Benjamin LEPORQ1, Thomas CHRISTEN2 and Ludovic DE ROCHEFORT3,4 1 CREATIS, CNRS, Inserm, INSA Lyon, Université Claude Bernard Lyon 1, France 2 GIN, Inserm, Université Grenoble Alpes, France 3 CRMBM, CNRS, Marseille, France 4 CEMEREM, AP-HM, Hôpital de la Timone, Marseille, France Conventional MRI produces images whose voxels represent signal intensities weighted according to different physical phenomena such as relaxation, diffusion and perfusion. The value of the signal intensity is intrinsically dependent on the chosen acquisition sequence parameters and hardware. Quantitative MRI, on the contrary, seeks to produce maps where the value in each voxel is a quantitative measure of a physical parameter (e.g. T2 relaxation time) or a parameter with biological, biochemical, functional or molecular meaning. The value of quantitative imaging lies in the objective information that it can provide, regardless of the scan protocol or hardware. Therefore, it is aimed at the research and reproducibility of imaging biomarkers, which are objectively measurable features by definition, as indicators of pathological processes or treatment response. From a technical point of view, quantitative MRI exploits advanced pulse sequences to measure signals that contain parametric information which has to be quantified. These signals have to be modeled, preferably using physical models, and an inverse problem has to be formulated to evaluate the quantitative values of a target parameter. Depending on the complexity of the problem, it can be solved using different numerical methods including optimization, regularization, or dictionary-based techniques in combination with machine learning approaches. Given the complexity of biological processes, several biomarkers are often required to properly characterize tissues. Besides using several separate quantitative MRI sequences, it is also possible to use a single “multiparametric” acquisition. However, this kind of simultaneous sequence containing multiple measurement dimensions carries the inconvenience of increased scan times (to obtain sufficient information) as well as reconstruction times (model complexity). To reduce them, emerging research works measure several parameters simultaneously using a single fast scan. In this chapter, we will present three different approaches enabling fast multiparametric quantitative imaging. The absence of a refocusing pulse in gradient echo imaging sequences adds undesired components to the signal, resulting in artifacts. Mostly, these effects are related to the chemical shift and macro- and microscopic inhomogeneities of the magnetic field B0. As described in Chapter 9, the chemical shift phenomenon is a modification of the resonant frequency of the protons within a molecule due to differences in the electronic environment. The protons of water and those of methylene groups, the main components of lipids, differ between them by a chemical shift of 3.4 ppm (220 and 440 Hz at 1.5 and 3.0 T, respectively). Without a refocusing pulse, a medium containing water and lipids would exhibit a modulation of the signal related to the dephasing of isochromats in the spectroscopic domain (the echo time domain). As discussed in Chapter 1, the absence of refocusing pulses makes the imaging sequence sensitive to local inhomogeneities of the magnetic field B0. The latter lead to additional dephasing to the spin-spin relaxation of the T2 time constant, causing the relaxation to occur with the so-called T2* time constant. These field inhomogeneities are a superposition of several phenomena such as differences in magnetic susceptibility between the tissues, effects of the readout encoding gradients, shim coils and chemical shifts, among others. Among these mechanisms, magnetic susceptibility χ can contain useful information since it is a characteristic property of the tissues. Magnetic susceptibility represents the ability of a substance to magnetize under the effect of a magnetic field. It is expressed as a proportionality factor between the applied static magnetizing field intensity H and the induced magnetization M: where μ0 is the vacuum magnetic permeability (4π × 10-7 H·m-1). We have mentioned that the absence of a refocusing pulse in gradient echo pulse sequences can be a source of artifacts. However, if the latter can be undesirable for obtaining anatomical images for diagnostic purposes, some artifacts also carry a lot of information in terms of tissue characterization. Their common aspect is that they affect the MRI signal in the spectroscopic domain. In order to make use of them, it is thus worth recording the time evolution of the signal. For this purpose, gradient echo pulse sequences with multiple echos are favored. To extract quantitative information from a signal sampled in the spectroscopic domain tn, the signal has to be modeled. For this purpose, parametric and physical models can be employed. The physical models are more relevant than the parametric ones since their parameters have a true physical and biological meaning, while parametric models are purely mathematical. The parameters of the models are then estimated by solving an inverse problem using optimization techniques. The complex signal Š as a function of echo time (tn) for a gradient echo sequence in a medium with water and lipids can be expressed by the following equation: where ρw represents the proton density of water and lipids, and R2*w is the transverse relaxation rate of water. Contrary to water, lipids consist of a combination of several spectral components, each characterized by proton density ρk, a frequency shift with respect to water fk and a transverse relaxation rate R2*k. ψ represents the frequency shift due to the inhomogeneities of the B0 field. The k components of lipids observable in MRI correspond to the different chemical groups composing the triglycerides. Eight of them can be distinguished, as illustrated in Figure 10.1. Figure 10.1. Triglyceride molecule represented with its glycerol group and linoleic acid. The protons of the different chemical groups yield eight specific resonances (k) characterized by a frequency shift fk with respect to the water’s resonance The model’s equation contains 27 parameters. In MR imaging, this type of equation is impossible to solve because the number of unknowns is too large compared to the number of echos. In addition, the problem is ill-conditioned, which requires sampling the frequency domain with a higher temporal resolution than what is achievable. Hence, simplifying strategies have been proposed, consisting of reducing the number of unknowns using a priori knowledge without affecting the estimation of key parameters. In this context, the proton densities of water and lipids are two important parameters because they enable the calculation of the quantitative value of the quantitiy of lipids, the proton density fat fraction (PDFF). Simplification approaches consist of expressing a single lipid proton density from the proton densities ρk of each resonance by introducing weighting coefficients to represent their relative importance (Hines et al. 2009; Leporq et al. 2013). The values of these coefficients have been calibrated using proton spectroscopy measurements of oils and human fatty tissues (Hamilton et al. 2011). These studies have also provided exact measurements of frequency shifts, which can be set in the model. Another approach consists of considering only one transverse relaxation term for water and the different lipid components (Horng et al. 2013). Then, only three unknowns remain in the simplified model, which can be written as: where ρf is the global fat proton density, Ck is the weighting coefficients of each spectral component, with a sum equal to 1. Such a model enables the estimation of proton densities of water and fat, as well as the common relaxation rate. The fat fraction can be then deduced for each voxel as: The Dixon two-point method with spin echo was the first one to provide a true decomposition of water and fat signals using two echos: a standard echo and a second echo acquired by shifting the refocusing pulse with respect to the echo’s center such that the signal is encoded when the water protons and the main lipid component (methylene-CH2) are in phase opposition (Dixon 1984). However, this approach did not allow for accurate PDFF quantification due to confounding factors, such as the integration of all of the lipid spectral components, and it did not take into account the T2* decay. On the other hand, this approach is limited by “swap artifacts” (inversion of the water and lipid signals) caused by not taking into account the frequency shift related to B0 inhomogeneities. More recently, the IDEAL approach (Iterative Decomposition of water and fat with Echo Assymetry and Least-square estimation) has enabled the quantification of PDFF (Reeder et al. 2005, 2007). This method employs several echos and the equation parameters are obtained after linearizing the problem, which optimizes the computation time considerably. At further development stages, different confounding factors have been included, such as the integration of T2* decay into the model, taking into account all of the lipid spectral components, and the effects of complex Gaussian noise (Liu et al. 2007; Hines et al. 2009). Nevertheless, the problem remains ill-conditioned when the frequency shift related to field inhomogeneities is close to the frequency shift between water and lipids, additionally producing swap artifacts, in particular in the areas with strong field gradients due to magnetic susceptibility. The issue of “chemical ambiguity” can be solved by including a priori knowledge in the optimization procedure (Hernando et al. 2010). In order to tackle the effects of B0 field inhomogeneities, research teams have proposed to quantify PDFF and the relaxation time T2* from the signal’s magnitude (O’Regan et al. 2008). This approach provides the advantages of reduced effects of the complex Gaussian noise and robust and accurate quantification of PDFF. However, the loss of information related to the magnitude conversion does not allow for quantification of PDFF greater than 50%. For this reason, such methods cannot be used to investigate adipose tissues and are restricted to steatosis studies (presence of fat in organs). Although simplifying the signal equation yields a model function that can be exploited for PDFF and T2* relaxation time quantification in imaging, using a priori knowledge leads to a loss of information. In fact, the pre-calibration of the contribution of the eight lipid spectral components by attributing predetermined parameters supposes that the composition of fatty acids of the triglycerides does not change, which is not the case in practice. On the other hand, determining each component’s contribution could help quantify the fatty acid amount in lipids. For this purpose, a research team has proposed an intermediate solution that includes sufficient prior information to solve the system with a suitable sampling of the spectroscopic domain while providing measurements of the lipid composition (Hamilton et al. 2011). The number of protons per triglyceride nK is quantified for each spectral component as a function of three parameters related to the chemical structure of triglycerides: (i) ndb, the average number of double bonds per triglyceride; (ii) nmidb, the average number of double bonds per triglyceride interrupted by a methylene group; (iii) CL, the average carbon chain length. An illustration is represented in Figure 10.2. The model function (equation [10.3]) becomes: It should be noted that this reformulation leads to a change of variables since ρw and ρf do not represent the proton densities of water and lipids but the number of molecules of water and triglycerides, respectively. nw is the number of protons per water molecule (i.e. nw = 2). Figure 10.2. Triglyceride molecule represented with its glycerol group and linoleic acid. The number of protons nk of each spectral component k is expressed as a function of three parameters ndb, nmidb and CL Starting from this new model function (equation [10.6]), Peterson and Månsson have modified the IDEAL method to estimate these parameters and deduce from them the fractions of saturated, monounsaturated and polyunsaturated fatty acids simultaneously with the measurement of PDFF and T2* (Peterson and Månsson 2013). However, the degrees of freedom remain numerous, requiring the acquisition of a large number of echos to solve the system, which can lead to issues in the robustness of parameter estimation. To reduce the degrees of freedom of the model, Bydder et al. (2011) have shown that there was a heuristic link between the main fatty acids found in vegetal oils and in the living (fatty acid chain length comprised between 16 and 20 saturated, monounsaturated, and diunsaturated carbons), enabling CL and nmidb to be expressed as a function of ndb: From this work, an approach was proposed to simultaneously quantify the composition of lipids, adipose tissue, and T2* without requiring an additional unknown. To do so, Leporq et al. (2014) proposed a segmented approach, focusing first on the temporal evolution of the unwrapped phase of the signal to measure the frequency displacement related to B0 field inhomogeneities (𝜟B0) (first order dephasing) and the phase at the origin (zero-order dephasing). The phase of the signal is then corrected and the “demodulated” real part images are reconstructed (Leporq et al. 2014). This simplifies the model by neglecting the imaginary part of the signal and the dephasing term related to the field inhomogeneities as: A first nonlinear optimization enables the estimation of R2* and PDFF by considering a model with fixed Ck coefficients. The R2* can then be replaced in the equation above and, by means of two other subsequent optimizations, ndb and nmidb can be estimated to retrieve the lipid composition. In the previous lines, we mentioned that the frequency shift related to the B0 field inhomogeneities was a superposition of undesired effects and an inherent physical property of tissues, magnetic susceptibility. Thus, the maps of field inhomogeneity reconstructed with the method described above provide access to such information. Some research groups have shown that it is possible to decompose the field related to the B0 inhomogeneities into two contributions: an external field composed of low spatial frequencies that combines the macroscopic aspects, such as the effects of the shim coils or other undesired effects, and an internal field with high frequencies that results from the effects of internal susceptibility of the tissues (Schweser et al. 2017). To separate the two contributions, several approaches have been proposed. Among these, we can cite the methods based on filtering techniques such as those based on homodyne high-pass filtering of the complex image (Abduljalil et al. 2003), on a polynomial correction of the phase images; or methods using parametric models, in particular those employing spherical harmonics (SHARP (Schweser et al. 2011) method, followed by V-SHARP (Wu et Liu 2011) and RESHARP (Sun et Wilman 2014)) and the projection onto a dipole field (PDF method (Liu et al. 2011)) that exploits the principle of orthogonality between perturbations contributing to the internal and external field. The PDF and RESHARP methods are the most used ones. To estimate the magnetic susceptibility of tissues, an analytical expression of the internal field Bint induced by a distribution of susceptibility χ has been introduced by integrating Maxwell’s equations (Salomir et al. 2003; de Rochefort et al. 2010). By considering Maxwell’s magnetostatic equations with a Lorentz-sphere correction term, we obtain: where r is the position vector, θ is the angle between the position vector and the Overall, the internal field Bint can be considered as a linear system whose transfer function h(θ) is the equation of the magnetic field emitted by a unitary dipole, with the susceptibility distribution χint given as an input. Thus, we obtain: In the frequency domain (or k-space), we have: F represents the Fourier transform and H(k) is the dipolar kernel in k-space: Thus, calculating χint consists of inverting the equation above in k-space or performing a deconvolution in the image domain. However, this inversion leads to an ill-posed problem since the kernel can become equal to zero when k2 = 3 kz2, which represents a conical region in k-space observed from the three spatial dimensions and a spin orientation θ with respect to the B0 field equal to the magic angle (54.7°). In such cases, the consequences are amplified noise, and artifacts in the reconstructed image (streaking artifact). Several methods have been proposed to tackle this problem. Among the first ones, there were methods with thresholding of the transfer function and those based on Wiener deconvolution. Other approaches using regularizations have shown good performance in terms of stability and artifact suppression. de Rochefort et al. (2010) have introduced additional regularization terms based on spatial a priori data extracted from magnitude images to penalize the contours, together with boundary conditions and signal-to-noise conditions. We have described that the artifacts of the gradient echo scans can be exploited to simultaneously retrieve quantitative parameters for tissue characterization. The latter has several clinical applications, which mainly include hepatic diseases related to metabolism disorders and obesity. In fact, such patients have a high risk of developing nonalcoholic fatty liver diseases (NAFLD). These diseases are often asymptomatic and can evolve into severe conditions where steatosis (presence of intrahepatic fat) is accompanied by inflammation or fibrosis, the so-called steatohepatitis. If left untreated, these patients can develop cirrhosis or hepatocellular carcinomas. The methods described here could be an alternative to hepatic biopsies for monitoring such patients. Besides PDFF, which is capable of precisely measuring the amount of intrahepatic fat, Xie et al. (2013) have shown that magnetic susceptibility could enable the evaluation of fibrosis because the macromolecules that compose it increase the diamagnetism of the tissues. In chronic hepatic diseases, this is particularly important given that fibrosis is the main prognosis factor and influences the therapeutic strategy. Leporq et al. (2017) have shown that the simultaneous measurement of adiposity, lipid composition and magnetic susceptibility can provide a complete and quick analysis of the liver in a single breath-hold to distinguish simple steatoses from steatohepatitis in patients with NAFLD. Research works have exploited these techniques to evaluate bone health in osteoporosis. In the context of femoral bone marrow, while simple adiposity measurements via PDFF appear limited due to age-related confounding factors, considerable differences in lipid compositions, especially an increase of saturated fatty acids fraction, have been reported in postmenopausal patients compared to premenopausal ones (Martel et al. 2018). On the other hand, different lipid profiles have been observed within a population of postmenopausal women between primary osteoporosis and secondary osteoporosis in response to glucocorticoid treatment (Martel et al. 2019). Moreover, it has been shown that magnetic susceptibility measurements enable the evaluation of trabecular density in the calcaneum (Diefenbach et al. 2019). Finally, simultaneity could provide an overview of bone health for monitoring osteoporosis via the evaluation of lipid metabolism and trabecular density. Proof-of-concept investigations in healthy volunteers have also shown the value of simultaneous measurements of lipid composition and adiposity in nutrition studies for multi-organ monitoring of the therapeutic fasting effect (Viallon et al. 2019) or in monitoring an overfeeding protocol (Nemeth et al. 2019). We have seen that, by exploiting the information obtained from scans that sample the echo time dimension (or acquisitions sensitive to chemical shifts), relevant multiparametric information can be extracted. In the following, we cover other approaches capable of extracting multiparametric information from gradient echo acquisitions. In general, gradient echo sequences consist of quickly repeating (at every TR) (Brown et al. 2014) a radiofrequency pulse with an angle α, eventually accompanied by a slice-selection gradient. Between two consecutive pulses, a series of gradients partially cover the k-space. We have mentioned before that the apparent transverse relaxation modulates the contrast of this type of sequence through the echo time TE. It is also well known that the flip angle α and TR both affect longitudinal magnetization. Indeed, if the transverse magnetization is considered negligible between two repetitions, the signal obtained after some identical repetitions, at the “Ernst’s” equilibrium for the transverse magnetization, can be simply described as:
10
Physical-model-constrained MRI: Fast Multiparametric Quantification
10.1. Introduction
10.2. Multiparametric MRI based on chemical-shift-sensitive acquisitions
10.2.1. Signal’s origin and chemical-shift-encoded acquisitions
10.2.2. Physical models and optimization methods for the quantification
becomes a complex term whose imaginary part represents the transverse relaxation rate R2* and the real part corresponds to the frequency shift related to B0 field inhomogeneities (𝜟B0) such that:
field and B0 is the value of the applied static field.
10.2.3. Clinical and preclinical applications
10.3. Multiparametric MRI using steady-state acquisitions in repeated fast sequences
10.3.1. Steady state in a stationary sequence without transverse effects
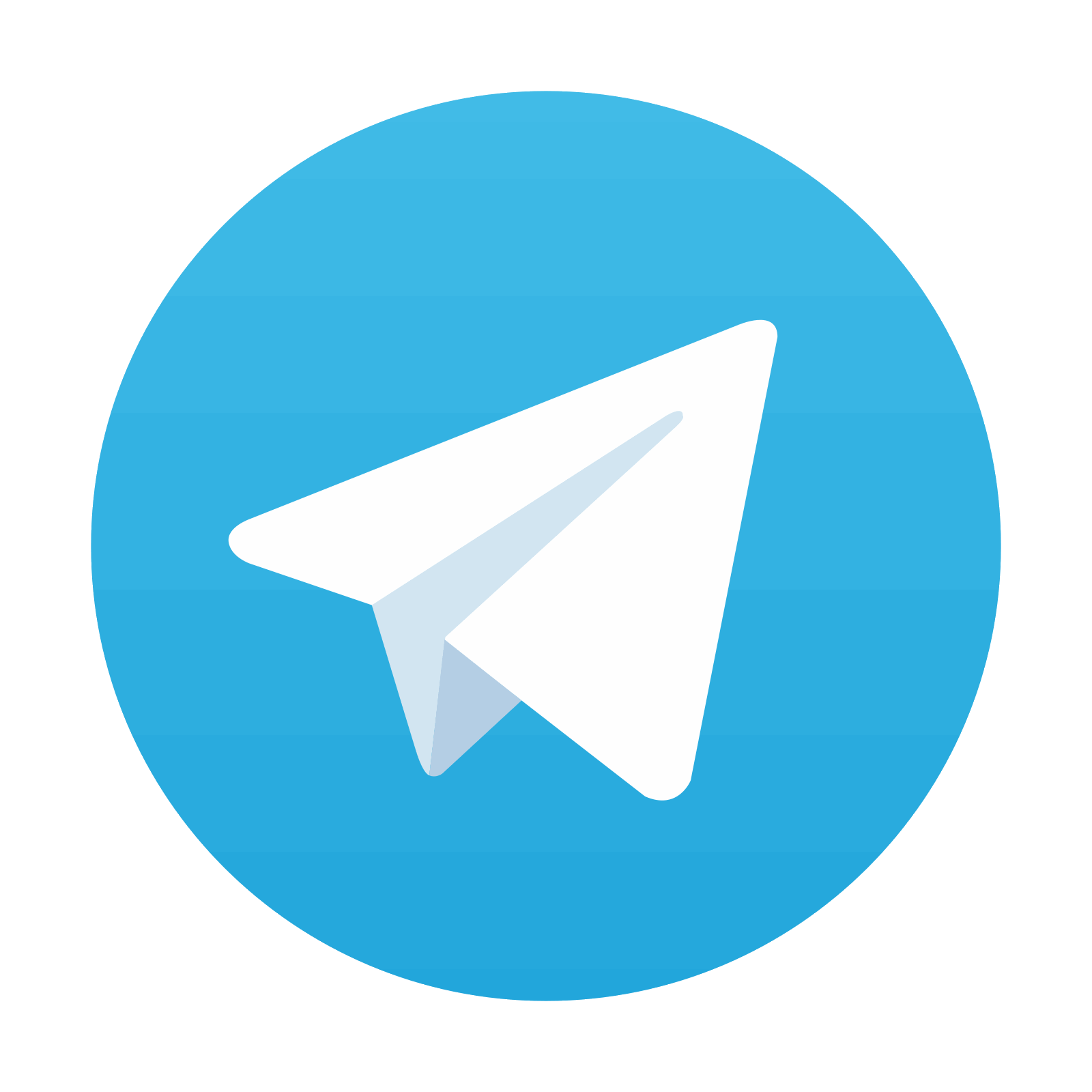
Stay updated, free articles. Join our Telegram channel

Full access? Get Clinical Tree
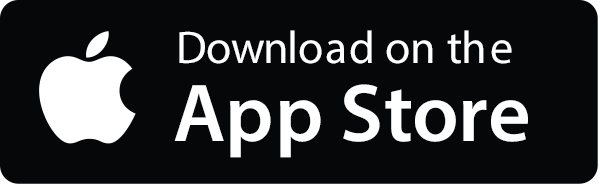
