Background
Personalized medicine is yielding increasingly precise treatment and prevention strategies for groups of individuals based on their genetic makeup, environment, and lifestyle, as enabled by approaches including genomics, transcriptomics, proteomics, metabolomics, and so forth. In oncology, the goal of using such approaches is to increasingly harness individual-level information versus population-level or traditional clinical information (e.g., tumor stage, age, gender) to select the most successful cancer treatment regimen for each patient.
Molecular tumor characterization can be performed using genomic and proteomic approaches, but this requires tissue sampling from invasive surgery or biopsy. Currently, large-scale genome cancer characterization that would include genetic testing for every individual is not feasible because of the high costs, considerable time burden, and technically complex data analysis and interpretation. Moreover, even when molecular characterization is performed using tissue sampling, samples may not accurately represent the entire lesion as they are often obtained from a small portion of a heterogeneous lesion with inherent selection bias during biopsy.
By contrast, imaging can provide a more comprehensive view of the tumor in its entirety via radiomics and radiogenomics. Radiomics is an approach pertaining to the extraction and correlation of multiple imaging parameters with different variables of interest (patient characteristics as well as histopathologic, genomic, molecular, or outcome data) to create decision support models. Such models can be used for multiple purposes, such as treatment planning, risk assessment, and outcome prediction. When imaging data is correlated with genetic data in particular, this approach is referred to as radiogenomics. Coupling with artificial intelligence (AI) techniques allows us to more fully harness the power of radiomics/genomics. Because of the noninvasive nature of medical imaging and its ubiquitous use in clinical practice, the field of AI-enhanced imaging is rapidly evolving.
With continuous advances in radiomics analysis and machine learning (ML), such as deep learning (DL), we are now on the cusp of providing more effective, more efficient, and even more patient-centric breast cancer care than ever before. In this chapter, we will begin with the basic concepts of radiomics, radiogenomics, and AI methodology in breast magnetic resonance imaging (MRI). The rest of the chapter will be devoted to reviewing AI-enhanced MRI and AI-enhanced diffusion-weighted imaging (DWI), whereby we will present the current knowledge and future applications of AI-enhanced MRI and DWI in clinical practice and address their challenges and limitations.
Basic Concepts of Radiomics and AI Methodology
Radiomics analysis can be divided into two arms based on how imaging information is transformed into mineable data: handcrafted and AI based ( Fig. 10.1 ). Handcrafted radiomics extracts features that are used to fingerprint phenotypical characteristics in images, whereas AI uses a complex network to create its own features.
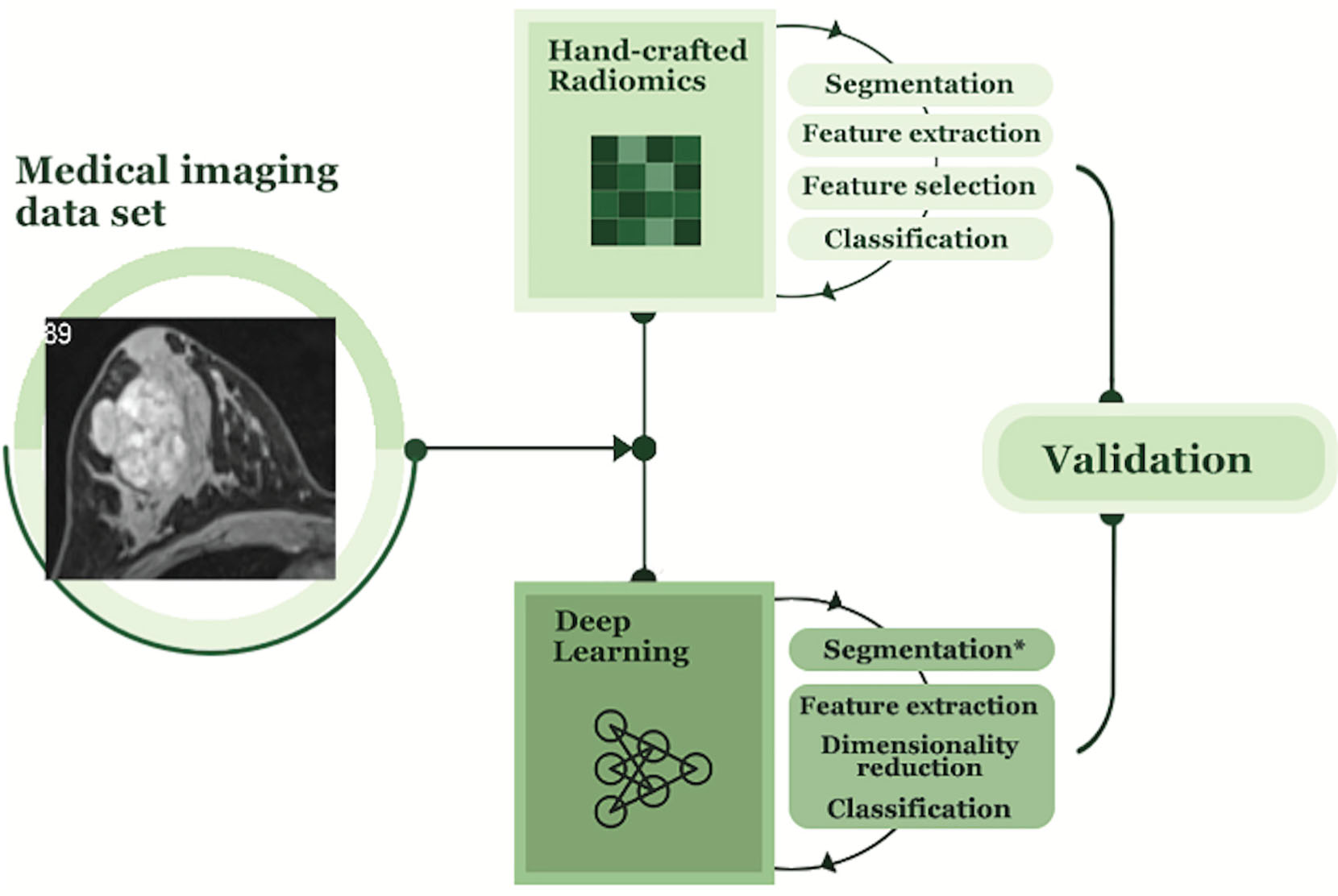
Handcrafted radiomics methodology usually follows this workflow: image acquisition (2D, 3D, 4D); normalization to pixel intensities evenly across a data set and within a standardized range; image annotation and segmentation (manual, semiautomatic, or fully automatic; Fig. 10.2 ) and for definition of region of interest (ROI) and feature extraction; radiomics analysis (feature selection and reduction); and classification and modeling. Handcrafted radiomics analysis includes first-order features based on the distribution of pixel intensities (histogram based) and higher-order features based on how pixels are positioned in relation to each other (e.g., co-occurrence matrices, run length matrices, size zone matrices, neighborhood gray-level dependence matrices, Minkowski functionals, local binary patterns, wavelet analysis). Because a large quantity of imaging features are extracted, which are not necessarily all relevant to the task proposed to the model, feature selection and reduction is an essential step, followed by classification and modeling to answer the specific question we are proposing. Handcrafted radiomics studies typically use AI methods (e.g., decision trees, support vector machines, random forests, neural networks) to select features and construct models. Ideally, the model’s performance should be validated in external data sets to avoid overfitting, which refers to spurious correlations in the data that do not allow generalization to other similar data sets. If no external validation data set is available, cross-validation techniques can be applied to split the data into different subsets (training and validation sets). To be able to expand the interoperability of models to all hardware, acquisition, and reconstruction parameters in general clinical practice, rigorous standardization is necessary but is hard to achieve. Standardized data collection, evaluation criteria, and reporting guidelines will be required for radiomics to mature as a discipline. To gauge the quality of published radiomics studies, a radiomics quality score has been proposed ( Fig. 10.3 ), and to address the issue of radiomic feature reproducibility, some harmonization methods such as Combine Batches (ComBat) have been investigated in the literature.
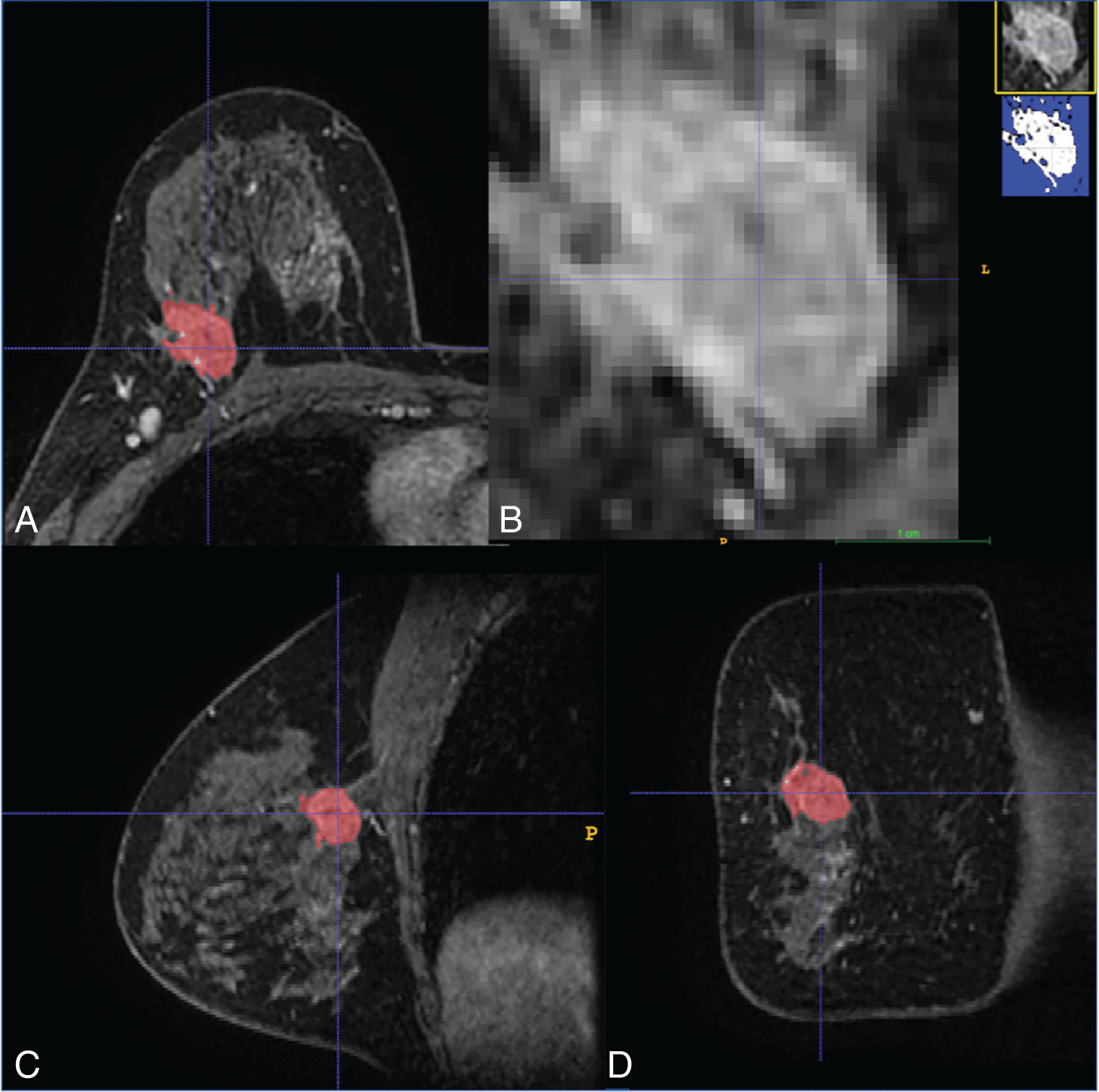
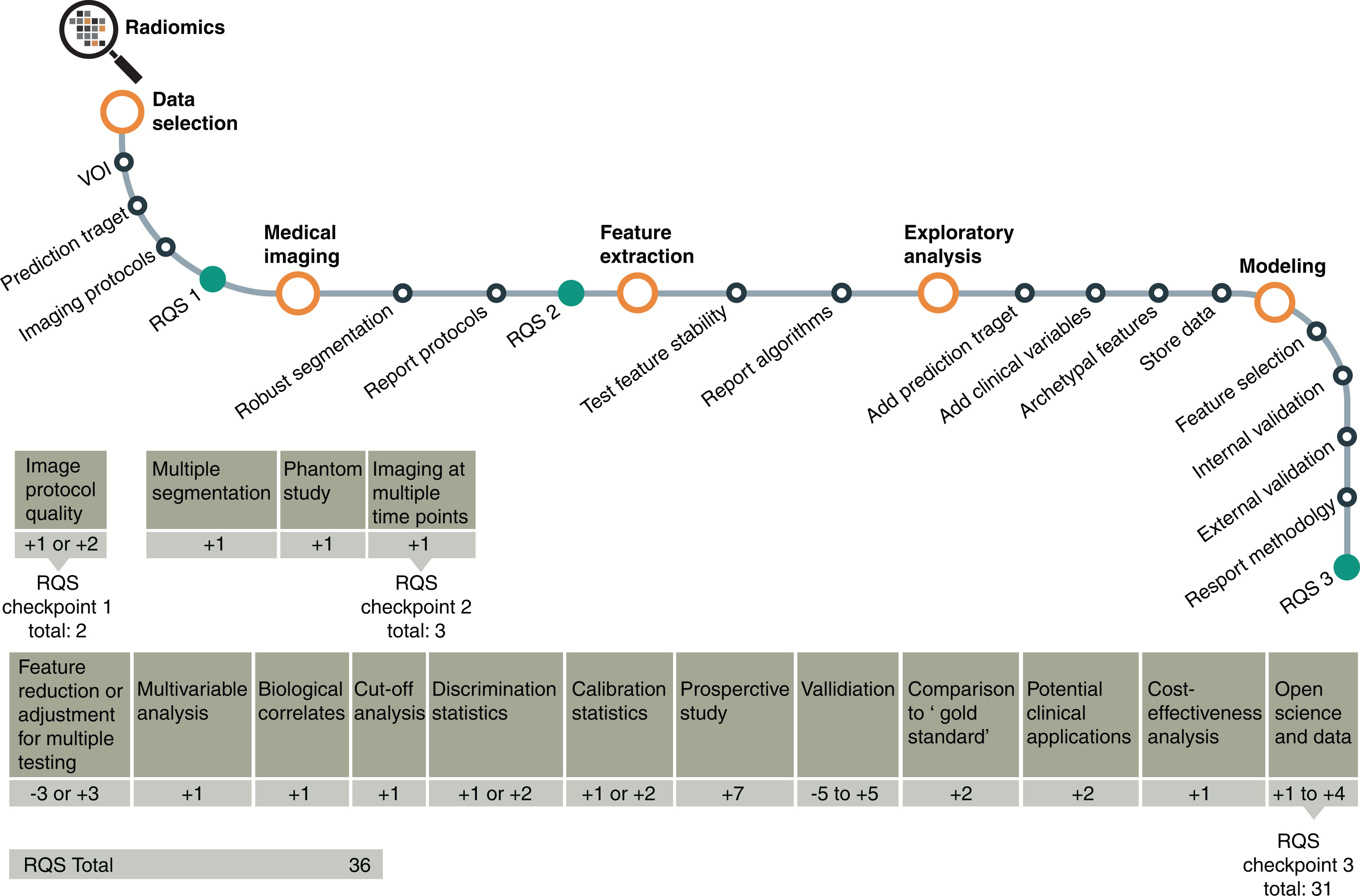
DL is a new class of ML that uses neural networks with multiple layers of processing inspired by human brain architecture. In contrast to traditional radiomics-based ML approaches, where humans engage in handcrafted feature extraction, DL networks learn both the feature extraction and classification steps in tandem and are able to extract very high-level features from imaging data. These recent advances in software and also hardware (to support higher computational power requirements) have given DL models the potential to surpass human performance in some image analyses tasks.
To date, most DL applications in medical imaging use convolutional neural networks (CNNs), which are particularly well suited to visual tasks. CNNs can be used for both image classification and image segmentation. In supervised learning approaches, which constitute almost all the DL in medical imaging literature to date, it is necessary to supply the CNN with large numbers of labeled imaging data. To develop a CNN model, imaging data sets must be divided into three independent groups: training, validation, and testing data sets. First the DL model is trained on the training set images and learns to predict the label. This process is repeated many times with different model hyperparameters, with intermittent evaluation of performance using the validation data set to prevent overfitting. Then, once the DL model parameters and hyperparameters have been finalized, the held-out test set is used to evaluate final CNN performance and results are reported with a standard set of relevant statistical metrics (e.g., area under the curve [AUC], precision recall, sensitivity, specificity). DL studies must pass through rigorous validation steps, which includes definition of the image sets (training, validation, and test sets) and ground truth reference standard; detailed description of the model, training approach, and metrics of model performance; and validation or testing on external data.
Further reviews of the process of radiomics/genomics analysis coupled with AI (image acquisition, volume of interest selection, segmentation, feature extraction and quantification, database building, classifier modeling, and data sharing) are described in detail elsewhere.
AI-Enhanced Breast MRI
Breast MRI is the most sensitive modality for breast cancer detection, with a pooled sensitivity of 93% and pooled specificity of 71%. Dynamic contrast-enhanced MRI (DCE MRI) is the primary sequence of the breast MRI examination, which relies on intravenous injection of a gadolinium-based contrast agent and provides excellent morphological information and functional information about abnormal vascularization as a tumor-specific feature. DCE MRI is regarded as the most sensitive imaging technique for breast cancer detection. However, it has been criticized for its variable specificity.
To overcome limitations in DCE MRI specificity and to obtain more valuable functional data, additional MRI sequences have been combined with DCE MRI; this approach is known as multiparametric MRI (mpMRI). In the multiparametric context, DWI with apparent diffusion coefficient (ADC) mapping or more advanced markers (see Chapter 8 ) has emerged as the most robust and valuable additional parameter, with a reported sensitivity of up to 96% for breast cancer detection and a specificity of up to 100% for breast tumor characterization; it is therefore increasingly implemented in clinical routine.
Compared with other imaging modalities, breast MRI is the most sensitive for detection and additionally offers quantitative biomarkers with value for breast cancer diagnosis. As a result, it is well suited to AI-based research, and AI-enhanced breast MRI is increasingly studied for a variety of applications, particularly for lesion detection and classification. Table 10.1 summarizes the current studies and its use cases for AI-enhanced breast DWI.
Use Case | Field Strength (T) | b -Values | Segmentation | Input | AI Approach |
---|---|---|---|---|---|
Detection | |||||
Bickelhaupt et al. | 1.5 | 0, 1500 | 3D, manual | DWI, DWIBS, ADC | Radiomics/ML |
Dalmis et al. | 3 | 50, 800 | 2D, marker placement | DCE, T2, ADC | DL |
Lo Gullo et al. | 1.5/3 | 0, 800 | 2D, semiautomated | DCE, ADC | Radiomics/ML |
Molecular Subtyping | |||||
Leithner et al. | 3 | 0, 1000 | 2D, manual | DCE, ADC | Radiomics/ML |
Leithner et al. | 3 | 0, 1000 | 2D, manual | ADC | Radiomics/ML |
Sun et al. | 1.5/3 | 0, 1000 | 2D, manual | DCE, DWI | Radiomics/ML |
Xie et al. | 3 | 0, 400, 800 | 3D, semiautomated | DCE, ADC | Radiomics/ML |
Zhang et al. | 3 | 50,800 | 3D, semiautomated | ADC | Radiomics/ML |
Treatment Response Prediction and Assessment | |||||
Amornsiripanitch et al. | 3 | 0, 800 | 2D, manual | DWI, ADC | ML |
Liu et al. | 3 | 0, 1000 | 3D, manual | DCE, T2, DWI, ADC | Radiomics/ML |
Tahmassebi et al. | 3 | 50,850 | n/a | DCE, T2, ADC | ML |
Thakur et al. | 3 | 0, 600, 800 | 2D, manual | DWI, ADC | n/a |
Use Cases
Detection
Fully automated detection of breast cancer on screening MRI using CNN has shown to be possible, not only for systematic diagnostic interpretation but also to identify tumor-containing slices stored on picture archiving and communication systems. The latter can be particularly useful for nonsystematic image review, such as for research purposes or interdisciplinary tumor board meetings. The growing use of breast MRI for both screening and conventional imaging problem-solving purposes has posed significant challenges in clinical practice due to the high number of incidental MR-detected lesions. In this context, different approaches have been tested to help classify breast lesions identified on MRI as benign or malignant. For example, Truhn and colleagues compared the diagnostic performance of radiomics with ML and CNN to radiologists for the classification of DCE MRI–enhancing lesions. They evaluated 447 patients with 1294 lesions and found that CNN (AUC = 0.88) was superior to radiomics/ML (AUC = 0.81) for lesion classification, but both approaches were inferior to radiologists’ performance.
Focusing on DWI used in a biparametric contrast-agent-free MRI context, Bickelhaupt and colleagues investigated radiomics with DWI in combination with T2-weighted imaging for the classification of lesions that were deemed to be suspicious on breast cancer screening with mammography as benign and malignant. In this study, 50 asymptomatic women who underwent screening mammography and who were diagnosed with a suspicious finding were examined with multiparametric MRI with DCE, T2-weighted, DWI, and DWI with background suppression (DWIBS) sequences with ADC mapping. For the purpose of this study, out of this standard multiparametric MRI protocol an unenhanced, abbreviated DWI (ueMRI) including T2 -weighted, DWI, and DWIBS sequences and corresponding DC maps was extracted and used for radiomics analysis. Three-dimensional segmentations of the MR index lesions were generated manually and performed separately on T2TSE images, and DWIBS b -1500 images and radiomics features were extracted. Segmentations of the background parenchyma on DWIBS b -1500 images and normal-appearing fat on the T2-weighted image, which were used to normalize the MR intensities of the corresponding images in terms of lesion-to-background ratio, were performed. In addition to radiomics analysis, an expert radiologist assessed this unenhanced abbreviated protocol for lesion classification as well as a full multiparametric protocol, including DCE MRI. From the ueMRI with DWI, DWIBS, and T2-weighted imaging, three Lasso-supervised ML classifiers were constructed (i.e., univariate mean ADC model, unconstrained radiomic model, and constrained radiomic model with mandatory inclusion of mean ADC) and compared with the clinical performance of a highly experienced radiologist. The radiomic classifiers allowed a differentiation of malignant from benign lesions with a AUC of 84.2% for the unconstrained and 85.1% for the constrained, respectively, compared with 77.4% for mean ADC and 95.9% for the ueMRI protocol and 95.9% for the full multiparametric protocol of the experienced radiologist. The results of this study indicate that DWI radiomics classifiers can perform well in breast cancer diagnosis and achieve higher performance than the mean ADC parameter alone. Diagnostic performance was lower than that of an experienced breast radiologist, but results indicate the potential of AI-enhanced DWI to provide a diagnostic decision tool to benefit less-experienced readers to achieve near expert reader performance.
Dalmis and colleagues also applied AI for classification of breast lesions using a multiparametric MRI protocol with ultrafast DCE MRI, T2-weighted imaging, and DWI. A final AI system combining all imaging information achieved an AUC of 0.852, significantly higher than ultrafast DCE alone ( P = .002) and with fewer false positives when operating at the same sensitivity level of radiologists. Thus, the application of AI for the interpretation of multiparametric breast MRI may improve specificity, reducing the number of unnecessary breast biopsies. In another study, a similar conclusion was reached using a DCE MRI radiomics AI 4D classifier including automatically extracting BI-RADS curve types and pharmacokinetic enhancement features, which was able to avoid up to 36.2% of unnecessary biopsies.
The ability to accurately classify and reduce the number of unnecessary breast biopsies is particularly important in the setting of screening-detected, nonpalpable lesions such as subcentimeter lesions, nonmass-like lesions, and high-risk lesions. AI can improve the diagnosis of these type of lesions in breast MRI. For example, Lo Gullo and colleagues evaluated subcentimeter enhancing lesions in BRCA mutation carriers and showed that radiomics/ML based on multiparametric breast MRI improved diagnostic accuracy compared with qualitative morphological assessment using BI-RADS classification and could be used as an adjunct to spare unnecessary biopsies for benign-appearing small breast masses in this population. However, DWI signal analysis did not contribute to the accuracy of assessing these lesions; the authors stated that this might be partly due to the limited spatial resolution of DWI, which makes it challenging to accurately evaluate subcentimeter masses on DWI. In another study, the same group investigated whether radiomics coupled with ML based on multiparametric MRI could help in predicting malignant upgrade in atypical ductal hyperplasia (a high-risk lesion) to avoid surgical excision. Unfortunately, this approach was not able to accurately predict which biopsy-proven atypical ductal hyperplasia lesions would be upgraded to malignancy; radiomic features from DWI in particular did not add any value to the ML model.
Molecular Subtyping
In the past decade, gene-expression profiling has revolutionized breast cancer classification and replaced traditional categorizations based on immunohistochemistry with molecular subtypes. Four intrinsic molecular subtypes of breast cancer have been revealed from extensive profiling at the DNA, microRNA, and protein levels by the Cancer Genome Atlas (TCGA) Network : luminal A, luminal B, HER2-enriched, and triple negative (TN). Molecular breast cancer subtypes are unevenly distributed within patients, occur with different tumor phenotypes, and are associated with distinct prognosis, response to treatment, preferential sites of metastasis, and recurrence or disease-free survival outcomes. Since 2011, the St. Gallen International Expert Consensus panel has maintained molecular subtype–based recommendations for systemic therapies for breast cancer.
Associations between MRI characteristics and molecular breast cancer subtypes have been investigated in several studies. In a systematic review and meta-analysis published in 2014, Elias and colleagues reported that higher tumoral enhancement is associated with the luminal B subtype, whereas HER2-enriched cancers are more likely to show fast initial enhancement or washout kinetics with circumscribed margins. Elsewhere, TN cancers have been associated with high T2 signal intensity and the presence of rim enhancement. In a more recent study by Grimm and colleagues, associations between breast MRI findings using the BI-RADS lexicon descriptors and breast cancer molecular subtypes were assessed. For this purpose, qualitative BI-RADS descriptors were evaluated on DCE MRI in 278 patients with breast cancer presenting as masses or nonmass enhancement (NME); results showed significant correlations between mass shape and basal cancers, mass margin and HER2 cancers, and internal enhancement and luminal B cancers. In another study, Yamaguchi and colleagues assessed the relationship between the delayed phase of enhancement of DCE MRI and molecular subtypes, finding that ER-positive and/or PgR-positive and HER2-negative cancers demonstrated less washout. Focusing on DWI in particular, HER2-enriched tumors have been shown to have the highest ADC values, whereas luminal B/HER2-negative cancers showed the lowest values.
More recently, radiomic features have themselves been associated with molecular breast cancer subtypes. While most of the data is available for DCE MRI, the concept of molecular subtyping can be extended to other MRI sequences including DWI.
In DCE MRI, Mazurowski and colleagues showed that extracted MRI radiomics features that relate to an increased ratio of tumor-to-background parenchymal enhancement were associated with HER2-positive cancers. This difference might be due to the increased vascularization found in HER2-positive subtypes mediated by VEGF, which leads to increased vessel diameter, vascular permeability, and extracellular fluid. Grimm and colleagues found correlations between extracted morphological, texture, and dynamic radiomics features from routine MRI and luminal A and B breast cancer subtypes. In a study including 143 patients, Leithner and colleagues evaluated the diagnostic performance of radiomic signatures extracted from DCE MRI for the assessment of breast cancer receptor status and molecular subtypes. In the training data set, radiomic signatures yielded the following accuracies >80%: luminal B versus luminal A, 84.2% (mainly based on co-occurrence matrix [COM] features); luminal B versus TN, 83.9% (mainly based on geometry features [GEO]); luminal B versus all others, 89% (mainly based on COM features); and HER2-enriched versus all others, 81.3% (mainly based on COM features). Radiomic signatures were successfully validated in the separate validation data set for luminal A versus luminal B (79.4%) and luminal B versus TN (77.1%). The authors concluded that radiomic signatures with DCE MRI have the potential for the assessment of breast cancer receptor status and molecular subtypes with high diagnostic accuracy. Other studies reported similar findings, and the data indicates that specific molecular subtypes seem to carry radiomics signatures on DCE MRI images that can be used to accurately classify lesions with respect to receptor status and molecular subtypes. These signatures may have the potential to provide prognostic indicators derived from the whole tumor, while biopsy sampling, currently used for molecular subtyping, is only giving a portion of the bigger picture. This could be especially useful to monitor biological changes during treatment, which may vary throughout the tumor.
Focusing on DWI in particular, in a study by Leithner and colleagues, features extracted from ADC maps achieved accuracies over 80% for breast cancer subtype differentiation. The authors found that luminal B and HER2-positive cancers seemed to carry distinct radiomic features that were different from others. Further investigation with multiparametric MRI also showed AUCs over 0.80 for noninvasive differentiation of TN and luminal A breast cancers from other subtypes. Accuracy was superior for radiomics features extracted directly from the ADC map ( Fig. 10.4 ). In another recent study, Wang and colleagues explored whether radiomic features on DWI can be used to identify TN breast cancer (TNBC) and other subtypes (non-TNBC). They showed that breast tumors exhibit differences in radiomic features with DWI, allowing a good discrimination between TNBC and non-TNBC tumors with an accuracy of 83.4% and an AUC of 0.804.
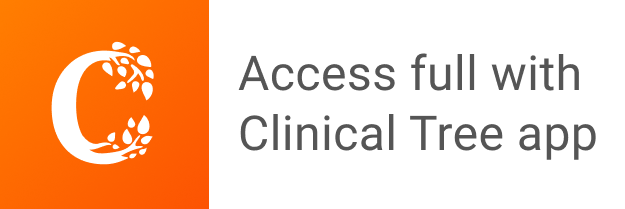