27.1. Introduction
Although the practice of medicine is both science and art, major shifts are occurring as the scientific components underlying health and disease are better elucidated. A major contributor to the transformation of healthcare delivery is what is referred to as the “molecular revolution” in medicine. Progressively more diseases are being categorized by their molecular signatures (i.e., the collection of genetic and proteomic changes associated with a particular disorder), rather than the constellation of signs and symptoms with which patients present. As our knowledge of the extraordinarily complex biochemical and metabolic cellular pathways grows, and as technology gives us the capability to completely identify all of the molecules in a cell, it is likely that we will eventually discern that each patient has a unique molecular signature (phenotype) for whatever disease is present.
One consequence of this molecular understanding of disease and more scientific approach to the practice of medicine is the need for clinical tests that give reproducible, objective, quantitative information about biochemical and physiologic events in patients’ normal and abnormal cells and tissues. Since most disorders are likely related to abnormalities in the quantity of molecules that are otherwise structurally normal, or reaction rates in biochemical pathways where the molecules are structurally normal, it is increasingly important to quantitatively measure and monitor a diverse portfolio of molecular activities. Tests that simply indicate whether particular molecular events are present or not are increasingly insufficient. Tailoring therapy to the molecular heterogeneity underlying the molecular basis of disease has engendered the phrase “precision medicine” – i.e., the process of identifying the therapy, or combination of therapies, most likely to benefit a particular patient. As medical care shifts toward this molecular interpretation of disease and evidence-based model of care, treating physicians increasingly need: (1) tests to identify and quantify the biochemical phenotype of a patient’s disease so that it can be matched to the appropriate therapy; (2) tests to indicate whether a selected dose of drug is maximally effective for the given patient; and (3) tests to measure response to therapy so the therapy can be changed as soon as possible if the initial choice is not working as expected.
Much of the clinical information needed by contemporary medicine is provided by a variety of biomarkers. A widely-accepted definition of a biomarker, used by both the National Institutes of Health and Food and Drug Administration, is “a characteristic that is objectively measured and evaluated as an indicator of normal biological processes, pathogenic processes or a response to a therapeutic intervention” (Kessler et al., 2015). The term “biomarker” is often assumed to imply a laboratory test, but it can also refer to any clinically relevant measurement such as blood pressure or the measurable output of a clinical imaging scan. Imaging methods that provide information about the nature and amount of matter or activities present can be considered biomarkers and are conceptually similar to laboratory assays. An assay is a procedure that tests for the presence and amount of a chemical substance. Functional imaging methods, in particular, that provide quantitative biochemical information can and should be thought of as in vivo assays. Standard terminology and methods have become established in medicine to describe, evaluate, and validate laboratory assays. The same concepts and approaches should be applied to imaging assays, and this has begun to occur in an organized way in the past few years.
In addition to the fact that the molecular basis of disease is likely different in each patient, it is now apparent that the molecular profile often varies in different parts of the diseased tissue even within a single patient. Such a heterogeneous presentation of disease can be particularly challenging for conventional specimen biomarkers (tissue or fluid) that are typically obtained from a tissue sample (biopsy) and almost always represent but a tiny portion of the normal or diseased tissue. Therefore, for such specimen biomarkers, spatial sampling bias is an ever-present problem. Imaging scans, on the other hand, typically cover a broad segment or even all of the patient, and potentially provide more comprehensive information about normal and diseased tissue heterogeneity. Also, specimen biomarkers from biopsies represent a single point in time, whereas imaging data can be obtained dynamically. For these reasons, imaging biomarkers are of considerable interest in evidence-based clinical decision making and for therapeutic development (e.g., drug trials).
As healthcare delivery becomes more of a science and less of an art, the increased emphasis on evidence-based medicine requires treating physicians to integrate data from clinical examinations, laboratory tests, and imaging studies when constructing a treatment plan, and to assess and alter the plan as necessary during treatment. Such integration of data from multiple sources is becoming increasingly automated, and this requires that input data be machine-readable and, ideally, quantitative. Decision support tools and artificial intelligence (AI) algorithms need to perform their tasks using objective data. Thus, this new era of healthcare implies that clinical imaging must increasingly provide quantitative information that is relevant to the therapeutic options available to the treating physicians. Furthermore, data from controlled clinical experiments (especially randomized clinical trials) are the preferred source of information (as opposed to collections of anecdotal observations) on which clinical decisions are based. Controlled clinical trials are scientific experiments and therefore any imaging tests used as important (primary or secondary) endpoints in such clinical trials must also provide quantitative, not qualitative, results. As imaging becomes more sophisticated and more central to clinical decision making, any observed change or variation on a clinical imaging study should reliably reflect biology, and not a random difference due to instrumentation or subjective difference due to interpreting physician variability.
As healthcare resources tighten, there is an accompanying growing emphasis on measuring the quality of outcomes. Calculating metrics of outcome requires that the accuracy of information on which those outcomes depend be measurable. Consequently the value of various imaging tests to patients and society is increasingly being scrutinized. Thus, economic forces are also contributing to the need for outputs from diagnostic tests to be objectively measurable. The net effect of these shifts in our healthcare system is that diagnostic information must be expressed in a quantitative form so that information from clinical examination and diagnostic tests can lead to and ensure personalized, predictable, and reproducible outcomes.
Clinical radiology has been slow to embrace advanced quantitative measures. One study found that only 2% of 761 computed tomography (CT) and magnetic resonance imaging (MRI) reports contained an advanced quantitative metric, defined as a “numerical parameter reporting on lesion function or composition, excluding simple size and distance measurements” (Abramson et al., 2012). Even for simple measurements such as size, radiologists have generally been reluctant to actually measure structures on images and record those measurements in the radiologic report. The reluctance seems related to two factors. One is that radiologists are skeptical about the accuracy of measurements made on clinical images. Second, radiologists think it is unlikely that substituting a measurement of some structure for a qualitative statement about its size would actually lead to clinical benefit.
One example of this is tumor size measurement. In oncology, although tumor measurements are typically mandatory in clinical trials, it is unusual for radiologists to measure and record tumor measurements in their routine radiologic reports. They believe it is sufficient to state qualitatively that the tumor is getting larger or smaller or staying the same. Oncologists, however, faced with an increasing array of therapeutic options and responding to pressure to practice evidence-based medicine, do use linear tumor measurements in their decision making and would like radiologists to record such measurements in their reports (Jaffe et al., 2010). There are similar examples of differences of opinion between radiologists and treating physicians about the accuracy of and clinical value of anatomic measurements in cardiology, neurology, orthopedics, and other specialties.
The practice of radiologic interpretation of clinical images by human observers is a complex mental process that is not completely understood, but is extensively explored in other chapters of this book. In simple terms, a radiologist examines an image and identifies regions of interest (ROIs). These ROIs usually include one or more areas judged to be abnormal and, for comparison purposes, one or more areas judged to be normal. This process of choosing ROIs, whether by a human observer (such as a radiologist) or a machine (such as a computer algorithm), is known as segmentation. The radiologist performs a mental comparison of the chosen ROIs against expected norms, assessing whether attributes such as size, structure, or intensity of signal differ from expected, and makes judgments about the potential clinical importance of the presence or absence of such differences. This sequence of events (i.e., an observer (human or machine) identifying areas of interest and making probabilistic conclusions about them) is perception.
However, studies performed since the 1940s, combined with years of clinical experience, tell us that for human observers this subjective process of identifying ROIs in images and mentally applying comparisons to them results in significant intra- and interreader variability (Birkelo et al., 1947). Computer algorithms can potentially perform similar tasks on digital images and do so with more consistency than human observers. Thus, “perception” can be considered as something that can also be done by machine, and that is the topic of this chapter.
In general terms, the differences in interpretations among radiologists are due to, among other things, differences in skill and in value judgments. Skill manifests itself in how accurately a radiologist identifies all the normal and abnormal structures in a given image. The radiologist’s system of values influences what qualitative interpretation or emphasis the radiologist then places on those findings. For example, radiologists who report every possible abnormality, even if minor and not likely to be of clinical importance, are generally referred to as “overcallers.” This type of radiologist emphasizes the parameter of diagnostic sensitivity in his or her reports. Conversely, radiologists who downplay the clinical significance or importance of various deviations from normal and even omit them from their reports are often referred to as “undercallers.” These radiologists emphasize the parameter of diagnostic specificity in their reports. Both of these attributes (i.e., skill and value judgments) are very hard to modify in a given individual, and therefore it is extremely difficult to reduce variability in a group of radiologists by trying to alter either of these two factors. Between the two, skill is more amenable to improvement. Skill can be improved by real-world practice and feedback from known truth or the opinion of an acknowledged expert (i.e., “teacher”). On the other hand, the system of value judgments that an individual has is extremely difficult to modulate. However, education and repeated feedback about the individual’s relevant performance metrics may have some impact on value judgments.
This variability among radiologists has been recognized as a significant problem for the specialty of radiology (Macari and Megibow, 2011). Seeking to reduce the variability of qualitative interpretations by somehow making radiologists’ skill and value judgments more uniform, however, is very challenging, as noted above. Therefore, developing methods of extracting results from clinical images that depend to a lesser extent on radiologist involvement or input has the potential to improve the reproducibility of clinical imaging studies. Extracting reproducible quantitative measurements from images is one such method. Technological improvements in clinical imaging scanners, coupled with advances in contrast agents (especially radiopharmaceuticals) and computing power, make it feasible to extract quantitative parameters from all clinical imaging modalities.
27.2 Definitions of Quantitative Imaging and Quantitative Imaging Biomarkers
Quantitative imaging refers to the extraction of quantifiable features from medical images for assessment of disease, injury, or chronic conditions. The term quantitative imaging has been formally defined as:
the extraction of quantifiable features from medical images for the assessment of normal or the severity, degree of change, or status of a disease, injury, or chronic condition relative to normal. Quantitative imaging includes the development, standardization, and optimization of anatomical, functional, and molecular imaging acquisition protocols, data analyses, display methods, and reporting structures. These features permit the validation of accurately and precisely obtained image-derived metrics with anatomically and physiologically relevant parameters, including treatment response and outcome, and the use of such metrics in research and patient care (Sullivan et al., 2015).
By combining the definitions for biomarkers and quantitative imaging, we can define a quantitative imaging biomarker (QIB) as “an objectively measured characteristic derived from an in vivo image as an indicator of normal biological processes, pathogenic processes or a response to a therapeutic intervention” (Sullivan et al., 2015).
Clinical images are intrinsically quantitative. The process of administering energy of known quantity and distribution to a living organism, and measuring with spatial and/or temporal localization the energy that is emitted, transmitted, or reflected, lends itself inherently to quantitative interpretation. For example, the difference between the quantity of radiation administered and the quantity detected tells us something about the properties of matter with which the radiation has interacted. Most current methods of medical imaging involve the solution of inverse problems. That is, computer algorithms provide an estimate or hypothesis as to the nature of matter that the radiation (ionizing or nonionizing) encountered, and this estimate is displayed as the image we view. Many of our conventional imaging methods process and/or display the difference between administered and detected radiation in a way that tells us primarily about the structural properties of the living subject. However, there are also many imaging methods that reflect information about the chemical properties of matter with which the radiation has interacted (e.g., molecular imaging).
The general concept of clinical imaging described here (i.e., recording energy signals with temporal and/or spatial localization) means that all clinical images are inherently n-dimensional (n-D) data sets. In other words, every digital clinical image is a set of numbers and is therefore fundamentally quantitative. Today, virtually all clinical images are digital. Every pixel or voxel has a number associated with it. Many standard clinical images are two-dimensional data sets; others such as volumetric CT, MRI, positron emission tomography (PET), and tomosynthesis images are three-dimensional data sets; and dynamic volumetric studies are four-dimensional data sets. All medical images are ordered sets of numbers.
During the past two decades, remarkable advances in medical imaging technology have made it possible to obtain from clinical images high-resolution anatomic, functional, metabolic, and physiologic information, all of which reflect in some way the molecular substrate of the healthy or diseased tissue, organ, or person being imaged. With appropriate calibration, most of these imaging technologies can provide quantitative information about some properties of the material with which the applied radiation has interacted. For example:
The CT signal from soft tissues is proportional to electron density and the resulting images have high spatial resolution. Therefore, it is possible to obtain accurate distance measurements (e.g., tumor dimensions or volumetry), tissue characterization (especially with dual-energy CT), quantitative functional information with contrast agent administration, attenuation correction for PET/CT imaging, and electron density measurements for radiation therapy planning.
The PET signal is proportional to atomic decay events and has high sensitivity. Accordingly, if one knows the relevant physiology of the labeled substance being detected, it is possible to relate PET signal intensity to the molecular quantity present. The spatial resolution of PET images, however, is inferior to those obtained using CT or MRI.
MRI signals are complex. They are quantitatively, but nonlinearly, related to a wide range of intrinsic tissue parameters, including, but not limited to, T1 and T2 relaxation phenomena and proton density. Nevertheless, with appropriate calibration and standardization, MRI techniques can be devised for which the signal intensity is quantitatively meaningful in terms of specific properties of the tissue being imaged.
Ultrasound data are also complex, but with appropriate calibration and standardization quantitative information about attenuation, refraction, reflection, bulk tissue properties, and elasticity of the tissue being imaged can be calculated. In addition, quantitative distance and Doppler flow measurements can be extracted from ultrasound signals.
While each of these imaging modalities can provide image data that can be used to quantitatively assess tissue parameters, there are many technical obstacles hindering our ability to extract information from routine clinical images in objective, quantitative ways. The infinite variations in normal and abnormal biologic systems, and additional inconsistencies from the imaging devices themselves, make it very difficult to develop fully automatic (i.e., with no human intervention) segmentation or analysis algorithms. Nevertheless, some fully automated, and an increasing number of semiautomated, segmentation algorithms are improving in sophistication.
After normal and/or abnormal ROIs are identified and characterized, whether by computer or human observer, comparisons based on a variety of features are made (classification). AI techniques, such as neural networks, have been designed to try to replicate the results of the human cognitive process but, although developments in AI in many applications are impressive, it has proved very difficult to develop computer algorithms that accurately mimic the analytical process that a trained radiologist uses in interpreting clinical images. This is one area where image perception research could be applied – helping better define exactly what image data (features) humans use during search and interpretation of medical images that could then be incorporated into the AI algorithms. Although the power of the human mind will need to be a component of the medical image interpretive process for some time to come, advances in computer-assisted segmentation and AI techniques are rapidly maturing and increasingly provide reliable quantitative data to aid the radiologist’s analysis of findings on clinical images.
To effectively extract quantitative information from a given image, we must consider a key question: what portion of the signal represented in a given pixel or voxel is the biologic signal of interest and what portion is noise? Each data point within the ROI (i.e., from each pixel or voxel) has an associated component of noise relative to the signal of interest. Some of this noise comes from normal, random variations in the physical, engineering, and manufacturing aspects of the imaging device, and some comes from biologic and physiologic variation in the subject being imaged. With regard to biologic noise, consider that even with submillimeter spatial resolution, a single pixel or voxel in a clinical image represents hundreds of thousands or millions of cells and orders of magnitude larger numbers of individual molecules. For example, if the biologic signal we are considering is glucose utilization from a fluorodeoxyglucose (FDG) PET scan, each single pixel or voxel in the PET image represents signals from hundreds or thousands of fluorine-18 (18F) atoms combined into a single number. Most of those 18F atoms will be attached to FDG (and therefore convey the glucose utilization information we are interested in), but not all. Furthermore, during the several minutes of time required to acquire the PET scan, the 18F-labeled molecules are affected by perfusion, diffusion, and transport mechanisms that are complex with regard to stimuli and with time scales that we do not completely understand. Therefore, because of all these physical and biologic sources of uncertainty, obtaining absolute quantification in dynamic biologic systems is extremely difficult.
To be useful in the era of molecular and precision medicine, biomarkers must be objective (ideally quantitative), accurate, and precise. The concepts of accuracy and precision, and the four common combinations of these parameters, are shown graphically in Figure 27.1.
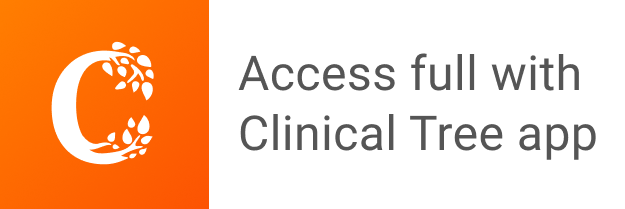