The organizers of this project have given me the honor and the opportunity to look back on some of the highlights of my career in the field of image science. When I started in 1972, many of the pioneers in this field were still active, so these reminiscences will take us back more than a half-century. I spent many delightful conferences, discussions, and dinners with all of the people I’ll be referring to here. This review is just a sample of some of these memorable moments involving topics of continuing interest to many of us today. The chapter is organized according to the four categories of limitation to imaging performance: (1) quantum-limited imaging; (2) anatomical background-limited imaging; (3) artifact-limited imaging; and (4) reader- or doctor-limited imaging.
We trace some critical moments in the history of image science in the last half-century from first-hand or once-removed experience. Image science used in the field of medical imaging today had its origins in the analysis of photon detection developed for modern television, conventional photography, and the human visual system. Almost all “model observers” used in image assessment today converge to the model originally used by Albert Rose in his analysis of those classic photodetectors. A more general statistical analysis of the various “defects” of conventional and unconventional photon-imaging technologies was provided by Rodney Shaw. A number of investigators in medical imaging elaborated the work of these pioneers into a synthesis with the general theory of signal detectability and extended this work to the various forms of computed tomography (CT), energy spectral-dependent imaging, and the further complication of anatomical background-noise limited imaging. We call here for further extensions of this work to the problem of undersampled and thus artifact-limited imaging, which will be important issues for high-speed CT and magnetic resonance imaging (MRI).
6.1 Quantum-Limited Imaging
For most of us, the dominant figure from the mid-century was Albert Rose of RCA (called the Radio Corporation of America in my childhood). In the 1930s and 1940s Rose developed several advances in the sensitivity of pick-up tubes used in early television. He co-invented the Image Orthicon pick-up tube, which was used in guided missiles in World War II and served for several decades after the war as the major source of live television broadcasts.
Al Rose was doing fundamental research in photon detection and its conversion to electrical signals, and also had a keen interest in solar energy conversion. In the course of his many technological developments, he investigated the fundamental limits to image and signal detection using optical and electronic systems, including human vision and displays (Rose, 1946, 1948, 1953, 1973).
Rose realized that when imaging Poisson-distributed photons there are absolute limits on low-contrast detail detectability imposed by the statistical fluctuations in the finite number of detected photons. It is remarkable to observe today – given the fact that Rose’s work was taking shape just a few years into the quantum age – how he appreciated the fact that the granularity of light quanta imposed limits to practical imaging system performance. An ideal detector was one that was limited only by that quantum noise – and this served as the baseline against which the so-called detective quantum efficiency (DQE) of real detectors with greater noise could be measured. Rose thus put many phenomena on a common footing, including human and electronic vision and photography.
Rose used his classic sequence of six images of the woman with flowers (Rose, 1953) – at different levels of exposure and therefore different signal-to-noise ratios (SNRs) – to estimate the DQE of practical imaging systems. He imaged that sequence of images with the system under test and observed how the results matched the originals with respect to the quality of what could be seen.
One of my favorite examples where he used that work was his analysis and prediction (over 40 years ago) that home movies under “available light” using the photographic medium would not be able to compete with electronic movie making for picture quality (Rose, 1976). He provided arguments that the DQE of a practical detector working with available light would have to exceed that of the human eye (a few percent) by one to two orders of magnitude. (One order of magnitude has to do with the reduction in lens aperture – and thus loss of light – needed for depth of focus of the camera; the other is related to the fact that the movies will be displayed at a higher brightness than the recorded brightness, which will make the eye more demanding.) The DQE of photographic film was stuck in the order of magnitude of 1% and he expected the DQE for electronic devices to approach 100% – and it is roughly halfway there now. In any case, I no longer know people who are shooting home movies on film, so the day he predicted has already arrived. (Photographic purists still prefer the conventional silver halide medium when high magnification is required, but digital technology is rapidly approaching their needs.)
Albert Rose also contributed a fundamental tool to our culture that we use every day, namely, what we all call “the Rose model” of signal detectability. In that model (Rose, 1946, 1948), the “signal” is the average integral over the region of interest on the image (assumed known a priori) minus the comparable measurement over the same area of the background. The “noise” is given by the fluctuations averaged over the comparable area of the background. We refer to this as the simplest form of “matched filter” since one integrates over an area matched to the expected signal. All forms of SNRs that arise from more formal approaches to signal detection theory degenerate into the Rose SNR in the limit of a simple disc signal in a background of uncorrelated noise. An excellent historical overview of all of these issues has been given by Burgess (1999) and Burgess et al. (1999).
Otto H. Schade, Sr. – who also worked at RCA from the 1940s to the 1970s – was actually the first hero I discovered when I came into this field. Schade developed somewhat more elaborate models of signal detection than those of Rose. One of his monumental achievements was the analysis and consistent measurements of the imaging process of great interest to the TV viewing audience, namely, the chain from photographic movie capture on negative film to positive print and the optimal TV pick-up and coupling of that to a displayed version of the movie on a home TV screen (Schade, 1975).
I noticed that Schade’s SNRs – including display for human vision – were excellent approximations to SNRs that derive from formal signal detection theory for an observer we later called the nonprewhitening matched filter. This observer does not take advantage of the optimal preprocessing of correlated noise known as “prewhitening,” and so we started referring to it as the “nonprewhitening matched filter.” Several key references to the relationship between the performance of the prewhitening matched filter, the nonprewhitening matched filter, and the incorporation of an eye filter and/or a finite number of receptive fields or channels are reviewed in Burgess (1999), Wagner and Weaver (1972), and Barrett et al. (1993).
For the 50th anniversary of the landmark work of Albert Rose, a special issue of Journal of the Optical Society of America (Section A, Optics, Image Science, and Vision) was published in March, 1999. The editors of that issue (A. Burgess, R. Shaw, and J. Lubin) provide an anecdotal historical introduction (Burgess et al., 1999). Then Burgess (1999) provided an elaborate review of the way in which the Rose model and signal detection theory came together.
Burgess himself played many pivotal roles in this history. He spent a sabbatical around the year 1980, split between the Cambridge (UK) labs of the vision researcher Horace Barlow and our own imaging research group here at the US Food and Drug Administration (FDA) outside of Washington, DC. He used the concept of the ideal (or Bayes) observer of signal detection theory as the standard against which to measure human performance for detection and discrimination tasks with noisy images and found human observer efficiencies in the range of 25–50%. These results encouraged us that the approach to signal detection analysis that we were using was indeed quite relevant to the perception of noisy images by human observers.
One of the central figures in the field of image science has been Rodney Shaw. In fact, I believe the expression “image science” was formally coined with the appearance of the book of that title by Dainty and Shaw (1974). The first half of this book analyzes the photographic process from the point of view of statistical efficiency. Shaw greatly elaborated on the approach of Albert Rose and later Peter Fellgett (Shaw’s Cambridge University (UK) mentor) to reach some conclusions regarding the statistical efficiency (DQE) of photography that were surprising to many investigators at the time – and are still surprising to our contemporaries who are not familiar with their work. Looking back over the DQE culture launched by Rose, Shaw remarked (Burgess et al., 1999) that “the traditional photography community at first thought very little of having an absolute measure foisted on them by an outsider, especially since this measure made them custodians of a technology rated at less than 1% absolute efficiency.”
In the book of Dainty and Shaw, the authors review the set of fundamental “defects” that lead to the low DQE of photographic film. Film has a so-called “threshold defect” – a minimum of about three photons are usually required to render a grain developable (for protection against fogging). So the maximum DQE one can expect from film is in the order of 33%. In the interest of providing photographic latitude, this number is designed to be a variable – but this variability is itself another source of noise which degrades the DQE. The variable grain size and variability of grain position are further sources of noise. Finally, film also has a saturation defect – that is (at least for general black-and-white photography), a grain is a binary or two-level (off-on) recorder. This coarse degree of quantization degrades the DQE further. In the end, the overall DQE comes down into the range of 1%.
In more recent years Shaw (2003) has carried out an analysis of the process of digital photography and finds practical levels of DQE for that technology in the range of 10–30% (the latter for the black-and-white process).
An apparently subtle point was developed by Shaw in the course of all this work. Shaw spoke of the “inevitability” of the view that photographic noise will be thought of as amplified photon noise. His use of the word inevitability reflected the fact that a very long time was to elapse before this view became widely accepted in the photographic community (decades in the case of some investigators). The defects at each stage of the photographic process recounted in the previous paragraphs have an effect on the photographic SNR equivalent to that of a series of filters that would throw away corresponding fractions of the incoming photons – as in the famous family of six images of the woman associated with Al Rose’s early work (Figure 4.3). The resulting noise or SNR is thus equivalent to that which would result from random capture of a number of photons much smaller than the number in the incoming photon stream, the fraction being in the order of 1%. Photographic granularity or noise is thus not some additive aftereffect, but rather the result of this multiplicative cascade of random imperfect phenomena.
I always notice this phenomenon at work when I go to the movies and see a Western film and – presumably from now on – the reader will too! Whenever there is a scene of the wide-open sky, the film granularity is obvious not only because the scene is fairly uniform there but also because the noise is more perceptible when the scene is brighter. That is, the visual threshold for detecting small changes in contrast is lower when there is more light. I always think at this point, “I’m viewing amplified photon (or quantum) noise!” This may be among the few moments in life when the granularity of light photons is experienced so vividly. We rarely experience this with ordinary vision because the amplifier gain of the visual process appears to be set so that the noise is not quite visible (except at very low levels of light). (The reader who wonders why this noisy-sky phenomenon is not so obvious when watching Westerns on TV is encouraged to simply get up and turn off the room lights the next time such a scene comes on to the screen.)
Our appreciation of the work of Rose, Schade, and Shaw and our study of signal detection theory led my colleague David Brown and me in the mid-1980s to a formulation of the ideal observer performance for ten different modalities and geometries then used (or soon to be used) in medical imaging (Wagner and Brown, 1985). Kyle Myers, a protégé of Harry Barrett at the University of Arizona, soon joined our group and we were then invited by Professor Peter Sharp of Aberdeen to serve on the International Commission on Radiation Units and Measurements (ICRU) Report Committee #54. A synthesis of the fundamental principles of medical image science that derive from this and related works, together with many applications, was published in that report (International Commission on Radiation Units and Measurements (ICRU), 1996).
An interesting historical sidelight was the fact that, before the appearance of the ICRU report, there were some investigators in the medical physics community who claimed that the model underlying the so-called noise-equivalent quanta (NEQ)/DQE approach was not so obvious as its proponents claimed. NEQ is the numerator that combines the classical imaging measurements – including the modulation transfer function (MTF) and the noise power spectrum – in the modern formulation of DQE. Our response was that the NEQ/DQE approach is not a model (Metz et al., 1995). NEQ is simply a scaling of the measured noise power back to the axis of the exposure quanta through the system transfer characteristics. The comparison of the measured NEQ (what the image is “worth”) with the measured level of the exposure quanta (what the image “cost”) yields the DQE.
Several examples of the very good consistency between measurements and analysis following ICRU Report #54 compared to results of human observer experiments were given by Gagne et al. (1996). This consistency is our common experience when the task is simple signal detection against the background of a uniform test phantom.
One of the early applications of this approach makes an interesting historical point. When CT first became available it was not obvious why the exposures or doses were so high. The algorithm developers at the time thought it had something to do with the algorithms being suboptimal. My colleagues and I measured the physical characteristics of CT scanners – including the noise power spectrum of CT images on the absolute scale provided by CT numbers and their calibration to the attenuation of water – and compared them with what would be expected from ideal detection and reconstruction. We found that the two results were similar. (We are ignoring here the major problems with collimation in some early systems.) In our 1985 paper (Wagner and Brown, 1985) and the ICRU report (ICRU, 1996) we showed how the noise multiplexing intrinsic to the CT process was responsible for the high exposures required – a natural phenomenon that could not be finessed by better algorithms. The noise multiplexing arises during the fundamental process of strip or ray integration in CT; the signal of interest is collected from a region of a strip containing a lesion, say, while noise is collected from the entire strip (and ditto for every projection or view). This phenomenon is responsible for the high exposures necessary to achieve the great step in improved low-contrast sensitivity provided by CT. But the benefit is obviously not having to saw open the body for the task that CT addresses.
At that same time, Markku Tapiovaara was visiting our labs from the Radiation and Nuclear Safety Authority (STUK) in Finland. As he was preparing to return to Finland he asked us if we had some further problems we were worrying about at that time. We did – we were wondering about the issues that arise from the fact that X-ray detectors essentially measure an energy or current rather than counting photons.
Markku’s work on that problem led to an elegant formulation of the solution that we call the matched filter in the energy domain (Tapiovaara and Wagner, 1985). The idea is analogous to looking for an orange in a noisy black-and-white photograph of a “still life.” Such an image suppresses the energy or spectral information in the original scene. However, if the spectral information is available – say, for the task of discriminating various kinds of soft tissue and/or calcifications in medical imaging – that information can be optimally weighted just as one does when one looks for the orange in a color photograph that carries the spectral information. Optimal image detection based on these principles will soon become available with photon-counting detectors and optimal signal processing (Lundqvist et al., 2003).
The history of our understanding of photon detectors that both amplify the incoming primary events and scatter the resulting secondaries provides another fascinating chapter of modern image science. Classical literature on this subject dates to the 1930s, but was limited to the large-area response. Shaw had generalized the earlier results into the spatial frequency domain and fine-detail response using semiquantitative arguments based on well-understood limiting cases. A landmark contribution to the classical literature in this field – which capitulates much of the earlier work – was the paper by Rabbani et al. (1987) that provided a more general approach to that problem. This work was reviewed and extended to complex multistage systems by Cunningham and Shaw (1999) in the special Rose celebratory issue of Journal of the Optical Society of America cited earlier. We encourage young investigators to become familiar with this literature because many are surprised to learn that the Poisson component of the radiation stream goes through an amplifier uncolored by the system transfer function. Only the non-Poisson component gets weighted by the square of the system transfer function or MTF. This has many practical implications; in particular, it does not allow one to measure the MTF from the color of the output noise in a system with a Poisson-distributed input. Harry Barrett “reminds” his students that Poisson is French for “independent”!
The work sketched in the previous paragraph depends on the assumption of noise stationarity and the appropriateness of noise power spectral analysis. More recently, Barrett and colleagues (Barrett and Myers, 2004; Barrett et al., 1997) have provided a more general approach to this problem based on correlated point processes that does not require this assumption.
6.2 Anatomical Background-Limited Imaging
We traditionally justified the use of simple phantoms and Fourier analysis of low-contrast detectability (e.g., NEQ and DQE analysis) because historically gallbladder imaging and angiographic imaging were considered to be quantum-limited tasks, and we always wished to be conservative vis-à-vis those tasks.
Nevertheless, all these years we knew from the work of Harold Kundel and colleagues that real medical images give rise to limits to detection – often the dominant limitation – from the very anatomy itself, chest imaging being the paradigmatic example (Kundel, 2000; Samei et al., 2000).
A landmark step in the direction of a combined analysis of quantum-limited and anatomical-limited imaging has been taken by Arthur Burgess and colleagues in recent years (Burgess et al., 2001). They analyzed the limits to lesion detectability generated by the variable tissue background in mammography. Modeling that background as power-law noise, and using the kind of model observers discussed in ICRU Report #54 (ICRU, 1996) (especially the appendices), they found very good agreement between the performance of human observers and that of the model observers. A striking result of that work was the fact that lesion detectability degraded with increasing size of the lesion in mammographic backgrounds whereas lesion detectability improves with increasing size of the lesion in the elementary Rose model-like SNRs and simple phantoms where the background is uniform. The problem of sorting out radiation-limited tasks from anatomical-limited tasks has emerged as one of the dominant themes in contemporary research (Tingberg et al., 2004).
6.3 Artifact-Limited Imaging
An image is always a sampled version of the input scene or object, and this is particularly obvious with digital imaging systems. It is well known that undersampling of the original image scene leads to artifacts such as streaks, rings, or other patterns in the image that do not correspond to the original scene or object. Perhaps the most familiar example of this phenomenon is the Moiré pattern seen on TV images when the TV raster lines “beat” against a striped pattern of the clothing of a person on camera, resulting in the annoying fringe pattern that varies with the movement or position of the person.
A very nice summary of the Fourier-based approach to the understanding of undersampling artifacts for linear shift-invariant systems – including the concept of aliasing – was presented by Albert and Maidment (2000), by analogy with the analysis of periodic structures in solid-state physics.
Barrett and colleagues (1995) have taken a more general approach to this problem that is free of the assumption of shift invariance, and have demonstrated the role in that analysis of the “cross-talk matrix” previously described by them. The diagonal elements of this matrix are analogs of the system MTF squared; the off-diagonal elements measure how much interference or “cross-talk” a spatial frequency component in one input channel generates in the other spatial frequency channels. It is the hope of these authors that someone might build a test tool to measure the cross-talk matrix someday, and we still maintain that hope. A remarkable curiosity is that the approach of Barrett et al. is based on a Fourier series analysis on a finite support, not Fourier integrals, and their Fourier series approach does not assume shift invariance. The papers by Albert and Maidment (2000) and by Barrett et al. (1995) show – for problems where the noise is stationary – that a form of shift invariance is induced by averaging over the continuous range of possible positions for the target or lesion of interest. Gagne has presented some nice examples of this phenomenon (Gagne, personal communication, 2004). He demonstrated how, for low-contrast targets in simple backgrounds with stationary noise, averaging over the position of the target allows one to use the NEQ-based SNRs of ICRU Report #54 even for digitally sampled imagery.
Artifacts are a major issue for CT and MRI. In 1980 Joseph and Schulz took a fundamental approach to the analysis of the artifacts in CT. We consider here only the special problem of the finite number of views (as opposed to the finite number of samples per blur spot, which affects the presence or absence of aliasing). For the special case of parallel-projection geometry, their analysis reduces to the following compact result for the minimum number of projections Nmin required for artifact-free reconstruction over a region within a radius R when the maximum spatial frequency in the reconstruction is vmax:

Joseph and Schulz (1980) gave very vivid practical demonstrations of this expression at work using 90 views. This work moved into the background as the number of projections used in two-dimensional X-ray CT was increased to 180 and 360 or more as the technology evolved. However, it is relevant again today in the field of MRI, as we mention next.
Some of the original MRI images were made using the projection-reconstruction (PR) geometry and reconstruction methods used in two-dimensional CT at that time (early to mid-1970s). Fairly soon, however, investigators discovered how to obtain MR images in two-dimensional Cartesian k–space, or spatial-frequency space on an x-y grid. Many methods were then explored for traversing k–space quickly and more sparsely in the interest of faster imaging.
A contemporary approach to this problem has been pursued by Mistretta and colleagues (Barger et al., 2002; Peters et al., 2000; Vigen et al., 2000), by reverting to the original PR geometry of CT imaging and obtaining speed by undersampling the number of views, with particular emphasis on three-dimensional PR MRI. These investigators demonstrated a 35-fold undersampling and associated speed-up for doing blood vessel imaging with an MRI contrast agent. For many applications, their so-called vastly undersampled isotropic projection imaging provides visual image quality competitive with much slower contemporary Cartesian-based approaches.
We mention these issues because a major goal of investigators from the school that I represent here is to devise model observers in the spirit of those used in ICRU Report #54 and related literature that are able to address the complication of the artifacts that arise in digital imaging in the presence of undersampling.
Myers et al. (1993) showed that it is indeed possible to devise such observers even for the extreme case of CT imaging using only eight views. These model observers were used to explore the dependence of image quality on the parameters of nonlinear algorithms used for fast undersampled systems. They showed that it was possible to design model observers whose performance tracked well with that of human observers of their images. We look forward to the day when test images of anatomically realistic phantoms that challenge deterministic artifacts can be quantitatively analyzed as readily as images of uniform phantoms of low-contrast signals are analyzed today using the NEQ/DQE approach.
6.4 Reader- or Doctor-Limited Imaging
In more recent years the general field of receiver operating characteristic (ROC) analysis for studying the performance of imaging systems in the clinical setting has evolved into a multivariate approach called the multiple-reader, multiple-case (MRMC) ROC paradigm. This situation was driven by the great variability observed in the measurement of performance of human readers for a wide range of clinically important imaging tasks. One of the more dramatic examples of this variability was that studied by Beam et al. (1996) for the population of US mammographers. The MRMC approach is now the predominant method for assessing medical imaging and associated methods of computer-aided diagnosis, both among academic investigators as well as sponsors of new technologies submitted for review to the FDA. An overview of the issues and several public case studies has been published by myself and a number of colleagues, as part of our efforts in the process of consensus discovery and guidance development for further industry and FDA interactions (Wagner et al., 2002, 2007). Some further practical advice for study designers was published in 2003 (Wagner et al., 2003). One of the contributions of our group in all of this is an approach for analyzing an observer study into its components of variance, allowing us to separate the uncertainties that arise from the readers – including the effects of computer aids to reading (Beiden et al., 2001) – from that which arises from the finite sample of cases and the underlying physics.
6.5 The Future
In this overview I’ve tried to provide samples of some critical moments in the evolution of our understanding of the problems associated with assessing the performance of medical imaging systems. During the last decade a major project was undertaken to analyze this problem in a very formal way using the same level of rigorous mathematics that was used in the development of quantum mechanics. A contemporary landmark of this project is the publication of Foundations of Image Science by Barrett and Myers (2004). This work includes the results of several decades of investigations by the authors and their colleagues, including many PhD and postdoctoral projects. It is easy to forecast that this monumental work will have an indefinitely long shelf-life. There is material in the book for launching countless new PhD and postdoctoral projects – and hopefully further efforts toward consensus measurements and guidance for practitioners in this rapidly maturing field that we share.
References

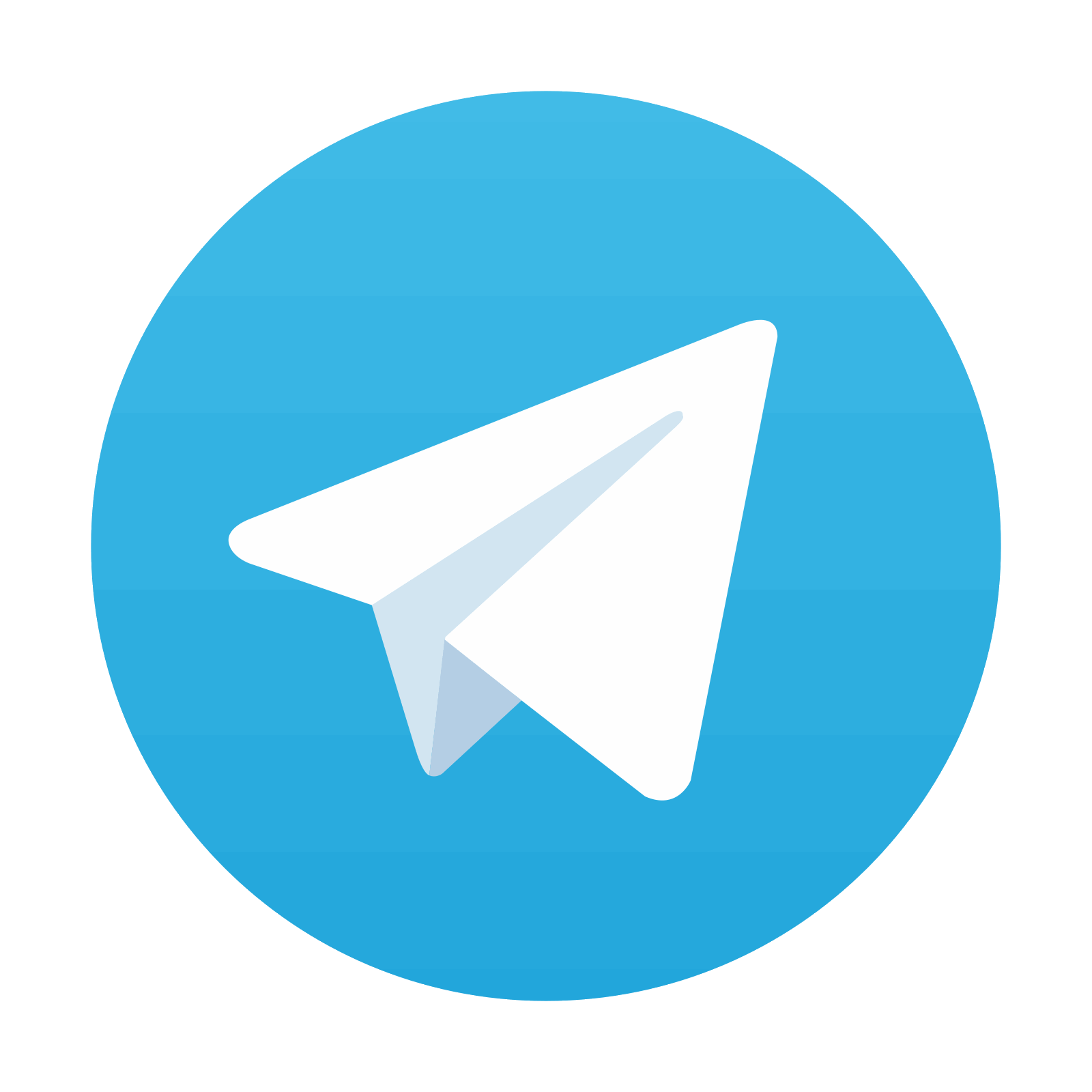
Stay updated, free articles. Join our Telegram channel

Full access? Get Clinical Tree
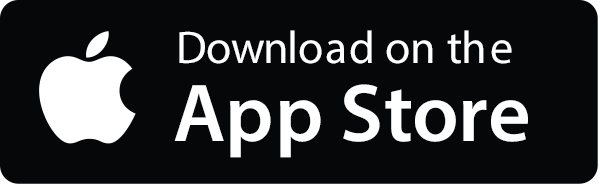

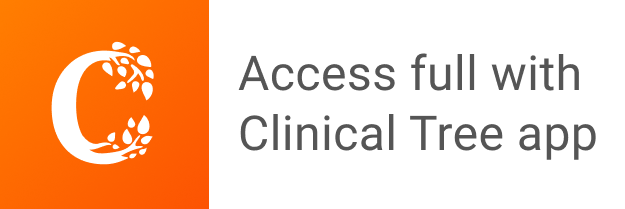