Abstract
Artificial intelligence (AI) is defined as the theory and development of computer systems able to perform tasks normally associated with human intelligence. At present, AI has been widely used in a variety of ultrasound tasks, including in point-of-care ultrasound, echocardiography, and various diseases of different organs. However, the characteristics of ultrasound, compared to other imaging modalities, such as computed tomography (CT) and magnetic resonance imaging (MRI), poses significant additional challenges to AI. Application of AI can not only reduce variability during ultrasound image acquisition, but can standardize these interpretations and identify patterns that escape the human eye and brain. These advances have enabled greater innovations in ultrasound AI applications that can be applied to a variety of clinical settings and disease states. Therefore, The World Federation of Ultrasound in Medicine and Biology (WFUMB) is addressing the topic with a brief and practical overview of current and potential future AI applications in medical ultrasound, as well as discuss some current limitations and future challenges to AI implementation.
Introduction
Aim
Endorsed by the WFUMB, the aim of the paper is to provide a brief and practical overview of current and potential future artificial intelligence (AI) applications in medical ultrasound, as well as discuss some current limitations and future challenges to AI implementation.
Materials and methods
We searched the PubMed and Web of Science databases for all research published in English up to December 1, 2023, using the search terms “Artificial Intelligence” and “Ultrasound.” We also included some narrative and systematic reviews to provide our readers with adequate details within the allowed number of references. In addition to the database searching, a hand search was performed, consisting of the reference lists of related articles and reviews and Google scholar search engines.
The articles were reviewed to determine the relevance based on the following criteria: studies that involved the analysis of ultrasound images by AI, including point-of-care ultrasound, echocardiography, elastography, and contrast-enhanced ultrasound. The articles that were beyond this coverage were excluded. Due to the varying prevalence of different diseases in different body parts and the recommended imaging techniques for diagnosis, ultrasound is less commonly used in diagnostic procedures for skin diseases and lung diseases, while is typically preferred for the diagnosis of thyroid and breast diseases. As a result, there is an uneven distribution of ultrasonic AI research across various diseases. Therefore, for each section, we select only representative literature for discussion and analysis.
All the titles and abstracts for all eligible articles were reviewed by authors independently. If the abstracts were not relevant, then they were discarded, and the full-text articles were accessed. Further reviewing the full-text papers may lead to deserting some irrelevant documents and finally retaining articles that met the inclusion criteria in this review. When there was any discrepancy in the ultimately included articles, a consensus negotiation was reached to form the final inclusion result between the authors.
Summary of artificial intelligence
AI is defined as the theory and development of computer systems able to perform tasks normally associated with human intelligence [ ]. The development of AI over the decades is briefly summarized in Figure 1 . Machine learning (ML) is a subfield of AI that uses various methods to automatically detect patterns in data, improving or “learning” by adjusting internal weights and subsequently, use these patterns to make predictions and/or decisions [ ]. Deep learning is a type of ML that learns to represent complex and abstract concepts in terms of multiple simpler concepts by passing inputs through a large number of layers of interconnected nonlinear processing units [ ]. AI has its own nomenclature and definitions, which can be confusing for those new to the field. Commonly used terms are defined and described in Table 1 .
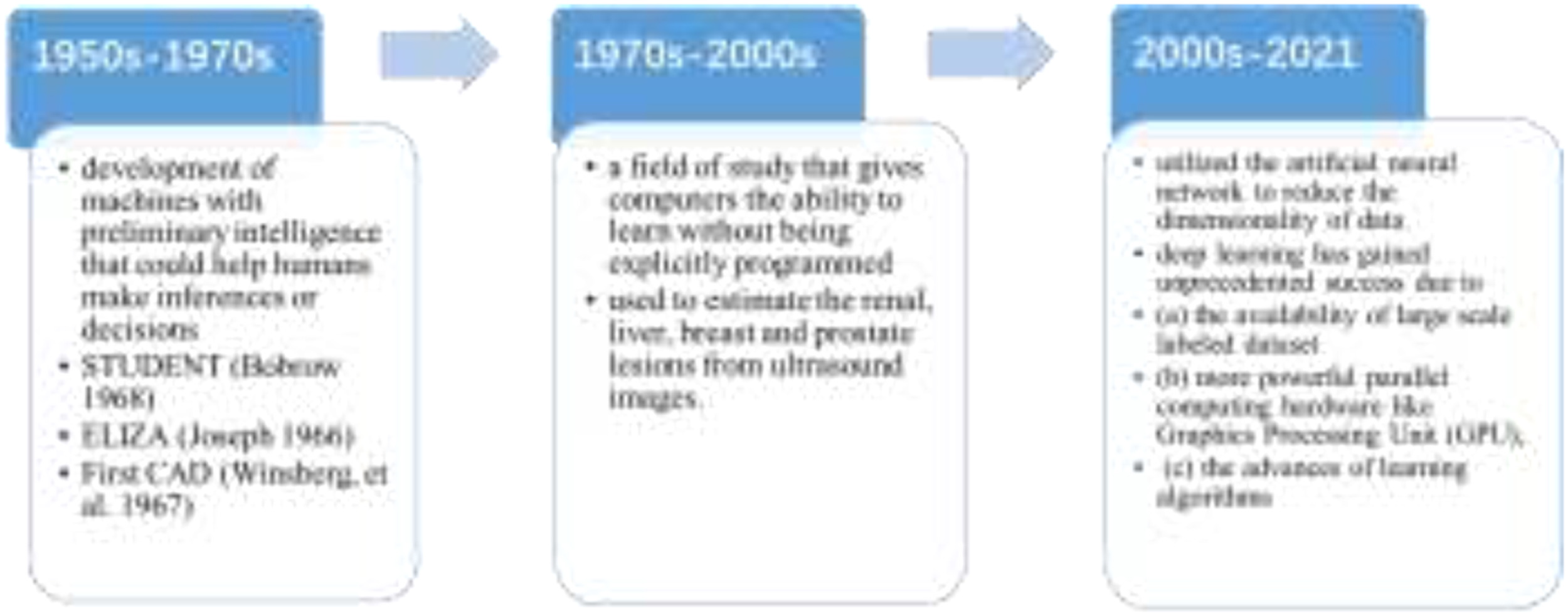
Term | Definition |
---|---|
Narrow AI | AI that can perform prespecified tasks, which may be very complex and specialized, but not other tasks. Includes all of the current AI tools. |
General AI | The hypothetical ability to perform any intellectual task that a human can. Current focus of active research. |
Machine learning (ML) | A subfield of AI that uses various algorithms and techniques to design systems or “models” that learn from data. Learning occurs by repeatedly adjusting internal values to improve the model’s accuracy. |
Deep learning (DL) | A subset of ML that uses a layered structure of interconnected information nodes that was inspired by the neural network of the brain. Responsible for many of the recent dramatic advances in AI, for example image recognition tasks. |
Convolutional neural networks | A type of deep neural network where images are processed to extract features that are passed to “deeper” layers, which are combined to predict the most likely outcome (generally a classification or segmentation task). Has resulted in the dramatic improvement of automated image interpretation. |
Large language model (LLM) | A model that is used to interpret and generate language. May be integrated into many processes or applications to allow users to ask questions or provide answers in “natural language.” |
Natural language processing (NLP) | The field of AI dealing with language as normally used by humans. Allows the input of “normal” speech and writing to the algorithm. |
Supervised learning | A model is trained using known, labelled outputs. Most common technique for training medical imaging models. |
Unsupervised learning | A model does not require labelled datasets. Can determine groupings or hidden patterns. |
Reinforcement learning | Beginning with basic rules, a model learns to make a sequence of decisions to complete a task by succeeding or failing. Suggested may be able to assist with sequential decision making or treatment protocols. |
Transfer learning | A technique that uses models trained on large datasets that are fine-tuned on data specific to the problem. Allows training on much smaller datasets. |
Image segmentation | Dividing an image into different image regions or objects. F or example—selecting the segment of the image that represents the liver or thyroid. |
Radiomics | Methods of extracting quantitative image features from medical images using data-characterization algorithms |
Explainability | The concept that the output (result) from an algorithm output can be understood (by human experts) in terms of its inputs. Lack of explainability implies errors cannot be understood as to “why” they occurred. |
As the field has progressed, the type of data that can be used has evolved. Initially, most algorithms used numerical or categorical data, often referred to as “tabular” data (as it is organized and viewed in tables). The development of multilayered neural networks, called deep learning (DL) networks and convolutional neural networks (CNN) resulted in the remarkable advances achieved in image processing over the last decade where the input is a digitized image [ ]. The relationship between AI, ML, DL, and CNN can be seen in Figure 2 and the architecture of CNN can be seen in Figure 3 . Most recently, the use of deep learning models incorporating a technique called “transformers” as well as learning datasets and computing resources that are orders of magnitude larger than used previously has accelerated the field of natural language processing (NLP) resulting in the development of large language models (LLMs), such as Chat-GPT, that allow the input of “normal” written or spoken language. Different types of data, or the output from different AI techniques can be combined to provide even more accurate models, such as inputting an image to an image model, then combining the output with demographic data (age, gender, etc.) and NLP output (e.g., from medical notes) to provide a final classification or prediction (e.g., likelihood of malignancy or survival) [ , ]. This and other advances will enable greater innovations in ultrasound AI applications that can be applied to variety of clinical settings and disease states.
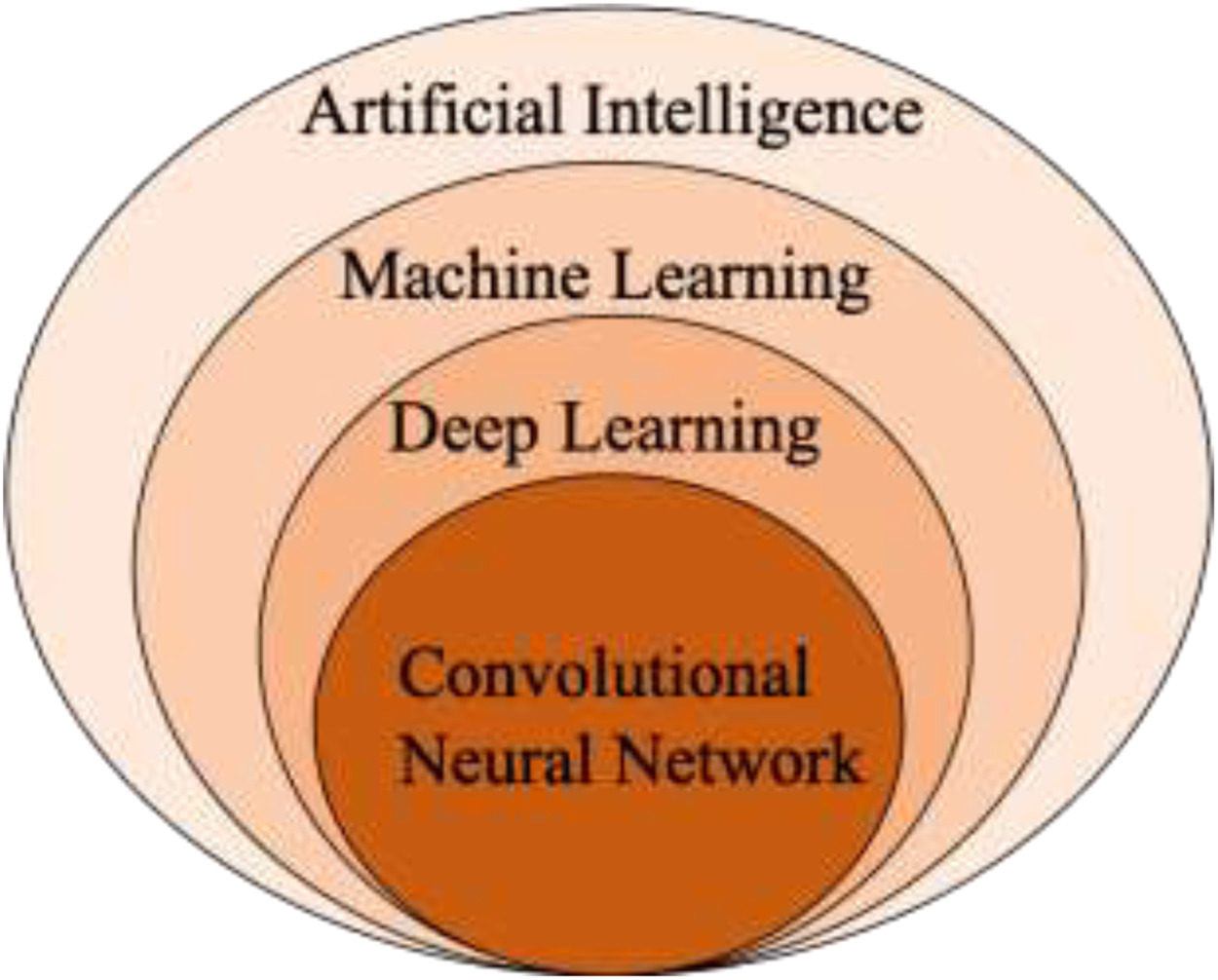
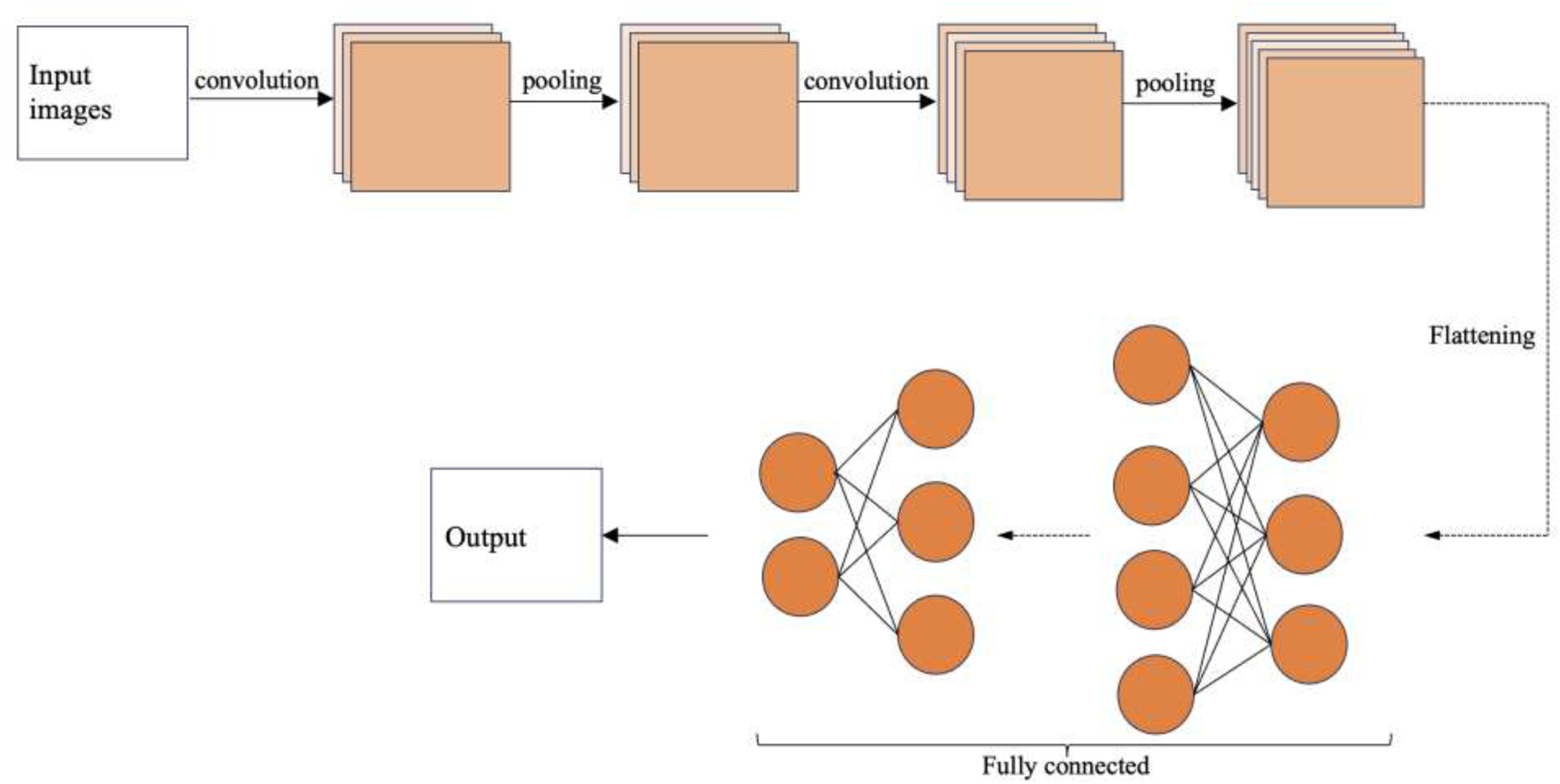
Application of AI in ultrasound image acquisition and interpretation
The characteristics of ultrasound, compared to other imaging modalities, poses significant additional challenges to AI. However, overcoming these challenges may result in greater opportunities than for other imaging modalities due to the potential far reach of ultrasound to almost any setting worldwide. Image acquisition in ultrasound examinations is much more user dependent than other imaging modalities, with the possible exception of automated breast ultrasound systems. Whilst this increases the difficulty in automatically analyzing images, due to lack of standardization, AI offers opportunities to reduce variability during image acquisition, which enables the optimal images can be automatically captured and stored for reporting. Measurements can be automated, as seen in systems from many manufacturers that incorporate at least some measurement automation. The range of applications available for these automated measurement techniques is steadily increasing with, such as selecting which image to take the measurement from during cine loop acquisition, or 3D sweeps [ ]. In addition to reducing variability, such systems can improve workflow by reducing repetitive tasks for operators.
Many pathologies can have similar appearance on ultrasound to the human eye (e.g., the overlapping appearance of many focal liver lesions), especially for less experienced practitioners, resulting in high intra and interobserver variability, thus lowering ultrasound’s utility. Application of AI can not only standardize image interpretation, but can identify patterns that escape the human eye and brain. This may allow AI in the future to outperform even human experts as has already been suggested by multiple AI studies in CT and MRI applications. Currently, there is an extensive (and rapidly growing) body of research comparing algorithms to human expert interpretation.
Finally, inclusion of demographic and other clinical information may further improve image interpretation accuracy and can be automatically incorporated into diagnosis, even for relative novices.
Education
In addition to the direct use of AI for patient care, AI systems may be applied to ultrasound education, similar to the growing use of AI in other fields of education. One of the main limitations to the more widespread use of ultrasound, especially by novice users, is the lack of instructors [ ]. Automated learning systems may be able to provide specific, individually tailored, real-time teaching of image acquisition and interpretation. For example, AI systems can guide the operator, assisting in obtaining standardized images and indicate when images are adequate for diagnosis [ ]. Additionally, novice users may find it difficult since image interpretation begins with identifying anatomical structures and images may be acquired from nonstandard views. However, algorithms can identify anatomical structures or perform image segmentation, helping novices through the process [ , ]. These educational systems are often easier to bring to market, with little to no regulatory barriers, and are often already created for clinical use and may require little modification for educational implementation.
Application of AI in ultrasound image denoising
During ultrasound imaging, speckle noise caused by sound wave reflection, diffraction, and interference with tissue can lead to such problems as background disorder, uneven brightness, hazy edges, etc., which not only impacts the image quality and thus the diagnosis of lesions, but also affects the process of feature extraction, segmentation, and alignment of ultrasound images by computer technology. There has been a great deal of research in the past to mitigate or eliminate the speckle noise inherent in ultrasound images by using different filters, such as spatial domain filters [ ], transform domain filters [ ], anisotropic diffusion filters [ ], and nonlocal means filters [ ]. These traditional filters have demonstrated a good performance in speckle suppression while preserving edges and details in ultrasound images. Currently, researchers have proposed various CNN-based approaches that focus on developing innovative network models to combat speckle noise [ ]. Content-aware priori and attention-driven techniques, which enables the noise-reducing network model to eliminate scattering noise while maintaining clear lesion boundaries [ ]. The dual-path image denoising network extracts global semantic information through the semantic path and transmits finer pixel features through the pixel path, which can facilitate the information interaction between semantic and pixel features and thus realize the parallel transmission of feature information [ ].
Application of AI in ultrasound image reconstruction
An image reconstruction method that can simultaneously provide high image quality and frame rate is necessary for accurate clinical diagnosis of lesions. The delay-and-sum algorithm is the most common beamforming algorithms used in ultrasound image reconstruction. However, the images produced by delay-and-sum suffer from low contrast and resolution. Currently, Various beamforming techniques based on DL methods have been investigated to improve imaging performance. Deep neural network can be trained to perform image reconstruction to obtain high quality images based on the similar assumptions as delay-and-sum [ ]. Alternatively, training neural network by mapping prebeamformed radio frequency data to a speckle-reduced ultrasound image can reconstruct images [ ]. Moreover, image reconstruction can be achieved by training neural networks to learn a nonlinear mapping from low-quality images acquired at a high frame rate to high-quality images acquired at a low frame rate [ ].
Application of AI in ultrasound image registration
Image registration of ultrasound images with other medical images such as MR, CT, or X-ray images can present anatomical details of tissues, organs, or lesions to help physicians understand the progress of a disease and plan treatment. Deep learning-based image registration methods can be categorized into supervised and unsupervised learning. In supervised learning methods, the image similarity between the registered and fixed images can be assessed by measuring the difference between the predicted and the ground truth transformation matrixes [ , ]. Unsupervised learning methods, on the other hand, directly measure image similarity based on pixel-level intensity information of the image [ ]. In addition, a weakly supervised strategy using high-level labels representing anatomical structures was developed to measure the correspondence of multimodal images to assess image similarity and guide the learning process [ ].
Applications of AI in ultrasound
Point-of-care ultrasound (POCUS)
Point-of-care ultrasound (POCUS) refers to “ultrasound performed at the bedside an interpreted directly by the treating clinician.” It therefore covers a wide range of uses and users, who may range from experts to novices, with the majority being less experienced than those traditionally thought of as “ultrasound operators” (e.g., radiologists, sonographers, or sonologists). In fact, the vast majority of potential POCUS users worldwide are complete novices with no ultrasound experience. This fact amplifies the operator dependence and variability that already exists in ultrasound imaging and poses a significant challenge and opportunity for developers of POCUS AI applications. Most manufacturers have already recognized that prior to an interpretation, an application is needed to ensure the POCUS operator obtains the correct images, either through outright guidance (e.g., direct instruction on moving the probe), image quality grading and feedback or a combination of these. Such systems have been shown to be able to guide novice users to provide accurate results [ , , ]. Most POCUS AI applications target a focused assessment, fitting the nature of diagnostic POCUS studies which are typically binary and seek to answer specific questions. Ultrasound guidance in procedure performance is often attempted by relative POCUS novices (who may be experienced in the nonimage guided procedure). To assist, algorithms have been developed to identify key structures in the arm to assist identifying and targeting vessels for cannulation under ultrasound [ ]. Combination robotic, ultrasound and AI devices have been developed to guide novice users to cannulate vessels. Several researchers tested the device with users on gel phantoms and pig models using hypotension to mimic difficult clinical conditions. Cannulation was achieved rapidly and successfully in multiple trials [ ].
AI applications in POCUS are potentially as varied as its many uses. However, the most important work concerns bridging the gap between lack of ultrasound skills by novices and the need for patients to have expert image acquisition, interpretation and procedure guidance. Future AI application development will be driven not only by attractive diagnostic and guidance targets, but also by the need to guide the operator`s hand in a majority of cases worldwide.
Echocardiography
Echocardiography is an area of ultrasound that seems particularly well suited to the development of AI based applications. Many institutions have datasets spanning decades that contain tens of thousands of images, use standardized views, and have been quantitatively analyzed by experts [ ]. AI algorithms exist to assist almost every step of the echocardiography process, including guiding the user to obtain better images, assessing image quality to ensure adequate images are obtained, enhancing images to improve quality, performing automated measurements (freeing the clinician from repetitive measurement tasks and improving workflow), and interpreting images and findings to make the final diagnoses [ ]. AI has been successfully applied to classify echocardiogram views, perform cardiac segmentation, and assess echocardiogram image quality [ ]. Once segmentation has occurred, further analysis can assess chamber volumes and function. Left ventricular ejection fraction (LVEF) is currently one of the most important indicators of cardiac function. Manual calculation of LVEF can be cumbersome and difficult for the novice. There is also significant interobserver variability in measurement [ ]. A number of AI algorithms have been shown to be highly accurate for estimating LVEF [ , ]. Regional wall motion abnormality is important for the diagnosis of cardiac ischemia but is difficult for nonexperts to identify and subject to high inter-rater variability. DL approaches have been successful in identifying regional wall motion abnormality, with an accuracy approaching experts [ ]. Analysis of right ventricular (RV) function can be challenging but is now being evaluated by AI algorithms [ ]. AI approaches have been successfully applied to automatically identify and characterize multiple other aspects of echocardiograms including hypertrophic obstructive cardiomyopathy, pericarditis, pulmonary hypertension, and amyloidosis [ ]. There is also interest in applying AI to the analysis of 3D echocardiograms [ , ]. Analysis of existing echocardiogram parameters by AI algorithms may be better able to predict mortality than traditional risk stratification tools [ ]. Further models can provide information not usually identifiable (such as age and gender) or make assessments in the absence of information that is usually considered essential (e.g., estimating severity of aortic stenosis without left ventricular outflow tract velocity or dimension [ ]).
Liver disease
Globally, hepatocellular carcinoma (HCC) is a frequent fatal malignancy, the sixth most common cancer, and the third-leading cause of cancer-related mortality in the world [ ]. Ultrasound is the first-line medical imaging modality for the detection and diagnosis of focal liver lesions (FLLs) but is limited both by its operator dependent nature and the considerable overlap in ultrasound appearance of different pathologies. AI models have been developed to classify FLLs according to the Liver Imaging Reporting and Data System (LI-RADS) classification [ ], distinguish benign from malignant lesions with an accuracy that matched CT [ ], distinguishing primary from metastatic liver malignancy [ ], and to predict malignant subtyping and microvascular invasion in HCC [ , ]. Both B-mode ultrasound (BMUS) and contrast-enhanced ultrasound (CEUS) have been used to predict outcome and response to ablation [ , ]. For diffuse liver disease, deep learning radiomics of elastography (DLRE) were also used for assessing liver fibrosis stages in chronic hepatitis B [ ]. Nonalcoholic fatty liver disease has also been studied with reports of high accuracy using different AI approaches [ ].
Acute appendicitis
Acute appendicitis is one of the most common causes for emergency surgery worldwide and ultrasound is recommended as a first line imaging modality [ ]. However, it remains a diagnostic challenge [ ]. AI has been used to identify appendicitis from ultrasound images [ ], and to predict the diagnosis based on a combination of clinical, laboratory, and ultrasound findings [ ]. Notably, when the AI failed to accurately detect the appendix, it negatively affected the evaluators’ confidence in their diagnosis [ ]. Undeniable, combining scan guidance for novices with image identifying AI can greatly expand patient access to diagnostic ultrasound for acute appendicitis. Therefore, it is important to consider the limitations and strategies when AI assists in clinical decision making in order to minimize the potential negative impact on clinical decision making.
Surgery and trauma
Focused assessment with sonography in trauma (FAST) is probably the most frequently utilized ultrasound technique worldwide for triaging of the unstable trauma patient and may often be performed by clinicians with limited imaging expertise. Models have been developed to assess the adequacy of views and interpretation of images with accuracies greater than 90% [ ]. On the other hand, AI can assess the quality of the FAST exam to reduce expert time and save costs, as quality assurance is a core process for all ultrasound activities in the emergency department, following the guidelines of the American College of Emergency Physicians, the Clinical Ultrasound Accreditation Program, and the American College of Surgeons’ Quality Improvement Program for Trauma [ ]. Additional AI application development for image interpretation coupled with guidance for novice operators could lead to greater standardization for FAST examinations [ ] and improved sensitivity and specificity for intra-abdominal free fluid detection.
Inflammatory bowel disease
A CNN based deep learning model has been trained to detect bowel wall thickening (>3 mm) which is used as a surrogate of bowel inflammation [ ]. Although tested in a porcine model only, researchers achieved an area under the ROC curve as high as 0.978 and suggested their approach could enable inexperienced operators to use ultrasound through automated detection of inflammatory bowel disease, while at the same time leading to better standardization in image interpretation. In another study, ultrasound radiomics combined with complete blood cell count and serum biochemical biomarkers for inflammatory bowel disease assessment in cats broadening the study of AI interpretation of gastrointestinal ultrasound images [ ]. The diagnostic accuracy of inflammatory bowel disease using ultrasound radiomics exceeds 90% expanding the capabilities of ultrasound research in inflammatory bowel disease. [ ].
Gallbladder
Gallbladder polyps are common findings, which although usually benign, can have overlapping appearances with malignant polyps [ ]. Models have been used to differentiate benign and malignant lesions, some that incorporate demographic information [ , ] and therefore could lead to better risk prediction. Based on endoscopic ultrasound images, AI showed good performance in distinguishing gallbladder polyp lesions, with a diagnostic accuracy of 89.8% [ ]. AI-assisted diagnosis can help inexperienced novices improve accuracy in diagnosing gallbladder polyps [ ].
The diagnosis of biliary atresia (BA) from gallbladder US images remains a challenge, especially in rural areas with limited expertise. In order to solve the problem, Zhou et al. developed an ensembled DL model that yielded expert level performance, including on smartphone images and video sequences [ ].
Renal
Ultrasound can be the test of choice for evaluating the kidney and perirenal tissue because it is real-time imaging that is not dependent on kidney function. However, the high subjective variability in image acquisition and interpretation makes it difficult to translate experience-based prediction into standardized practice [ ]. For pediatric patients, a model has been developed to distinguish between congenital urinary tract abnormalities and mild hydronephrosis in children [ ], In adults, models have been developed to distinguish normal appearance from medical renal disease and renal cysts [ ], and predict glomerular filtration rate (GFR) from renal images [ ].
Prostate
Transrectal ultrasound (TRUS) is used to evaluate prostate malignancies and infections. It is used both diagnostically and to guide procedures, such as biopsy and the placement of radioactive sources for brachytherapy. DL networks have shown good ability to accurately segment the prostate [ , , ]. AI models have been used to guide prostate biopsy, using combinations of BMUS, elastography, CEUS, and radiomics [ ]. Models have shown the potential to guide brachytherapy [ , ] and improve fusion imaging [ , , ].
Thyroid
Ultrasound is the primary imaging modality for thyroid disease and because thyroid was the target for some of the earliest machine learning exploration, the use of AI for thyroid US is more mature than many other fields. This is reflected in the number of academic manuscripts published and the number of commercial applications that have regulatory approval [ ]. AI models have been developed to select the thyroid from other tissues (referred to as image segmentation) [ ], detect thyroid nodules [ ], and localize and classify nodules [ ] (see Supplement Table 1 ). Most work has been done on classifying benign and malignant nodules, using a variety of techniques [ ]. Imaging techniques utilized include B-mode, elastography [ ], and 3D CEUS [ ] (see Supplement Table 2 ). Some studies have included external validation sets [ ] and compared both AI models performance alone with AI supported reporting. Interestingly, in some cases, particularly in studies using S-Detect, expert performed worse with AI support than without [ ]. Clearly this requires more data and continued evaluation are needed to confirm if these AI models hold true across a variety of practice settings. Other specific applications have examined thyroid tumor grading [ ], calcification detection [ ], immunohistochemical characteristics [ ], BRAFV600E mutation diagnosis [ ], and thyroid follicular neoplasm classification [ ]. For diffuse disease, ML has been used to evaluate lymphocytic thyroiditis [ ] and predict the outcome of Graves’ disease following drug withdrawal [ ].
Breast
Globally, female breast cancer has the highest morbidity and mortality among female cancers [ ]. Ultrasound is widely used in the diagnosis of breast diseases. However, the lack of specificity and high operator-dependency may lead to errors resulting in unnecessary biopsy. AI can be used to reduce interobserver variation. Models are generally based on CNNs and may also incorporate radiomics. AI is mainly used for detection and diagnosis of breast lesions. Use of lightweight neural network structure allows real time detection of lesions during manual scanning [ ], whilst other models show good performance on automatic detection of breast lesions in automatic breast ultrasound (ABUS) images [ ] and 3D ABUS [ ]. Classification of lesions, based on BMUS and color Doppler, according to the BI-RADS classification is accurate and comparable to that of experienced radiologists [ ]. As in other ultrasound applications, AI can extract features that cannot be recognized by the human eye, thereby, helping to reveal more subtle differences between benign and malignant BI-RADS 4A lesions (which have a malignant rate of 2%–10%, frequently resulting in biopsy) [ ]. Models that perform classification of lesions into benign or malignant classes also equal or outperform radiologists [ ], and such systems are now being incorporated into commercial machines as a support tool [ , ].
Elastography is recommended to enhance the differentiation of benign and malignant breast masses [ ]. Multiple models have been developed that incorporate elastography imaging, using DL networks and radiomics, with results outperforming radiologists [ ].
Pancreas
Several AI applications have been developed to increase the potential of endoscopic ultrasound (EUS) examinations. Training systems designed to standardize EUS examinations based on a structured approach have been recently developed, showing that they can improve the miss rate for EUS stations and structures during the learning period [ ].
AI algorithms can help to reduce the variability in image interpretation between different endoscopists, with both detection and classification used in various AI systems [ ]. Thus, AI algorithms can be trained to identify and classify both solid and cystic lesions in Gray-scale EUS images with high accuracy [ ]. Deep learning models can also be combined into complex systems used for the differential diagnosis based on elastography and/or contrast-enhanced EUS [ ]. Even though individual articles had a high degree of heterogeneity, several meta-analyses looked at the accuracy of AI applications [ ]. AI algorithms can be used to analyze EUS images and other clinical data to predict the prognosis of diseases, such as pancreatic cancer [ ]. This information can be further used to guide multidisciplinary board treatment decisions and improve patient outcomes.
Deep learning can be used to provide real-time guidance to endoscopists during EUS-FNA and FNB procedures. This can help to ensure that the needle is placed at the optimal location and that adequate tissue samples are obtained, thus improving the accuracy and safety of EUS-FNA/FNB procedures. Furthermore, DL models can be used to improve workflow with automatic diagnosis documentation [ ].
Overall, AI has the potential to significantly improve the accuracy, efficiency, and safety of EUS. As AI technology continues to develop, we can expect to see even more innovative and effective applications of AI in EUS applications in the future.
Lymph nodes
Lymph node metastasis is a crucial indicator for the pathological staging, prognosis, and guidance of treatment in patients with cancer [ ]. US is a primary tool to predict lymph node metastasis [ ]. However, most patients with early stage-cancer who have clinically negative lymph nodes have no obvious signs on imaging, despite some having micrometastases [ ].
In breast cancer, models have been developed to predict lymph node involvement based on imaging of the lymph node [ ], primary tumor [ ], or primary tumor and peritumoral tissue [ ], with or without elastography [ ]. Models have also predicted lymph node metastatic burden [ ]. In papillary thyroid carcinoma, models based on radiomics have shown improved predictions for cervical lymph node metastases [ ]. Zou et al. used routine ultrasound data to demonstrate promise for integrating machine learning methods into clinical decision-making processes [ ]. In early tongue cancers, intraoral Doppler images were used to predict late cervical metastasis accurately [ ].
Blood vessels
US is used to measure and evaluate carotid plaque to risk stratify cerebrovascular disease. AI models are used to segment images and measure intima-media thickness and plaque burden [ , , ]. Ultrasound radiomics-based nomogram accurately predict cerebral cardiovascular events in patients with asymptomatic carotid plaques [ ] and Type 2 diabetes mellitus [ ], improving the sensitivity and accuracy of visual qualitative carotid plaque scoring by radiologists. Intravascular ultrasound is used in cardiology to assess and risk stratify complex lesions. AI algorithms are able to segment and classify lesions and may allow faster and less variable classification when compared to manual interpretation and radiofrequency derived virtual histology [ ].
Lung
Lung ultrasound has gained growing attention in the clinical and radiology world [ ]. AI models can distinguish between A lines and B lines [ ], and can quantify B lines [ ]. Commercial versions of these algorithms are now incorporated on some scanners. Fetal lung maturity can be predicted from prenatal imaging [ ], and a commercially available software package showed high accuracy predicting neonatal respiratory morbidity [ ]. Investigative techniques incorporating elastography have predicted lung mass density in interstitial lung disease [ ]. A large number of studies reported the use of lung ultrasound in patients with COVID-19. AI models were developed for screening [ ], and distinguishing between COVID-19, non-COVID acute respiratory distress syndrome, and hydrostatic pulmonary edema [ ]. Models demonstrated high accuracy in detecting COVID-19, some outperforming X-ray and CT [ ], and predicting severity [ ].
Obstetrics and gynecology
Ultrasound is the primary imaging modality for obstetrics and gynecology applications. The accurate measurement of fetal biological parameters is important for estimating the gestational age and weight of the fetus, as well as identifying the status of fetal development, but this is time consuming and operator dependent. Automated measurement algorithms have been developed to improve measurement accuracy and operator efficiency, including femur length [ ], head biometry [ ], and nuchal translucency [ ]. Two AI-assisted fetal central nervous system biometry tools are now available on commercial platforms [ ]. Fetal echocardiography, neurosonography, and other fetal anatomy studies can be assisted by guiding image acquisition, automated acquisition of standard views from specific 4D volumes and assessment of image quality, all aiming to improve image standardization. In addition, multiple other algorithms have been designed for direct detection of abnormalities [ ]. As with other fields, external validation of models is uncommon [ , ].
Algorithms to distinguish between benign and malignant ovarian masses have been developed with high accuracy that outperform radiologists and that improve radiologist`s performance when used as a decision support tool [ ]. Intrauterine adhesions have been examined using CNNs applied to 3D transvaginal images with high accuracy [ ].
Musculoskeletal applications
In comparison with other musculoskeletal (MSK) imaging modalities, AI applications for MSK US are relatively under-developed [ ]. Definite categorization of soft tissue lesions into benign or malignant categories on ultrasound remains challenging. Because of these difficulties, AI has been proposed as a tool that can augment the radiologist’s performance by providing an accurate classification of soft tissue masses into benign versus malignant groups, and classify common benign tumors [ ]. Burlina et al. developed a model to distinguish different types of myositis [ ]. Early diagnosis of developmental dysplasia of the hip (DDH) is critical to prevent permanent disability, but US assessment has poor interobserver reliability [ ]. Models have been developed to perform semiautomated segmentation (to assist improved measurements) [ ], or to perform fully automated diagnosis of DDH [ ]. MSK US is increasingly used to evaluate synovitis in inflammatory arthropathies, but standardization and quantification is time consuming. Furthermore, AI has been used for grading of inflammation with high accuracy [ ].
Dermatology
CNNs have been used to classify inflammatory skin diseases with promising results [ ]. Deep learning algorithms can differentiate not only between benign lesions in dermatology ultrasound images [ ], also between benign and malignant lesions [ , ]. In the analysis of high-frequency ultrasound images, deep learning algorithms can accurately perform multiclassification diagnosis for a total of 17 dermatological diseases, including common benign skin diseases and various types of skin cancers and precancerous lesions [ ]. Algorithms to classify burn depth have been developed in animal models [ ].
Limitations and risks
Despite the many potential benefits of AI applied to a clinical setting, and the increasing number of available tools, it has not yet been widely adopted for clinical care due to many challenges including those related to the tools themselves, and those related to applying the tools for clinical applications.
AI model development requires data for training, and many techniques require large amounts of accurately labeled data. Access to sufficiently large datasets for training is currently a major limitation in many areas of AI research, especially health related fields. Creating adequate databases is challenging due to variation in medical record systems and privacy concerns. The technique of “data augmentation” involves adding new information to an existing data collection by means of picture manipulation. An imagine may be transformed in a plethora of ways, including geometric ones like translate and rotate, pixel-value transformations like invert, solarize, and equalize, and noise addition techniques like Gaussian and speckle. Nevertheless, choosing the best transformation techniques for the available data necessitates a great deal of trial and error, including deep learning model training [ ]. Standardization of medical record formats (e.g., DICOM) can reduce the first barrier, but variations in nonstandard systems (such as medical records which store outcomes) may limit data collection. Ethical and regulatory requirements for data privacy may make collecting and sharing sufficient data to create databases difficult. Many countries have strict regulations on storing and exchanging personal and health data, such as the United States’ Health Insurance Portability and Accountability Act (HIPAA) and the European General Data Protection Regulation (GDPR) that researchers and users must comply with. Developments in AI itself can increase concern over privacy and require more stringent actions to prevent data breaches, for example facial recognition algorithms can be applied to cranial imaging data to reidentify individuals [ ]. Privacy preserving techniques (e.g., federated machine learning, differential privacy, etc.) are being developed to assist in overcoming these concerns, both within and outside health applications, but are yet to be widely adopted [ ]. Finally, even when large datasets are available, they must be accurately labelled to be used for supervised learning, which can be extremely expensive.
Although LLMs have dramatically improved the ability to extract data from unstructured reports, variations in report structure, local terminology, abbreviations, and other differences between medical reports and general language remain significant challenges. If not available, labelling requires experts to review and label each data point. ML techniques may be used on a small amount of labelled data to perform “semisupervised” learning to partly overcome this problem [ ].
Once developed, AI models must still overcome several barriers before being implemented. Like any prediction tool, there may be many biases which can limit the accuracy and applicability of the model. In the field of imaging, AI models may not perform as expected on images from different patient populations, different imaging protocols, or different machines (even if the resulting images have better resolution [ ]). Reliance on small or selected populations (for example, due to the limitations described above) increases these risks. Use of surrogate or nonpatient centered measures to train models can create bias. Once bias exists, it can be amplified by feedback loops during model training [ ]. Most models are trained on datasets where the “ground truth” (i.e., the “gold standard” result) is lesion detection by either radiologic abnormality or pathological results. These endpoints, however, may not be clinically meaningful as they may ignore the type and biological aggressiveness of a lesion, resulting in increased sensitivity, but increasing rates of overdiagnosis of subclinical or indolent disease [ ], although it should be noted that this may also present an opportunity to broaden understanding of the full spectrum of a disease. Testing of models in the relevant clinical setting is therefore essential to ensure applicability and reliability in the clinical setting [ ]. A survey of studies published in 2018 revealed that only a small proportion of studies performed external validation [ ], although as the field evolves, researchers are moving towards reporting outcomes of AI imaging systems implemented in real world settings [ ]. To assist the researcher and end user, there are recommendations and guidelines for reporting prediction models [ ] and evaluating research on AI [ ].
Subsequent to successful model development, there may be diagnostic drift over time due to evolving patient groups (e.g., aging), disease (e.g., appearance of COVID affecting chest radiograph interpretation), comorbidity, or treatment. AI models will therefore need to be updated regularly to ensure accuracy.
Even when developed and (believed to be) accurate, AI models may not be implemented due to lack of acceptance by users—both clinicians and patients, and uncertain (even obstructive) regulatory environment. ML models, and neural networks in particular, are often described as “black box” models as they may be difficult to understand generally and especially opaque as to how an individual result is determined. Allied to this is the difficulty in determining the circumstances when a model may be less reliable, even if overall it outperforms human experts. “Interpretability” and “explainability” are used to describe the ability to understand how a model works and how (in human expert terms) a model may have arrived at its prediction or output, and this is currently an active area of research in AI [ ]. Regulation of AI tools is currently a focus for many national and international bodies, challenged by the rapidly evolving technology [ ], but areas such as legal liability and ethical frameworks are still uncertain [ ]. As a result of all these factors, current uptake of models is less than expected by the researchers who developed these AI models. For example, despite outperforming other risk stratification tools and being integrated into the hospital information system, an ML based risk stratification model for chest pain was only used by clinicians in 12% of relevant presentations [ ].
Conclusion
AI offers great potential to improve the performance and interpretation of almost all areas of ultrasound. Many studies have shown performance at least as good as experts in interpreting images, but the majority of studies lack external validation. Models need to be validated on external data sets, preferably prospectively. Results should be assessed in real world settings, measuring outcomes that are patient centered or demonstrate improved work flow for operators.
Conflict of interest
The authors declare that they have no known competing financial interests or personal relationships that could have appeared to influence the work reported in this paper.
Acknowledgment
The authors thank the members of the WFUMB Publication Committee for advice.
Appendix
Supplementary materials
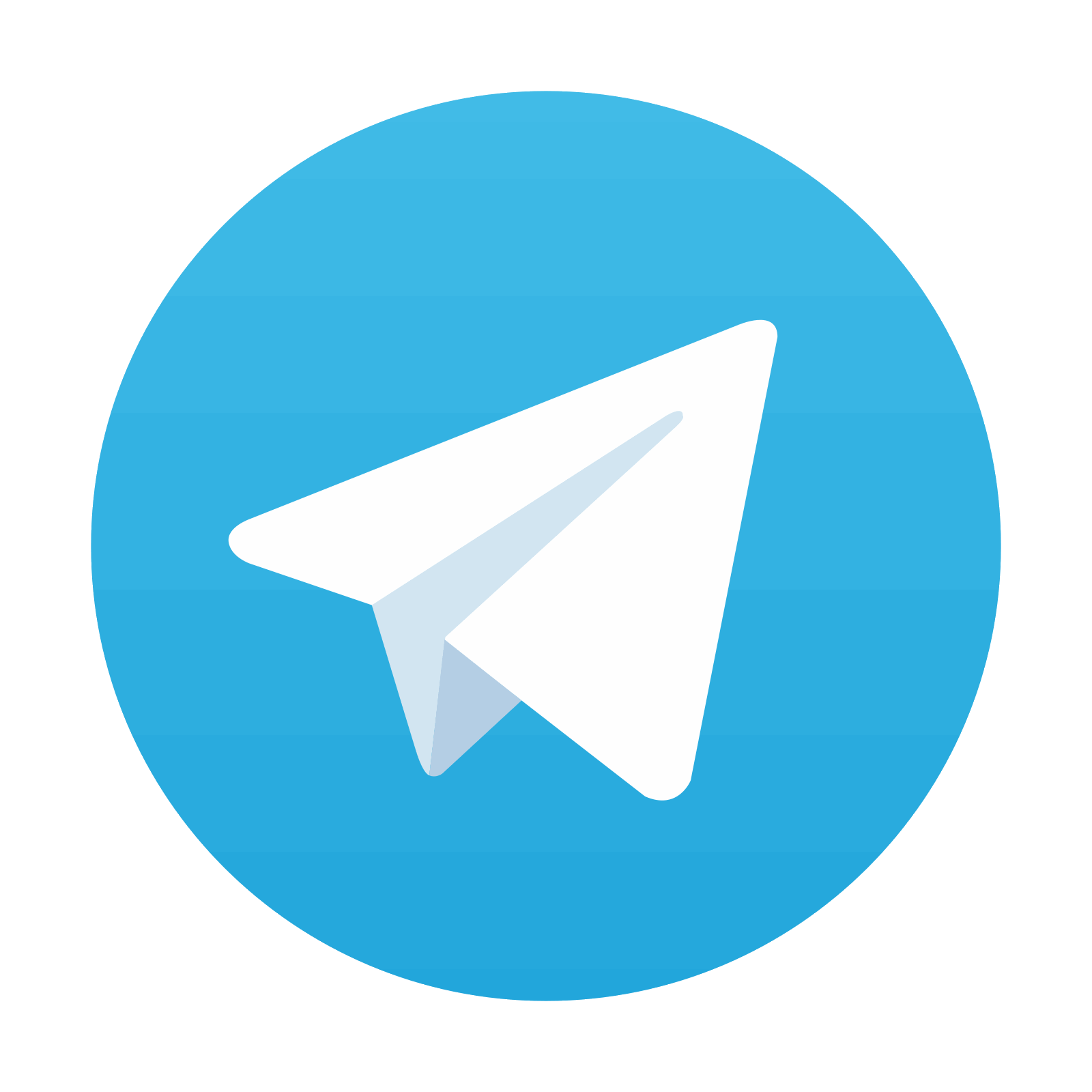
Stay updated, free articles. Join our Telegram channel

Full access? Get Clinical Tree
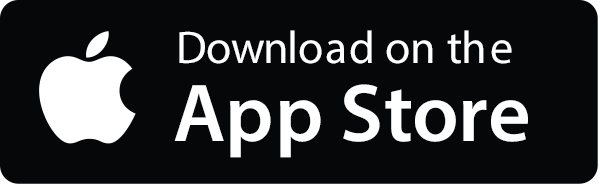

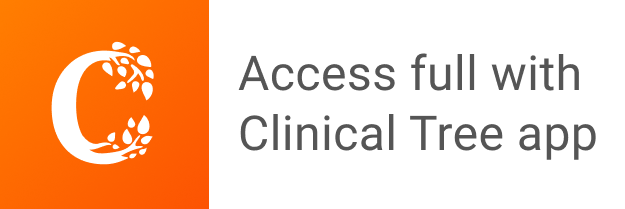