© Springer Science+Business Media New York 2016
Wim Van Hecke, Louise Emsell and Stefan Sunaert (eds.)Diffusion Tensor Imaging10.1007/978-1-4939-3118-7_1818. DTI in Psychiatry
(1)
INSERM U955 Team 15 “Translation Psychiatry”, Fondation Fondamental, APHP, Hôpitaux, Universitaires Mondor, Créteil, France
(2)
NeuroSpin Neuroimaging Center, UNIACT Lab, Psychiatry Team, CEA Saclay, Gif-Sur-Yvette, France
(3)
Translational MRI, Department of Imaging and Pathology, KU Leuven, and Radiology, University Hospitals, Leuven, Belgium
(4)
Universitair Psychiatrisch Centrum (UPC), KU Leuven, Leuven, Belgium
Keywords
DTI findings in psychiatric disordersSchizophreniaMood disordersAnxiety disordersPersonality disordersNeurodevelopmental disordersLearning Points
DTI is not used routinely in clinical practice owing to special challenges inherent to defining psychopathology, the practical issues associated with scanning patients and the lack of sensitivity and specificity of DTI measures. In the future, it could be used to inform invasive neurosurgical treatments of psychiatric illness, such as deep brain stimulation.
DTI is increasingly used as clinical research tool in psychiatry. It can be used to inform neurobiological models of psychiatric illness, such as those based on “connectivity.” DTI metrics can be used in combination with other neuroimaging data as potential biomarkers that may aid patient stratification and improve treatment.
DTI studies in psychiatry face a number of issues. Specifically, the categorical classification of mental disorders is subjective and definitions are continually evolving. Clinical samples are therefore highly heterogeneous with regard to clinical history, psychiatric and medical comorbidity, active symptoms, and medication. Alcohol misuse may represent a significant confound in studies of psychiatric populations.
Scanning psychiatric patients presents some practical challenges, including obtaining informed consent, reduced compliance with procedures owing to anxiety or hyperactivity, and increased movement within the scanner compared to healthy subjects.
DTI has been used to investigate a number of psychiatric disorders, including, but not limited to schizophrenia, mood, anxiety, personality and neurodevelopmental disorders. Findings are largely nonspecific and suggest varying degrees of white matter microstructural abnormality in cortical and subcortical cognitive and limbic networks.
Role of DTI in Psychiatry
Clinical Research
Presently, DTI is not used routinely in clinical psychiatric practice. There are a number of reasons for this, which broadly relate to both the complex nature of defining psychopathology and the practical challenges associated with scanning patients with mental illness. These issues are discussed in more detail in the body of this chapter. Nevertheless, DTI does have an increasingly significant role in psychiatry, and that is in the field of clinical research.
Early clinical neuroimaging studies in psychiatry used computed tomography (CT) and subsequently classical structural magnetic resonance imaging (MRI) (using T1, T2, and fluid attenuation inversion recovery—FLAIR—sequences) [1]. These studies have allowed us to better understand the volumetric changes present in psychiatric disorders such as schizophrenia, mood disorders (bipolar and unipolar disorders), anxiety disorders, addiction, personality disorders, autism, and attention deficit hyperactivity disorder (ADHD). As an example, in schizophrenia, we now know from these neuroimaging studies that global brain volumes are decreased in patients compared to controls, even before the first clinical episode [2, 3]. Regional volumes are also decreased, especially in the prefrontal cortex [4]. High-risk subjects are also a population of interest in these pathologies and are generally defined as healthy relatives of patients. They thus share some common genetic risk with the patients, but without the expression of the disease per se and without some confounding factors such as medication and number of episodes. Usually, these high-risk subjects share most of the same features regarding brain volumes, though at a lower amplitude than patients [5]. However, although such computational morphometry based studies are useful, they are unable to provide information beyond total and regional white matter volume, density, and shape.
Functional MRI has also provided insight into the mechanisms of psychiatric disorders, via the identification of over- or under-active areas during the completion of specific tasks in groups of patients [6].
Strikingly, T1, T2, FLAIR, and fMRI studies point to crucial abnormalities of white matter in major psychiatric disorders. On T1 scans , total white matter volume has been found to be reduced in schizophrenia, whilst regional volumetric reduction (e.g., corpus callosum) has also been reported in schizophrenia and in other conditions such as bipolar disorder [7]. In mood disorders, white matter hyperintensities observed on T2 and FLAIR are the most commonly reported abnormalities, especially in bipolar disorder and late-life depression [1]. Altered functional connectivity between brain areas, as measured by inter-regional BOLD levels correlations, has been reported in schizophrenia, bipolar disorder, and anxiety disorders, both during the completion of specific tasks and at rest [8]. Some authors believe that schizophrenia and even bipolar disorder can be conceptualized as “connectivity disorders.” Schizophrenia, for example, is characterized by a global alteration in brain connectivity [9]. This could explain the widespread cognitive deficits characteristic of the disorder. Neurobiological models of mood disorders assume compromised functional regulation of prefrontal-limbic connectivity. As functional connectivity is obviously linked to structural connectivity, there is a need to precisely explore and characterize white matter in the context of psychiatric illness [10].
This is why DTI has steadily gained importance as an investigative tool in psychiatric disorders. Its unique ability to examine WM microstructure in vivo provides a means to build upon findings from previous classical MR studies. When integrated with findings from functional neuroimaging studies and molecular biology, it can be used to refine neurobiological models of psychiatric disorders. A brief review of DTI findings in selected psychiatric conditions is provided at the end of this chapter.
The Development of Imaging Biomarkers
The assessment of psychiatric disorders is currently based entirely on clinical evaluation, without any possibility of laboratory tests. Diagnosis, prediction of the transition to illness, course, and outcome of major psychiatric illnesses thus continue to be very challenging and remain difficult to predict using classical clinical instruments. The absence of an objective biomarker to assess the evolution and severity of the illnesses leads to mismanagement and increased burden [11]. There is therefore a strong need to develop biomarkers of outcome to perform more personalized healthcare plans. Recent studies have raised hopes of identifying possible biomarkers that are usable at an individual level [12]. The most promising predictive biomarkers include neuroimaging features such as white matter abnormalities. The development and use of such biomarkers of prognosis may help to identify patients that should receive specific targeted interventions [13].
One technique to achieve the development of individual neuroimaging biomarkers usable at the bedside is “Machine learning ” [14]. Techniques such as support vector machines have been developed in recent years and have already shown potential to classify patients with psychiatric disorders using neuroimaging data [15–17]. In such machine learning multivariate algorithms, the computer applies a specific mathematical method (e.g., support vector machine algorithms) to find specific patterns in a “ learning dataset” (group information supplied to the computer) that form the basis of rules for distinguishing the MRI scans of different groups (e.g., patients from those of healthy controls). The computer then applies these rules to new datasets (e.g., for the automatic classification of patients and healthy subjects within the sample). Therefore, a biomarker is constructed, with measurable metrics such as specificity, sensitivity, positive and negative predictive values, and accuracy.
Proof-of-concept of such approaches in psychiatry has already been demonstrated in schizophrenia and autism. In 2005, Davatzikos and colleagues [18] applied such an automated classification technique to T1 MRI scans from 69 patients with schizophrenia and 79 healthy controls. They achieved a classification accuracy of 81 %.
Such techniques have also proven capable of predicting clinical outcome with MRI data in neuropsychiatric disorders in recent studies. Koutsouleris et al. used multivariate machine learning algorithms to predict disease transition in schizophrenia: using T1 MRI scans from at-risk subjects, they were able to predict transition to psychosis 4 years later, with an accuracy of 82 % [16]. They performed this study with only 15 subjects having a transition to psychosis and 18 without such a transition.
In mood disorders, a recent study has highlighted the utility of such approaches to predict relapses. Farb et al. [19] recruited 16 remitted unipolar depressed patients who underwent fMRI while viewing sad and neutral film clips. They used a receiver operating characteristic analysis to determine signal cutoffs for predicting relapse. Within the depressed group, relapse was predicted by medial prefrontal cortical activity and contraindicated by visual cortical activity with sensitivity and specificity scores all above 80 %. This study clearly demonstrates the feasibility of discovering neuroimaging-based predictors of clinical outcome in mood disorders. It must be noted however that the sample size of this study was quite small.
Planning Psychosurgical Procedures
Neurosurgical treatments of severe, intractable psychiatric disorders using procedures that destroy or disconnect brain tissue have a controversial history and despite their reported efficacy are not widely used. A major criticism of such procedures is that the pathways involved in psychiatric illness are ill defined and therefore reliable surgical targets are lacking, resulting in widely variable postsurgical outcomes. Nevertheless, four major techniques are in use, which are generally accepted as safe and efficacious: anterior cingulotomy, subcaudate tractotomy, limbic leucotomy, and anterior capsulotomy [24]. All these procedures target the limbic territory and its connections.
Another promising surgical approach, particularly in the treatment of depression, is deep brain stimulation (DBS) . This technique involves the targeted stimulation of brain tissue via an electrode in order to modulate neurotransmission. In the case of depression, improvements have been reported when using DBS to target the subcallosal cingulate, ventral striatum, and anterior limb of the internal capsule (ALIC). The ALIC has also been targeted in obsessive-compulsive disorder [25].
Given the ability of DTI to virtually delineate major pathways, it can be used to investigate the connectivity profile of ablation and electrode target sites in order to understand more about the biological basis of the therapeutic and unwanted effects associated with the procedures, and about the neural circuitry involved in different aspects of psychopathology. For example, recent DTI tractography studies have found that typical psychosurgical lesion and DBS sites share similar fiber bundles within various cortical and subcortical circuits involving the prefrontal cortex and limbic networks, including, for example, the medial forebrain bundle and anterior thalamic radiation [26–28].
As the neurocircuitry of psychiatric disorders is unraveled, DTI could also be informative in guiding neurosurgical placement of the electrode in DBS (see Chap. 14) and for refining psychosurgical targets. Although presently such applications are very much in their infancy, in the future, DTI or advanced versions of the technique such as HARDI (see Chap. 21) may rejuvenate modern surgical interventions in psychiatry [29].
Special Challenges in the Application of DTI in Psychiatry
The application of DTI, and neuroimaging in general in psychiatry, is an exciting challenge. Nevertheless, specific caveats must be kept in mind, which are related to the current classification systems in psychiatry and to the psychiatric condition per se. These caveats are not all specific to DTI, but are generally common to all neuroimaging studies of patients in psychiatry.
Diagnosis and Patient Stratification Presently Based on Clinical Assessment, Not Biomarkers
To date, diagnoses in psychiatry are solely based on clinical assessment. The classification and definition of the illnesses rely on guidelines and manuals approved by the psychiatry community such as the “Diagnostic and Statistical Manual of Mental Disorders” (DSM; current version DSM-V) of the American Psychiatric Association and the “International Statistical Classification of Diseases and Related Health Problems” (ICD; current version ICD-10) of the World Health Organization.
These classification systems define a mental disorder based on a collection of clinical signs and symptoms (“a syndrome”) and their consequences. As an example, the DSM-V defines a mental disorder as a syndrome that occurs in an individual, the consequences of which are clinically significant distress or disability, that must not be merely an expectable response to common stressors and losses or a culturally sanctioned response to a particular event, that reflects an underlying psychobiological dysfunction and is not primarily a result of social deviance or conflicts with society. Other accepted validity criteria for psychiatric disorders include those established by Robins and Güze in 1970 that are a common clinical description, the exclusion of other disorders, longitudinal studies (for stability over time), familial studies, and laboratory tests.
These definitions and the classification systems thus rely largely on statistical clustering of symptoms in individuals. No single pathophysiological process is assumed for a disease definition such as in other medical fields. The “underlying psychobiological dysfunction” is vague and secondary, largely because psychiatry presently has no unitary pathophysiological model for most diseases (schizophrenia, bipolar disorder, autism etc.).
The issue with such a situation is that neuroimaging and DTI studies currently investigate groups of patients based on clinical classifications only. As an example, when we compare a group of 30 patients with “schizophrenia” with “healthy controls,” one cannot know if the 30 patients share a common underlying etiological mechanism or various physiopathological processes. This may explain some false negatives (because of the inclusion of patients with heterogeneous neurobiology). This situation may also explain the heterogeneity of the results if different groups studying the same “disease” have included non-comparable groups. Indeed, the DSM-V authors state that an inter-rater kappa for most diagnoses between 0.4 and 0.6 would be a realistic goal, and 0.2 and 0.4 would be acceptable [30]. Therefore, one cannot assume that groups of patients with an identical diagnosis are similar between studies.
In addition, the stability of psychiatric diagnoses over time is also open to debate. A very recent study explored this question in a cohort of 470 first-admission patients with psychotic disorders who were systematically assessed at baseline and during a 10-year follow-up [31]. Diagnoses were based on best-estimate consensus. In this report, 50.7 % of study participants’ diagnoses changed at some point during the study. Therefore, a study scanning patients with “first-episode schizophrenia” may include patients with first-episode schizophrenia, but also patients with other future diagnoses such as bipolar disorder.
Boundaries of diagnoses are also unclear. The distinction between schizophrenia and bipolar disorder has been debated since 1896, when Emil Kraepelin proposed that a fundamental dichotomy exists between those two diagnoses (the “Kraepelinian dichotomy”). The existence of mixed clinical forms (“schizoaffective disorders”), shared genetic vulnerability, diagnosis instability, and common risk factors have led several authors to consider those two illnesses as belonging to the same fundamental process [32, 33]. Some authors even include autism in this picture (Kanner, himself, firstly described autism as “early-onset schizophrenia”).
Finally, an additional layer of complexity comes from the variation in time of the diagnostic criteria used. As an example, diagnoses in the DSM-III and DSM-IV are not strictly identical, and thus, studies using these different manuals cannot be directly compared. Some diagnoses disappear from the classifications, while others arise.
On the other hand, neuroimaging may help to better define homogeneous and valid diagnostic groups, by identifying clear physiopathological processes involved. The initial goal of the DSM-V revision was indeed to define illnesses by using the new knowledge stemming from biological, including neuroimaging studies. To achieve this goal, large studies comparing patients across diagnoses are recommended.
Psychiatric and Medical Comorbidity, including Alcohol and Substance Abuse
Heterogeneous results have been obtained in neuroimaging studies of psychiatric conditions. Several sources of heterogeneity can be identified. Amongst them, the heterogeneity of the clinical samples recruited is a crucial issue. One source of heterogeneity comes from the classification systems used (see previous paragraph). But potential biases are specifically present in neuroimaging of patients with psychiatric illnesses: comorbidity, heterogeneity of the illness, medication, impact of illness duration, and episodes and impact of symptoms.
Comorbidity
Patients suffering from a psychiatric illness often exhibit high rates of psychiatric and somatic comorbidities. In schizophrenia, anxiety and depressive symptoms are very common with an estimated prevalence of 29 % for PTSD and 23 % for OCD. Depression occurs in 50 % of patients with schizophrenia and 47 % also have a lifetime diagnosis of substance abuse [34]. In patients with bipolar disorder, substance use comorbidities are present in up to 72 % of patients, along with anxiety or multiple comorbidities [35].
For somatic conditions, cardiovascular diseases are far more frequent than in general population in patients with bipolar disorder [36]. In schizophrenia, most of the common medical conditions are more frequent than in general vpopulation [37]. The cause for this is unclear. Two hypotheses are proposed: firstly, there is a delay in diagnosis and lack in the care of somatic conditions in patients with psychiatric illnesses. Secondly, some of these somatic conditions are inherent to the pathophysiology of psychiatric diseases [36].
The very high rates of comorbidities in psychiatric illnesses raise two challenges in DTI studies. First of all, the inclusion of patients with comorbidities may introduce a bias in the interpretation of the results. The differences found between patients and controls may be caused by the psychiatric illness itself or by its comorbidity. As an example, DTI differences between patients with bipolar disorder and controls may be linked to alcohol use disorder in these patients. Indeed, even detoxified subjects with alcohol use disorder exhibit DTI abnormalities [38], which are probably of larger magnitude than those of bipolar disorder. Even somatic conditions such as diabetes may bias the DTI results [39]. One solution to this issue may be the inclusion of comorbidity-free patients in DTI studies. However, this approach introduces a sampling bias as most of the patients have comorbidities and therefore, comorbidity-free patients may not be representative of typical patient populations.
Heterogeneity of Illness
Another source of heterogeneity in the results of neuroimaging studies is the heterogeneity of the clinical samples, which probably confounds the observed results. The clinical characteristics of the patients studied are diverse, with, for example, different forms of schizophrenia (with or without hallucinations etc.) or different subtypes of BD (e.g., types I and II, rapid cycling) and differences in age at onset (early, intermediate, late). Unipolar depression is probably even more diverse. In anxiety disorders, PTSD may arise from various types of trauma. Some of these clinical features such as the presence or absence of hallucinations in patients with schizophrenia have already been associated with specific DTI findings [40].
Illness duration, severity, number of episodes, and current symptoms may also vary between samples and are known to have an influence on DTI findings in most conditions.
A last source of heterogeneity is the recruitment mode. Patients recruited via the press, inpatient or outpatient facilities differ on many demographic and clinical characteristics.
Medication
Another major confounding variable is psychotropic medication. For major psychiatric illnesses such as schizophrenia, bipolar disorder, or severe unipolar disorder, virtually all patients are taking one, or more usually, several psychotropic medications such as antipsychotics, mood stabilizers, antidepressants, and benzodiazepines. All these psychotropic medications may affect brain structure. The most common example is the neurotrophic effect of lithium on grey matter volumes. There is presently a lack of knowledge regarding the effect of these medications on brain white matter, but current evidence suggests a limited impact on DTI variables [41].
Similarly to the comorbidity issue, the recruitment of medication-free patients, apart from being very difficult, may lead to sampling issues.
Special Considerations in the Scanner: The Effect of Motion, Active Symptoms, and Informed Consent
Another source of noise and bias that is crucial in DTI studies is the compliance of the patients to the instructions given by the scanning staff. More specifically, head motion is a major source of noise in most neuroimaging studies [42]. Patients are more prone to head motion than controls because of several factors including minor neurological signs associated with the disease itself, medication, motivation, and anxiety. In DTI, head motion is also a source of noise despite motion correction algorithms [43]. In movement disorders, FA values have proven to be robust despite head motion, which is very encouraging for the psychiatric field [44].
Aside from motion, the nature of psychiatric symptoms that patients present with during scanning may hinder optimal data acquisition. For example, depressed subjects may be less motivated to attend scanning sessions or suffer higher levels of anxiety. Manic or actively psychotic individuals may be too restless or anxious to tolerate scanning. The resulting scans may suffer more motion artifacts or scanning may be terminated before the acquisition is complete. In all these cases, the most optimum results will be obtained by employing strategies to increase patient compliance with the scanning procedures. It is therefore extremely important that staff scanning subjects with active psychiatric disorders pay special attention to ensuring that patients receive clear and complete instructions on the scanning procedure and what they can expect to experience whilst in the scanner. Along these same lines, it is vital that the nontherapeutic investigation of psychiatric patients conforms to an ethical framework that takes into account the ability of the patient to provide informed consent [45].
DTI findings in Psychiatric Disorders
Despite the inherent difficulties in acquiring good comparative data in psychiatric populations, research in psychiatry has greatly benefited from neuroimaging. Earlier work using CT, MRI, and PET fundamentally altered the perception of psychiatric illness from an intangible, unquantifiable, functional disturbance without organic pathology, to a collection of disorders for which measurable neurological changes in brain structure and biochemical function could be identified and visualized. With the ability to investigate white matter, DTI continues to advance our understanding of the nature of these structural changes. Since the advent of the technique, the role of white matter alterations as a core feature of mental illness pathophysiology has become apparent, and the concept of psychiatric disease arising from altered structural connectivity has been strengthened.
Which Fiber Bundles Are of Interest in Psychiatric Disorders?
DTI has been applied to the investigation of a number of psychiatric disorders to varying degrees and using a range of analysis methods to explore whole brain white matter, specific fiber tracts, and tract subregions. The most commonly reported deficits are found in frontal and temporal white matter and tracts that subserve the limbic system. Such tracts include the various subregions of the corpus callosum (CC), cingulum bundle (CB), superior (SLF) and inferior longitudinal fasciculi (ILF), thalamic radiations, and uncinate fasciculus (UF) [1, 46]. Impaired WM microstructure in these regions is hypothesized to contribute to a breakdown in the regulation of higher functions relating to cognition, emotion, and memory, which are typically compromised in psychiatric illness. Some ascending and descending fiber systems such as the corona radiata, internal vcapsules, cerebral and cerebellar peduncles feature more predominantly in neurodevelopmental disorders such as autism and ADHD, and also in schizophrenia, and may underlie the psychomotor features of these illnesses.
Reported alterations are however by no means limited to these areas and neither are such findings universal. This likely reflects the heterogeneity of both the clinical populations studied and the methodology employed to investigate them. Furthermore, several regions such as the WM of the medial temporal lobe and corpus callosum emerge consistently in meta-analyses of different disorders. This illustrates the lack of specificity of DTI changes in psychiatric illnesses and may be reflective of the considerable overlap in symptomatology between them. In this context, DTI metrics in isolation cannot be used diagnostically but provide useful additional data in a multimodal framework incorporating for example, genetic, neuropsychological, psychosocial, and clinical measures.
DTI Findings in Selected Psychiatric Disorders
Schizophrenia
Schizophrenia is a disorder of thought, perception, emotion, and behavior affecting an estimated 1 % of the population. Patients may experience both “positive” symptoms, such as hallucinations, delusions, altered thoughts and feelings of being controlled, and “negative” symptoms characterized by withdrawal, flattened affect, and anhedonia.
It is the most widely studied psychiatric disorder using DTI, with over 300 studies listed on PubMed at the time of writing (early 2015). There are few negative studies, with the majority reporting FA reductions in more than one brain region [47]. Although FA reduction in frontal and temporal WM appears most frequently reported, there are also reports of such decreases in parietal, occipital, and even cerebellar white matter, suggesting widespread diffuse whole brain pathology, consistent with findings of widespread grey matter reductions and functional impairments detected using other imaging modalities [10]. A recent meta-analysis of DTI studies in schizophrenia described two distinct regions where FA was reduced consistently: one in the left perigenual WM of the frontal lobe and a second region, in the medial temporal lobe [48]. The authors postulate that these regions represent two distinct networks that are compromised in schizophrenia, leading to a disconnection of important fronto-temporal grey matter functional areas.
Mood Disorders
The next most widely investigated psychiatric conditions are major depressive disorder ( MDD) and bipolar disorder (BD) . Depression is a common disorder affecting up to one in five people in their lifetime. It is characterized by extended periods of low mood, sadness, anhedonia, impaired concentration, altered sleep and appetite, feelings of guilt and worthlessness, and in severe cases, suicidal thoughts. Bipolar disorder is less common, affecting 1 % of the population, and is characterized by alternating periods of severe depression and hypomania or mania. During (hypo) manic episodes, patients experience elevated mood, increased energy, reduced need for sleep, talk more quickly, may make unrealistic plans, overspend, engage in risky behavior, become irritable, aggressive, and abuse alcohol and drugs. Some patients may also experience psychosis, a state in which their perception of reality becomes distorted. In this context, symptoms of BD and schizophrenia overlap. It is interesting that DTI findings in BD also parallel those in schizophrenia. However, FA reductions are less widely reported across the whole brain in BD and there are considerably more negatives studies. There are also some reports of regional FA increase [49]. Regionally, FA reductions tend to be found in frontal and temporal WM. Corpus callosum deficits feature strongly, particularly anterior (genu) and posterior (splenium) projections [50–52]. FA reductions also predominate in anatomically closely related tracts, such as portions of the SLF, ILF, IFOF, posterior thalamic radiation, and cingulum [53]. Such regions are classically associated with emotional regulation, working memory, and facial processing; functions that are impaired in BD. Interestingly, these regions, which emerged consistently in a meta-analysis of 11 DTI studies [46], parallel the two regions identified in the schizophrenia meta-analysis described above.
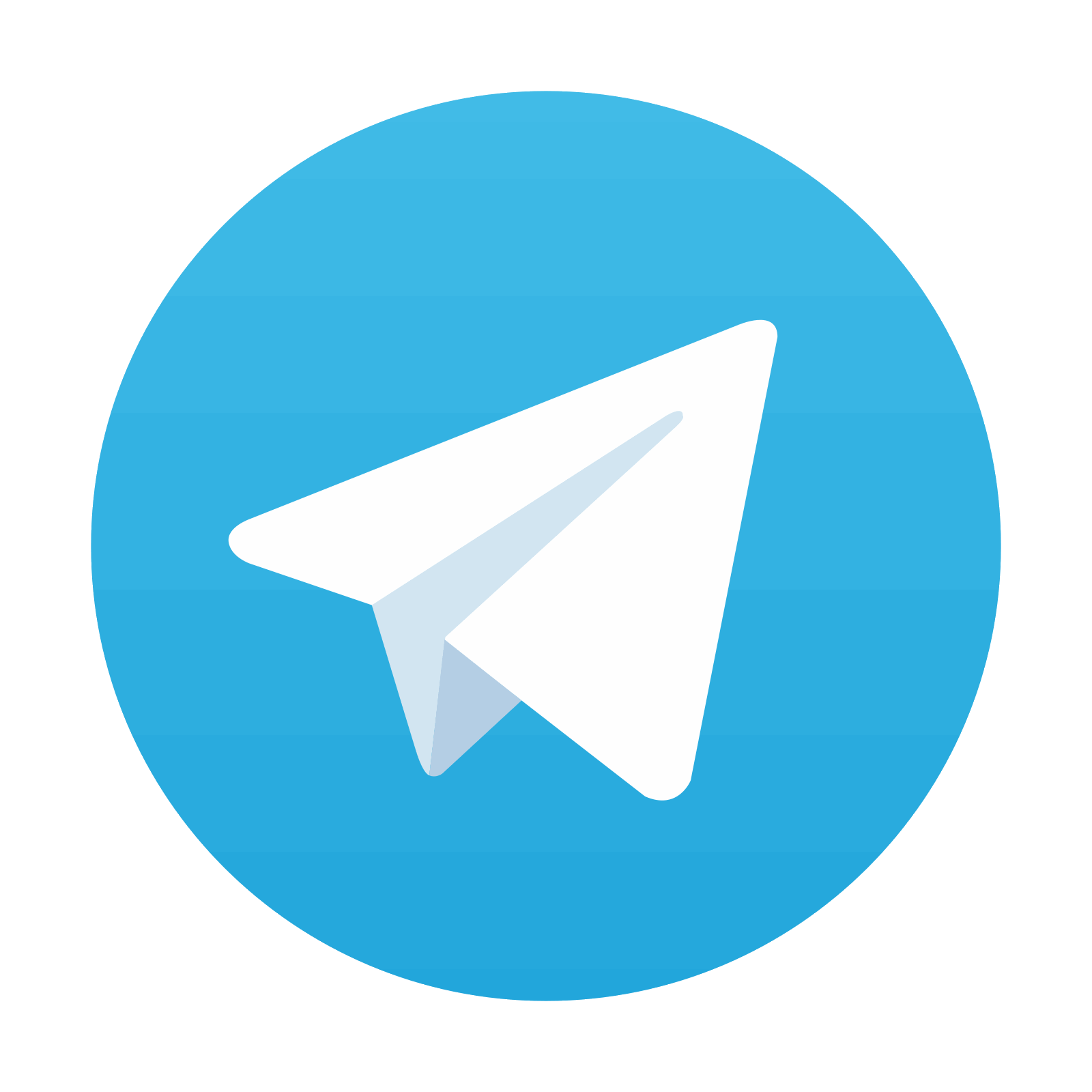
Stay updated, free articles. Join our Telegram channel

Full access? Get Clinical Tree
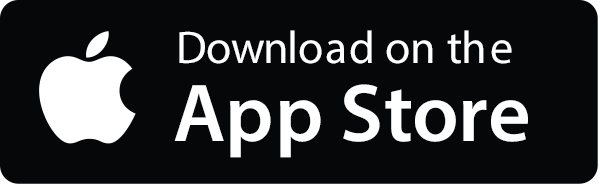
