Artificial intelligence technology promises to redefine the practice of radiology. However, it exists in a nascent phase and remains largely untested in the clinical space. This nature is both a cause and consequence of the uncertain legal–regulatory environment it enters. This discussion aims to shed light on these challenges, tracing the various pathways toward approval by the US Food and Drug Administration, the future of government oversight, privacy issues, ethical dilemmas, and practical considerations related to implementation in radiologist practice.
Key points
- •
The FDA does not currently possess an AI-specific regulatory paradigm, but instead applies its risk stratification framework for medical devices.
- •
In the coming years, the FDA may turn to a total product lifecycle approach to regulating AI products, similar to cGMP.
- •
Integrating AI products into radiology workflow patterns may require changes to reimbursement, interoperability, data security, and training patterns.
- •
AI is only as robust as the data it trains upon; high-quality, diverse, and adequately labeled datasets are essential to its success.
- •
Questions of liability in a clinical AI context are complex and remain unresolved.
Introduction
The past decade has borne witness to a remarkable transformation—the evolution of artificial intelligence (AI) technology from engineering novelty to revolutionary instrument. Having emerged from a desultory “AI winter,” it now proliferates across multiple industry segments and extends deep through supply chains, enabling innovations as far afield as high-frequency trading, autonomous vehicles, personality computing, and mass surveillance.
Health care has not escaped its sweep; AI technologies are increasingly gaining traction from drug discovery to surgical planning, to the extent it has earned the moniker “the stethoscope of the 21st century.” These developments are of particular salience to radiology, a data-intensive field leveraging pattern recognition, prognostic estimation, spatial modeling, and disease surveillance. Indeed, more AI products have been approved in the United States for radiologic application than for use in any other medical specialty, and many of these innovations originate from within imaging departments themselves. ,
Despite its promise, AI technology exists in its nascency and remains largely untested in the clinical space. This nature is both a cause and a consequence of the uncertain legal–regulatory milieu it faces. The following discussion aims to shed light on these challenges, tracing the various pathways toward the US Food and Drug Administration (FDA) approval, the future of federal oversight, privacy issues, ethical dilemmas, and practical considerations related to implementation in radiologist practice.
Surveying the regulatory landscape: An alphabet soup
Existing Pathways
At present, the FDA adopts a functional approach to the review, approval, and postmarket surveillance of AI technologies; no purpose-built regulatory framework specific to AI as yet exists. These products are assessed through standard paradigms, which primarily consider their (1) risk profile and (2) intended clinical use in determining the appropriate level of scrutiny.
The threshold question is whether or not a given AI-enabled product constitutes a medical device. In relevant part, the federal Food, Drug & Cosmetic Act defines this term as “an instrument . . . intended for use in the diagnosis. . .or in the cure, mitigation, treatment, or prevention of disease . . . which does not achieve its primary intended purposes through chemical action.” More specifically, the FDA incorporates by reference the International Medical Device Regulators Forum definition of Software as a Medical Device, which includes “software intended to be used for one or more medical purposes that perform these purposes without being part of a hardware medical device.” Should these conditions be met, 3 available pathways exist (ranging from class I to class III in increasing order of rigor) distinguished by the scope of patient risk exposure, each with differing data submission requirements. Premarket approval (to which most class III devices are subject) represents the most stringent classification; absent a showing to the contrary, this is the default level of review accorded to de novo applications and requires clinical studies to demonstrate safety and efficacy. A successful submission through this method confers FDA “approval” of the software in question.
Depending on the characteristics of the device, class I and II submissions may trigger the less comprehensive premarket notification, also known as 510(k). This process culminates in the device’s FDA clearance, as opposed to approval; although devices processed via either pathway may be marketed, this distinction is critical for the purposes of accurate labeling. Premarket notification requires a showing of “substantial equivalence” to a previously approved predicate device, in many cases simplifying the application and review processes considerably. However, this designation is unlikely to applicable to many groundbreaking AI technologies that lack preapproved predicates owing to their novelty. The FDA has spoken directly to its classification of radiologic image analyzers and distinguishes between computer-assisted detection and computer-assisted diagnosis devices. It defines the former as tools that “identify, mark, highlight, or in any other manner direct attention” to features of a radiologic study, rather than those that autonomously drive diagnosis or staging. Commensurately, the FDA has designated a number of computer-assisted detection products as class II devices while maintaining the heightened level of class III scrutiny for computer-assisted diagnosis technologies.
The 21st Century Cures Act (hereafter “Cures Act”) amends the Food, Drug & Cosmetic Act to establish a number of exemptions to the statutory definition of “device,” and by extension, from the purview of FDA device regulation. Per its §3060(a), these include products (1) intended for administrative support, or used in (2) the maintenance of a healthy lifestyle, (3) electronic patient records, (4) storage or display of clinical data—and critically for radiology—(5) “unless the function is intended to acquire, process, or analyze a medical image . . . for the purpose of (i) displaying, analyzing, or printing medical information . . . (ii) supporting or providing recommendations to a health care professional about prevention, diagnosis, or treatment and . . . (iii) enabling such health care professional to independently review the basis for such recommendations to make a clinical diagnosis or treatment decision regarding an individual patient.”
The FDA has clarified the scope of these provisions in twin guidance documents, nonbinding policy statements delineating the FDA’s interpretation of the law and articulating enforcement priorities. In Clinical Decision Support Software (CDS Draft Guidance), the FDA recognizes “independent review” as the critical operative term of the Cures Act’s exemptions, establishing that health care professionals “be able to reach the same recommendation . . . without relying primarily on the software function” for the device to escape formal regulatory oversight. The FDA furthermore requires developers to disclose the “purpose or intended use of the software function, the intended user (eg, ultrasound technicians, vascular surgeons), the inputs used to generate the recommendation (eg, patient age and gender), and the rationale or support for the recommendation.” That said, it remains to be determined at the time of writing how the FDA will interpret the sufficiency of said “rationale or support” and whether regulatory bodies possess a legal “right to explanation.” Developers, for their part, may prove hesitant to reveal trade secrets in what is already a burgeoning, competitive market. However, failure to disclose these potentially sensitive details hazards classification of their product as a “device,” outside of the ambit of the §3060(a) exceptions and subject to a substantial regulatory burden. Developers are thus confronted with a dilemma: either reveal proprietary details of their product for accelerated regulatory approval or rely on opacity to preserve their competitive advantage. The situation is only further complicated in the cases of deep learning or “black box” algorithms, for which an accurate description of a continuously learning internal mechanism may prove a practical impossibility. Beyond mere compliance with the terms of the Cures Act, such technologies lay bare the shortcomings of existing regulatory regimes, conventional clinical trial design, and the current state of postmarket surveillance mechanisms as applied to sophisticated AI products.
Through a second guidance document, Changes to Existing Medical Software Policies (Changes Guidance), the FDA recapitulates its principles of enforcement discretion with respect to the aforementioned exemptions. In brief, the FDA aims to focus its regulatory efforts on those products displaying patient-specific data and “alert[ing] a caregiver to take immediate clinical action” based on clinical parameters.
Future Directions
The unique complexities of Software as a Medical Device products beckon novel approaches to regulation. This has not been lost on the FDA, which in 2017 established a pilot program for Software as a Medical Device precertification as part of its Digital Health Innovation Action Plan. Known as Pre-Cert 1.0, it looks to features of the developer, rather than the product, in a total product lifecycle approach. This derives inspiration from the current good manufacturing practices paradigm, which the FDA requires device manufacturers to comply with. A number of service providers (to include Apple, Fitbit, and Verily, among others) currently participate, invited after having demonstrated “a robust culture of quality and organizational excellence, and a [commitment] to monitoring real-world performance.” The program continues as of 2020; however, it has not yet expanded beyond its 9 initial participants and the FDA acknowledges that its expansive breadth may require statutory authorization. Nonetheless, it offers key insights into what a potential AI regulatory regime may resemble.
The FDA released its Proposed Regulatory Framework for Modifications to Artificial Intelligence/Machine Learning-Based Software as a Medical Device (Discussion Paper) in April 2019, presenting a wholly novel schema and seeking input thereof. At its core, it underscores the inadequacy of existing regulatory architecture to govern continuously learning algorithms. Would an approved AI device require 510(k) clearance after each autodidactic “modification?” When do said modifications translate into clinical significance? Which qualitative factors would be relied on in making this determination? Should devices be required to maintain logs of each decision path? At what point would an adaptive algorithm accumulate so many modifications that it may no longer be credibly termed “substantially equivalent” to its previously approved form? Conventional frameworks largely leave these unanswered, although the FDA has provided some direction into answering the first of these questions, albeit within the limited context of locked algorithms.
By contrast, the Discussion Paper advocates for a flexible total product lifecycle–based design control model borrowing from the Pre-Cert 1.0 program. It analyzes developer characteristics with an eye to establishing “good machine learning practices” and encourages cooperation with the FDA across the length of a product’s lifespan. The FDA draws particular attention to the critical role of high-quality data, emphasizing consistency, generalizability, and the maintenance of distinctions between training, tuning, and test datasets. Performance data are to be shared routinely with the FDA, which in turn would be well-placed to provide dynamic regulatory assessments from development to postmarket performance monitoring.
Turning to features of the product itself, the Discussion Paper calls for a multiaxial approach to risk stratification incorporating the significance of the information the product offers to the health care decision, the state of the health care condition, and whether or not the algorithm involved is locked or adaptive. Developers are moreover to anticipate modifications ex ante and establish protocols to govern future algorithm change. These modifications would then be categorized into one or more of the following categories requiring tailored premarket review: (1) performance modifications with no change to input or intended use (eg, training on new datasets), (2) input modifications with no change to intended use (eg, developing interoperability, including new data types), and (3) modifications to intended use (eg, application to new patient populations or pathology). Such an approach aims to balance an appropriate degree of regulatory oversight with the flexibility necessary to encourage continued innovation.
Implementation challenges: The growing pains of artificial intelligence expansion
Investment and Integration
Securing regulatory approval is one matter, but adoption another entirely. This section concerns the practicalities of implementing AI technologies in real-world settings. For one, financial considerations hinder ready incorporation into existing infrastructure. A recent survey of hospitals and imaging centers confirms this finding, revealing cost as the primary impediment to adoption, followed by a “lack of strategic direction.” Software price tags only tell part of the story; factoring in the costs of annual licenses, servers, and the sophisticated hardware products necessary for deep learning applications, to say nothing of training and technical support service fees, complete AI packages could easily range into the hundreds of thousands of dollars. It is unclear how this additional expense would figure in the broader payor environment. Would the costs of AI-enabled radiologic studies be passed on to patients—the final consumers in this picture? As a further twist, these present costs must be weighed against speculative and difficult-to-quantify future savings in the form of increased radiologist productivity, enhanced workflow efficiency, and the averted costs of downstream treatment. In addressing this issue and incentivizing the use of AI, there may be a role for novel reimbursement mechanisms to include new Current Procedural Terminology codes and modernized radiologist relative value unit schedules. ,
Radiologic applications of AI moreover face a rapidly evolving landscape and remain in a relatively early stage of development. As a strategic matter, health care institutions may opt to wait until the field matures further and more advanced alternatives become available before incurring the costs of implementation. This tactic would enable them to exploit second-mover advantage rather than bear the risk of investing in first-generation products that may quickly approach obsolescence. The field also faces a plethora of competing products across the value chain, raising a number of interoperability concerns. For example, image analysis, triaging, and report generation algorithms may derive from different developers, interface with different servers, and lack a common means of integrating with a given imaging department’s workflow pattern. Establishment of a vendor-neutral framework is thus an integral step in avoiding process fragmentation and facilitating AI adoption. To this end, the Digital Imaging and Communications in Medicine Working Group 23 has been tasked with addressing interoperability challenges; once finalized, its recommendations may offer an avenue forward. The state of existing AI-enabled imaging technology is moreover hampered by a marked user unfriendliness. It stands to benefit from streamlined user experience optimization, perhaps through full integration with picture archiving and communication systems, facilitating adoption, operator training, and troubleshooting.
Paths to incorporating AI tools into existing image analysis protocols may assume a variety of forms. One study presented 5 options along a sliding scale toward increasing integration: (1) maintaining an AI-specific workstation electronically separate from picture archiving and communication systems; (2) establishing built-in image processing functions that automatically interface with picture archiving and communication systems; (3) deploying multiple programs in parallel, whose output is synthesized on a purpose-built integration framework; (4) investing in multiple, mutually interoperative AI products requiring no third-party platform for integration; and (5) full-spectrum integration directly linked to the electronic health record. There is no one-size-fits-all approach to AI implementation; each of these options bears its own combination of advantages and drawbacks. Rather than treating this list as a spectrum of technological sophistication, it may be more appropriate to consider the specific resource demands of the institution contemplating AI expansion. The 5 options are suited to particular uses (eg, patient volume, deployment in imaging centers vs hospitals, general vs specialty practices) and impose variable capital requirements, both of which inform financial decision-making at the level of the individual group.
Clinical Validation
Clinical validity distinguishes mere technological curiosity from bona fide adjunct to patient care. Although a number of radiologic AI products have indeed proven revolutionary, this fact alone cannot be taken for granted. For instance, computer-assisted detection mammography became a nearly ubiquitous fixture of breast imaging in the first decade after FDA approval. Across the same span, a breakthrough study revealed that cancer detection sensitivity actually decreased among certain segments of radiologists who used this technique. Although the outcome was likely multifactorial in origin, it suffices to demonstrate that AI technology, by itself, is no panacea. In a more modern iteration, rule-out algorithms display a number of similar shortcomings when applied to real-world clinical environments. For example, those designed for the rapid detection of intracerebral hemorrhage may function well for the task at hand, but neglect to account for the no less acute ramifications of a negative result, to include ischemic stroke or an inflammatory process. Contemporary AI technologies reveal a limited ability to contextualize findings. They furthermore lack the capacity to approximate radiologist judgment calls regarding overdiagnosis; by their inherent overinclusive qualities, they may paradoxically contribute to a local increase, rather than a decrease, in medical expenditures.
At present, the development of radiologic AI applications is limited by the availability of use cases with well-annotated inputs and standardized outputs. This issue turns on both the quality and quantity of data, although both are interrelated; high-quality output scales with data, particularly for deep learning algorithms. Additionally, large training datasets are required for sensitive and specific detection of low-prevalence pathologies. Yet consideration of volume does not necessarily reveal the full picture. This point is critical to note, because extrapolation from clinically unrepresentative datasets, however large, may introduce confounding variables and amplify noise. The importance of a drive toward externally validated training datasets containing generalizable information can, therefore, not be understated. Worrisomely, a 2019 meta-analysis indicated that only 6% of published AI studies under review performed external validation. These issues may in part find remedy by the establishment of open-source data repositories in the mold of the National Institutes of Health All of Us Research Program, drafting of multi-institutional data-sharing agreements, expansion of federated learning techniques, and research shift toward multicentric trials. These agreements would stand to offer further usefulness from implementation of the FAIR Guiding Principles, which offer a scaffold for data stewardship through “findability, accessibility, interoperability, and reusability.”
Privacy and Ethical Issues
The sheer scale of patient data used in AI applications invites a number of concerns related to ownership, remuneration, privacy, and liability. At present, there is limited federal direction regarding the former. The Health Insurance Portability and Accountability Act establishes a patient right to inspection over their own medical records, but does not otherwise confer ownership rights. Similarly, high court jurisprudence is largely silent on this matter; in the closest analog, the Supreme Court invalidated a Vermont statute prohibiting the commercial use of physicians’ prescribing records on First Amendment grounds. The issue has thus devolved upon the states, among whom only one—New Hampshire—expressly provides for patient ownership of data. Of the remaining states, 28 do not speak to control and the remaining 21 consider providers, or provider organizations, the ultimate owners of medical information.
A well-cited Hastings Center study articulated a novel framework in which users as well as principals of a health care system bear the ethical imperative of improving it. Some scholars have further expanded on this line of reasoning, contending that fiduciary duties are incumbent upon secondary users of clinical data (as distinguished from primary users who leverage patient information to provide patient care directly). , They establish a new theory of property envisioning these users as stewards of a public good, rather than proprietors outright, maintaining that it would be unethical to “sell” such data for profit. The theory does not explicitly require patient consent as a precondition for its use, given that downstream security measures are taken. Although seemingly heterodox, this is certainly not an isolated view in the bioethical community, particularly when consent would be impracticable or unduly burdensome to obtain. In any event, federal regulations consider retrospective review of de-identified clinical data as exempt from traditional patient consent requirements, provided that the “information … is recorded by the investigator in such a manner that the identity of the human subjects cannot readily be ascertained directly or through identifiers linked to the subjects, the investigator does not contact the subjects, and the investigator will not re-identify subjects.” That said, federal waiver of the consent requirement may do little to thwart interlopers from attempting to re-identify anonymized medical information, particularly from digital reconstructions of imaging studies. The custodians of clinical data (AI developers, radiology centers, hospitals, and server administrators, among others) must therefore remain vigilant against any possible intrusions from malign, naïve, or lax actors. , Decentralized federated learning models and even blockchain solutions have been proposed to supplement traditional cybersecurity measures in protecting data integrity.
There remains the risk that AI technologies would only benefit those whose data are accessed. More concerningly, algorithmic bias bears the potential of amplifying racial and demographic disparities. Training datasets may not reflect the heterogeneity of pathology across institutional, state, and regional levels and are largely subject to a small pool of individuals’ designations of ground truth. When deployed on a national scale, these AI applications may even unwittingly contribute to implicit discrimination. These realities underscore the importance of maintaining large, robust datasets capturing the diversity inherent within the American health care system and establishing consensus guidelines for ground truth labeling. AI expansion within smaller, rural, or more modestly funded health care institutions presents additional complexities. These may either lack the wherewithal to purchase AI applications or, conversely, invest in AI as a means of expanding their imaging capabilities, but lack the physicians to exercise appropriate oversight. In this manner, its deployment may accentuate outcome disparities inadvertently. There is a significant niche for professional societies to establish guidelines on AI implementation in low-resource settings to dampen these untoward effects.
Liability
Radiologic applications of AI are born into an uncertain liability environment. Owing to both its technological complexity and the multitude of stakeholders involved in its deployment, traditional tort doctrine strains to provide satisfactory answers. Radiologists, their employers in the health care system, and AI developers confront variable degrees of risk exposure and are in turn subject to various legal theories of liability, each of which will be discussed in turn.
As was the case before the advent of AI technologies, radiologists will continue to face medical professional liability under standard negligence principles. Plaintiffs in malpractice suits bear the burden of demonstrating (1) the existence of a duty [of care], (2) a breach of said duty, and (3) that damages were sustained (4) through a causal relationship between the latter 2 factors. Professional society standards and departmental protocols play a major role in establishing a court’s treatment of (1) and (2). Proper integration of AI into radiology workflow patterns and careful drafting of protocol terms are therefore essential.
Health care systems may in turn encounter vicarious liability through the doctrine of respondeat superior , in which the faults of subordinates flow up the chain of agency to attach to principals. Translated into a clinical context, hospitals, imaging centers, and physician groups would thus be responsible for the malpractice of their employees’ use of AI programs. Vicarious liability generally operates according to a “strict liability” basis, rather than on the negligence grounds outlined elsewhere in this article. One may think of this as a legal “on–off” switch, wherein a mere demonstration of harm suffices to trigger liability. Again, these concepts are neither particularly novel nor unique to AI.
The risk of AI developers, together with their interaction with the 2 previously discussed stakeholders, stares into legal terra incognita . It has been postulated that products liability frameworks would govern, although courts have generally not considered software as “products” in a tangible sense. , There is reason to assume that this may change as AI technologies become increasingly embedded within physical platforms capable of causing bodily harm. If this is so, a negligence framework would apply; however, questions of AI developer duty remain unanswered. Which industry standards would be called on in the absence of an AI-specific framework? Are independent developers subject to different standards of care than multinational tech conglomerates? What of health care institutions who modify the programs they purchase or train them on new datasets? In this sense, AI technologies may prove victims of their own novelty, given the relative juvenescence of the field and sparse body of relevant case law from which to derive judicial precedent. It remains to be determined how courts will interpret fault and causation for continuously learning algorithms. Alternatively, AI developers could be held to principles of strict liability should plaintiffs assert that manufacturing defects inherent to the AI product itself caused their injuries.
These questions are further complicated by the apportionment of liability between these 3 sets of actors. For instance, a sued physician group may indemnify an AI developer in a malpractice suit and accordingly seek compensation. Plaintiffs may moreover sue any one or a combination of these 3 stakeholders in their own right. Qualitatively, AI technology pushes the frontiers of contemporary tort theory, raising novel issues of control, foreseeability, and ex ante quantifications of the magnitude and probability of harm in manners substantially more complicated than with human actors alone.
Clinics care points
- •
Not all clinical AI products require FDA approval; those used for administrative support or record management may be exempt.
- •
Radiology departments and clinics should seek to integrate AI technologies with PACS and the EHR.
- •
Radiologists should receive training in the basics of AI deployment and troubleshooting.
- •
Physicians, administrators, and support personnel must take measures to maintain the security, confidentiality, and robustness of patient data used in AI products.
- •
Radiologists and their employers should maintain standard operating procedures for clinical AI to reduce harm and mitigate liability exposure.
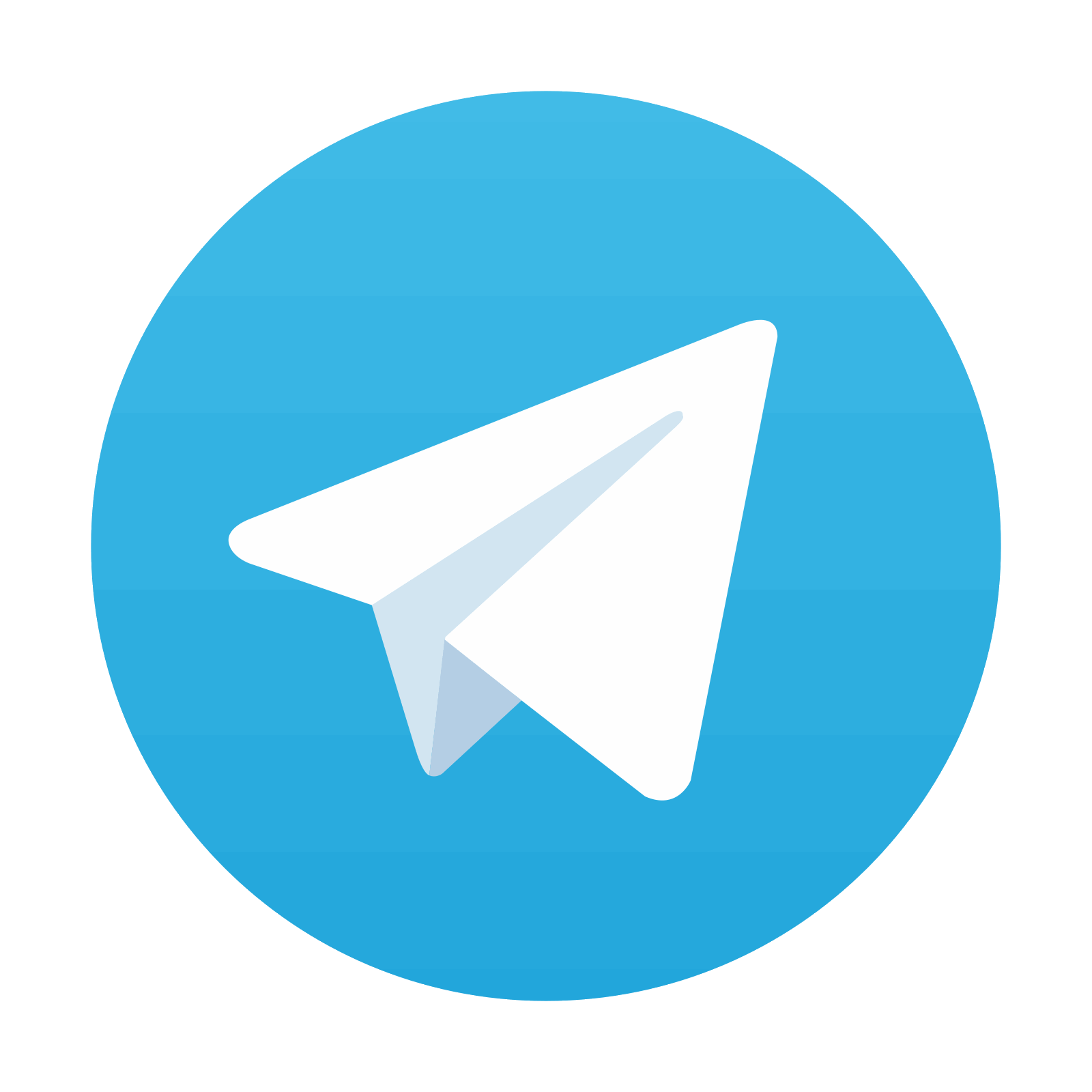
Stay updated, free articles. Join our Telegram channel

Full access? Get Clinical Tree
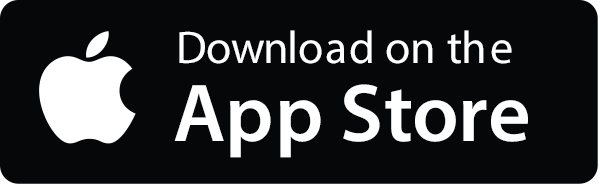

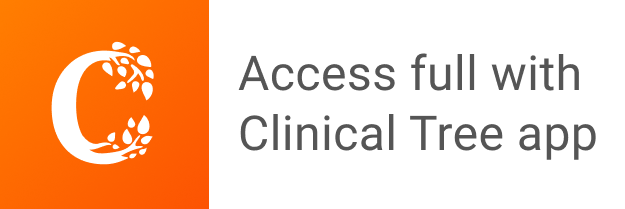