2
Patient Selection and Life Expectancy Tools
Srinivas Raman, Nicholas Chiu, Na Zhang, and Edward Chow
INTRODUCTION
Life expectancy estimates for advanced cancer patients are necessary considerations in the fields of oncology and palliative care. While difficult to predict and uncomfortable to communicate, life expectancy estimates are important for a variety of clinical and personal reasons. In particular, accurate life expectancy estimates serve to: (a) guide appropriate clinical decisions, (b) direct planning of supportive services, (c) help patients and families plan end-of-life issues, (d) steer the allocation of resources, and (e) determine eligibility for hospice referrals or enrollment into clinical trials.
In terms of clinical decisions, the decision to treat, the modality of treatment, and the radiation dose schedule may all depend on survival prediction.1 The decision between the use of supportive care alone versus radiation therapy (RT) in cases of complicated or uncomplicated bone metastases patients depends on life expectancy estimates.2 Furthermore, a patient’s treatment preferences, particularly in deliberating between life-extending therapies and palliative treatment, are influenced by the prognosis communicated to him or her.3
Survival estimates play an essential role in the planning of end-of-life issues and patients’ and families’ preparations for death. Steinhauser et al. conducted a cross-sectional, stratified random national survey to determine the factors considered important at the end of life by patients, families, physicians, and other providers.4 An agreement was found across all groups on the need for symptom management, preparation for death, contemplation about one’s life, being treated as a whole person, developing a relationship with medical care providers, and the usefulness of advance directives.4 Moreover, in a study of patients’ perspectives regarding quality end-of-life care, key domains of quality end-of-life care include the achievement of a sense of control and strengthened relationships with loved ones.5 Addressing such factors in a limited time span requires accurate life expectancy estimates.
The allocation of resources and provision of cost-effective treatments require accurate survival prognosis.6,7 Cost has become a key concern in reimbursement of expensive, high-technology procedures.7 With shrinking resources and an aging population, the delivery of cancer treatment may require major changes as cancer patients of different ages and prognostic demographics compete for the same limited resources.7 With regards to radiation oncology practices in particular, the use of single-fraction RT is more cost effective.8
Enrollment in hospice or a phase I clinical trial is often contingent on a patient’s prognosis. To be considered for entry into phase I clinical trials, patients must typically have at least a 2- to 3-month estimated survival time, while that estimate is no more than 6 months for entry into a hospice program.9 Temel et al. found that earlier referral to a hospice program leads to better management of patients’ symptoms, stabilization of their conditions, and prolonged survival.10 Given these factors, the importance of accurate survival estimates is evident, as they may aid in treatment decisions that could potentially boost quality of life and prolong survival.
This chapter on life expectancy and patient selection is written in recognition of the importance of accurate life expectancy estimates in the care of patients with advanced cancer. We describe some of the prognostic models currently available to help determine life expectancy and the limitations of those models. Furthermore, we discuss the changing landscape for prognostication in metastatic cancer, and elaborate on how survival estimates can affect clinical recommendations.
DESCRIPTIONS OF LIFE EXPECTANCY MODELS
Studies have found that a number of tumor factors, patient factors, and laboratory tests can be used to predict life expectancy in patients with advanced cancer.11 Tumor factors are varied and broadly include primary tumor site and subtype, site(s) of metastases, and number of metastases. Metastatic breast cancer and prostate cancer are generally associated with a favorable prognosis compared to other cancers, but also this depends on the tumor subtype. For example, metastatic breast cancers with a triple negative biomarker profile have a more aggressive course and worse prognosis in comparison to metastatic breast cancers with a hormone receptor positive biomarker profile. This difference may be partially related to favorable biology, but the availability of therapeutic strategies that target the biology also contributes; for example, endocrine therapy for hormone positive breast cancer. The site(s) of metastatic disease is also an important factor. Patients with bone-only metastases generally have a more favorable prognosis than patients with involvement of visceral organs such as the liver and brain. Patients with a limited number of metastases can have prolonged survival and may benefit from more aggressive treatment.12
Patient factors usually include age, symptom burden, and performance status. Clinically significant symptoms predictive for survival include dyspnea, anorexia, nausea, xerostomia, confusion, mood, fatigue, and weight loss. Performance status is a measure of a patient’s overall ability and functionality. The most commonly used metrics for performance status are Eastern Cooperative Oncology Group (ECOG) score, Karnofsky performance score (KPS), and palliative performance status (PPS). The KPS ranges from 0 to 100, where 100 is a perfect score with no functional impairment experienced by the patient and 0 is the worst score, signifying death. The PPS is similar to the KPS but also includes information about self-care, intake level, and consciousness level. The ECOG scale (also referred to as Zubrod or WHO scale) is similar to KPS/PPS but ranges from 0 to 5, where 0 represents an asymptomatic patient and 5 represents death. Performance status is predictive for survival. Multiple studies have found that a KPS less than 50 is consistently associated with a prognosis of less than 8 weeks.13–17 Performance status is not solely predictive, particularly at higher KPS values or when KPS changes over time.18 Laboratory tests, including lactate dehydrogenase (LDH), C-reactive protein (CRP), hemoglobin, white blood cell count/differential, and albumin level, have prognostic value when applied independently or in combination with other prognostic factors.11
To address the heterogeneity in patients with advanced cancers, numerous groups have proposed different multivariable life expectancy models using combinations of known tumor factors, patient factors, and laboratory tests. The most common indications for palliative RT include painful bone metastases, spinal cord compression, and brain metastases with the goals of symptom relief and/or local control. Local control is particularly important in brain metastases and spinal cord compression, as prompt treatment can mitigate, avoid, or delay neurologic compromise. Models developed for prognostication must reflect both the patient population and the goals of treatment. Accordingly, the focus of this section will be to introduce prognostic models developed specifically for patients who are seen in the setting of palliative RT. Appendix B contains prognostic models developed in other populations of patients that are particularly useful for predicting very short-term survival. Readers are also referred to an excellent review by Krishnan et al. for further information.11
Models Developed in the General Palliative RT Setting (Table 2.1)
A commonly used model for predicting life expectancy was developed by Chow et al. in patients with metastatic cancer attending an outpatient palliative RT clinic in Toronto, Canada.19 The model was developed in a training dataset of 395 patients using a Cox’s proportional hazards regression model. From 16 prognostic factors, six prognostic factors were found to have a statistically significant impact on survival: primary cancer site, site of metastases, KPS, and fatigue, appetite, and shortness of breath scores from the modified Edmonton symptom assessment scale (ESAS). Both a survival prediction score (SPS) method and number of risk factors (NRF) method were used to predict survival. For the SPS method, each prognostic variable was assigned a weight based on the level of significance and a score from 0 to 32 was generated. The survival rates at different time points were determined for different SPS categories: ≤13, 14 to 19, and ≥20. The NRF method simply sums the total NRF present. Chow et al. validated the model on a temporal dataset in the same institution and an external dataset from a different institution.20 Both the SPS and NRF discriminate survival in the three prognostic groups.
Chow et al. later simplified the model to include only three variables: (a) primary cancer site, (b) site of metastases, and (c) KPS.20 Both the three and six variable models were found to predict survival similarly with no statistically significant difference in performance. The three-variable NRF model (non-breast primary cancer, metastases other than bone only, and KPS ≤60) is the simplest to use in clinic and performed similarly to the more complex models. It has been externally validated in an outpatient pain and palliative care clinic at Memorial Sloan Kettering Cancer Center21 and in a palliative RT clinic in Norway.22
The TEACHH life expectancy model developed by Krishnan et al. identifies patients at different ends of the prognostic spectrum23; that is, patients having a life expectancy less than 3 months and greater than 1 year. The model was developed from a database of 862 patients with metastatic cancer who received palliative RT in Boston, United States. The median survival was 5.6 months. A Cox proportional hazards model and multivariate analysis identified factors predictive for a shorter life expectancy: T stands for primary tumor (lung and other vs. breast and prostate), E for ECOG performance status (2–4 vs. 0–1), A for older age (>60 vs. ≤60 years), C for prior palliative chemotherapy courses (≥2 vs. 0), H for hospitalizations within 3 months before palliative RT (0 vs. ≥1), and H for hepatic metastasis. Patients were divided into three groups with different median survivals: (a) group A (0–1 risk factors, overall survival [OS] = 19.9 months), (b) group B (2–4 risk factors, OS = 5 months), and (c) group C (5–6 risk factors, OS = 1.7 months).
Two models have been developed to predict those patients with a very poor prognosis, those with a life expectancy of less than 30 days.24 Recursive partitioning analysis (RPA) was used to analyze 579 palliative RT courses at a single Norwegian institution. The median survival in the dataset was 6.3 months and the 30-day mortality was 9%. Factors predictive for 30-day mortality included primary lung or bladder cancer, ECOG PS 3–4, opioid use, steroid use, low hemoglobin, and progressive disease outside the RT volume. A larger population-based study of 14,972 cases treated at a single institution in the United Kingdom identified patients at risk of 30-day mortality after palliative RT.25 Overall, the median survival was 5.6 months and the 30-day mortality was 12.3%. Factors predictive for 30-day mortality included sex, primary tumor, treatment site, and fractionation schedule.
TABLE 2.1 Life Expectancy Models, Developed in General Palliative RT Clinics
Models Developed in Specific RT Settings (Table 2.2)
Models developed in patients with bone metastasis
Specific models for patients receiving RT to particular metastatic sites have also been developed for bone metastasis, spinal cord compression, and brain metastasis. van der Linden et al. developed a survival model from 342 patients in the Dutch Bone Metastasis study who received RT for painful Harrington Class I and II spinal metastases.26 The median OS for all patients in the dataset was 7 months. A Cox proportional hazards model and multivariate analysis identified the major predictors for survival: KPS (10–40, 50–70, 80–100), primary tumor site (breast, prostate, lung), and presence of visceral metastases. A score was assigned to each category and the final classification system included three groups. Group A had a median OS of 3 months, Group B had a median OS of 9 months, and Group C had a median OS of 18.7 months. The patients in Group C had the most favorable prognostic features: primary breast cancer, good performance status, and absence of visceral metastasis. Westhoff et al. developed a prognostic model from the larger cohort of 1,157 patients enrolled in the Dutch Bone Metastasis study.27 The median survival for the patients in the dataset was 30 weeks. Univariate and multivariate Cox proportional hazard models with backward selection were used to select and combine the variables most predictive for survival. The best predictive model had a C-statistic of 0.72 and included sex, primary cancer site (breast, prostate, lung, other), presence of visceral metastases, KPS (90–100, 70–80, 20–60), general health rating on a visual analog scale (VAS-gh 67–100, 34–66, 0–33) and valuation of life on a verbal rating scale (VRS-vl 1–3, 4, 5–7). A simplified model with a C-statistic of 0.71 identified KPS and primary cancer site as prognostic variables. Median survival and survival rates at 3, 6, 9, 12, and 18 months were reported for each combination. External validation of the model was performed in a separate dataset of 934 patients at a single Dutch Institution with a C-statistic of 0.72 and a similar calibration plot.
Chow et al. developed a survival prediction model from patients with a KPS ≥50 undergoing reirradiation for bone metastases in the SC-20 trial.28 The training and model validation datasets included 460 and 351 patients, respectively. A Cox regression model using backward selection was used to select statistically significant variables; only KPS and primary tumor site were found to be predictive for survival. Partial scores were assigned to KPS (90–100, 70–80, 50–60) and primary tumor site (breast, prostate, lung). An SPS ranging from 0 to 5 was generated where the 1/3 and 2/3 percentile scores were 2 and 3.6, respectively. In the testing dataset, the median survival for the group with the best prognosis was not reached. In the other two groups, the median survivals were 11.3 and 5.2 months. The 3-, 6-, and 12-month survival rates for the worst group were 64.4%, 43.0%, and 19.7%, respectively.
TABLE 2.2 Life Expectancy Models, Developed Using Patients With Specific RT Indications
Malignant spinal cord compression (MSCC) is caused by displacement or compression of the spinal cord by tumor and is described in detail in Chapter 4. MSCC can be associated with significant morbidity despite aggressive and emergent intervention; the prognosis for MSCC is thought to differ from uncomplicated spinal and bone metastases. A scoring system was developed using six factors found to be significant on a multivariate analysis of 1,852 patients undergoing RT for MSCC at multiple international institutions: tumor type, interval between cancer diagnosis and development of MSCC, presence of other sites of disease, ambulatory status, and duration of motor deficits.29 Each factor was assigned a prognostic score. The summed scores were then divided into five categories with 6-month survival rates of 4%, 11%, 48%, 87%, and 99%. In the two categories with the highest 6-month survival, long-course RT, defined as 10 to 20 fractions delivered over 2 to 4 weeks, was associated with a statistically significant improvement in survival. This prognostic model was validated by a separate dataset of 439 patients with similar 6-month survival rates.30 The model was simplified with different prognostic score cut-offs and associated 6-month survivals of 14%, 56%, and 80%.30
Models Developed in Patients With Brain Metastasis
With an incidence of 10% to 20% in patients with cancer,31 brain metastases are a common indication for palliative RT. Prognostication represents a unique challenge in the treatment of patients with brain metastasis since only 30% to 50% of patients will die from their brain metastasis. Predictive models need to account for successful control of both intracranial and extracranial disease since both are closely tied to prognosis. Gaspar et al. developed the RPA using data from 1,200 patients from three Radiation Therapy Oncology Group (RTOG) trials: 79-16, 85-28, and 89-05. All patients received whole brain RT (WBRT), with a subset of patients in RTOG 85-28 receiving a boost dose.32 Based on the analysis of 18 pretreatment and three treatment-related variables, three RPA categories were developed (Table 2.3).
The RPA model was independently validated in a dataset of 432 patients from RTOG 91-04.33 This tool was later validated in a separate population not included in the RTOG trials, patients with small-cell lung cancer.34 A potential criticism of this tool is that it was developed in patients who presented with symptomatic brain metastases in an era that predated routine magnetic resonance imaging (MRI); today, many patients present with asymptomatic brain metastases incidentally found on MRI.
TABLE 2.3 RPA Classification and Median Survival by Class
To incorporate the number of brain metastases, which is also a prognostic factor, Sperduto et al. developed the graded prognostic assessment (GPA) in a database of 1,960 patients from four RTOG trials.35 A score of 0, 0.5, or 1 was assigned to the following categories: age, KPS, number of brain metastases, and presence of extracranial metastases. The median survival times for the four GPA categories were 2.6, 3.8, 6.9, and 11.0 months. Due to heterogeneity in the primary tumor sites and implications on prognosis, Sperduto et al. also subsequently developed a diagnosis-specific GPA (DS-GPA). From a database of 3,940 patients, a multivariate analysis identified the most prognostic variables for each primary tumor site and a GPA score was assigned to each variable.36 Median survival resulting from disease-specific GPA was reported for patients with lung cancer, melanoma, breast cancer, renal cell carcinoma, and gastrointestinal cancers (Table 2.4).
The RPA, GPA, and DS-GPA are the most commonly used tools for predicting life expectancy in patients with brain metastases and the majority of current clinical trials in brain metastases still use these indices as stratification factors. However, numerous other life expectancy tools have also been developed for use in brain metastases, including the Rotterdam system,37 the score index of radiosurgery,38 the basic score for brain metastases,39 the Golden grading system,40 and the two classification systems developed by Rades et al.41,42 Readers are directed to an excellent systematic review by Rodrigues et al. for further description and performance of the various prognostic indices in patients with brain metastasis.43
INACCURACY OF LIFE EXPECTANCY ESTIMATES AND LIMITATIONS OF LIFE EXPECTANCY MODELS
Inaccurate life expectancy estimates by physicians have been well documented in the literature.3,6,44–46 Survival predictions by physicians are often overly optimistic,1,44,46 although Janisch et al. found that they can be overly pessimistic as well.45 Six radiation oncologists provided survival estimates for 739 patients in a study by Chow et al.1 Physicians overestimated survival by more than 3 months. Similarly, Chistakis et al. found that 63% of physician estimates were overly optimistic, while 17% were overly pessimistic.46 The results of such studies underscore the inaccuracy of clinical life expectancy estimates on the part of physicians.
TABLE 2.4 Prognostic Factors From the DS-RPA
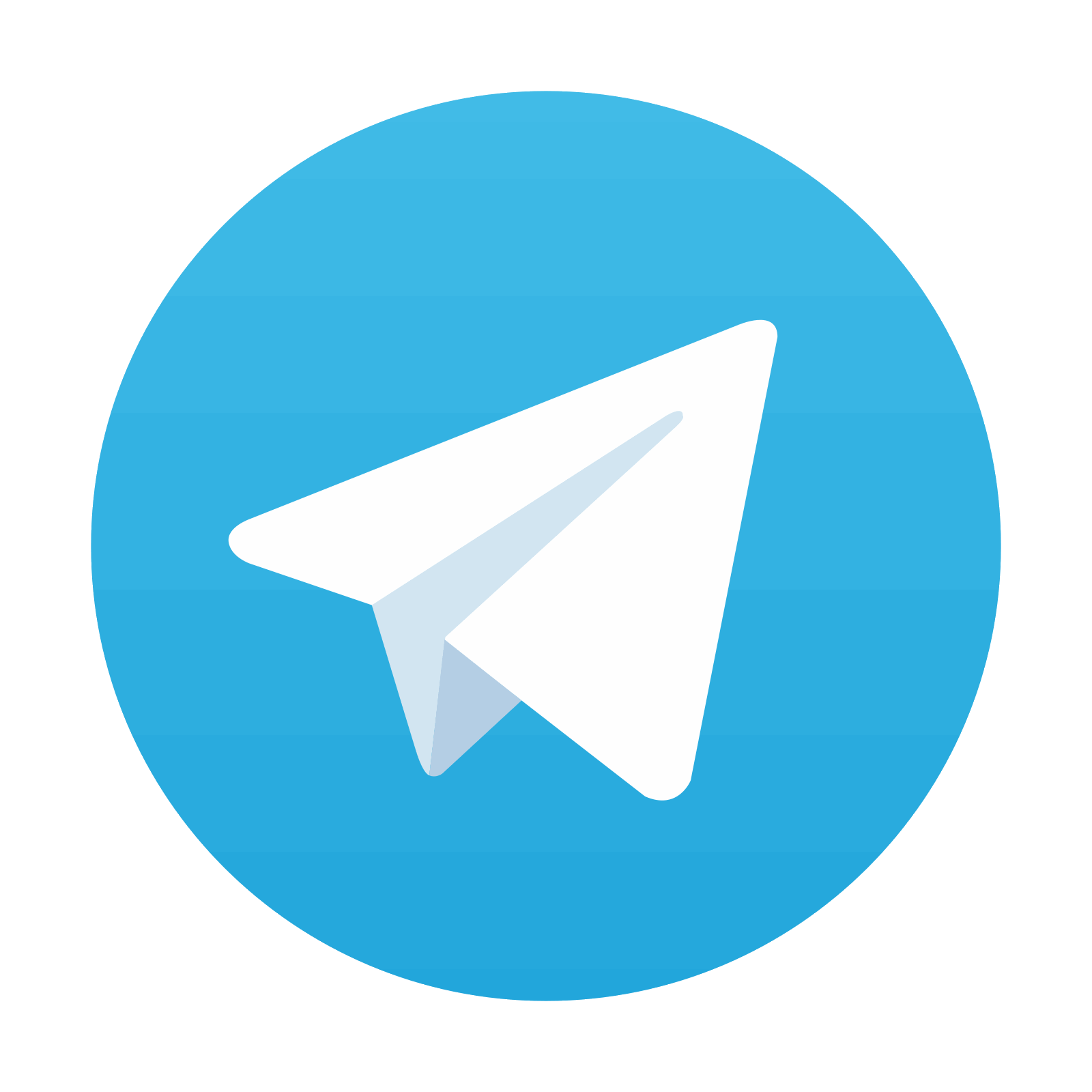
Stay updated, free articles. Join our Telegram channel

Full access? Get Clinical Tree
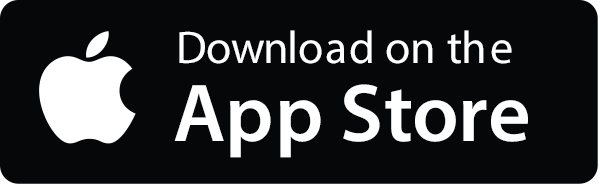
