Liver perfusion magnetic resonance (MR) imaging is currently being actively investigated as a functional imaging technique that provides physiologic information on the microcirculation and microenvironment of liver tumors and the underlying liver. It has gained importance in light of antiangiogenic therapy for hepatocellular carcinoma and colorectal liver metastases. This article explains the various model-free and model-based approaches for liver perfusion MR imaging and their relative clinical utility. Relevant published works are summarized for each approach so that the reader can understand their relative strengths and weaknesses, to make an informed choice when performing liver perfusion MR imaging studies.
Key points
- •
Understanding the basis of the various model-free and model-based methods for dynamic contrast-enhanced (DCE) liver perfusion magnetic resonance (MR) imaging would allow one to adopt an appropriate technique.
- •
Perfusion MR imaging of the liver with DCE-MRI is an evolving technique that has the potential to interrogate intravoxel microcirculatory parameters and may serve as an imaging biomarker for response to antiangiogenic therapy as well as liver fibrosis and cirrhosis.
- •
Additional work is required to confirm preliminary findings and optimize scanning and analytical techniques before DCE-MRI can be routinely used in the clinical setting.
Introduction
Perfusion imaging of the liver with dynamic contrast-enhanced (DCE) magnetic resonance (MR) imaging demonstrates functional microenvironmental changes in small vessels that are beyond the resolution of clinical MR imaging for direct visualization.
Perfusion information is derived by observing and quantifying the T1 signal change as contrast material transits through tissue. Information obtained from liver DCE-MRI includes blood flow, capillary permeability, proportion of intravascular space, proportion of interstitial space, and proportions of arterial supply contributed by the hepatic artery and portal vein. Such functional information allows for the assessment of tumor response to antiangiogenic therapy with the potential of selecting subgroups of patients who may benefit preferentially from such therapy. There is also emerging evidence for microcirculatory information to be used to assess early response from local ablative therapy, such as transarterial chemoembolization and radionuclide therapy. Functional information also allows assessment of increased arterial fraction and the volume of the interstitial space of Disse in diffuse liver disease, such as liver fibrosis and cirrhosis.
DCE-MRI of the liver is still largely a research and explorative technique. Although promising results have been reported by some groups, these findings are not consistently confirmed. As such, much future work is required before a clinical role can be defined. DCE-MRI of the liver is also a heterogeneous and evolving technique. This article aims to explain the principles of liver DCE-MRI and to provide a framework for the reader to understand the various models used and make sense of the various microcirculatory parameters published by different authors. A summary of the main works in perfusion liver imaging is also provided. This article hopes to help the reader embark on liver DCE-MRI and to help the reader make an informed decision regarding the choice of technique and model.
Introduction
Perfusion imaging of the liver with dynamic contrast-enhanced (DCE) magnetic resonance (MR) imaging demonstrates functional microenvironmental changes in small vessels that are beyond the resolution of clinical MR imaging for direct visualization.
Perfusion information is derived by observing and quantifying the T1 signal change as contrast material transits through tissue. Information obtained from liver DCE-MRI includes blood flow, capillary permeability, proportion of intravascular space, proportion of interstitial space, and proportions of arterial supply contributed by the hepatic artery and portal vein. Such functional information allows for the assessment of tumor response to antiangiogenic therapy with the potential of selecting subgroups of patients who may benefit preferentially from such therapy. There is also emerging evidence for microcirculatory information to be used to assess early response from local ablative therapy, such as transarterial chemoembolization and radionuclide therapy. Functional information also allows assessment of increased arterial fraction and the volume of the interstitial space of Disse in diffuse liver disease, such as liver fibrosis and cirrhosis.
DCE-MRI of the liver is still largely a research and explorative technique. Although promising results have been reported by some groups, these findings are not consistently confirmed. As such, much future work is required before a clinical role can be defined. DCE-MRI of the liver is also a heterogeneous and evolving technique. This article aims to explain the principles of liver DCE-MRI and to provide a framework for the reader to understand the various models used and make sense of the various microcirculatory parameters published by different authors. A summary of the main works in perfusion liver imaging is also provided. This article hopes to help the reader embark on liver DCE-MRI and to help the reader make an informed decision regarding the choice of technique and model.
Qualitative explanation of dynamic clinical MR imaging
Radiologists have long recognized the importance of observing the MR signal intensity of a focal liver lesion during T1-weighted contrast-enhanced dynamic scans. It is well recognized that a hypervascular lesion such as hepatocellular carcinoma (HCC) shows arterial hyperenhancement (wash-in) and becomes hypointense relative to the liver (wash-out) during the portal venous and equilibrium phases. By contrast, hypovascular and desmoplastic tumors, such as colorectal liver metastases or cholangiocarcinoma, show progressive enhancement with mild enhancement in the arterial phase, increased enhancement in the portal venous phase, and maximum enhancement in the delayed phase. These findings can be explained by understanding tracer kinetic modeling. For example, DCE-MRI tracer kinetic modeling has shown that in neuroendocrine liver metastases, a wash-in and wash-out pattern occurs when the proportion of intravascular space is greater than that of the interstitial space, whereas the proportion of interstitial space is greater than the intravascular space in lesions showing progressive enhancement.
To facilitate qualitative understanding of the basis of tracer kinetic modeling without abstract mathematics, simplification is in order, and, for the moment, the portal venous blood supply to tumors should be ignored.
A single voxel of abnormal tissue consists of intravascular and interstitial spaces supplied by an arteriole ( Fig. 1 ). Following an intravenous bolus injection, contrast in the vascular space is maximal in signal intensity in the arterial phase because it is minimally mixed with unopacified blood in the rest of the body. Contrast in the vascular space during the portal venous phase is reduced in signal intensity because it has more time to circulate and mix with unopacified blood from the rest of the body. In a similar way, contrast in the vascular space during the equilibrium phase would have the lowest signal intensity.
The signal of the vascular space in the tumor shows maximum signal intensity in the arterial phase and decreases gradually in the portal venous and equilibrium phases. As the tumor arterioles are beyond the resolution of MR imaging to directly visualize, the acquired voxel images the average concentration of the entire system. As the intravascular space is large in hypervascular lesions such as hepatoma, the imaged signal comes predominantly from the vascular space and hence the lesion would show a “wash-in” and “wash-out” pattern (upper series of boxes in Fig. 1 A).
The signal in the interstitial space depends on the transport of tracer due to convection (difference in hydrostatic pressure between the capillary and the interstitium) as well as tracer diffusion (difference in tracer concentration between the capillary blood and the interstitial fluid). Convection occurs in all 3 phases. As the concentration of tracer in the interstitium is relatively low compared with the intravascular space, it is likely that the tracer concentration is lower relative to the capillary even in the equilibrium phase. Hence, diffusion also occurs in all 3 phases. Therefore, there is gradual increase in tracer concentration from the arterial phase to the equilibrium phase (progressive enhancement). In tumors with large interstitial spaces due to desmoplasia and a relatively small intravascular space, such as colorectal liver metastases, the signal in the acquired voxel is more dependent on the interstitial space than the small intravascular space and hence the tumor demonstrates progressive enhancement (upper series of boxes in Fig. 1 B).
As one can see from this simplified qualitative explanation, tracer kinetic modeling can provide information about the microcirculation.
Qualitative explanation of DCE-MRI tracer kinetic modeling
Going one step further, one can quantify microcirculatory parameters such as blood flow, capillary permeability-surface area product, percentage of intravascular space (the first space encountered by the tracer), and percentage of interstitial space (the second space the tracer encounters due to convection and diffusion), with tracer kinetic modeling.
First consider a simplified voxel representing tumor cells perfused by capillaries ( Fig. 2 ). Contrast does not enter cells. Imagine that a fixed amount of contrast can be injected instantaneously into the capillary (mathematically known as an impulse) and the contrast flow can be watched out of the capillary into the interstitium. The system continues to be observed as the tracer that had been transported into the interstitium reenters the capillary and is cleared by the flowing blood. Effectively, it is observed how much tracer remains in the system (mathematically known as impulse retention function, IRF). Notice that as tracer flows into the capillary and is transported into the interstitium, the overall observed signal is still 100% because the voxel images the average concentration in the entire system. In this empirical example, a decrease in signal is only noticed when 70% of the tracer flows out into the venules. This sudden drop in signal reflects the first-pass extraction of the system and depends on how permeable the capillary is. The subsequent decay of the signal depends on both the permeability and the blood flow.
An example of a typical IRF is illustrated in Fig. 3 A. Fig. 3 B illustrates a capillary that has the same permeability as Fig. 3 A but higher blood flow. Notice the more rapid signal drop due to higher blood flow. Fig. 3 C illustrates a capillary that has higher permeability than Fig. 3 A. Note the higher takeoff of the curve as more tracer exits to the interstitium due to higher permeability. Fig. 3 D illustrates a capillary that is not permeable. Note that no tracer is left in the interstitium after the impulse has flowed across the vascular space. Hence, qualitatively, the IRF can be very informative concerning the microcirculation.
It is impossible to inject tracer into tumor capillaries as an impulse. However, it can be considered that the arterial concentration-time curve (arterial input function, AIF) serves as a series of impulses separated by small intervals of time ( Fig. 4 , upper panel ). The tumor concentration-time curve is mathematically a convolution of the AIF with the tumor IRF (see Fig. 4 , lower panel ). The mathematics of deconvolution allows the derivation of the IRF of the tumor from the observed AIF (typically from a large vessel such as the aorta) and the observed tumor concentration-time curve. Tracer kinetic models can then be created with different microcirculatory parameters so as to describe a model IRF. The computer takes over and attempts to perform curve fitting of different combinations of each microcirculatory parameter to make the model IRF resemble the actual IRF and hence elucidate the actual microcirculatory parameters.
Many tracer kinetic models have been described. They vary in complexity and underlying assumptions. For an introduction to the subject, the reader is referred to Paldino and Barboriak. For a detailed mathematical treatment, the reader is referred to Koh and colleagues.
Single-compartment versus dual-compartment
The liver has a unique microcirculatory environment that requires additional considerations. The liver cells form plates, which are impermeable to contrast. The vascular space is predominantly composed of the hepatic sinusoid, which has large fenestrae and no basement membrane. The interstitial space in the normal liver is the space of Disse, which lies between the sinusoid and the liver plate. Because the fenestrae are large, there is free passage of tracer between the sinusoid and the space of Disse ( Fig. 5 A). As such, in the normal liver, the interstitial space is effectively zero because tracer concentration in the interstitium is the same as that in the vascular space. Some models use a single compartment to describe the liver on this basis, instead of a dual compartment (see Fig. 5 C).
With cirrhosis, the sinusoids become more capillary-like as basement membrane is laid down, fenestrae close, and capillary junctions form. There is also increased deposition of collagen in the space of Disse by the stellate cells. As such, the interstitial space becomes measurable and nonzero, such that the liver parenchyma is effectively a dual compartment (see Fig. 5 B). It is useful to note that for a single-compartment model, parameters derived are related to the vascular compartment (blood flow, mean transit time, and percentage of intravascular space). It is not possible to derive parameters related to extravasation of contrast into the interstitial space (permeability-surface area product, percentage of interstitial space) because the interstitial space is not modeled.
Single versus dual input
The liver is supplied by the hepatic artery and the portal vein. Liver tumors are predominantly supplied by the hepatic artery but they may also be supplied by the portal vein.
From a modeling perspective, the AIF is a concentration-time curve of the tracer input into the model. Traditionally, this input comes from the artery and hence its name. However, given the dual perfusion pathways of the liver, the “arterial” input function may come from both the artery and the portal vein. It is important to determine whether a model is described by a single-input AIF (from the aorta [ Fig. 5 D]) or a dual-input AIF (from both the aorta and the portal vein). A dual-input model can determine the relative contribution of the arterial and portal flow to the total blood flow. Some investigators may choose to use a population AIF instead of the patient’s AIF. This choice is especially so when the AIF cannot be determined with accuracy or if the scan planes preclude the direct measurement of the AIF.
Conventional compartment model versus distributed parameter model
Many models assume that the tracers in the vascular and interstitial compartments are instantaneously mixed. Hence, the mathematics only need to consider one concentration value for every time point irrespective of the position along the vessel; this is the approach of the conventional compartment model.
However, the mean transit time of normal liver parenchyma is on the order of 12 seconds. If one acquires dynamic images with temporal resolution of 4 seconds and less, it is possible to take into account the concentration gradient that exists across the vascular space from a high concentration at the input end to a low concentration at the hepatic venous end ( Fig. 6 ). Mathematically, this approach is more complex and is the approach of the distributed parameter model. The distributed parameter model requires high temporal resolution to image the system as the tracer flows through the vascular space. The conventional compartment model does not require such a high temporal resolution.
Table 1 summarizes the various models used. For all of the models described, there is a choice to calculate the actual tracer concentration by a multiple flip angle method or to assume that the concentration encountered is linear to signal intensity. The observed signal intensity in MR studies does not vary linearly with gadolinium concentration. In general, estimation of gadolinium concentration by the multiple flip angle technique gives a more accurate result.
Number of Inputs | Number of Compartments | Type of Model | Parameters Derived | Reference |
---|---|---|---|---|
Single-input | Single-compartment | Conventional Compartment | K trans (transfer constant, commonly taken as permeability-surface area product) v e (% interstitial space) | Tofts |
Single-input | Single-compartment | Conventional Compartment | F (blood flow) V IV (% intravascular space) | Scharf |
Single-input | Dual-compartment | Distributed Parameter | BF (blood flow) MTT (mean transit time) PS (permeability-surface area product) BV (% intravascular volume) | Sahani , Chen |
Dual-input | Single-compartment | Conventional Compartment | Hepatic arterial flow Hepatic portal flow % Intravascular space | Materne |
Dual-input | Single-compartment | Conventional Compartment | HPI MTT (mean transit time) % Intravascular space Total hepatic blood flow Hepatic arterial blood flow Hepatic portal venous blood flow | Cuenod |
Dual-input | Single-compartment | Conventional Compartment | F (total liver blood flow) DV (% intravascular space) g (% flow due to hepatic artery) | Miyazaki |
Dual-input | Dual-compartment | Conventional Compartment | Fa (arterial plasma flow) Fv (portovenous plasma flow) v e (extracellular volume) Ki (intracellular uptake rate of gadoxetic acid) | Sourbron , Armbruster |
Dual-input | Dual-compartment | Distributed Parameter | F (total hepatic flow) A (% flow due to hepatic artery) F A (hepatic arterial blood flow) F PV (hepatic portovenous blood flow) t 0 (bolus arrival time) v1 (% intravascular space) v2 (% interstitial space) PS (permeability-surface area product) E (extraction ratio of tracer from single passage through vascular space) | Koh |
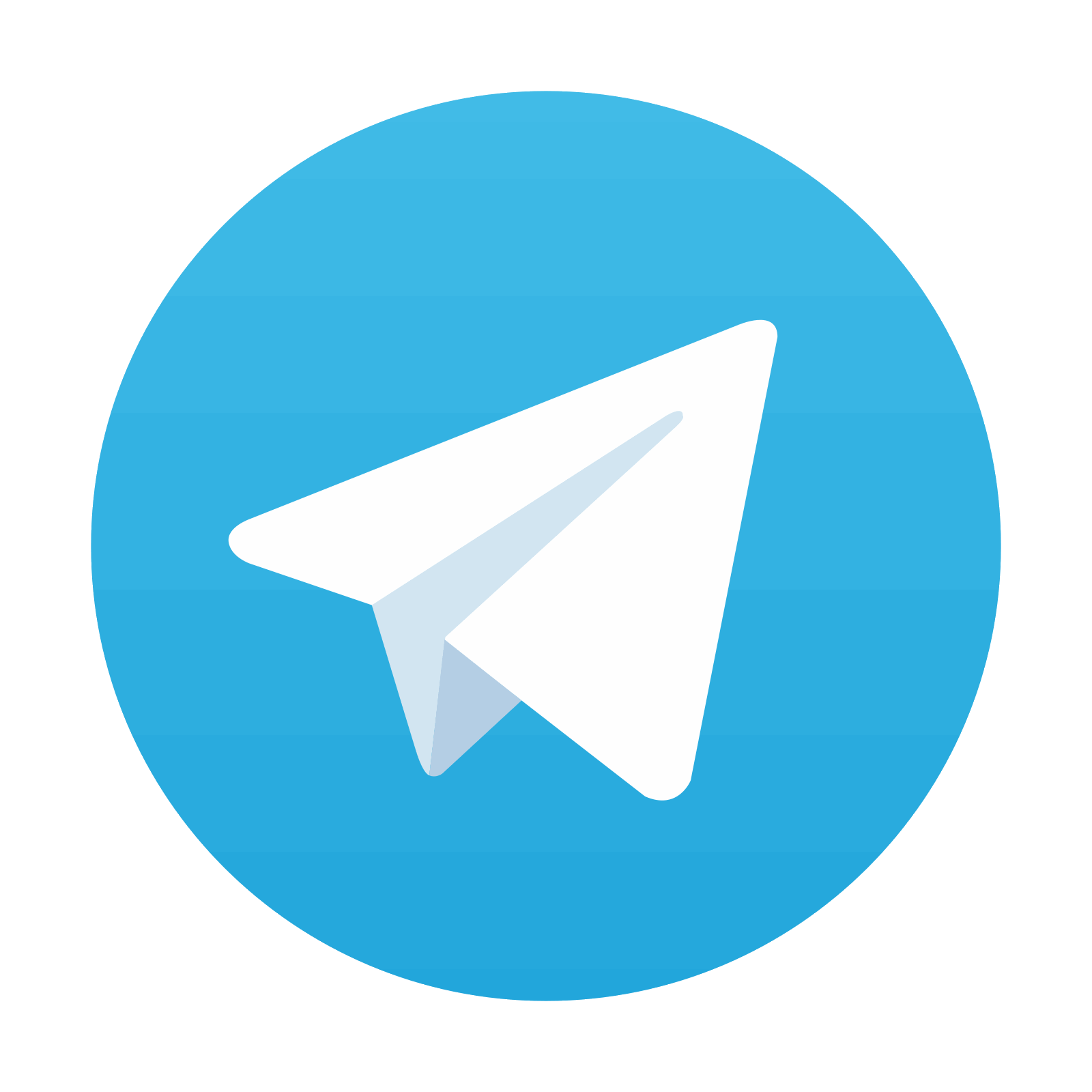
Stay updated, free articles. Join our Telegram channel

Full access? Get Clinical Tree
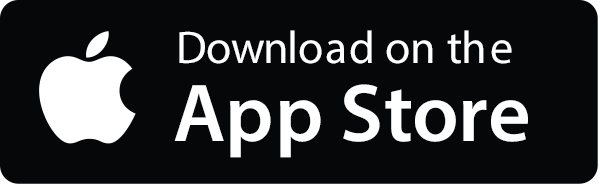

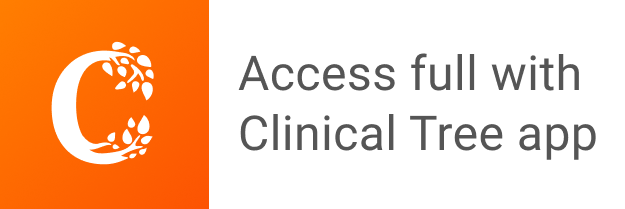