Abstract
Purpose
To investigate the reliability of ChatGPT in grading imaging requests using the Reason for exam Imaging Reporting and Data System (RI-RADS).
Method
In this single-center retrospective study, a total of 450 imaging referrals were included. Two human readers independently scored all requests according to RI-RADS. We created a customized RI-RADS GPT where the requests were copied and pasted as inputs, getting as an output the RI-RADS score along with the evaluation of its three subcategories. Pearson’s chi-squared test was used to assess whether the distributions of data assigned by the radiologist and ChatGPT differed significantly. Inter-rater reliability for both the overall RI-RADS score and its three subcategories was assessed using Cohen’s kappa (κ).
Results
RI-RADS D was the most prevalent grade assigned by humans (54% of cases), while ChatGPT more frequently assigned the RI-RADS C (33% of cases). In 2% of cases, ChatGPT assigned the wrong RI-RADS grade, based on the ratings given to the subcategories. The distributions of the RI-RADS grade and the subcategories differed statistically significantly between the radiologist and ChatGPT, apart from RI-RADS grades C and X. The reliability between the radiologist and ChatGPT in assigning RI-RADS score was very low (κ: 0.20), while the agreement between the two human readers was almost perfect (κ: 0.96).
Conclusions
ChatGPT may not be reliable for independently scoring the radiology exam requests according to RI-RADS and its subcategories. Furthermore, the low number of complete imaging referrals highlights the need for improved processes to ensure the quality of radiology requests.
Highlights
- •
ChatGPT is an artificial intelligence chatbot trained on vast text data.
- •
RI-RADS is a grading system that assesses the thoroughness of radiology requests.
- •
ChatGPT has poor reliability in scoring radiology requests according to RI-RADS.
- •
Most radiology requests are incomplete and lack useful information for reporting.
1
Introduction
The field of medicine is experiencing a major shift as artificial intelligence (AI) continues to mature. Large Language Models (LLMs) are a specific type of AI that leverage Natural Language Processing, a subfield of AI focused on computer-human language interaction [ ]. One such LLM attracting significant attention is ChatGPT, released by OpenAI in November 2022 [ ]. In radiology, ChatGPT displays exceptional capabilities in understanding, generating, and manipulating human language. This translates to promising applications such as generating structured radiological reports, potentially leading to improved efficiency through streamlined workflows, automated data extraction, and explanations of findings[ ]. However, it’s crucial to emphasize that formal validation and review by experienced radiologists remain essential for outputs generated by ChatGPT due to the possibility of errors [ ].
Radiologists rely on high-quality radiology request forms to choose the right imaging technique and interpret imaging examinations accurately and efficiently. This ensures a correct diagnosis and allows for appropriate guidance to referring physicians for further patient management [ ]. A recent development in this area is the Reason for exam Imaging Reporting and Data System (RI-RADS). This five-point grading system assesses the thoroughness of radiology requests based on the details provided in the request form. RI-RADS focuses on the presence of three crucial elements in the imaging referral: “impression”, “clinical findings”, and “diagnostic question” [ , ].
Due to the textual nature of radiology request forms, it is conceivable that ChatGPT could be leveraged for their evaluation, acting as a supportive tool for both referring physicians and radiologists in busy clinical settings. This study aims to investigate the reliability of ChatGPT in grading radiological examination requests using the RI-RADS criteria.
2
Material and methods
This research adhered to the ethical principles outlined in the Declaration of Helsinki (2013 revision). The study solely utilized anonymized data, eliminating the need for ethical committee approval due to the absence of patient-identifiable information. We conducted a single-center retrospective analysis aiming to identify consecutive imaging referral forms for inpatients. The timeframe spanned from October 2, 2023, and targeted 150 requests per imaging technique (computed tomography [CT], magnetic resonance imaging [MRI], and conventional radiography [CR]), resulting in a total of 450 requests. Our focus was restricted to inpatients as their unstructured radiological requests are directly entered into our electronic health record system by their treating physicians.
Two independent reviewers assessed all included imaging requests: a radiologist with six years of experience (as standard reference) and a radiology resident with three years of experience. Both reviewers assigned scores using the RI-RADS system, also collecting the evaluation of the three key categories per request. In fact, the RI-RADS score hinged on three key categories: 1) impression (e.g. differential diagnosis or working), 2) clinical findings (e.g. relevant medical/surgical history, signs and symptoms, pertinent laboratory tests, episode chronicity, and any available previous imaging reports), and 3) diagnostic question (e.g. pre-operative planning, exclusion/confirmation of a diagnosis, treatment response monitoring, disease staging, or follow-up assessment). RI-RADS A denotes the presence of all three key categories (adequate request), RI-RADS B indicates the presence of all three categories with poor clinical information (barely adequate request), RI-RADS C signifies the presence of only two key categories (considerably limited request), and RI-RADS D indicates the description of just one (deficient request). In RI-RADS X no key category is provided [ ].
Using the GPT 4 builder function, we created a customized RI-RADS GPT (available at https://chat.openai.com/g/g-QUMGg9DAU-ri-rads-gpt ) that was trained with specific instructions and an RI-RADS example for each grade (Supplementary Data). The task of this GPT was to provide, in response to the given inputs, only the RI-RADS score along with the evaluation of the three key categories. Additionally, the built GPT had access to the two scientific articles that introduced and tested RI-RADS on radiology examination requests in clinical practice [ , ]. The radiology examination requests included in the study were therefore copied and pasted as prompts in RI-RADS GPT without any modifications, within the timeframe between April 15 and 19, 2024.
2.1
Statistical analysis
To assess whether the distributions of the categories “RI-RADS”, “impression”, “clinical findings”, and “diagnostic question” differed statistically significantly between the radiologist and RI-RADS GPT, we used Pearson’s chi-squared test. Inter-rater reliability for both the overall RI-RADS score and its three constituent components was assessed using Cohen’s kappa (κ) statistic. This analysis evaluated agreement between the radiologist and the radiology resident, as well as between the radiologist and the RI-RADS GPT. Interpretation of the κ statistic followed a six-point scale: less than 0 denoted no agreement, 0.01–0.20 indicated slight agreement, 0.21–0.40 suggested fair agreement, 0.41–0.60 denoted moderate agreement, 0.61–0.80 indicated substantial agreement, and 0.81–0.99 signified near-perfect agreement [ , ].
3
Results
RI-RADS D was the most prevalent grade assigned by human readers (54% of cases), while ChatGPT more frequently assigned the RI-RADS C grade (33% of cases). Additionally, human observers more frequently indicated the lack of the “impression” and “diagnostic question” subcategories (69% and 59% of cases, respectively) and the incompleteness of the “clinical findings” subcategory (about 60% of cases). On the other hand, ChatGPT more frequently indicated the presence of the “impression” and “diagnostic question” subcategories (56% and 94% of cases, respectively) and the absence of the “clinical findings” subcategory (59% of cases). In 7/450 (2%) cases, RI-RADS GPT should have assigned an RI-RADS score of D instead of C based on the ratings given to the subcategories. The distributions of the RI-RADS grade and the subcategories differed statistically significantly between the radiologist and RI-RADS GPT (ρ-value <0.0001), with the exception of RI-RADS grades C and X. Table 1 summarizes the distribution of the collected data.
Radiologist | Radiology resident | RI-RADS GPT | |
---|---|---|---|
RI-RADS | |||
A | 4/450 (1%) | 5/450 (1%) | 37/450 (8%) a |
B | 27/450 (6%) | 33/450 (7%) | 111/450 (25%) a |
C | 142/450 (31%) | 135/450 (30%) | 148/450 (33%) |
D | 244/450 (54%) | 242/450 (54%) | 132/450 (29%) a |
X | 33/450 (8%) | 35/450 (8%) | 22/450 (5%) |
Impression | |||
Presence | 139/450 (31%) | 139/450 (31%) | 254/450 (56%) a |
Absence | 311/450 (69%) | 311/450 (69%) | 196/450 (44%) a |
Clinical findings | |||
Presence | 9/450 (2%) | 11/450 (2%) | 74/450 (16%) a |
Incomplete | 270/450 (60%) | 279/450 (62%) | 111/450 (25%) a |
Absence | 171/450 (38%) | 160/450 (36%) | 265/450 (59%) a |
Diagnostic question | |||
Presence | 198/450 (44%) | 196/450 (44%) | 422/450 (94%) a |
Absence | 252/450 (56%) | 254/450 (56%) | 28/450 (6%) a |
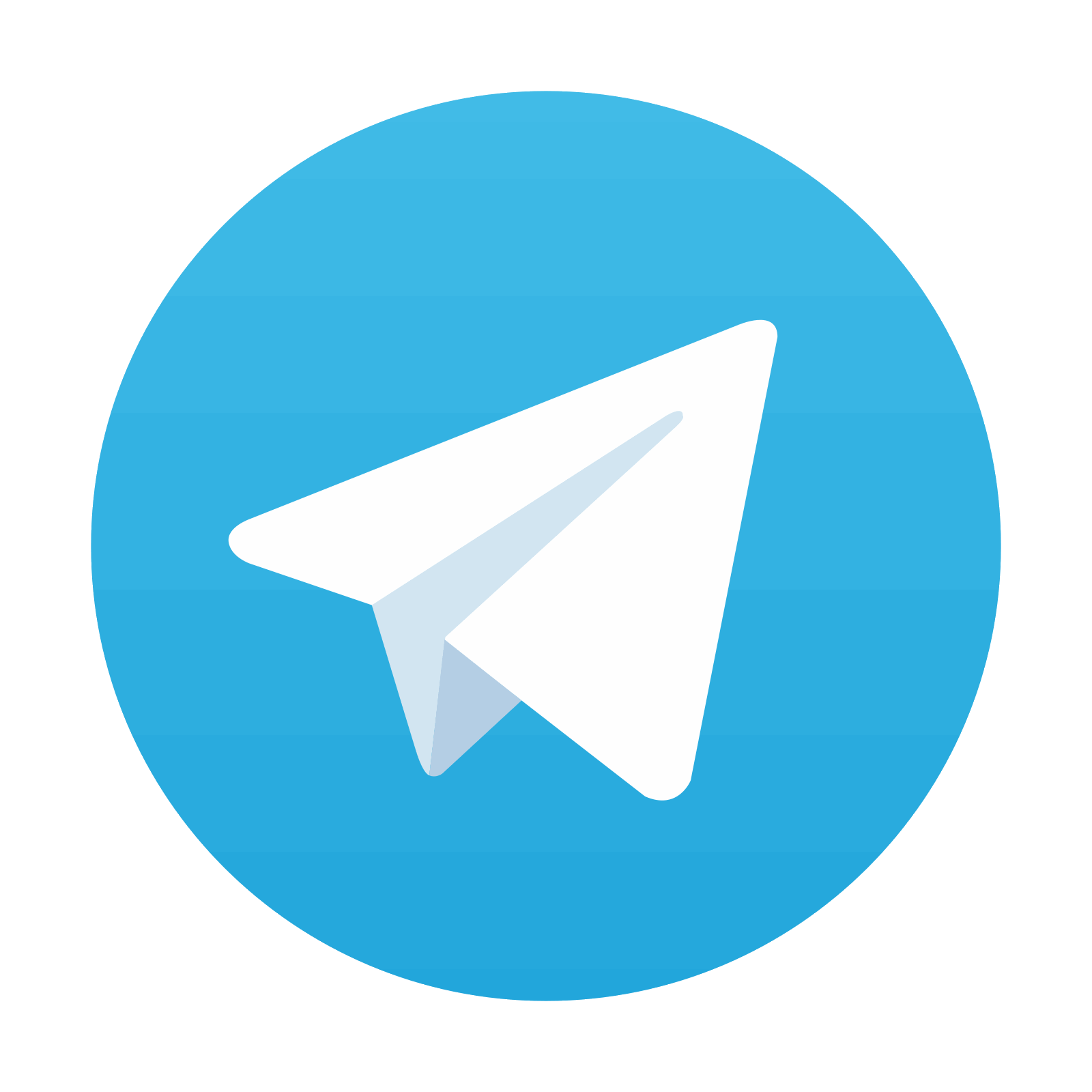
Stay updated, free articles. Join our Telegram channel

Full access? Get Clinical Tree
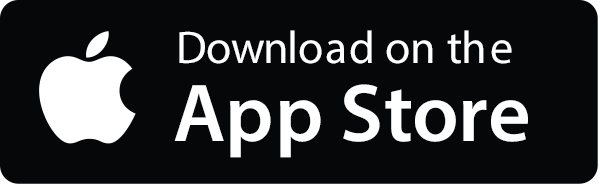

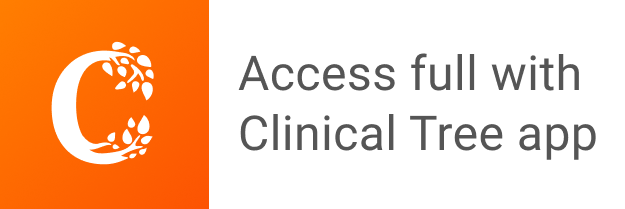