No one knows what the paradigm shift of artificial intelligence will bring to medical imaging. In this article, we attempt to predict how artificial intelligence will impact radiology based on a critical review of current innovations. The best way to predict the future is to anticipate, prepare, and create it. We anticipate that radiology will need to enhance current infrastructure, collaborate with others, learn the challenges and pitfalls of the technology, and maintain a healthy skepticism about artificial intelligence while embracing its potential to allow us to become more productive, accurate, secure, and impactful in the care of our patients.
Key points
- •
Software architecture is likely to undergo a modern renaissance and provide radiologists and patients with more autonomy in how they use and interact with AI solutions.
- •
Larger medical image specific image databases for training and pre-trained neural networks for transfer learning are likely to emerge in the future.
- •
AI will not only impact the imaging evaluation workflow, but will also aid in operational logistics in the medical imaging center.
- •
Standards used to store and interact with medical imaging data such as DICOM will continue to evolve.
The future cannot be predicted, but futures can be invented. —(Dennis Gabor, awarded 1971 Nobel prize for inventing holography)
Introduction
Any attempt to predict the next 10 years, much less beyond, must keep in mind that just a decade ago, 2 of Geoffrey Hinton’s University of Toronto graduate students achieved a major serendipitous conceptual leap forward in computer vision that was not anticipated by any futurists. Alex Krizhevsky realized that graphics processing units (graphics processing units were mostly associated then with video games) could profoundly speed up the execution of an algorithm invented in 1986 and subsequently improved by Hinton in the mid 2000s; the restricted Boltzmann machine. Subsequently, Alex’s colleague, Ilya Sutskever, realized that this combination could be applied to the Stanford ImageNet challenge, which ultimately resulted in a landslide victory. They published the seminal paper, “Imagenet classification with deep convolutional neural networks” in 2012. These deep convolutional neural networks have become so widely implemented that they have become what is now often generically referred to as artificial intelligence (AI).
Future predictions could similarly miss out on anticipating that type of game-changing leap forward, which could come in the form of new or previously described algorithms and existing or new hardware. Quantum computing could provide this type of quantum leap, but other major paradigm shifts are more likely in a 10-year time period, rendering the incremental and evolutionary predictions in this article obsolete. William Orton, President of Western Union’s statement about the telephone in 1876 in which he asked, “why would any person want to use this ungainly and impractical device when he can send a messenger to the telegraph office,” or Ken Olson, Chairman and Founder of Digital Equipment Corporation’s statement that there “is no reason for any individual to have a computer in his home,” come to mind when thinking about the potential folly of conjuring up predictions about the future that we are attempting in this article.
The status of AI in medical imaging in the next 10 years will depend on regulatory policy, reimbursement models, success in the incorporation of AI into routine workflow, development and adoption of standards versus platforms for AI applications, and the level of success in generalizing deep learning algorithms to different machines, geographies, and diverse patient populations to minimize bias. It will also depend on a major cultural change not only in the level of acceptance of computers in detection, diagnosis, and treatment, but also in knowing how to provide the added value of human wisdom and judgment. Finally, it is critical to improve our understanding of the pitfalls of deep learning and maintain a healthy and constructive skepticism as we explore the tremendous potential of the technology.
Future regulatory environment
At this time, there are 114 medical imaging software as a medical device products that have been cleared by the US Food and Drug Administration. These developments are occurring at a rapidly accelerating pace from 2008 through 2020 with almost 70% of these receiving clearance in the past 2 years ( Fig. 1 ).
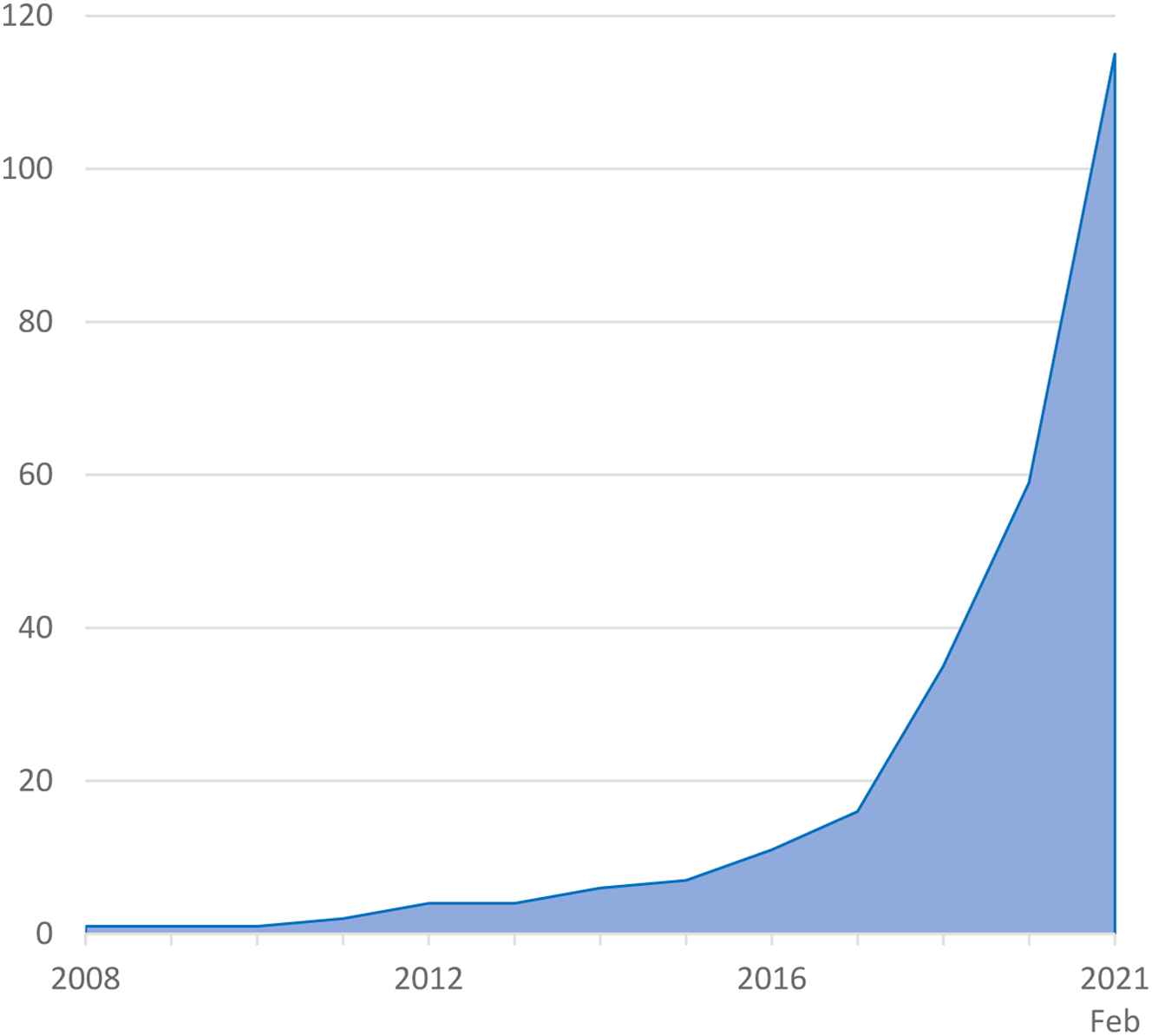
In January 2021, the US Food and Drug Administration published an action plan for medical AI algorithms detailing its intention to publish “prespecifications,” which address a fundamental change in the way in which algorithms are applied in clinical practice. These prespecifications describe how an AI application could change through learning, with feedback based on radiologist impressions as well as patient outcomes. This proposal for support for dynamic algorithms that could continuously adapt and improve would be a major game changer in AI and would revolutionize the way in which these algorithms are customized and improve with time.
Another impactful direction taken by the US Food and Drug Administration is the advancement of pilots to evaluate real-world performance of these algorithms in clinical practice. This postmarket surveillance and the feedback garnered through these efforts will also have a major impact on how AI is regulated and consumed in the next several years.
The patient as a direct consumer of medical imaging artificial intelligence
Nonradiologists Will Increasingly Have Access to and Rely on Artificial Intelligence Software
This shift is already happening with AI software designed to determine the likelihood that a patient has experienced an acute stroke owing to large vessel occlusion, with clinicians relying on the software to make a diagnosis to determine whether to expedite care for these patients. As these systems improve over the next few years, the relative added value of the radiologist in these types of cases will be called into question and their role in using their experience and judgment will be tested. At the end of 2018, there were an estimated 5 million subscriptions to AI dermatology applications with a projection of 7.2 million on smartphones by 2024. Within a 10-year timeframe, patients will have access to an increasing number of radiology AI apps, including those that purport to detect and diagnose disease. Initially these AI applications will take the form of improved intelligent scheduling and translation of medical jargon for radiology reports. But eventually applications providing an analysis of MR imaging brain studies, knee MR imaging studies, computed tomography (CT) scans of the thorax for evaluation of lung nodules or chronic obstructive pulmonary disease, and many others will be available to consumers routinely. Once patients have access to their images in a format in which they can easily submit them to cloud AI providers, as is already done with smartphone cameras and skin cancer applications, there will be many additional regulatory concerns that will need to be addressed.
Architecture of the future in the delivery of artificial intelligence algorithms
Architecture is the stage on which we live our lives. —Mariam Kamara (architect)
Despite almost 3 decades of experience with picture archiving and communication system (PACS) and filmless radiology, there has been surprisingly little change in the fundamental ways in which radiologists interact with their workstations and the largely monolithic model of having a single vendor supply their own proprietary suite of image review and visualization software, image storage, network and quantitative tools, and, in some cases, even speech recognition and reporting software. Although radiologists have supplemented these with third-party applications and products, these products rarely operate smoothly within the PACS workflow, although they can provide users with best of breed options for all these functions. These third-party applications typically require additional steps as well as additional physical workstations or monitors and are often time intensive and frustrating to use. Radiologists often prefer their own applications and workstation tools for different types of imaging studies, sometimes resulting in a warehouse of hardware and software solutions in the reading room environment. Access to clinical information through the electronic medical record is increasingly important as is access to all images in increasingly large health care networks.
The Academy for Radiology & Biomedical Imaging Research proposed a diagnostic cockpit, which is described as a “future-state digital platform to aggregate, organize, and simplify medical imaging results and patient-centric clinical data” to “help clinicians become ‘diagnostic pilots’ in detecting disease early, making accurate diagnosis, driving image-guided interventions, and improving downstream clinical management of patients.”
Today’s working environment can be very confusing, cumbersome, and inaccessible to even the most sophisticated and cutting-edge radiologists. Fundamental features such as hanging protocols (automated set up of how one or more imaging studies are arranged on workstation monitors) are currently surprisingly primitive and brittle to minor changes in imaging sequences, number of monitors and other factors. Machine learning algorithms will be more generally used to re-engineer the “hanging protocol” challenge for PACS by replacing a rigid, highly structured set of rules with a predictive engine that watches radiologist behavior, more closely emulating the way in which a trainee learns the preferences of an attending radiologist. These algorithms will be optimized for a particular type of study, patient, and clinical indication incorporating the electronic medical record ( Box 1 ).
- •
Decide on how images should be reconstructed and visualized similar to pathologists determining preparation of a pathology specimen
- •
Decide about postreconstruction visualization tools and image processing and enhancement
- •
Implement radiomics quantification for specific indications
- •
Design their working–living space similar to the way surgeons can optimize their operating theater
- •
Freely shop in a marketplace to find a product that suits their individual needs and preferences and workflow
AI applications in the future should be able to respond to and communicate with an “algorithm orchestrator” and with any component of a next generation radiology ecosystem through APIs based on agreed upon vendor-neutral and/or proprietary standards. Multiple apps and services will exist on a platform analogous to a smartphone operating system and marketplace ( Fig. 2 ).
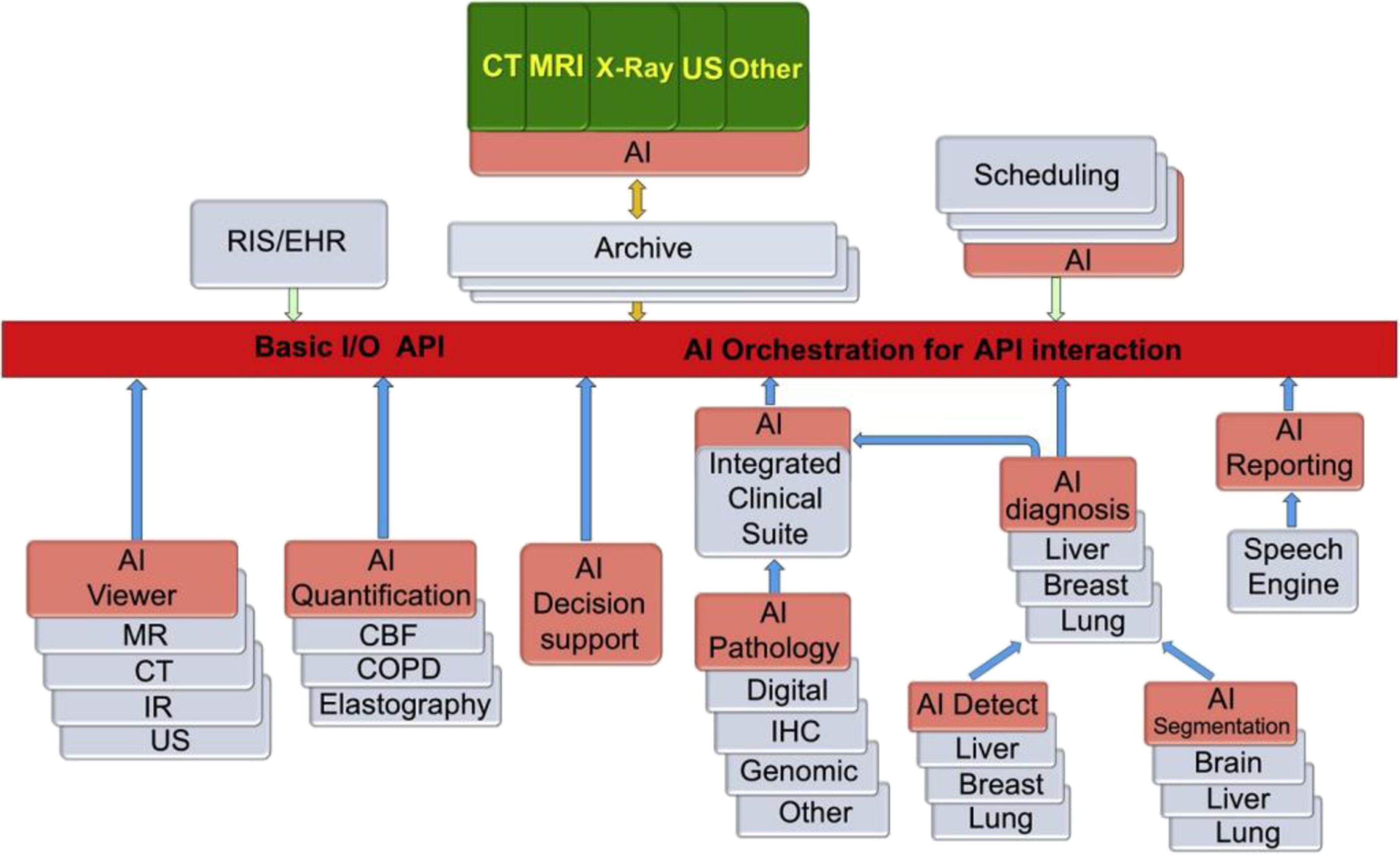
In an analogous manner to streaming the song you like rather than the previous model of purchasing the whole CD with 2 songs you want and 16 you do not, radiologists and clinicians will be able to select best of breed applications that meet their specific needs for a specific study. A radiologist who is not specialized in a particular area could potentially perform at the level of a subspecialist with the correct AI applications.
In the vendor-neutral algorithm model, all components will be selected by an algorithm workflow orchestrator based on best of breed quality, reliability, and/or cost in a dynamic fashion. A more dynamic, cost-effective, and interoperable storage infrastructure will facilitate exchange of medical images among various institutional clouds so that relevant comparison reports and images will be available when required. Radiologists will have the flexibility to select their preferred software for reviewing, analyzing, and reporting examinations, which will also enable smaller institutions or even individual radiologists the ability to level the playing field and have access to software and processing resources similar to those available currently at larger institutions.
Multifacility radiology and especially large national teleradiology groups are either incorporating or creating machine learning solutions to optimize case distribution to the radiologists. Two factors are typically considered in this optimization, namely, case urgency and radiologist specialization. Examples of the first include solutions for detecting certain critical findings on medical imaging examinations, such as intracerebral hemorrhage or stroke, pneumothorax, respiratory infections, and suspicious breast findings, and prioritizing these findings for review. An example of the second approach is a workflow manager that claims a 15% increased efficiency of radiology interpretation and 82% subspecialist assigned reads. An AI-driven complexity score could be used in the creation of a more sophisticated relative value unit assessment of radiologist productivity within a single group.
Ensembles of programs will be used to form a consensus of opinions or quantitative analysis and multiple programs could be used to complete various steps in the image interpretation process. For example, one could imagine an algorithm that could segment the adrenal glands, another that could analyze the distribution of density of pixels in this segmented region, still another that could compare contrast and noncontrast image, and still another that could create an a priori or post interpretation estimate of the odds of malignancy and determine the most likely diagnosis. These cooperative algorithms will become routine once advanced algorithm orchestration enabled platforms are available. These algorithm or workflow orchestration platforms will not only coordinate different applications, but also will allow interaction between the radiologist or clinician.
Data for artificial intelligence in the future
Initial attempts at creating de-identified public datasets such as The Cancer Imaging Archive have been extraordinarily useful to the research and commercial communities; however, annotation of these images has been limited and the size of available datasets is considered to be relatively small. Modifications to the DICOM standard and the adoption of annotation and mark-up standards such as the National Cancer Institute–sponsored Annotation and Image Mark-up initiative have the potential to provide the needed foundation for easier cross-institutional exchange, decentralized repositories, and richer data labels and elements.
The current state of the art for security in digital imaging and for electronic patient records is substantially behind other sectors of the economy. Numerous vulnerabilities are inherent to not only deep learning imaging dataset aggregation efforts, but also in routine clinical practice with relatively insecure clinical data storage, a lack of audit of data access, the ability to inject or remove pathology in images without any ability to detect these changes, and the inherent security issues around physical media such as CDs, DVDs, and USB thumb drives.
A next-generation approach to the storage of DICOM images will begin to be implemented in the next several years owing to increasing security concerns over clinical and research imaging archives. DICOM was originally designed to enhance image accessibility with limited security functionality. The increasing use of blockchain and related technologies in other industries will finally come to health care medical records and specifically to medical imaging. Hash-based data structures known as Merkle trees can be used for efficient data verification and are already used in peer-to-peer networks such as Tor, Bitcoin, and Git. These protocols will permit secure, fine grained access control of image exchange within and among health care facilities and will make it much more difficult to alter either DICOM tags or the pixels in a medical imaging study. These files will be interoperable with traditional DICOM storage and data exchange protocols, but will be optimized for performance and security, as well as the development of deep learning algorithms from stored medical images.
Federated machine learning models, which represent a class of distributed systems that rely on remote execution, will increasingly allow deep learning models to be trained on local data without having to upload the data from a facility’s firewall, decreasing the risk of a patient privacy breech. This method is viable, but not yet completely proven, for combining data from different institutions, machines, and geographic areas for a larger and more diverse database without the inherent issues related to sending studies outside of an institution’s firewall. Challenges include coordinating a uniform methodology for data curation at multiple sites, and the lack of an agreement on how to update the central model state to achieve results comparable to those in which all the data were combined into a single dataset. Homomorphic encryption involves performing deep learning directly on encrypted data rather than using encryption merely for communication and storage of the data. Homomorphic encryption will result in much more secure multiparty computation. Secure encrypted processing hardware will become routine in computers and mobile devices such as next generations of smartphones to maintain encryption at these user edge devices.
Another promising technique that will become increasingly popular creates synthetic medical images from generative adversarial networks to potentially supply unlimited numbers of images that could be created from one or more image databases.
Development of new radiology specific neural network architectures (beyond ImageNET)
Most of the current AI applications in medical imaging have relied on transfer learning from the widely used and popular visual database ImageNet. This database is large and has been used for a wide variety of different computer vision applications in addition to medical imaging and has resulted in impressive advances for convolutional neural networks in image recognition tasks. In addition to not using medical images, but various objects such as animals and flowers and cars and others, there are other limitations to ImageNet for medical imaging applications, including the fact that it used just a single label description of images rather than multiple labels on an image. This latter multiple labeling is more applicable to medical images that frequently contain more than 1 feature on a single image. Large, coordinated efforts such as the recently created Medical Imaging and Data Resource Center and others may make tens of thousands of cases publicly accessible and could serve as a basis for adjunctive databases that are optimized for medical image deep learning.
In the future, labeling of these and other datasets could be incorporated directly into a radiologist’s clinical workflow with improvements in annotation tools, incorporation of AI into viewer software, and with natural language generation. The use of structured DICOM (DICOM-SR) or annotation image markup for annotations over proprietary vendor-specific annotations may also help to realize this potential.
Non–pixel-based artificial intelligence applications will be developed in parallel to pixel-based ones
Non–pixel-based applications using a variety of natural language understanding technologies will become widely implemented. Like the advances in human vision made possible by the 1.3 million pictures in the ImageNet annotated dataset, database-based models such as OpenAI’s natural language processing model, GPT-3 are advancing natural language processing with more than 175 billion weighted connections between words.
The next generation of natural language processing and natural language understanding tools will be used to make radiology reports machine intelligible, given the fact that diagnostic imaging will be unlikely to make a full transition to structured reported within the next decade. Communication and follow-up of unexpected findings, synthesis of pertinent information from the electronic medical record for the radiologist, and automatic generation of an impression from the body of a report will use natural language understanding and will be ubiquitous within the decade. The extraction of a priori patient data from the electronic medical record will enable detection and decision support algorithms to provide more accurate and pertinent insights and these data will be made available to these AI algorithms.
The widespread use of liquid biopsies, which use cell-free DNA, RNA, circulating tumor cells, and extracellular vesicles, has not yet occurred owing to a variety of pitfalls, including a lack of reproducibility and discordant results. Once these issues are addressed, it will become common to have patients referred for imaging who are found by liquid biopsy and/or genomic analysis to have substantially increased risks of having specific types or classes of cancer or other diseases. Cohen and colleagues described a study with 10,006 women in which 31% of patients who were subsequently found to have cancer in 1 of 7 organs for which no standard screening is available, had a positive liquid biopsy. Additional workup to help localize these was done using PET/CT scans. The widespread use of liquid biopsies for screening will put increased pressure on imaging workflows, likely improving the efficiency of advanced imaging studies, which will have a higher pretest probability of an abnormal finding.
Another machine learning application will be to predict no-show rates for imaging examinations. This clinical scheduling issue has become even more of a challenge during the coronavirus disease 2019 (COVID-19) pandemic.
A recent AI approach to decrease no show rates resulted in a 17% decrease in the no-show rate within 6 months of implementation using predictive analytics. These tools are even more important now than they were in the past and they will continue to be used with increasingly sophisticated and improving approaches. Tools for business optimization such as these may be the earliest opportunity for AI adoption in medical imaging because there is a clear return on investment. Future refinements could be used to predict the potential impact of overbooking patients for studies, determining which patients might need additional notification of appointments or assistance with transportation, predicted patient arrival times for those arriving before or after scheduled appointments, and even anticipatory rebooking of patients at highest risk for no shows. The pandemic has also accelerated the transition to remote diagnosis and the use of the cloud, which, in turn, has made cloud-based AI applications more acceptable to medical imaging facilities. Additionally, the scramble to obtain shared COVID-19–related imaging datasets has increased the engagement of the medical imaging scientific community with the clinical medicine community and brought about new collaborations. Through the increasing availability of shared resources for COVID-19 data as well as research funding for solutions to the pandemic, new collaborations have allowed radiologists to interface directly with computer scientists, data scientists, engineers, and industry to improve availability of AI resources for diagnosis and management of COVID-19. This expansion of multidisciplinary collaborative science in support of the worldwide pandemic will open the door more permanently to more concerted and collaborative efforts.
Because teams of radiologists work remotely, there is an increasing need for workflow orchestration by PACS, VNA, and dedicated workflow vendors as well as a need to become even more efficient. We could be on the verge of an evolution/devolution of PACS into an AI-centric combination of independent modules for visualization, analysis, decision support, and reporting.
Artificial intelligence for the assessment and improvement of image quality
There are currently very few objective methods to assess imaging examination quality with only relatively superficial reviews of image quality itself performed by accreditation organizations. The real-time assessment of medical image quality could become an important indicator for patient quality and safety data and even credentialing bodies in the future. Investigations have been performed to evaluate the use of AI to assess the quality of imaging acquisition at the point of care. The examination could then be evaluated either after the study was completed or even during image acquisition to assess and optimize image quality.
There is a trade-off between image quality and parameters such as patient dose, image acquisition time, amount of contrast administered, and other factors. Using a combination of radiologist subjective ratings of overall image quality and indication-specific image quality as well as physics parameters such as image noise, target to background ratio, and contrast and spatial resolution, deep learning algorithms will be trained to predict radiologists’ ratings for the quality of images. Another interesting parameter for image quality will be the performance of AI algorithms for a given imaging technique. An example of this would be evaluating the impact of lower dose CT scans of the thorax using lung nodule detection AI algorithms.
Artificial intelligence as a scribe or personal assistant
Medical scribes have been shown to improve relative value units and provider satisfaction. Human assistants who serve as scribes are starting to be explored in diagnostic radiology as a means of improving efficiency where these assistants play multiple roles in optimizing the speed and quality of care and patient safety. With the use of these assistants, radiologists have been able to more than double throughput. This is largely due to the relative inefficiency of current case retrieval and hanging protocols and limitations of speech recognition technology. Studies performed at the Baltimore VA Medical Center in the 1990s found that only about 15%–20% of a radiologist’s time was spent in the actual review of images; the remainder consisted of the time required for the gathering of patient history and study indication and review of prior reports, image retrieval and arrangement, dictation, and other workflow tasks. The potential for AI to take over a subset or all the functions of these radiology assistants/scribes is intriguing and could represent one of the highest returns on AI investment in diagnostic imaging.
Artificial intelligence for image acquisition
One of the earliest and deepest implementations of AI is already occurring throughout the medical industry. AI as a means of optimization for image acquisition will continue to take advantage of the high level of redundancy in image acquisition systems, especially for MR imaging, CT scans, PET/CT scans, and nuclear medicine. Xu and colleagues found that the inclusion of MR image contrasts into the inputs for AI models could result in acceptable image quality using as little as one 200th of the dataset comparable to taking 3 seconds of data from a 10-minute acquisition. One of the major CT providers uses a library of CT studies reconstructed with both statistical (reconstruction in seconds) reconstruction and model-based (reconstruction in hours) reconstruction to generate the much more resource intensive model-based reconstruction in seconds rather than hours. The implications for scanning time or dose reduction or image quality improvement are intriguing for all imaging modalities and multiple subsequent studies have demonstrated impressive results. Incorporation of deep learning will become ubiquitous in image reconstruction, but new pitfalls and artifacts will be introduced with a wide variety of different applications of deep learning.
Future artificial intelligence will take previous studies into account
It has been suggested that a radiologist’s best friend is the prior images. Unfortunately, virtually all of today’s AI algorithms do not use information from prior studies nor do they create predictions based on trajectory of change over time. This factor represents a major deviation from routine radiology practice, where the goal of the radiologist is often to evaluate for significant interval change and use lack of change to suggest a benign etiology for a finding. Taking advantage of previous imaging studies performed hours or days before in the detection of stroke on a CT scan or previous mammograms in the detection of cancer could result in tremendous improvement in performance on these tasks.
Disease-based multidisciplinary clinical packages
Analogous to a multidisciplinary conference of clinicians, multidisciplinary AI approaches could help in further guiding the diagnosis or management for complex pathology in a more patient-centered manner. In the future, basic capabilities such as the detection of lung nodules or intracranial bleeding will become more of a commodity application while instead there is more of an emphasis on a broad clinical package such as multiple sclerosis, chronic obstructive pulmonary disease, ischemia, heart disease, and others that include detection, diagnosis, quantification metrics, radiophenomics, change over time, and recommendations for treatment and follow-up.
Autonomous artificial intelligence
AI algorithms continue to improve and approach or exceed the performance of a general or even specialty radiologist in certain well-defined areas. The first step toward this will be autonomous reading for a subset of studies of a particular type. This is an outgrowth of the current earlier stage of triage of worklists in which an AI program puts studies for example, of higher suspicion for intracranial bleed on a head CT scan at the top of a radiologist’s worklist. The next logical step will be for AI to perform final reads, but only when it has a high level of confidence in its diagnosis.
Recently, there have been considerable improvements in the performance of algorithms used to detect malignancy in mammography. Kyono and colleagues recently evaluated the use of deep learning in a dataset of more than 7000 women who were recalled for assessment as a part of the National Health Service screening program in the UK. They found that their AI algorithm could maintain a comparable with or better than human-level negative predictive value performance of 0.99 for an algorithm selected 34% of mammograms when the prevalence of disease was 15% (the algorithm picked the 34% to interpret to achieve that negative predictive value) and could achieve that 0.99 negative predictive value negative predictive value for 91% of mammograms when the prevalence of disease was 1%. This ability to select studies highly likely to be negative will be used differently throughout the world. In countries such as the United States, for the foreseeable future and given regulatory and billing concerns, this will represent a triage mechanism to allow radiologists to review higher yield studies more carefully in comparison with a less comprehensive review of lower yield studies. In other countries, this technology will be more likely to be used to prescreen which studies are selected for human versus computer only interpretation.
Challenges posed by the adoption of AI in medical imaging have been well-described and include the patient care and ethical implications of bias in datasets selected to train AI algorithms, misapplication of AI based on a lack of understanding of the narrow focus of an algorithm, the use of AI to create false images with, for example, tumors removed or inserted into imaging studies, perceived or actual loss of value of human radiologists and threats to jobs, large-scale security breaches, and AI performing a different task than intended owing to the black box lack of visibility into what the AI system is actually doing. A current real threat is that the perception of AI as a threat itself is likely steering a subset of misinformed medical students away from the specialty of diagnostic imaging.
Next-generation technologies in artificial intelligence
Deep learning will continue to evolve. The time-consuming and resource-intensive process of labeling data will encourage hybrid learning models in which one combines supervised and unsupervised learning. An example of this is the use of a semisupervised generative adversarial network achieving performance comparable with that required with hundreds of cases using only 25 samples. This improvement is based on the idea that, in learning to discriminate between real and synthetic images, a generative adversarial network can learn structures without concrete labels. Composite learning seeks to combine knowledge from multiple models. Transfer learning is one example of this methodology, as are generative adversarial networks and adversarial learning in which the performance of one model can be represented in relationship to that of others. Ensemble methods that combine multiple algorithms designed to solve the same problem using different approaches are another example. Finally, reduced learning approaches are becoming more sophisticated in creating lightweight AI, which creates smaller neural networks without the loss of performance to effectively run deep learning algorithms on devices such as smartphones, portable radiographs, ultrasound examination, or MR imaging systems.
AI systems of the future will need to be less reliant on large numbers of training sets, be more easily understandable (explainable AI rather than black box), and will need to be more efficient in transferring learned knowledge. Alternatives and improvements such as adaptive resonance theory, also referred to as incremental learning, which does not require retraining to incorporate new learning; or cogency maximization, which is less computationally intensive and addresses the explainability issues with deep learning; and fuzzy sets systems, which allows data to be represented as probabilities rather than just true or false or as a set numeric value, represent future advances in deep learning.
Beyond deep learning and artificial intelligence
As Wang and Yeung observed in their seminal paper, Toward Bayesian Deep Learning: A Framework and Some Existing Methods, next-generation AI systems will go beyond seeing, reading, and hearing to thinking. They foresee a Bayesian deep learning framework in the future and try to integrate them into a single probabilistic framework. This work will likely be a fruitful direction because so many tasks, especially in health care, require a combination of perception from neural networks, but also inference ability from probabilistic graphical models, which are described in this integrated framework. The combination of deep learning with Bayesian inferencing has the potential to significantly augment the utility of computers to help radiologists to not only observe but to also provide important insights to contribute impactfully for patient care.
Summary
Dennis Gabor’s observation that futures cannot be predicted, but futures can be invented, underscores the importance of radiologists serving as active players in the continuing evolution of AI in diagnostic imaging to enable us to determine our own future.
Ultimately, AI represents a tool with incredible potential for us to, once again, reinvent the practice of diagnostic imaging in a way that participates as fully as possible in the emerging health care ecosystem of data and decision support algorithms, to make us more efficient, reduce stress and burn-out, increased accuracy in diagnosis and follow-up recommendations, and improve communications and patient safety.
AI will undoubtedly make radiology more impactful and a more attractive specialty for potential trainees while making it an even more fascinating and wonderous subspecialty in health care.
Clinics care points
- •
The most important attribute of AI systems in medicine is their trustworthiness.
- •
Non-pixel based artificial intelligence applications will be developed in parallel to pixel-based ones.
- •
Radiologists must be actively involved in defining the future of AI in medical imaging.
- •
AI will undoubtedly make radiology more impactful and a more attractive specialty.
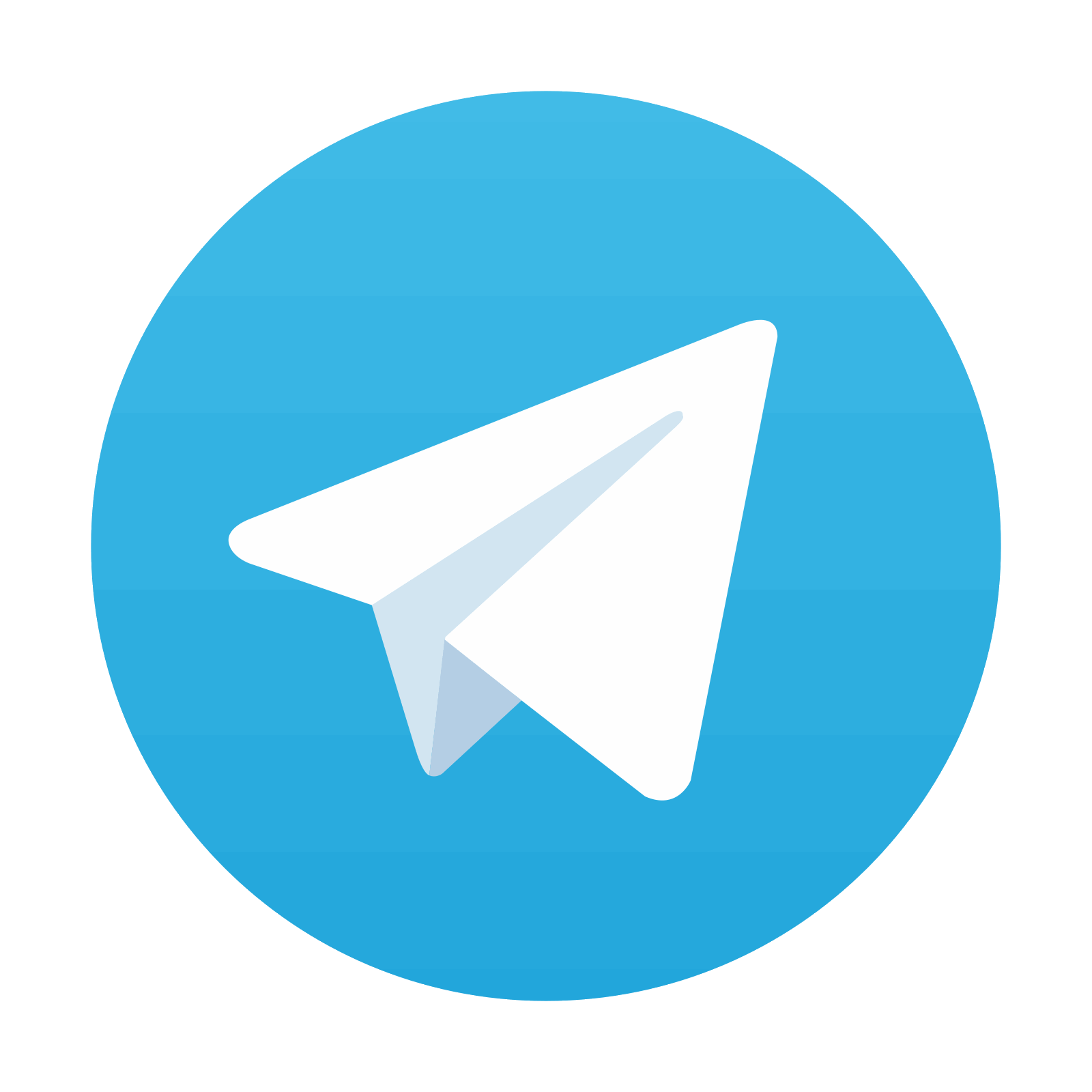
Stay updated, free articles. Join our Telegram channel

Full access? Get Clinical Tree
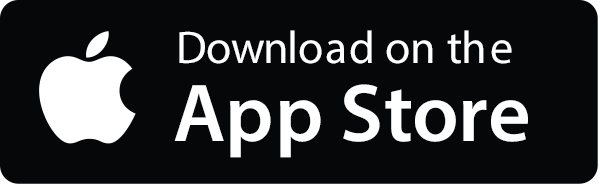

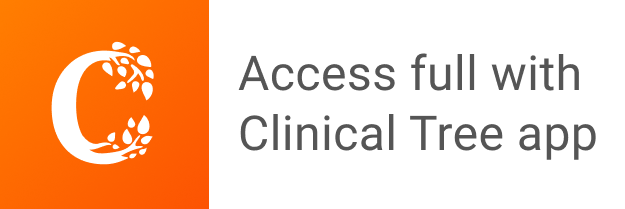