7.1 Clinical relevance
This chapter affirms the establishment of perception in the wider field of medical imaging. At present, the discipline continues with its strong dependence on fundamental science, informing our understanding of visual interpretation processes. But its clinical connections also highlight the importance of many research questions that are emerging from diagnostic policy and current practice, the economics of healthcare, as well as continuing changes to technology. How perception is studied and what aspects are targeted are implicated by the communication between these themes in medical image perception.
There are things known and there are things unknown, and in between are the doors of perception (Aldous Huxley).
7.2 Introduction
It is accepted that for medical imaging to have a positive impact on healthcare it is essential that images are read and interpreted and that an uninterpreted image therefore has no value (Beam et al., 2006). Medical images are not self-explanatory, are decoded (mostly) by human observers, and the process is subject to variance between and within subjects performing the task. Errors take place. Some imaging tests have a risk associated with them, so it is evident that they should never be performed without interpretation; but if the frequency of imaging events, their economic cost, and the diagnostic importance of the activity are included in this argument it becomes clear that not only should all images be read but that the process should have the highest level of quality control. It is perhaps a little surprising, then, that although great technical effort and innovation have refined the acquisition and recording of medical images for more than a century, perception and interpretation processes have only in recent decades been important in research and optimization programs.
Readers engage in the two related processes of perception and analysis when interpreting medical images. Perception is an awareness of the image content during its scrutiny, and analysis is determining the meaning of the perception in the context of the medical problem presented. Appropriately, radiologists have regarded image analysis as their primary field of interest, have assumed that what they perceive is a faithful representation of the images’ information, and have only been concerned with perception when it fails (Kundel, 2006). However, the failures of perception have inspired research into quantifying diagnostic performance, characterizing errors, redefining our concept of image quality, and understanding the nature of expertise, so establishing the discipline. This chapter makes note of some factors that have influenced perception research in the past and how some of its important contributions have brought about change. It will also emphasize how healthcare and medical imaging are different now and that this new context determines what we research and how we do it.
From its earliest development, medical image perception has been relevant to clinical care. This can be summarized in its work on misdiagnosis, performance measurement, and technology assessment. More recently, its ergonomic considerations for readers, aids to diagnosis, and the perceptual implication of new technologies have become important for an expanded and diverse area of diagnostic medicine. Current relevance of the human factors involved in medical image interpretation still, quite rightly, puts heavy emphasis on clinical care, but the economic dimension has gained importance and cannot be separated from this. Resource management constrains what can be achieved from finite budgets, so waste through avoidable error in one area reduces what can be allocated to care in another. This means that the penalties and costs of reader mistakes magnify the value of perception studies, even though the benefits to practice of these investigations may take a little time to become visible. Diagnostic error has traditionally had a lower profile than, say, medication error, perhaps because the immediacy of the effect is different. However, as the work of Graber (2013) has illustrated, the top alleged medical error insurance claims where the patient expired is reported as being attributable to faulty diagnosis (Figure 7.1).
Figure 7.1 Top alleged medical error named in claims where the patient expired (Physician Insurers Association of America (PIAA) Data Sharing Project Data 1985–2009, Physician Insurer, Vol 55, 2010).
Economic and clinical effects are not independent of each other on a national scale of expenditure. In the UK, 9.1% of the gross domestic product (GDP) was spent on total healthcare in 2014, of which radiology consumed ~0.1%, In the USA, in the same year, of the 17.1% GDP spent on total healthcare ~0.5% was in radiology (World Bank, 2016). There is a clear economic as well as clinical responsibility to apply all reasonable effort to ensure that error can be understood, reduced, and perhaps eliminated.
However, technical and organizational features of clinical imaging now show a distinct change from the way in which the specialty operated when human factors were first identified, and perception methodologies applied. The discipline emerged in response to questions that are still appropriate, but now exist in a different, more complex setting. Readers still search for and interpret image features and decide on their clinical significance. They use the same perceptual and cognitive activities but now carry out additional tasks using skills within that process that would have been unimaginable 50 years ago. The new tasks are mostly technical and are down to the way images are acquired and displayed. This has implications for the way that perception must be studied now compared with the past and it presents challenges to the community to choose research areas relevant to this new environment.
One of the most positive changes in the perception research setting is the way that clinical professionals and their governing bodies now welcome and seek out the expertise of image perception in studies designed to enhance or monitor their own performance. This is a welcome contrast to some experiences of the early workers in the field (Garland, 1949). Perception scientists always needed a good collaborative relationship with clinical colleagues to function effectively and bring about successful outcomes to problems frequently identified by the practitioners themselves. This is now an accepted norm and in a present-day context the discipline must continue with its fundamental, “pure” science activities but recognize the richness of clinical connections in identifying research questions in the real world of current diagnostic practice.
7.3 Performance Measurement
In a narrative review of diagnostic error in medicine Graber (2013) wrote:
The most fundamental principle of performance improvement is that “You can’t fix what you don’t measure.” Efforts to begin addressing diagnostic error must begin with measurement. In no area of patient safety is this need more acute than in trying to identify the true incidence of diagnostic errors, and the harm associated with these events.
Performance measurement has been central to activities and research investigations in perception and it remains perhaps the most important contribution that the discipline can make to radiology and pathology. The first published experimental work in the field was carried out by Birkelo and colleagues in 1947 and was an example of performance measurement comparing contemporary radiology techniques. Methods available at that time were rudimentary, but demonstrated that variance between readers was masking any possible differences between the image acquisition methods. The finding inspired the notion that optimization of performance for a radiology task can only be analyzed successfully if the variables are limited to the one of interest. Highlighting the human performance component of radiology led to a search for an ideal method of precision measurement in performance that continues, after many advances, over 70 years later.
These advances are important because they have made possible studies on innovative imaging methods that have a diagnostic gain over existing ones. In certain cases, these gains were clear and obvious, but marginal gains are more common and require rigorous measures to confirm their efficacy. Signal detection and receiver operating characteristic (ROC) methods quickly established themselves thanks to the work of Metz (1978), Dorfman, and others in the 1970s, and have become the method of choice for many observer studies, offering reliability and precision measurement. Some important modifications regarding location information were added (Chakraborty, 2013), giving greater power and versatility to the paradigm. A detailed description of the applications of signal detection and ROC methods is featured in a subsequent chapter in this handbook, but it is relevant to acknowledge here how refinements in statistical techniques for analyzing decision performance have helped perception research gain the confidence of clinicians and facilitated translation of research to practice. This is a continuing process and clinical usefulness remains a critically important motivator. That usefulness, however, must be well articulated so the results of studies are in a form that has the greatest meaning to the case manager and the patient population.
Mallet et al. (2012) commented that perception must always maintain a clear view of how data from studies should be interpreted to the best clinical advantage. They posed the following questions to this end that might be borne in mind by the perception researcher:
How does accuracy change with different diagnostic thresholds?
If sensitivity and specificity are compared for different conditions, they often change in opposite directions. Which is more important?
What are the clinical consequences of a false-negative or false-positive diagnosis? Can these risks be presented together expressing relative benefit?
What is the best way to include disease prevalence in the summary of clinical benefit?
Can results be presented in terms of what happens to individual patients, which are often the easiest for clinicians (and their patients) to understand?
7.4 Performance Guidelines from Professional Bodies
The metrics of performance from perception studies have been applied to audit and quality indicators used by the governing bodies for radiology and pathology, and have established working methods for practitioners to use as an integral part of professional life.
In their guidance to practitioners for the reduction of interpretive diagnostic error in surgical cytology and pathology, the College of American Pathologists published the outcomes of a systematic review of 137 articles that used methodologies common to many medical image perception studies in clinical settings (Nakhleh et al., 2016). These methods are predominantly those of reviews of diagnostic decisions with measured comparisons against a gold standard or degree of agreement measures and the kappa statistic. All articles in the review discussed the review of cases by one or more additional pathologist(s). Numerous studies demonstrated that the review of cases by a second pathologist detects disagreements and potential errors. The guidelines provided clear recommendations on how pathologists should develop procedures for the review of pathology cases to detect disagreements and potential interpretive errors, and so improve care. Consensus expert opinion of the reviews made it clear that the perceptual issue of interobserver variance was now fully understood as a potential source of error, and that it had some dependence on caseload experience. This conclusion from a very large study is familiar to many of those perception researchers who have carried out similar investigations in radiology.
Radiology has taken a similar approach to guidance to its practitioners on measures for error reduction, but has expressed a view in the USA that a more comprehensive approach is needed that identifies and separates personal and system errors. This was illustrated by a Radiological Society of North America (RSNA) Quality Initiatives teaching publication (Brook et al., 2010) that set out to describe a classification system for analyzing error in radiology, separating active and latent errors and highlighting the impact of error on practice. Its criticism was that the emphasis of perception studies to that date leaned heavily on human error to the exclusion of system processes. Schemes that focused only on perceptual (e.g., faulty search, display shortcomings) or cognitive errors (e.g., faulty reasoning, lack of knowledge) were incomplete, even though human error in radiology is highly important. That situation continues to change, and the perception scientist is now much more aware of the many predisposing factors that impact performance, such as management issues, understaffing, ergonomics, work volume, and fatigue. These factors demand higher-order research questions, but they are tractable and can be assessed through carefully designed observer studies that are very clearly of present interest to the perception community. Precise measures of performance in real-world settings with typical risk of error, or in simulations of conditions that are now commonplace, embrace the full range of errors referred to by Brook et al. Chapters in this book demonstrate the current approaches to the origin, measurement, and nature of error and its reduction.
The Royal College of Radiologists in its guidelines on standards of reporting has considered an additional feature of performance measurement relevant to the UK (Royal College of Radiologists, 2006). This considers the fact that nonmedically qualified healthcare professionals deliver some radiological services. It is now a well-established and successful practice that makes more effective and economic use of the skills of healthcare practitioners. Perception research was an important element in the decision by the Royal College of Radiologists to permit the fundamental change in the way radiology is practiced. The change required at its outset very rigorous training and measurement of performance for the establishment of clear boundary limits to the type of diagnostic decision nonmedical professionals can take. Comparisons between the performance of radiologists and other readers had to be extensive, so innovative methods to accelerate or monitor learning were encouraged, and opportunities taken to ask fundamental questions regarding the behavior of experts compared with learners for some typical tasks. Examples of these studies included eye-tracking comparisons (Figure 7.2) of experts and students carrying out image reading to seek a better understanding of how experts achieve their diagnostic performance (Donovan et al., 2008).
Figure 7.2 An eye-tracking study comparing the search characteristics and visual fixations for two lung lesions between an expert (left) and a student reader learning the task. The lesions are each marked x. Note that the fixations indicated by the discs are fewer and more effective in detecting the two lesions for the expert. The expert’s strategy could used for teaching purposes by showing the findings to the learner.
It continues to be a valuable study of the development of expertise and has been used to guide studies in many other imaging settings, including mammographic images displayed on large dual clinical monitors where complex activities like zooming and panning are performed by expert readers (Dong et al., 2016).
The initiatives from professional bodies governing the diagnostic decision makers in pathology and radiology are an example of how methods developed in the perception discipline, sometimes in a laboratory setting, find application in directing techniques for improving diagnostic outcomes and care in the clinic.
7.5 Perception and Image Quality
In discussing the question of image quality and its optimization we can learn how important mixed disciplines are by considering how perception has influenced the thinking in this area over time.
7.5.1 Defining and Measuring Image Quality
Perception is important to the concept of image quality because of an association between the fidelity of the displayed image and the diagnostic performance that comes from its interpretation. This is captured in comments often made by radiologists on the quality of an image that it must be “diagnostic” for the clinical question. It is a way of stating that if the necessary image features are made perceptually salient, detection would not only be possible, but likely, and an accurate diagnostic decision would result. This takes no account of the cognitive processes after detection essential for accurate decisions, but the sense of the idea is clear.
Originally the image quality–diagnosis association was not fully recognized as containing perceptual components, and introducing the observer into the system was felt to add subjectivity that made measurements unreliable.
In 1970, Rossmann and Wiley discussed image quality and diagnostic performance in the context of physical criteria that could be used to characterize images. Examples of these metrics (i.e., point spread function, line spread function, and modulation transfer function) were well known to the medical physics community then and now. However, they rarely considered the observer or the task to be performed using the images. It was clear that an objective study of the transfer of diagnostically important information from source to image could not give the complete story. Results could only be applied meaningfully to improvements in imaging techniques when their effect on diagnostic outcomes was fully understood. This was very insightful and still resonates for the perception and physics community in imaging. Our understanding is now much better but not yet complete.
Perception science made very significant contributions to both sides of the problem and gradually the understanding gap narrowed (Chesters, 1992). From measurements of the performance of the human visual system (such as its modulation transfer function) and work to determine the contrast threshold for the detectability of a signal (Barten, 1999), a range of test objects were developed. Barrett and Myers (2003) emphasized the importance of objective task-based metrics in image quality assessment and contributed a great deal to an understanding of the value of principles, mathematics, and statistics needed to evaluate imaging systems, with the use of mathematical model observers (e.g., ideal observer models). Metrics such as noise power spectrum and signal-to-noise ratio have been shown to influence the perception of some types of image features by these model observers, foretelling the contribution that image quality may well play in observer error.
7.5.2 Introducing Observers: Toward Realism
The test object sets (Figure 7.3) and the approaches now available thanks to this fundamental work have widespread use and sets of test objects have been designed for digital planar and tomosynthesis methods, computed tomography (CT) X-ray systems, ultrasound, magnetic resonance imaging, and nuclear medicine.
Figure 7.3 A typical contrast/detail test object that can be used to give a quantified measure of the image quality of one system versus another. It makes no attempt to simulate a clinical medical image but gives a valid and objective measure of detectability by the human visual system.
Test objects are valuable in routine quality control and standard comparisons made over time or between pieces of equipment in the image train, but they fall short of providing a comprehensive measure of image quality relevant to diagnostic performance. Radiologists do not see image quality metrics, they see features that inform a specific diagnostic task (Kundel, 1979). Thus, we must accept two measures of image quality: one is technical and the other is diagnostic. These are not impossibly separate if an approach is taken to quantify the features in an image that have direct relevance to the clinical question. Work in this area includes visual grading analysis where normal regions of displayed anatomy are scored for clarity, and attempts to quantify the salience of image features demonstrating pathology, as shown in Figure 7.4 (Szczepura and Manning, 2016). This method is suitable for all digital medical image modalities, but only works for discrete, focal features rather than diffuse disease patterns.
Figure 7.4 (a) The visual conspicuity, or salience, of the focal lesion shown in part of a chest image has been quantified by measuring its sharpness, contrast, size, and signal-to-noise ratio with its background by many profile samples through its image. (b) One of these profiles demonstrates how the values combine to allow calculation of an index for the lesion for this image. (b) Reproduced from Szczepura and Manning (2016), with permission of SPIE.)
Generally, approaches like this align with current thinking about image quality in the context of perception: its value in reducing diagnostic uncertainty is best defined in respect of the clinical question it was intended to inform. It demands realistic tasks for observers in studies that pose real-world questions to measure performance in decision making.
This philosophy has increased interest in the design of observer studies using real cases. This is by far the most rigorous method of addressing questions of performance by perception techniques and now large studies are carried out in some areas of radiology (such as breast screening programs, as explained in two other chapters), where standardized test sets of clinical images are used in reader performance monitoring. Case-based studies are, however, expensive in time and resources and have ethical aspects that often make them impractical or simply unacceptable. Model observers have a long and well-established position in image perception research. They are fast, convenient, and give valuable insight into optimum performance by considering an ideal observer. They lack the realism of human observers, but act as surrogates for them. Observer models are considered in detail in later chapters where their value and contribution are expanded on.
A compromise position is to use anthropomorphic phantoms (Figure 7.5). These objects are expensive to manufacture and have limited diversity compared with human populations, but they have developed into sophisticated simulations for imaging. They can be irradiated without the concern of ethical considerations required for patient groups and have the advantage to the experimenter of knowing “truth” regarding disease features. Their careful design allows variation in tasks presented to observers in studies made to test, for example, image acquisition procedures for optimization.
Figure 7.5 An anthropomorphic phantom (top) and a computed tomography image (bottom) showing its realistic simulation of lung fields and vessels. Many images can be produced without radiation dose detriment and the anatomy can be varied to simulate disease features.
7.6 Optimization and Perception
Optimization is a now dominant feature of quality testing. It is seen in the activities needed to produce the required diagnostic performance consistent with economy of input (e.g., radiation dose, time, computational expenditure). It is typically investigated when some change has been introduced and the effect this might have on readers, or the throughput of case reading in clinical areas. When image quality changes, it is important to assess its impact on perception to optimize the process and minimize error (Krupinski, 2010). The focus of optimization has generally been on the effect of changes to image displays, image acquisition (particularly CT reconstructions), pre- and postprocessing, and reading environment on image quality and accuracy.
7.6.1 Viewing Conditions: Then and Now
During the change in technology from film to digital displays, questions regarding the influence of display properties on diagnostic accuracy led to investigations of the ideal physical settings for diagnostic performance (Figure 7.6). Prior to the introduction of digital displays, viewing hard-copy film required control of just two factors: the stability/uniformity of output of viewing lightboxes and the ambient light levels of the reading room. If a clinician read films at a patient’s bedside, the poorly controlled lighting conditions compared with reading rooms made reliable interpretation rather unlikely. For the readers, no postprocessing or image manipulation was possible so the activity of film viewing included no means of changing image contrast or optical density (other than occasional use of a “bright light”). At the time, this presented some practical perceptual issues for optimization, but film viewing was a very simple technical activity and had few components in a descriptive task analysis.
Figure 7.6 Left: An early form of viewing a fluoroscopic X-ray image. Perceptual issues were less important than the risks from radiation exposure to the unprotected reader. (Reproduced from Flickr’s The Commons.) Center: Hard-copy film viewing on light boxes. This was the standard practice from the 1920s to 2000s. (Image by Nevit Dilmen on Wikimedia (GNU free documentation license) http://medicalchemy-radiology.blogspot.co.uk/2010/11/radiologist-reading-films-at-light-box.html accessed November 1, 2017.) Right: A typical digital radiology reading room suite.
In present-day soft-copy viewing, by comparison, variable display luminance and postprocessing activities by readers all now come under scrutiny to determine the best way of ensuring consistent accuracy. Reading rooms now have carefully controlled environments for optimized viewing but readers must deal with multiple digital displays and their veiling glare, complex multiple-section image data sets per case, and new forms of control for distributed viewing.
Additional forms of viewing, including handheld devices at the disposal of readers, are available to be used in any reasonable setting. This technical possibility has found application for orthopedic injury and intracranial hemorrhage, commonly encountered in emergency radiology, where accurate and timely diagnosis is important. Through teleconsultation, relevant images can now be transmitted electronically to a distant clinical expert. Comparisons between the handheld device and high-end reading room displays would be unreasonable, but it is fair to assume that if a mobile device could perform as well as a secondary form of display (2°D) of the type used in emergency treatment rooms, this would possibly give acceptable results.
For example, Toomey et al. (2009) set out to determine whether the diagnostic accuracy of handheld computing devices is comparable to displays that might be used in this type of emergency teleconsultation. The diagnostic efficacy of two handheld devices was tested against 2°D for each of two image types, wrist fracture radiographs and slices from CT of the brain, yielding four separate observer performance studies. For the CT brain study, the scores of personal digital assistant (PDA) readings were significantly higher than those of 2°D readings for all observers, and for radiologists who were not neuroradiology specialists. No statistically significant differences between the handheld device and 2°D findings were found for the PDA wrist images or in the iPod Touch device studies. Conclusions were that handheld devices can be used successfully in emergency teleconsultation for the basic tasks used in the study.
The technology of viewing and display devices changes rapidly and poses questions regarding the efficacy of last year’s purchase compared with more recent arrivals. Perception approaches may help sensible choices to be made in respect of achieving better matching of device performance to the capacity of the human visual system. The bit depth of displays has been questioned for the ideal capacity for diagnostic purposes. Medical-grade monitors generally display 8 bits (256 gray levels) of data, which is sufficient for interpretation tasks where the acquired data are 8 bits or less. However, many medical images are acquired at depths of 12–16 bits, or 4096–6553 gray levels. It is possible to lose information in the interpretation process if window/level is not utilized, and possible artifacts can be generated if images are down-sampled to 8-bit depth. Higher bit depth displays may improve image display fidelity, but the human visual system can only detect about 1000 gray levels (not 4000–6500) at the luminance levels used in medical-grade monitors. Thus, displaying more gray levels may not be useful (Barten, 1992, 1999). Whether this is important has been questioned by Krupinski and Kallergi (2007), who found there were no statistically significant differences between the 8-bit and 11-bit displays for any of three systems tested.
In commenting on perceptual implications of digital reading environments, Krupinski (2010) cites a study by Saunders and colleagues (2007) who examined the effects of different resolution and noise levels on task performance in digital mammography. Observer-based perception research like this can sometimes give answers that are not uniformly consistent with changes to technical figures of merit of image quality. In this case, there was a measurable perception difference between the effects of resolution and noise for the specified task.
Results with radiologist readers showed that, after reducing resolution for clinical images from a database at three different levels and manipulating noise to simulate levels for full clinical dose, half dose, and quarter dose, there was little effect on classification accuracy and diagnostic task performance for the changed resolution. Increasing noise caused classification accuracy to decrease significantly as the radiation dose to the breast was reduced to one-quarter of its normal clinical value. These noise effects were most prominent for microcalcification detection and mass discrimination. Conclusions suggested that quantum noise is the dominant image quality factor in digital mammography for these features, affecting radiologist performance much more seriously than display resolution. Changes in noise values are perceptible and this affects interpretation.
The result provided clear guidance in two respects for digital mammography: first, the resolution requirements of the display devices is not as critical as might be assumed; but second, the possibility of maintaining diagnostic performance in this task at a reduced radiation dose to the patient at the point of image acquisition appears to be very low.
7.7 Optimization of Image Acquisition
Perception methods have played a role in determining performance variance if image acquisition is changed for some reason, whether technical or otherwise. With radiation dose factors now a primary consideration in optimization, it is important to re-evaluate methods that have previously shown little or no difference in their diagnostic properties because one may have a dose advantage.
In CT imaging, for example, suspected but marginal effects on diagnostic performance due to image quality differences were studied between reconstruction with adaptive iterative dose reduction 3D (AIDR3D) and filtered back-projection (FBP) (Thompson et al., 2016). The study evaluated chest phantom nodule detection in images reconstructed with AIDR3D and FBP over a range of mAs values and found no statistically significant difference in nodule detection from the two image sets with either FBP or AIDR3D.
However, the level of image noise was higher in images reconstructed with FBP. This disparity between image noise, a physical measure, and nodule detection, an observer performance measure, was an important finding. The significant effect on physical measures between algorithms, but insignificant effect in objective observer performance, was demonstrated because the free-response measure used considered all factors affecting detection-rate, including visual search. The physical measures focus on a few individual parameters in isolation and disregard search. In any event, it seems that the tolerance of the human visual system permits perception of the target nodules despite a degraded, noisy image. It supports the idea that optimal image quality for some tasks can be achieved at a lower radiation cost than that expected from metrics such as signal-to-noise ratio. This must be confirmed by experimentation and then validated for the specific diagnostic task used.
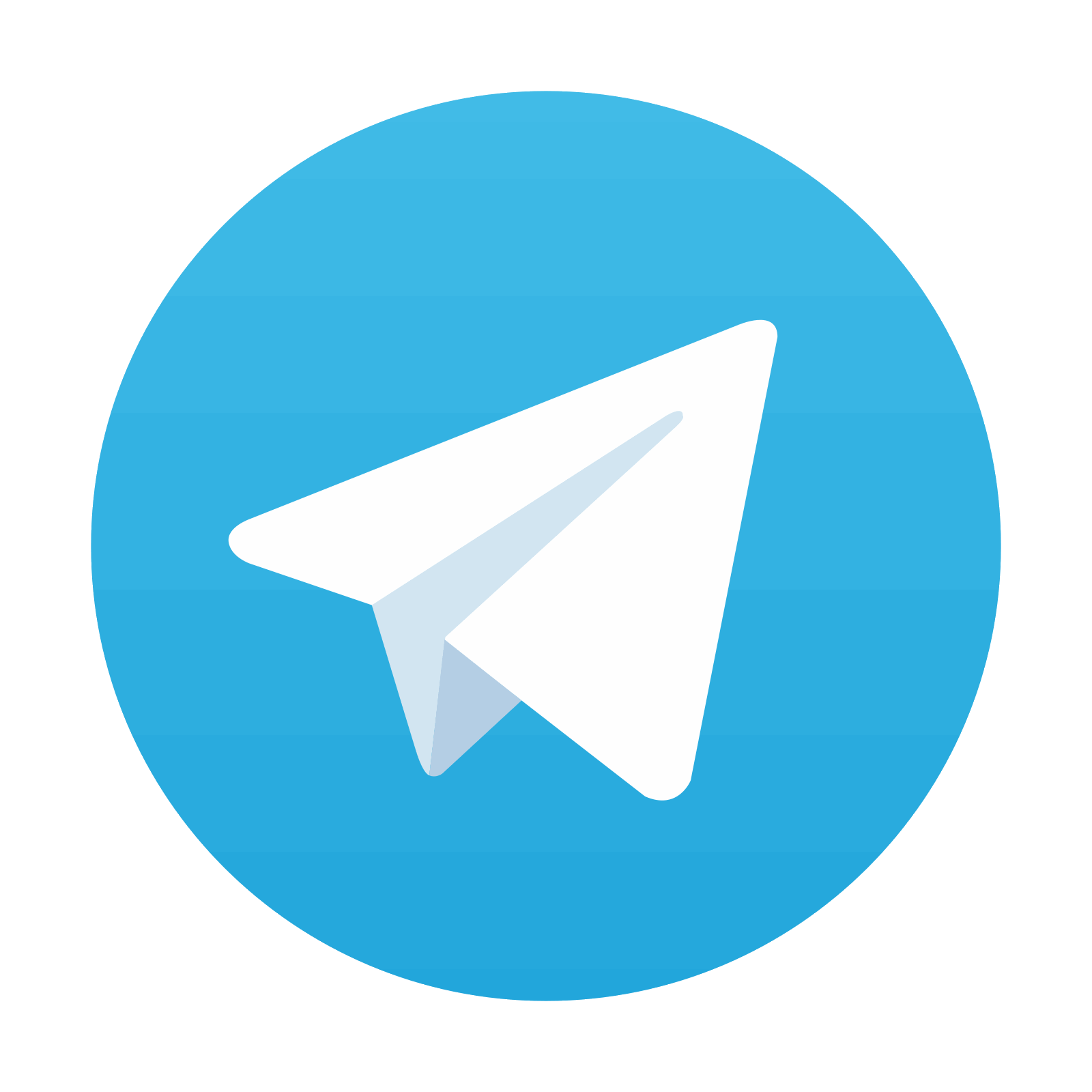
Stay updated, free articles. Join our Telegram channel

Full access? Get Clinical Tree
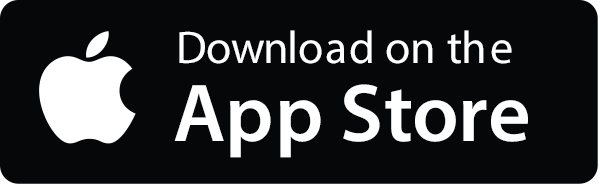
