradiologists and found that the software showed a higher correlation with expert consensus than each neuroradiologists individually ; this could help the treatment decision based on a more accurate score. In “Prediction of Tissue Outcome and Assessment of Treatment Effect in Acute Ischemic Stroke Using Deep Learning,” the DL model was better than other methods in predicting the final outcome (follow-up FLAIR image) based on the treatment and the MR imaging at admission, achieving an AUC of 0.88.
Patients with multiple sclerosis (MS) were also the focus of some studies, because they are subjected to frequent administration of gadolinium-based contrasts during their life. In “Deep Learning for Predicting Enhancing Lesions in Multiple Sclerosis from Noncontrast MRI,” the investigators aimed to identify active (enhancing) MS lesions from noncontrast MR imaging sequences, achieving a sensitivity of 72% and a specificity of 70%. Although this proof-of-concept study is insufficient to avoid contrast administration as of yet, it shows that noncontrast MR imaging contains information that can identify active MS lesions.
Machine Learning Competitions
ML challenges are complementary to hypothesis-driven research. Various ML competitions in the health care domain have occurred on many platforms, with Kaggle.com and Grand-challenge.org being the most well-known platforms. The Medical Image Computing and Computer Assisted Intervention Society has organized most ML competitions in neuroimaging. The Radiological Society of North America has launched competitions with the largest, publicly available, expertly annotated datasets comprising images from international research centers. Box 1 provides a list of the competitions related to neuroimaging.
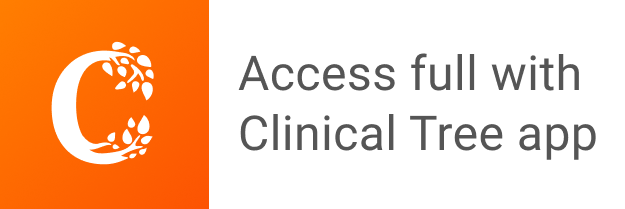