At the 2007 Radiologic Society of North America Meeting, Elias Zerhouni, MD (then Director of the National Institutes of Health) gave the Eugene P. Pendergrass New Horizons lecture on “Major trends in imaging sciences” (Zerhouni, 2008). His vision for the future of medical imaging was inspiring then and is still relevant today, noting that medicine in general is going through a transition from what he called the “hardware” phase of discoveries to the “software” phase. In the “hardware” phase the life sciences were geared toward understanding specific elements, while in the “software” phase we are now trying to understand how elements interact and work together in both normal and abnormal processes in anatomical form. As Sullivan and Jackson note in the present handbook (Chapter 27), these elements now include the “molecular revolution” that is propelling medical imaging and medicine in general into an entirely new realm of patient care possibilities.
The role of imaging in patient care is clear, as it has the potential to reveal structure, function, and metabolism in vivo. Because of this, medicine is entering a new era in which medicine will be predictive, personalized, pre-emptive, and participatory. Medical imaging is instrumental in advancing us toward the four Ps of the new medicine, as it offers a predictive and personalized perspective to each exam in such a way that can pre-empt medical intervention, and it does so in a participatory process with the patient and the healthcare providers.
The role of the human observer in this new healthcare environment is still (and always will be) critical. However, advances in computational methods offer an expanding role for computers in the analysis, assessment, and even interpretation of medical images and data. Today’s healthcare professionals use computers, decision support tools, electronic health records, telecommunications, and a host of other digital tools to render patient care. Mathematically based observer models rely heavily on computers to run and analyze simulated detection and classification tasks for technology assessments, image quality assessments increasingly use automated tools and techniques both in the clinical and in research studies, and artificial intelligence or deep learning techniques aim to “replicate” what the human image interpreter does using only computers. Yet in all of these applications, humans are the ultimate decision makers in today’s healthcare environment; without a fundamental understanding of what the human observer needs in terms of data acquisition and display, the technology and tools cannot be effectively applied. Thus, tools and technologies must be developed with the end user in mind and be optimized in ways to capitalize and leverage our perceptual and cognitive capabilities.
No matter where the future takes medicine and the imaging sciences, at the core of all image-based clinical specialties, especially in image-based specialties such as radiology and pathology, is the interaction of the clinician’s eye–brain system with visually presented medical image data. Any changes in the ways that images are acquired and displayed, and the information that they contain, will undoubtedly impact the clinical decision. As this book has hopefully demonstrated, our continued investigation into the mechanisms underlying this complex interpretation process will help ensure that the information presented to the clinician is done in an optimized and ergonomic fashion so that the detection and correct classification of abnormalities and diseases continue to improve.
Dr. Zerhouni noted in 2007 that the clinician practicing medicine in 10 years would, in all likelihood, be working in a very different environment. That in fact is precisely what has happened. In radiology, analogue films are a rare commodity, as are light microscopes in pathology. Clinicians in other specialties such as dermatology and ophthalmology are increasingly using images to render diagnoses and this is likely to grow even more rapidly as telemedicine reimbursement increases. There will be new types of digital images, acquired using different technologies than exist today, and processed in ways that have yet to be conceived. Molecular imaging is beginning to have an impact on the types of studies being done and the types of images being interpreted. The exchange of images and data from one specialty to another to enhance the decision-making process will also increase as hospital information systems become more sophisticated and integrated. As we move into the future and look further into the human body with advanced imaging techniques, we need to include the human interpreter of the data in our investigations and technology developments.
These goals are not new. In November of 1994 the National Institutes of Health, the National Cancer Institute, and the Conjoint Committee on Diagnostic Radiology sponsored a conference on Long-Term Plans for Imaging Research (Kundel, 1995). Five priorities for medical image perception research were identified: (1) develop psychophysical models for the detection of abnormalities in medical images; (2) improve understanding of the mechanisms of perception as they apply to medical images; (3) develop aids for enhancing perception that provide interactions between vision and displays; (4) study perceptually acceptable alternatives to sequential sections for viewing cross-sectional data; and (5) perform methodological research aimed at improving the evaluation of medical imaging systems.
After a series of Far West Image Perception Conferences that began in 1985, the Medical Image Perception Society (MIPS) was organized in 1997 to further promote these research goals and education in medical image perception, and to provide a forum for the discussion of perceptual, psychophysical, and cognitive issues. At that time, two articles were written by MIPS to update the goals of medical image perception research (Krupinski and Kundel, 1998; Krupinski et al., 1998). Those goals broadly included: (1) mathematical modeling of the detection task; (2) understanding visual search; (3) understanding the nature of expertise; (4) developing perceptually based standards for image quality; (5) developing computer-based aids to image perception; and (6) developing quantitative methods for describing natural images and for measuring human detection and recognition performance.
In 2001, the Far West Image Perception Conference matured into a new society and affiliated conference held every 2 years exchangeably in the USA and Europe. The society has also motivated and sponsored regular conferences with the Society of Photo-optical Instrumentation Engineers (SPIE) Medical Imaging Symposium and the Academy for Radiology and Biomedical Imaging Research. As an illustration of the ever-increasing influence of medical image perception research beyond radiology, the keynote speaker at the 2017 MIPS meeting was an ophthalmologist (Helen Li, MD) who highlighted how perception research with digitally acquired diabetic retinopathy images has contributed to establishing standards of clinical practice.
Even though computer-based image processing and analysis tools are becoming increasingly prevalent, as described in some of the chapters in this text, clinicians will continue to interpret medical images for many years to come and thus they will remain a central consideration in design, performance, and assessment of diagnostic systems. We need to understand the cognitive and perceptual mechanisms underlying reports of abnormalities and use this information to help guide the development of better acquisition and display devices, improving patient care and treatment. By studying how the observer samples parts of an imaging study and allocates attention and perceptual resources, we may inform the training of new readers and the development of effective machine readers.
Some topics we noted in the first edition for future research in this area are for the most part still quite relevant today, and in some circumstances even more so. We have added some new considerations to some of them.
1. Clinicians rarely get feedback about misses in clinical practice. Will this change with the Integrated Health Enterprise and will it actually impact how clinicians perceive/interpret images? Can we develop tools to provide such feedback and thus improve the efficiency and efficacy of training the next generation of image interpreters (see Chapter 31)? Will artificial intelligence and deep learning technologies be able to provide relevant feedback in real time in the clinical environment (see Chapter 27)?
2. Imaging clinicians must read increasing numbers of cases and images, resulting in perceptual and cognitive overload. We noted this over 10 years ago and the situation has actually exacerbated. Increasingly there are concerns regarding fatigue and burnout in medicine, and in radiology in particular. More research is being conducted on ways to improve the work environment to relieve physical, mental, and emotional stress and strain with the goal of reducing and hopefully avoiding costly and even deadly errors in image interpretation and patient care overall (see Chapter 32). Computer-aided detection schemes (see Chapters 24 and 25) and other image-processing and analysis tools can help, but they do not seem to help everyone and not in all interpretation tasks. Whether the current developments in artificial intelligence and deep learning will fare any better has yet to be established. Perception science can help better understand clinicians’ limitations as well as strengths (see Chapters 7 and 34) to better guide the development of perceptual and cognitive interpretation aids.
3. Molecular imaging is a reality and is increasingly having an impact clinically (see Chapter 27). It presents a whole new set of challenges to clinicians and so those studying medical image perception need to start looking at this area of imaging. How can we present this information to radiologists and other clinicians in a way that makes sense and can readily be incorporated into their decision-making process? Do we present images, or do we provide quantification of image information in some other format than the traditional renditions of anatomy and function (e.g., numeric data, color scales)? At what point do radiology and pathology become one science (see Chapter 33) as radiology gets closer and closer to the cellular level and imaging scientists develop imaging tools that can be used for virtual biopsies and intraoperatively to assess and characterize disease at the cellular level?
4. Increasingly, clinical decisions are made based on multiple types of images. New technologies (e.g., positron emission tomography magnetic resonance imaging, photon-counting computed tomography (CT), breast CT) are continuously being developed, as are new ways of rendering and presenting image data (e.g., three- and four-dimensional (see Chapter 21). How can we balance these new technologies and added sources of information with the challenges clinicians are already facing with the volume and variety of data currently available? Will virtual reality and other visualization technologies being used outside of medicine find use in medicine? What will we to do to adapt those technologies to the perceptual requirements of medical images?
5. Does the wide variability of vendor products used to present images impact the perception of images (see Chapter 29)? This seems likely and it has implications not only for clinical practice but for research as well. Perceptionists can help understand these differences and better optimize the imaging display (see Chapters 13, 14, 28, and 29), navigation tools, and reading environment.
6. Can standardized lexicons, training/search methods (see Chapter 31) and aids like checklists help reduce reader variability and errors such as satisfaction of search (see Chapter 10)? If we give the same tools to everyone it could be quite useful but would it simply take too long (if ever) to get everyone to the same level?
7. Is it worth developing training sets that can help with reducing disparities (see Chapter 31)? It would seem that the answer, at least in some areas such as mammography (see Chapters 22 and 23), is a definite yes, but can we extend these models to other types of images and disease entities?
8. Do we need more complicated receiver operating characteristic (ROC) designs – split-plot, unbalanced designs, and those that deal with missing data (see Chapter 15)? Most people still seem to be using basic ROC techniques developed years ago, even though much more advanced techniques are out there with validated software. Is it a publicity issue, lack of understanding, or lack of consensus within the scientific community as to what methods we ought to be using?
9. Detection of single abnormalities is still the core application for ROC, but there is the multiple-lesion issue, and the problem with truth or the gold standard. More concentrated efforts to define better ways of ascertaining truth are warranted.
10. Ideally, the way we measure diagnostic accuracy should emulate the interpretation tasks performed by the clinicians. Future research should focus on methods by which we can assess diagnostic accuracy that are most relevant in that way. For example, is the counterbalanced design used in many observer performance studies flawed due to perceptual memory effects (see Chapter 17)?
11. Is eye-tracking technology good enough yet for routine use in the clinic – could we use it as a feedback tool? Can we move visual search to more complex data – scrolling through CT slices and even looking at three-dimensional images (see Chapter 21)? Can computer-aided diagnosis (CAD) be improved with eye position recording? CAD misses some things and so does the human – if we combine them with eye tracking then can we do even better at finding abnormalities? How can we improve CAD technology and its assessment in general (see Chapters 23, 24 and 26)?
12. What can we learn about medical image interpretation from other realms of investigation? Are there lessons to be learned from studying basic perceptual and attention tasks using nonradiology stimuli (see Chapter 12)? Fundamentally our perceptual and cognitive capabilities rely on the same basic physiological mechanisms, so clearly the answer to some degree is yes, but are there unique skills or innate capabilities that radiologists possess that make them better at their tasks than others (see Chapters 7, 33, and 34)?
13. Are observer models useful for clinicians or are they just theoretical tools for researchers? Can we use the models for training? If we show what the ideal is and what is possibly preventing the observer from achieving the ideal, maybe perceptionists can help bridge the gap.
14. Most observer models are still very basic – simulated signals in simulated/real backgrounds without much visual search incorporated. Medical images are dynamic and much more complicated – we need to improve the complexity of the models being developed and used. New techniques are being developed to address these issues (see part III), but what more will be required as images become more complex?
15. Observer models are very useful to the extent they are validated against real or computational observers. But how can such models be considered validated? Would a sample application prove a model to be valid to be applied across other applications? If each application requires a validation of its own, what would then be useful about using observer models in the first place?
16. Observer models should be extended to enable processing image data from a variety of sources (e.g., multiple modalities, or temporal images), similar to the way clinical practice is increasingly performed – is this possible?
17. It is important to understand and correlate the various measures of image quality so that the results of different studies do not lead to conflicting conclusions. We need to have better quantitative metrics of image quality that are more closely and directly related to diagnostic accuracy (see Chapters 13, 14, 29, and 30). How easy are these methods and do they need to change as technology changes?
18. It is important to substantiate the impact of image quality on aspects of medical interpretations other than diagnostic accuracy. Those include timing efficiency, fatigue, and ease of interpretation (see Chapter 32), but do we also need to start considering ways to address the negative impact of technology on the clinician (e.g., increased volume and demand for more data interpretation)?
19. Given the multicomponent nature of medical imaging technology, it is important to characterize and optimize image quality in an integrated fashion, such that the relative contribution of each component is substantiated and the “bottle-neck” components are identified for closer examination and adjustment. How do we bring together the diverse investigators, medical physicists, statisticians, psychologists, human factors experts, engineers, and clinicians, to tackle these complex problems?
Image quality is obviously an important aspect of medical images. Image quality has many contributing components and many metrics by which it can be defined. The imaging chain is a complex sequence of events during which the image is susceptible to a wide array of potentially degrading factors that imaging scientists devote their careers to eliminating. The chain actually begins with the object being imaged (the patient) and proceeds through acquisition, transmission, display, and finally processing by the human eye–brain system. Since the images may need to be reviewed in the future, storage and retrieval also become important steps in the chain.
When dealing with image quality, it is tempting to think that the only thing of importance is the final “beautiful” image that the radiologist, pathologist, or other clinician views. The ultimate “quality,” however, should be judged not by our ability to provide clinicians with the most “beautiful” image, but with the image that helps them render an accurate interpretation in the most efficient and timely manner. The real question in medical image perception is not “is the image pleasing to look at?” but rather “can the information required to render an accurate and confident diagnostic interpretation be visualized by the human observer?”
One challenge in medical imaging, and medical image perception in particular, is that the definition and hence the quantitative and objective measurement of image quality differ depending on how the question is posed and to whom. If you take two images that you want to compare in terms of image quality and ask engineers “which one is better?” they are likely to suggest that you look at the raw image data and calculate some figure of merit such as mean square error or signal-to-noise ratio. If you ask the same question of medical physicists they will suggest measuring something like noise equivalent quanta or detective quantum efficiency. Image analysis scientists might argue that the “better” image is the one that a computer has analyzed or manipulated to provide CAD and diagnosis information to the human observer. Ask vision scientists and they tell you to measure perceived differences using figures of merit such as just-noticeable differences or to measure observer performance using ROC techniques. The clinician will say that the only valid metric is diagnostic accuracy and the ability to incorporate this into a treatment recommendation.
No single approach is the ultimate answer, as the chapters in this book have shown in the wide variety of approaches described to understand the medical image perception process. Medical image perception research needs to take into account all of these perspectives in order to better understand the interaction between the image and the eye–brain system of the person interpreting the image to produce the most accurate and precise clinical outcome.
It should be clear that there are many elements to consider when trying to optimize observer performance in the medical imaging environment. One must take the display, the room, the images, and especially the clinician into account to get a complete picture of the image interpretation process. Understanding even something as simple as how often a clinician should take a break from examining images to avoid fatigue and the probability of making errors is important. Even more important is to realize that our understanding of these factors and how the clinician performs is not a static question. Technology has changed and will continue to change and these changes will present new challenges to the clinician’s perceptual and cognitive systems. Our continued exploration into medical image perception and the way the clinician interacts with medical images will always be important.
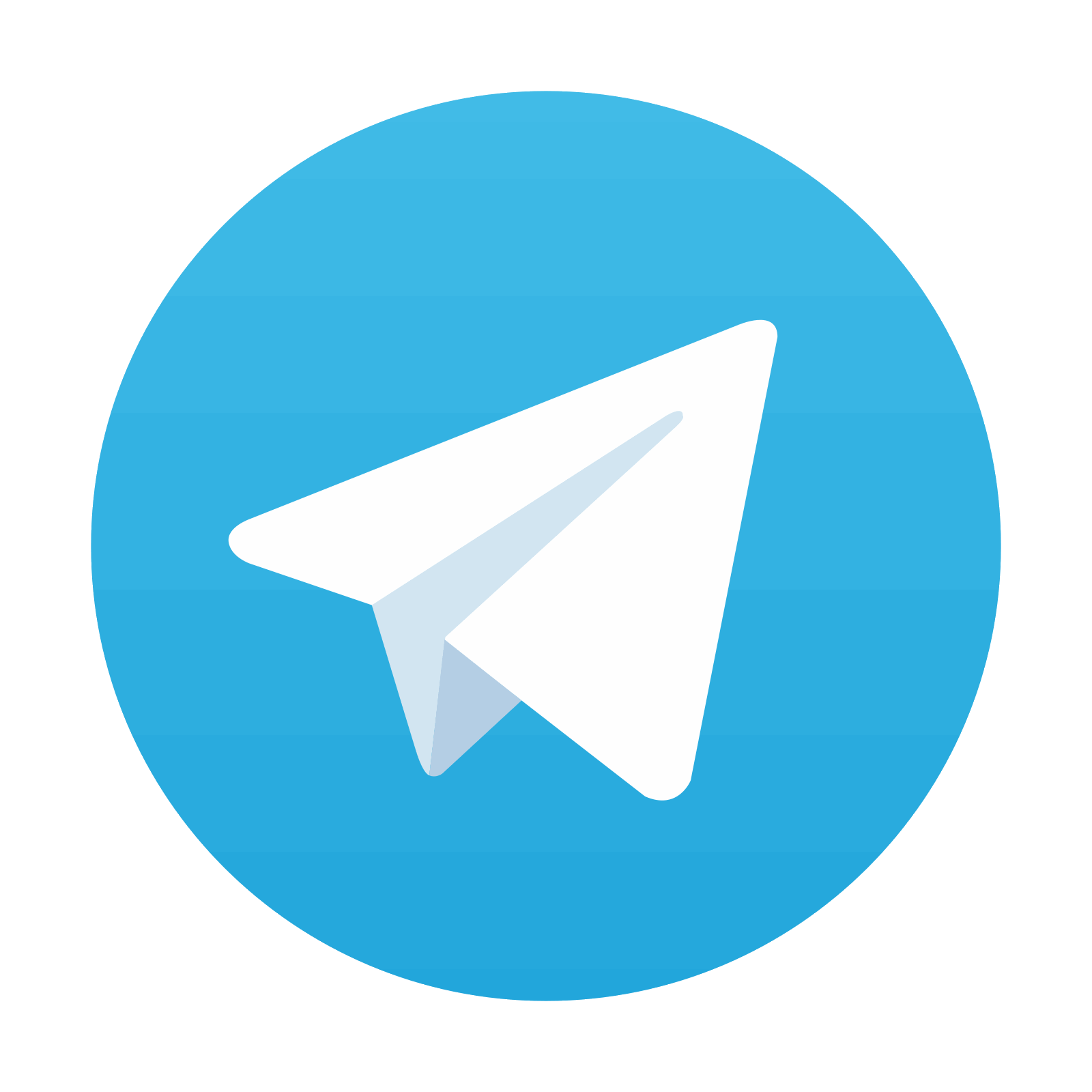
Stay updated, free articles. Join our Telegram channel

Full access? Get Clinical Tree
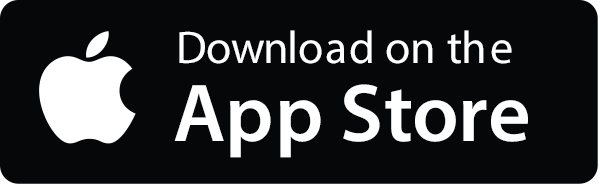
