Abstract
Background
Skeletal muscle echo intensity (EI) is associated with functional outcomes in older adults, but resistance training interventions have shown mixed results. Texture analysis has been proposed as a novel approach for assessing muscle quality, as it captures spatial relationships between pixels. It is unclear whether texture analysis is able to track changes following resistance training.
Objective
To examine changes in first-order (EI) and second-order (texture) features of muscle quality following lower-body resistance training in older adults.
Methods
Twelve older adults (2 males, 10 females; mean ± SD age = 70 ± 5 years) completed 6 weeks of resistance training, consisting of twice-weekly sessions at 85% of estimated 1RM. Testing included ultrasound imaging of the rectus femoris (RF) and vastus lateralis (VL), 5-repetition maximum (5RM) leg extension strength, and maximal voluntary isometric contraction (MVIC) force. Ultrasound images were analyzed for EI and texture features using gray-level co-occurrence matrix (GLCM) analysis.
Results
Large improvements were observed in 5RM leg extension strength ( p < 0.001, effect size [ES] = 2.09), MVIC force ( p = 0.006, ES = 0.969), and RF EI (uncorrected: p = 0.003, ES = 0.727; corrected: p = 0.012, ES = 0.864). No significant changes were observed in muscle size, VL EI, or texture features for either muscle.
Conclusion
Short-term resistance training improved strength and RF EI. However, texture analysis features were not sensitive to changes following training. These findings suggest that traditional EI measures may be more appropriate than texture analysis for tracking changes in muscle quality following resistance training in older adults.
Introduction
The gradual, age-related loss of skeletal muscle mass and strength, a condition known as sarcopenia, will become increasingly pervasive as the global population ages [ , ]. Sarcopenia impairs functional capabilities, leading to adverse outcomes such as falls, fractures, and mortality in older adults [ , ]. While many associate sarcopenia with low muscle mass, prospective, longitudinal studies suggest that the loss of muscle strength is ∼3 × as rapid as the loss of muscle mass [ , ]. Given this divergent timeline, many have pointed to muscle quality (e.g., strength relative to muscle mass) as a more important indicator of functional status among older adults [ ]. While the exact mechanistic contributors to muscle quality are still under investigation, increases in inter- and intramuscular adiposity have been associated with poor functional outcomes [ ] and disease risk [ ]. Given the importance of assessing muscle quality throughout aging, accessible tools that provide insights into these characteristics are of significant value to the field.
Skeletal muscle quality can be non-invasively estimated using B-mode ultrasonography by quantifying the echo intensity (EI) of a specific region of interest [ ]. As skeletal muscle consists of both contractile proteins and non-contractile components (i.e., intramuscular adipose and fibrous tissue) [ ], conducting a gray scale analysis of a region of interest can be used to quantify the mean pixel intensity, with black and white pixels corresponding to high and low muscle quality, respectively [ ]. While EI has shown promise in cross-sectional studies, investigations that have examined changes following resistance training have shown mixed results. For example, Yoshiko et al. [ ] found that after 10 weeks of combined walking and resistance training, there was a significant decrease in EI in the quadriceps femoris among older adults. Conversely, Scanlon et al. [ ] found that there were no differences in EI for the rectus femoris (RF) and vastus lateralis (VL) in older adults following 6 weeks of progressive resistance training. The reasons why resistance training studies have produced inconsistent changes in EI could be related to a variety of factors, including different methodological approaches, research participants, and the nature of the intervention (i.e., training volume, intensity, etc.). Establishing reliable protocols that can be used to track muscle quality changes in older adults following resistance training remains a high priority.
Texture analysis of B-mode images offers a novel approach to characterize skeletal muscle tissue. It involves the mathematical characterization of spatial distribution and pixel intensities within a region of interest [ ], and may be used to describe the inherent textural structure of muscle composition [ ]. First-order features examine individual pixel intensities while ignoring spatial relationships [ ], and are evaluated by using the frequency histogram of pixel intensities. This approach provides a count of the number of times a pixel intensity occurs and can be used to calculate mean, standard deviation, skewness, and kurtosis of an ultrasound image [ ]. Although easy to implement and interpret, these features are limited in their ability to describe underlying texture features of a muscle. Second-order features resolve this limitation by accounting for both pixel intensity and spatial distribution between two pixels using the grey level co-occurrence matrix (GLCM) texture analysis feature. The GLCM is a mathematical feature which describes the joint occurrence of pairs of pixel intensities at specified pixel intensities. Common statistical features such as energy, entropy, contrast, homogeneity, dissimilarity, correlation, and variance can be extracted from the GLCM to characterize textures [ ].
Despite the potential of texture analysis to assess muscle quality, several key gaps persist. The comparative sensitivity of first-order versus second-order features in detecting changes after resistance training interventions remains unclear. Additionally, the effectiveness of texture analysis in tracking changes in muscle quality in older adults has not been thoroughly investigated. Therefore, the purpose of this study was to examine changes in first- and second-order (GLCM) characteristics of skeletal muscle quality after lower-body resistance training among older adults. To further characterize the influence that resistance training may have on muscle quality measures, we also examined changes in muscle strength and mass. We hypothesized that both first-order (EI) and second-order (texture) characteristics would show significant improvements after resistance training, and second-order characteristics would demonstrate greater sensitivity to changes in muscle quality compared to traditional EI measures.
Materials and methods
Research design
The data presented herein represents a subset of a more comprehensive investigation [ ]. Participants completed a supervised, 6-week training program in which they were randomly assigned to either a traditional or functional resistance training group. Participants underwent a familiarization and two pre-testing visits before the intervention to become acquainted with the exercises, study protocol, and establish inter-session test-retest reliability. Participants then visited the laboratory for resistance training twice per week for 6 weeks. All visits to the laboratory were separated by at least 48 hours, but not more than one week. A comprehensive testing battery was used to evaluate pre-post changes, which included ultrasonography of the VL and RF, isometric and repetition maximum testing, among others. The order in which the assessments have been described below corresponds to the order in which they were conducted. Testing sessions were conducted at the same time of day (± one hour) to minimize diurnal effects. Our study design carefully controlled for or monitored hydration levels, nutrition, and physical activity outside of the laboratory. For full details, the reader is directed to the work of Pagan et al. [ ].
Participants
Data from 12 older adults (2 males, 10 females; mean ± SD age = 70 ± 5 years, body mass index [BMI]: 26.5 ± 4.0 kg/m 2 ) has been presented in this manuscript. Exclusion criteria included lower body surgery or use of an assistive walking device within the past year, history of cancer, neuromuscular or metabolic diseases, and myocardial infarction within the past year. Eligible participants had not engaged in lower-body resistance training for at least 6 months prior to enrollment. All participants signed informed consent documents prior to engaging in study activities. This study was approved by the University of Central Florida Institutional Review Board (#STUDY00004684).
B-mode ultrasonography image acquisition and analysis
A portable B-mode imaging device (GE Logiq e BT12, GE Healthcare, Milwaukee, WI, USA) and a multi-frequency linear-array probe (12 L-RS, 5–13 MHz, 38.4-mm field of view, GE Healthcare) were used to obtain ultrasonography images of the dominant VL and RF (based on kicking preference) before and after the training intervention. The following device settings were kept consistent: gain = 50 dB, dynamic range = 72, frequency = 10 MHz. Image depth was standardized at 5.0 cm for all participants and visits, with the exception of one participant, who required an image depth of 6.0 cm to view the entire RF; however, increasing image depth from 5.0 to 6.0 cm likely had a trivial influence on study results [ ]. Moreover, probe tilt was monitored and kept consistent throughout the study, as recommended by previous investigators [ ]. VL images were captured along the lateral thigh, at a 50% of the distance between the greater trochanter and the superior patella. Participants laid on their non-dominant side of the body with their legs stacked and the dominant knee joint positioned at an angle of 15°. Participants laid supine on the treatment table while ultrasonography images of the dominant RF were captured along the thigh’s midline, at 50% of the distance between the anterior inferior iliac spine and the superior patella, using the panoramic function in the transverse plane. A high-density foam pad was secured to the participant’s thigh to ensure that the probe moved in a straight line along the transverse plane. Three images were taken of each muscle. The same investigator captured all ultrasonography images.
All images were analyzed using ImageJ software (ImageJ, version 1.51, NIH, Bethesda, MD, USA). The polygon function was used to quantify muscle cross-sectional area (CSA) for the VL and RF. Subcutaenous adipose tissue thickness (cm) over each muscle was measured using the straight line function, and corresponded to the mean of left, center, and right values. The EI values were corrected for subcutaneous adipose tissue using an equation created by Young et al. [ ]. A texture-based image analysis was employed to investigate the spatial variation in pixel intensity of both muscles. The GLCM characteristics were examined using a plugin macro (Texture Analyzer v0.4) for ImageJ. This plugin requires rectangle-shaped regions of interest for the GLCM analysis, with the goal of incorporating as much muscle as possible without including aponeurosis or bone. The GLCM computation was performed in four directions (0°, 90°, 180°, and 270°), and the resultant values were averaged to mitigate the effect of direction . Outcome parameters were the angular second moment (ASM), contrast, correlation, inverse different moment (IDM), and entropy. In short, increased ASM, correlation, and IDM values indicate high homogeneity within an image, and increased contrast and entropy indicate high heterogeneity within an image. Increased homogeneity in skeletal muscle typically indicates more dense muscle (less infiltration of connective tissue and adipose . Mathematical formulas for each parameter can be found in Table 1 of Sahinis and Kellis . Reported parameters were averaged across the 3 images at each location.
Dependent variable | Pretest #1 | Pretest #2 | p | ES | ICC 3,1 | SEM | SEM (%) | MD |
---|---|---|---|---|---|---|---|---|
Calculated from Pagan et al. [ ] prior to group randomization; N = 37 | ||||||||
Leg extension 5RM (kg) | 28.9 ± 13.5 | 32.9 ± 14.2 | <0.001 | 0.787 | 0.931 | 3.62 | 11.7 | 10.0 |
MVIC force (N) | 424.9 ± 151.3 | 430.5 ± 154.1 | 0.405 | 0.139 | 0.965 | 28.5 | 6.7 | 78.5 |
VL CSA (cm 2 ) | 16.5 ± 6.3 | 16.0 ± 5.2 | 0.248 | 0.193 | 0.927 | 1.55 | 9.6 | 4.30 |
RF CSA (cm 2 ) | 4.6 ± 1.7 | 4.3 ± 1.7 | 0.087 | 0.289 | 0.903 | 0.53 | 11.8 | 1.46 |
N=11 | ||||||||
VL Uncorrected EI (A.U.) | 107.6 ± 18.9 | 107.1 ± 18.3 | 0.618 | 0.155 | 0.901 | 7.99 | 6.43 | 22.2 |
RF Uncorrected EI (A.U.) | 107.3 ± 18.0 | 108.2 ± 18.1 | 0.975 | 0.001 | 0.734 | 9.40 | 10.4 | 26.1 |
VL Subcutaneous Thickness (cm) | 0.731 ± 0.406 | 0.766 ± 0.369 | 0.353 | 0.293 | 0.953 | 0.086 | 11.5 | 0.238 |
RF Subcutaneous Thickness (cm) | 0.942 ± 0.437 | 0.900 ± 0.408 | 0.373 | 0.281 | 0.963 | 0.084 | 9.0 | 0.231 |
VL Corrected EI (A.U.) | 152.5 ± 23.3 | 150.7 ± 22.0 | 0.367 | 0.272 | 0.881 | 8.18 | 5.3 | 22.7 |
RF Corrected EI (A.U.) | 149.1 ± 20.4 | 149.5 ± 18.9 | 0.411 | 0.247 | 0.582 | 9.02 | 7.0 | 25.0 |
VL ASM | 0.000573 ± 0.000102 | 0.00050 ± 0.000107 | 0.153 | 0.466 | 0.139 | 0.000098 | 18.1 | 0.00027 |
RF ASM | 0.000645 ± 0.000158 | 0.000698 ± 0.000251 | 0.311 | 0.322 | 0.331 | 0.00017 | 24.8 | 0.00047 |
VL Contrast | 353.9 ± 125.9 | 367.6 ± 105.6 | 0.607 | 0.160 | 0.772 | 56.6 | 15.7 | 156.9 |
RF Contrast | 204.4 ± 73.9 | 204.2 ± 86.6 | 0.427 | 0.250 | 0.771 | 36.4 | 18.4 | 101.0 |
VL Correlation | 0.000573 ± 0.000158 | 0.000535 ± 0.000163 | <0.001 | 4.47 | 0.431 | 0.00010 | 20.0 | 0.00031 |
RF Correlation | 0.000775 ± 0.000181 | 0.000872 ± 0.000291 | 0.078 | 0.543 | 0.662 | 0.00016 | 18.8 | 0.00044 |
VL IDM | 0.160 ± 0.016 | 0.152 ± 0.010 | 0.074 | 0.601 | 0.535 | 0.092 | 5.9 | 0.026 |
RF IDM | 0.182 ± 0.022 | 0.186 ± 0.027 | 0.312 | 0.321 | 0.668 | 0.014 | 7.4 | 0.038 |
VL Entropy | 8.06 ± 0.15 | 8.13 ± 0.24 | 0.465 | 0.229 | 0.293 | 1.70 | 2.1 | 0.47 |
RF Entropy | 7.92 ± 0.22 | 7.86 ± 0.26 | 0.152 | 0.241 | 0.561 | 0.15 | 1.9 | 0.41 |
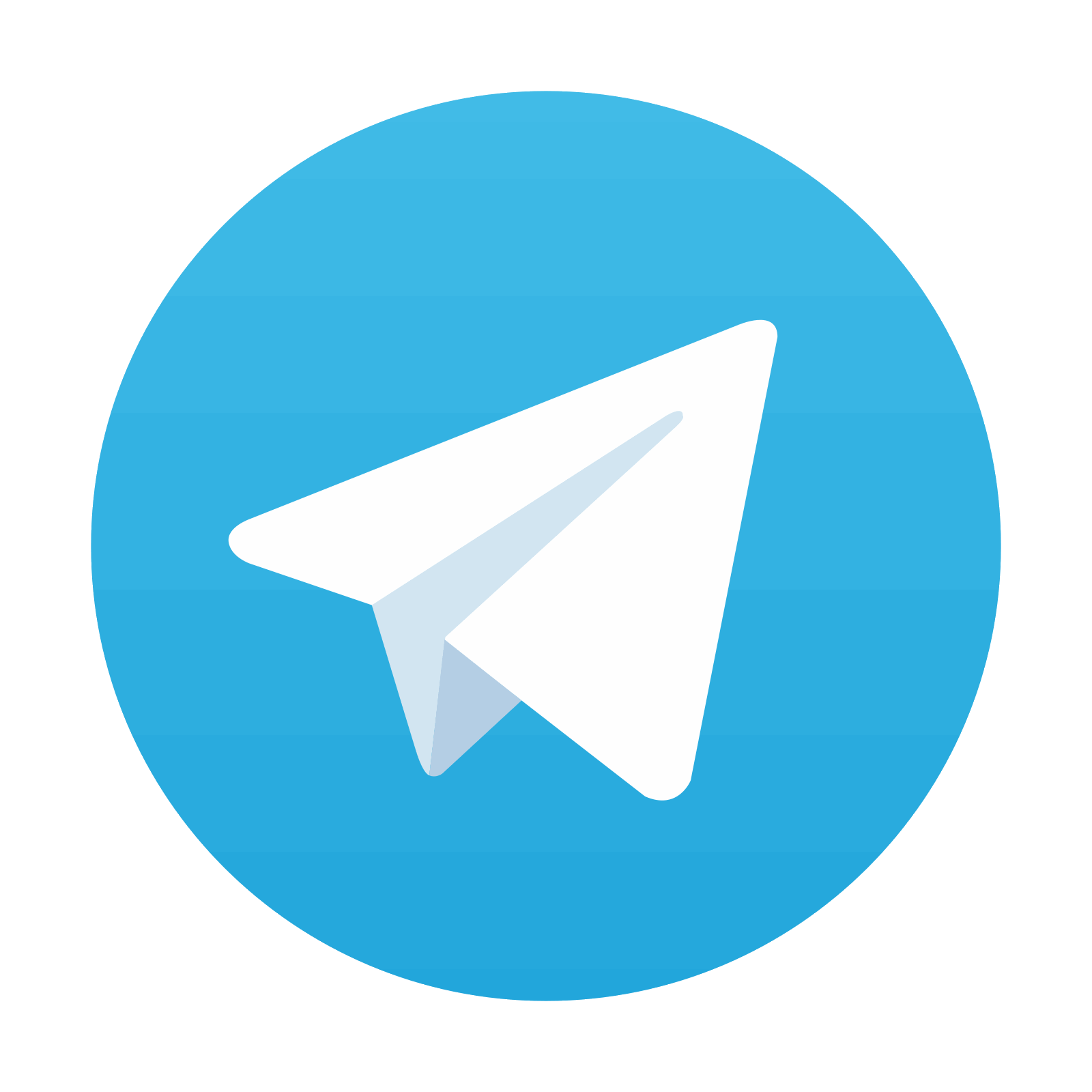
Stay updated, free articles. Join our Telegram channel

Full access? Get Clinical Tree
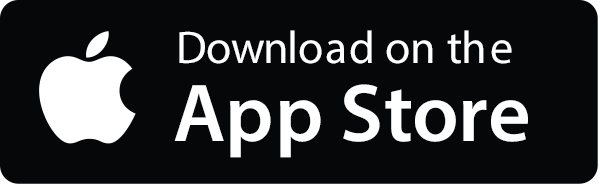

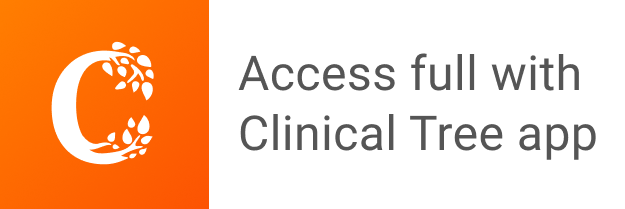