Artificial intelligence (AI) technology shows promise in breast imaging to improve both interpretive and noninterpretive tasks. AI-based screening triage may help identify normal examinations and AI-based computer-aided detection (AI-CAD) may increase cancer detection and reduce false positives. Risk assessment, quality assurance, and other workflow tasks may also be streamlined. AI adoption will depend on robust evidence of improved quality, increased efficiency, and cost-effectiveness. Reliance on AI will likely proceed through stages and will involve careful attention to its limitations to prevent overconfidence in its application.
Key points
- •
The use of AI in radiology has great potential to improve patient safety and clinical outcomes.
- •
Breast imaging is an attractive target for AI given the relevant clinical problem, the algorithmic nature of the workflow, the narrow focus of the disease process, and the reliance on imaging data.
- •
Although image analysis and interpretation will be useful, noninterpretive tasks will also benefit from AI technology.
- •
Leveraging the strengths of both human and machine will likely produce the best value, but only if the AI tools are adequately validated with prospective studies and designed with human interaction and workflow in mind.
Introduction
Artificial intelligence (AI) is a cross-disciplinary field in which computer scientists, mathematicians, and engineers work to create algorithms that “learn” to take complex inputs and deliver outputs faster and more accurately than the human mind. Although some AI scientists imagine creating the most humanlike machine possible (general AI), most recent work has focused on areas in which AI can perform a specific task (narrow AI).
The recent availability of large digital datasets (big data) combined with improved hardware (graphical processing units or GPUs) has made AI applications into a practical reality. One of the key approaches powered by these advances is machine learning (ML). Whereas early AI consisted of distilling human thought and behavior into discrete steps that can be programmed as clear instructions, ML uses statistical models to allow computer systems to learn and improve without explicit programming.
In the past few years, AI has propelled into the public consciousness and common usage, spreading dramatically into all facets of our lives, including security surveillance (facial recognition), personal assistants (Siri, Alexa), self-driving cars (Google, Uber), financial bots (high-frequency trading, fraud detection), and countless others.
An intense interest in the use of AI in image recognition began in approximately 2009 when a massive dataset was created (ImageNet) and researchers competed in various challenges to evaluate algorithms designed for large-scale object detection and image classification. These successes led to an explosion in the interest of using these techniques to facilitate medical image diagnosis.
ML systems may use imaging features not evident to human perception. This raises the exciting possibility that AI could someday not only make imaging more efficient, but achieve quality improvements such as more reliable diagnoses than what is possible by humans alone.
Breast imaging, with its focus on a single diagnosis determined almost exclusively by imaging, represents an ideal use-case for AI efforts in radiology. Screening mammography is particularly well-suited to development of AI applications given the standardized views, comparison images, and robust outcome data, all of which support the training of AI systems.
Breast Cancer Screening
The strengths and limitations of screening mammography will be familiar to breast imagers. Screening mammography is one of the most successful tools for early breast cancer detection and has been shown to decrease mortality. Despite its relative success, screening mammography is still far from perfect, with up to 13% of cancers missed at time of interpretation. Approximately 10% of women who undergo breast screening each year are recalled for additional diagnostic imaging, whereas only a small fraction (∼5%) of these women are eventually diagnosed with breast cancer. The high rate of false positive screening recalls may lead to patient anxiety and unnecessary biopsies, and add to annual mammography screening costs. If AI technology could help to reduce false positives and simultaneously increase cancer detection rates, it would be a significant improvement to the breast cancer screening process.
Computer-Aided Detection
Computer-aided detection (CAD) is a generic term that refers to the approach of using computers to assist the radiologist to identify a potentially significant finding on an image. The idea of using computers to aid in the mammographic detection process is not new. From the late 1980s through the late 1990s, significant efforts were made to use quantitative analysis of mammography images to identify suspicious lesions such as clustered microcalcifications, masses, and architectural distortions. ,
In 1998, The Food and Drug Administration (FDA) approved the first CAD technology (ImageChecker; Hologic, Bedford, MA) for mammography. When the Centers for Medicare and Medicaid Services (CMS) provided increased payment for its use in 2002, CAD was widely adopted by the breast imaging community, and by 2012 its use in screening exceeded 80%.
However, despite the early promise of improved performance, subsequent research showed that the use of this type of CAD was associated with decreased accuracy and increased biopsy rate. , Although it continues to be a feature in many mammography systems, early CAD is viewed by many as a failure. , The reason is that early CAD algorithms suffer from a high false positive rate. To put this in perspective, the average number of CAD marks per full examination ranges from 1.5 to 5.1. Assuming 2 marks per examination and added detection of 0.1 to 1 cancer per 1000 examinations, this translates to 1 in 2000 to 20,000 marks as true positives. With this high rate of “false alarms,” it is likely that radiologists have learned to largely ignore the CAD markings.
This early version of CAD used human-driven, rule-based mathematical models to determine the presence of abnormal imaging features and could not subsequently improve with additional inputs. Recent advances in ML have opened up a new line of techniques for CAD that offer advantages over prior approaches, thereby creating an opportunity to revisit the idea of CAD for mammography as well as other breast imaging modalities.
In this article, we first briefly review the breast imaging workflow to illustrate the many decision points and show where AI technologies may be applied. We then move to a discussion of the value proposition of AI in breast imaging, its evaluation and limitations, and plausible near-term applications. The intent is to point industry toward the most fruitful use-cases and to provide a framework for breast imagers who are looking to adopt AI into their practice.
Breast imaging workflow
Breast imaging workflow consists of multiple steps, which can be subdivided into 4 stages: prescreening, screening, diagnostic, and management ( Fig. 1 ). At each stage, there are decision points that guide the next steps in the workflow. A careful understanding of these decision points can serve as a roadmap for how AI technology could be applied to the breast imaging process.
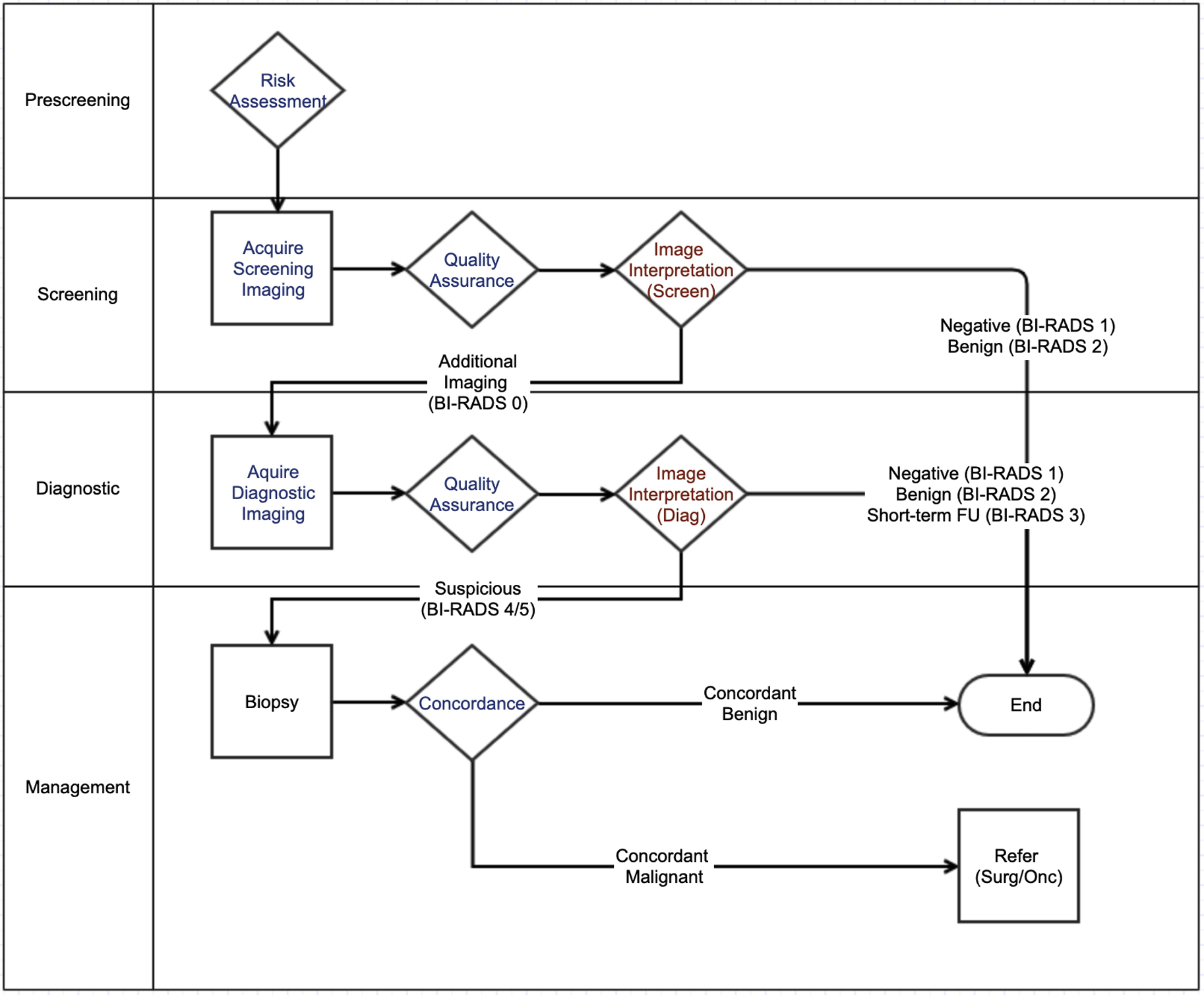
Applications of artificial intelligence
We have summarized the opportunities for AI enhancement of breast imaging in Table 1 .
Interpretive AI | Noninterpretive AI |
---|---|
|
|
Interpretive Artificial Intelligence
Screening triage
Radiologists review screening mammograms looking for signs of cancer, including masses, architectural distortions, and microcalcifications. This process is essentially a triage to decide if the patient requires further investigation and has 2 basic outcomes: “normal” (Breast Imaging-Reporting and Data System [BI-RADS] 1 & 2) and “possibly abnormal” (BI-RADS 0). Although this seems straightforward, there is high variability in reader performance with high rates of false positive recalls and a significant number of false negatives (ie, missed cancers). AI technology could improve the screening process in several ways.
Although there is much excitement in proving that a computer could reliably detect cancer, another more pragmatic function could be to reliably identify the negative and benign cases. Recent research has shown that differentiating “normal” exams (BI-RADS 1 & 2) from the “possibly abnormal” exams (BI-RADS 0) could reduce the overall number of cases that require human review by 15% to 20%. These normal results could be shared immediately, which would decrease overall anxiety for patients awaiting test results. ,
Screening AI could decrease reader variance by helping radiologists with different levels of training and experience achieve more uniform performance.
AI systems could also serve as stand-alone second readers in screening programs in which double reads are performed. ,
Although much attention is focused on 2-dimensional screening mammography, other screening modalities such as tomosynthesis, ultrasound, and MR imaging would also benefit from AI development. Because these use more images per study than mammography, the use of AI could potentially result in efficiency gains with equal or improved quality.
Artificial intelligence–based computer-aided detection
After triage, AI could be leveraged in screening to help detect areas of concern (AI-based CAD). This is where the bulk of current AI research is focused, and numerous recent studies have shown promising results. The combination of human review with AI-based CAD would be synergistic for making final determinations on examination recalls, which should simultaneously decrease recall rates while improving cancer detection rates.
At the diagnostic imaging stage, the imaging task shifts from lesion detection to lesion characterization and risk stratification for potential biopsy. Additional mammographic views and/or ultrasound are used to isolate the abnormality and allow for detailed feature analysis. AI in this setting would become a tool to analyze the region of interest identified by radiologists to characterize its features. This would increase overall accuracy (positive predictive value [PPV] of biopsy) and decrease intraobserver and interobserver variation by decreasing the impact of differences in training and experience. AI could also be used as a “second reader” to help validate radiologists’ impressions. ,
More advanced uses might include detecting features not visible to humans and advanced statistical analysis. Research is under way to predict cancer based on enhancement features, predict cancer subtypes and genomics, predict response to treatment, and assess lymph nodes for signs of spread.
Noninterpretive Artificial Intelligence
Although much of the focus of AI in mammography has been on image analysis and interpretation, there are many noninterpretive aspects of the breast cancer workflow that would benefit from AI. ,
Risk assessment
Before any imaging takes place, the first step in the cancer screening process should be to understand a woman’s individual cancer risk. Accurate risk analysis can then help guide the initiation of screening, frequency, and optimal modality(ies).
Without risk analysis, mammography screening guidelines necessarily lump all women together. This overgeneralization has produced differing recommendations of when to begin screening and at what frequency, with the resultant controversy creating a confusing message for women and their primary care providers.
To help improve screening personalization, several breast cancer risk models have incorporated patient-specific characteristics such as age, family history, and hormonal factors to refine breast cancer risk estimation. Mammographic breast density was recently added to risk models, and legislation in many US states requires that women be notified of their breast density with the well-meaning intent of informing them about the decreased sensitivity of mammography in dense breasts and helping them understand the impact on breast cancer risk. However, density assessment suffers from significant interreader variability, which may cause confusion if the density is assessed differently year to year. , Moreover, the focus on density may result in overemphasis of a single factor in risk, rather than improving overall risk estimation.
A recent study has shown that AI analysis of mammography (without including traditional risk factors) can significantly improve breast cancer risk prediction, which would be especially helpful when family history is unknown. , The same research shows that when AI image analysis is combined with traditional risk model information, performance increases further still. Such improvements would allow each woman to have a personalized and data-driven approach to breast screening, with screening commencement and frequency adjusted accordingly. This approach could help to mitigate the controversy from overgeneralized, age-based screening recommendations.
Image controls
Applying AI in the image acquisition process has the potential to improve patient safety, image quality, process efficiency, and technologist performance.
Dose reduction
The ALARA principle (“As Low As Reasonably Achievable”) is familiar to radiologists. Radiation dose is especially relevant to a screening examination that is repeated over many years. One of the major potential benefits of AI in breast imaging would be to reduce the dose without compromising image quality. Novel techniques are being tested that could maintain high image quality while dose is reduced by up to 50%. ,
Quality assurance
Because the features of cancer on imaging may be subtle, high-quality imaging is critical. In the United States, rigorous imaging standards are required by the Mammography Quality Standards Act and the American College of Radiology. , Image evaluation includes an assessment of positioning, compression, artifacts, exposure, contrast, sharpness, noise, and labeling.
In the current mammography workflow, a radiologist makes a subjective assessment of image quality at the time of interpretation. If it does not meet quality standards, a “technical recall” is issued and the patient must return for inconvenient and wasteful repeat imaging. From a Lean manufacturing principles standpoint, quality problems should be detected and corrected at the time of acquisition or prevented altogether.
ML algorithms that automatically assess the image quality could be built into the image acquisition process. This way, blur and/or positioning problems can be detected while the patient is still in the room and can be re-imaged with minimal increased effort.
AI applied to mammography quality assurance would reduce or even eliminate technical recalls and improve technologist performance by giving just-in-time feedback. It could also facilitate accreditation by automatically identifying high-quality images. Moreover, AI could assess overall system performance by retrospective image review to identify potential quality improvement initiatives.
Radiologic-pathologic correlation (and beyond)
After a biopsy, breast imagers review the pathologic result and compare it with the imaging appearance to determine “radiology-pathology correlation.” This is a manual process and is subject to significant variability in decisions and management, especially when results include “high-risk” pathologies. AI could standardize the decision making by incorporating quantitative imaging features, semantic features of the radiology and pathology reports, histopathologic slide imaging features, as well as patient risk factors.
Moreover, there is growing evidence that imaging has potential use in understanding genomic properties of disease. Radiomics is an approach that extracts features from radiographic images using data-characterization algorithms, which may uncover disease characteristics humans are unable to perceive. Radiogenomics refers to the relationship between the digital pattern in disease imaging (imaging phenotype), and its genetic expression, including gene mutations and other genome-related characteristics. The combination of radiogenomics with AI tools could provide a powerful approach for uncovering radiologic-pathologic-genomic relationships and increasing prognostic potential.
Workflow optimization
In emergency radiology, AI is being used to help prioritize studies with urgent positive findings. such as intracranial hemorrhage, pulmonary embolism, spinal fracture, and others. , This new approach to organizing and prioritizing examinations could be leveraged in breast imaging as well. AI-identified normal examinations could be overread in an expedited fashion (or given an AI-only reading) to give immediate results. Examinations flagged with probable abnormalities could be prioritized for immediate diagnostic evaluation, or they could be concentrated for review at the beginning of the day when radiologist attention is fresh and before image fatigue. Examinations with very high probability cancers could automatically trigger workflows that could alert care coordinators and preschedule a diagnostic examination, biopsy appointment, and prompt surgical consultation.
Discussion
Integrating Artificial Intelligence
Humans and machines have different strengths; the goal of AI in health care should be to leverage the advantages of both.
Some tasks are better suited for computers. Machines never get tired. They produce consistent and repeatable results. They can “see” what humans cannot, analyzing data beyond the limits of human perception and at remarkable speed. Machines excel at pattern recognition, even those involving large volumes of data with complex associations, and can therefore provide improved predictive power.
Humans, on the other hand, can synthesize disparate points of data. We can make inferences from minimal data and solve a wide variety of problems. Humans can incorporate context, adapt to regional practice patterns or other idiosyncratic situations, build working relationships, and clearly communicate with referring clinicians and patients. Humans can extract value and meaning from the vast store of unstructured and messy information that is the current state of medical data.
In breast imaging, there are numerous points of correlation, judgment, procedural skill, assessment, and communication with both patient and referring clinician. Clearly, AI is not a replacement for these high-level, highly nuanced tasks. Although the introduction of AI has often been cast as a struggle between humans and machines, the true power of AI will be realized when it is incorporated in a way that harmonizes the skill of humans with the power of technology using an interface that optimizes that synthesis and allows for iterative feedback. Lack of this harmonization and feedback is likely one of the reasons why traditional CAD failed to meet expectations.
As we consider how to integrate AI into practice, it may be instructive to compare with other industries. For example, the automotive industry has identified 6 levels of automation to describe the process of integrating AI into the driving process. In breast imaging, we might use a similar paradigm to understand when and how to bring AI into the process ( Fig. 2 ).
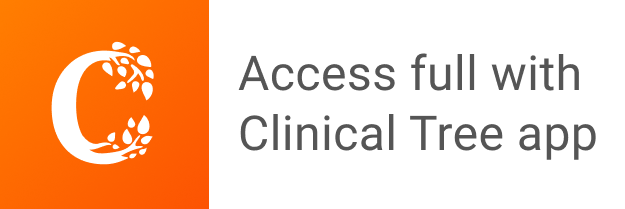