Artificial intelligence (AI) can provide significant utility in the management of hip disorders by analyzing MR images. AI can automate image segmentation with success. Current models have been successfully tested in the diagnosis of osteoarthritis, femoroacetabular impingement, labral tears, developmental dysplasia of the hip, infection, osteonecrosis of the femoral head, and bone tumors. Many of these models have shown strong performances with accuracies in the range of 76% to 97%, and area under the curve of 77% to 98%. The recent trends indicate high interest and adoption of these tools in MR imaging assessment of hip disorders.
Key points
- •
Artificial intelligence has the potential to facilitate the management of hip disorders by aiding in the interpretation of magnetic resonance images.
- •
Image segmentation can be automated with artificial intelligence with results comparable to manual segmentation.
- •
Artificial intelligence models show strong performance with high accuracy and area under the receiver operating characteristic curve values.
Introduction
Hip pathologies are a common cause of pain and disability. Diagnosing the underlying cause of symptoms is not always possible with clinical examination and plain radiography and requires advanced imaging. MR imaging provides valuable diagnostic information by creating excellent soft tissue contrast, allowing the assessment of the cartilage, ligaments, synovium, labrum, muscles, and tendons in addition to the osseous structures.
Artificial intelligence (AI) refers to automated computer algorithms that are developed to perform tasks that typically require human intelligence. AI has rapidly advanced, including in many medical applications. AI algorithms can aid physicians in patient care including in diagnosis, prognostication, and clinical decision-making. In radiology, AI can diagnose and characterize disease, with performance on certain tasks on par or better than trained radiologists. Radiomics refers to the computation analysis of radiological images and is widely used for feature extraction for the development of machine learning (ML) algorithms. Analyzing image characteristics that cannot be analyzed by human readers has the potential to allow AI algorithms more accurate diagnostic capabilities.
Developing an ML model involves a stepwise workflow of data collection and labeling of ground truth, data preprocessing and feature extraction, model selection, model training, and validation and testing of the final model, occasionally on an external dataset. Model architecture is selected based on desired task (eg, segmentation or classification), computational complexity and requirements, training data size, and other factors. Models range from lower complexity algorithmic approaches such as support vector machines (SVMs) and gradient boosting, to deep learning approaches, including convolutional neural networks (CNNs) and more recently transformers. Numerous metrics can be used to determine overall model performance on the test data, where the definition of model success and the appropriate metric to use depends on the task. Common performance metrics include receiver operating characteristic (ROC) curves and area under the curve (AUC), overall accuracy, sensitivity, specificity, and positive predictive value (PPV) and negative predictive value (NPV). Dice score, used to score image segmentation performance, is a measure of pixel overlap between predicted segmentations and ground truth segmentations with higher values indicating increased overlap.
AI applications have great utility in the diagnosis of hip pathologies. AI segmentation can recognize the anatomic structures that constitute the hip from the neighboring anatomic areas. This ability can be leveraged to differentiate between distinct anatomic components of the hip (cortex, cartilage, labrum, joint capsule, and surrounding soft tissues), allowing the delineation of bone morphology, characterization of bone marrow signals, and measurement of the joint fluid volume. Classification models can diagnose certain pathologic conditions such as congenital, degenerative, infectious, metabolic, and neoplastic processes. Numerous diseases could benefit from the integration of AI into the diagnostic algorithms, such as osteoarthritis (OA), femoroacetabular impingement (FAI), labral tears, developmental dysplasia of the hip (DDH), prosthetic joint infection, femoral head osteonecrosis, and bone tumors.
We aimed to review the current literature regarding the application of AI and ML in MR imaging of the hip to investigate and highlight its utility, scope, effectiveness, and potential drawbacks in the segmentation and diagnosis of a variety of conditions.
Segmentation
Image segmentation is the process of splitting a digital image into various components for further processing and use. AI has shown significant ability for segmentation of radiologic images, automatically labeling anatomic structures and/or pathology on a pixel level. Automated hip MR imaging segmentation primarily involves segmentation of the proximal femur for the characterization of anatomy and morphology, achieving high accuracy generally using CNN architectures. Other anatomic structures in or adjacent to the hip can be automatically segmented as well. Meier and colleagues successfully applied CNNs for the segmentation of hip cartilage and labrum from hip MR arthrography and reported Dice coefficients of 0.89 ± 0.02 (0.88–0.90) and 0.71 ± 0.04 (0.69–0.73), respectively. Enabling automatic segmentation in a matter of minutes could substantially increase the productivity of radiologists, thereby improving MR imaging turnover rates.
Radiomic analysis of automatically segmented anatomy or pathology can also benefit disease diagnosis and characterization. Fischer and colleagues utilized a deep learning-based model for fully automated segmentation of the proximal femur and visualized pelvic bones from hip MR imaging studies and subsequently derived numerous morphologic measurements from the segmentation data including the centrum–collum–diaphyseal angle, center-edge angle, alpha angle, head-neck offset (HNO), and HNO ratio along with the acetabular depth, inclination, and anteversion, with high similarity to manual assessments by radiologists. In a retrospective study using 31 hips from 26 symptomatic patients with hip dysplasia or FAI, deep learning was used to automate segmentation of three-dimensional (3D) MR imaging-based models achieving Dice coefficients of the proximal femur and acetabulum of 98% and 97% accuracy, respectively, when compared with manual MR imaging-based models. These studies collectively underscore the transformative impact of AI in the segmentation of hip anatomy on MR imaging, offering innovative solutions that enhance diagnostic accuracy, efficiency, and the overall utility of radiomics in the management of hip disorders.
Osteoarthritis of the hip
OA of the hip, characterized as a degenerative joint disease, affects over 27 million US citizens annually. The most common imaging modality for hip OA diagnosis is direct radiography, which detects features such as joint space narrowing and osteophyte formation. However, hip OA poses diagnostic challenges, particularly in the early stages, as standard radiography is often unable to illustrate internal derangement of the hip joint and signs of early cartilage degeneration. On the other hand, MR imaging is emerging as an important tool to evaluate joint pathologies because of its ability to provide detailed images of bones and soft tissues but faces challenges due to greater time of image acquisition and interpretation, limited expert readers, and associated diagnostic variability.
Recent developments of AI in medical imaging show promise in addressing challenges related to musculoskeletal imaging by potentially improving diagnostic efficiency, accuracy, and consistency. For instance, deep learning has been able to classify various joint pathologies in imaging such as cartilage lesions, fractures, meniscal tears, and chondrocyte patterns, as well as predict the risk of future OA. In a retrospective study evaluating 764 MR imaging hip volumes from 364 patients for automatic binary classification of cartilage lesions, bone marrow edema-like lesions, and subchondral cyst-like lesions, a deep learning model was able to achieve AUCs that beat the results of experienced radiologists ( Fig. 1 ). For cartilage lesions, bone marrow edema-like lesions, and subchondral cyst-like lesions, the AUCs were 0.80 (95% CI 0.65–0.95), 0.84 (0.67–1.00), and 0.77 (0.66–0.85), while the sensitivities and specificities of the radiologist were 0.79 (0.65–0.93) and 0.80 (0.59–1.02), 0.40 (0.02–0.83) and 0.72 (0.59–0.86), and 0.75 (0.45–1.05) and 0.88 (0.77–0.98). In the same study, an AI-assisted tool providing saliency maps was deployed, helping improve interreader reliability for the 3 pathologies from 53% to 60%, 71% to 73%, and 60% to 68%, respectively.
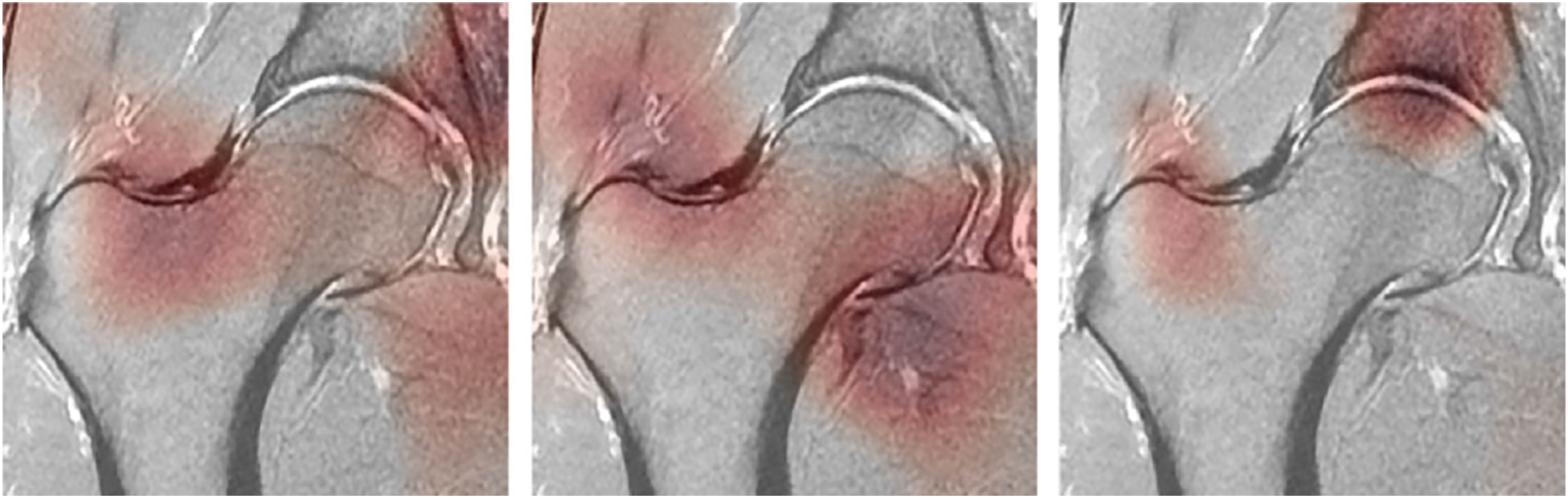
Joint effusion is another important feature of OA that is a target for both therapy as well as automated MR imaging detection and classification using AI. Using the Hip Osteoarthritis MRI Scoring system, effusion can be qualitatively assessed as “mild, moderate, and severe” (grades 0–3) with high interreader reliability, which has clinical correlations with pain. On the other hand, AI can be used to quantitatively measure joint fluid on MR imaging, known as “volumetric quantitative measurement”, for a more objective assessment that may be clinically correlated with pain as well as other symptoms such as stiffness, disability, and clinical outcome. In a comparative study including 358 hip MR imaging examinations from 93 patients with symptomatic hip OA before and after receiving fluoroscopically guided steroid injections, baseline hip joint effusion measurements by AI software demonstrated a high correlation with the mean of those by 2 human readers, with intraclass correlation of 0.82, 0.93, and 0.86 for the left hip, right hip, and per patient, respectively. The AI software developed by the authors was used to automatically identify voxels of high signal intensity in a region of interest around the hip joint outlined by the user, which was used to train a CNN for automatic segmentation of effusions ( Fig. 2 ). While the AI tool was found to overestimate fluid volumes, its initial results demonstrate promising reliability that may be further refined with greater training data.
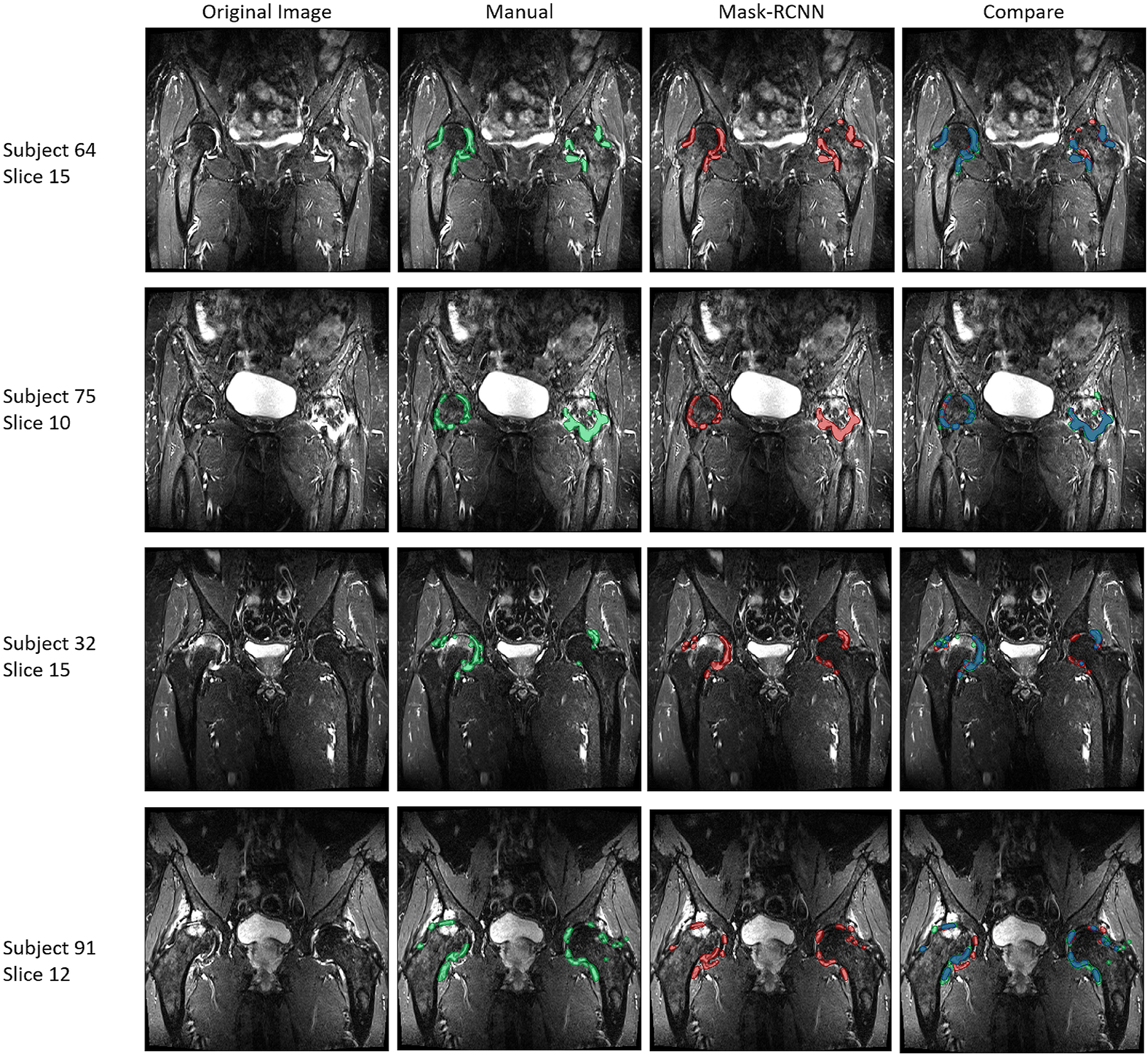
These early results demonstrate the enormous potential for AI in OA diagnosis. First, the AI assist tool improved interreader reliability on all 3 diagnoses tested, demonstrating its potential to supplement radiologists if it were deployed today. The model also demonstrated early promising results regarding the diagnostics of OA and related musculoskeletal pathologies. An AI model to detect OA can assist with triage, allowing radiologists to focus on more complicated cases, as well as possibly detecting hip OA earlier by identifying image features previously undetected by musculoskeletal (MSK) radiologists. As a result, it may serve as a possible early screening tool that can lead to early intervention to prevent further degeneration, a measure that could improve the quality of life of the millions of people who would eventually develop OA of the hip.
Femoroacetabular impingement
FAI, a hip pathology characterized by abnormal contact between the femoral head and the acetabulum, is often indistinguishable from other causes of hip pain with clinical history and physical examination, emphasizing the salient role of imaging in its diagnosis. However, FAI can pose diagnostic challenges due to its subtle morphologic features. Using 3D MR imaging, radiologists can diagnose FAI with an accuracy, sensitivity, and specificity of up to 90%. Other imaging modalities such as radiographs and computed tomography (CT) do not achieve the same accuracy as with MR imaging studies and can result in potentially harmful radiation exposure to the pelvis in young patients. In particular, radiographs are limited in the ability to diagnose FAI due to the two-dimensional (2D) representation of 3D anatomy.
Using MR images, AI models employing radiomics may be trained to diagnose FAI. Texture analysis, a type of radiomics quantifying the perceived texture of an image, may include voxel classification based on gray level and gray-level distributions in the case of MR imaging to achieve a level of analysis beyond what is possible with human perception. In a study using presurgical MR imaging studies of monolateral FAI in 17 patients from which 182 radiomic features were extracted from each hip, a K-nearest neighbor model yielded a performance with 0.970 accuracy, 0.968 specificity, 0.972 sensitivity, and 0.970 AUC for differentiating healthy joints from impingement joints. The model described achieved a higher diagnostic performance than that accomplished by current methods of detecting FAI using radiographs, CT, and MR imaging. In a study applying MR imaging texture analysis to compare and classify asymptomatic cam-negative (19 patients), asymptomatic cam-positive (25), and symptomatic cam-FAI hips (24), 4 XGBoost classification models achieved a mean validation accuracy of 79% in classification. For binary classification, the validation accuracies/AUCs were 88%/0.88 for control versus asymptomatic cam, 96%/0.93 for control versus symptomatic cam, and 87%/0.88 for asymptomatic versus symptomatic cam. These findings suggest the presence of structural changes because of cam morphology leading to processes such as altered biomechanics or physiologic changes and eventual degenerative changes and remodeling that may be detectable on MR imaging. The application of radiomics in MR imaging for FAI diagnosis streamlines the diagnostic process and enhances accuracy, reducing reliance on time-intensive manual assessments. As AI and radiomics continue to evolve, their role in musculoskeletal imaging, particularly in the diagnosis of and early intervention for complex conditions like FAI and later OA, is expected to become increasingly vital, enhancing both the efficiency and efficacy of clinical diagnostics.
Labral tears
The labrum plays an essential role in maintaining stability of the hip joint. After labral injury, hip cartilage is more easily worn down, predisposing patients to premature severe osteoarthropathy. Hip arthroscopy is the main method of treatment of these labral tears, which requires MR imaging for accurate preoperative assessment. The application of deep learning approaches for the classification of labral tear grade offers a promising solution to enhance the accuracy and efficiency of diagnosing labral tears. For instance, in a study by Ni and colleagues, a fully automated system consisting of 3 models for extraction, discrimination, detection, segmentation, diagnosis, and classification was developed. A LeNet-5 model was used to diagnose and classify labral injuries from oblique coronal (OCOR) and oblique sagittal images (OSAG). This model was trained on a dataset of 1016 patients divided into normal (n = 168) and abnormal labrum (n = 848) groups. This model achieved an accuracy in diagnosis and classification of 0.94/0.94 in the OCOR images and 0.92/0.91 in the OSAG images, while a weighted model combining the OCOR and OSAG models achieved an accuracy in diagnosis and classification of 0.94 and 0.97, respectively. These models achieved superior results to the accuracies of 4 board-certified musculoskeletal radiologists in the diagnosis and classification of labrum injuries, ranging from 0.84 to 0.92 and 0.78 to 0.94, respectively ( Fig. 3 ). Furthermore, the total diagnosis time of the radiologists was an average of 7.3 hours. The study demonstrates the potential for deep learning to automatically segment and diagnose labral tears in MR imaging. This approach has the potential to assist radiologists in improving the efficiency and accuracy in the diagnosis of labral tears.
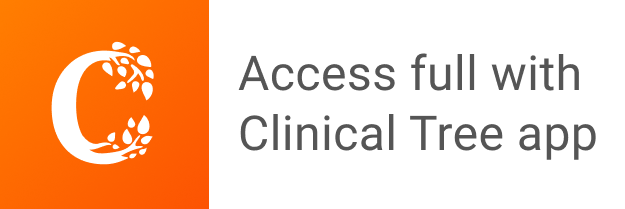