The interpretation of medical images relies on a combination of many factors, including perception, cognition, human factors, technology, and even to some extent innate talent. It is difficult, if not impossible, to consider any of these in isolation because they interact in a number of both clear and subtle ways. However, when we study the interpretation of medical images, by necessity we sometimes must examine only a single variable at one time in order to try to fully understand its contribution to the overall interpretation process. Such is the case with perception, so when designing medical image perception studies it is important to understand what the capabilities (and limitations) of the human eye–brain system are. When one considers how clinicians read medical images, three basic stages are typically regarded as being involved: seeing, recognizing, and interpreting. It may sound simple, but the potential for failure at any point in the interpretation process is actually quite high – errors are made, lawsuits filed, and, more importantly, patient lives impacted (Lee et al., 2013; Siegal et al., 2017; Taylor, 2017; Waite et al., 2017a, 2017b).
Radiology has for many years been the most common clinical specialty in which medical images are used to render diagnoses. Because of this, most image perception research to date has been done in this area. With the advent of telemedicine, however, there are a number of other clinical specialties in which images are used routinely, such as pathology, dermatology, and ophthalmology. Virtual pathology is based on the scanning of glass slides into “virtual slides” (Bashshur et al., 2017; Weinstein et al., 2004, 2009), and with the 2017 approval of whole-slide imaging systems for clinical use it will see more rapid growth in the next few years (Food and Drug Administration, 2017). Teledermatology uses off-the-shelf consumer-grade digital cameras to acquire photographs of skin conditions (Figure 8.1) (Krupinski et al., 1999a; Lee and English, 2018), and teleophthalmology uses digitally acquired photographs of the retina and other eye structures using digitally based nonmydriatic cameras (Rathi et al., 2017; Taylor et al., 2007). The clinical areas in which digital images are being acquired for remote consultations via telemedicine are increasing tremendously (Krupinski et al., 2002), and in these areas rigorous image perception research is required to optimize presentation for efficient and effective diagnostic interpretation and management recommendations.
Figure 8.1 Typical teledermatology image acquired with a 1 Mpixel digital off-the-shelf consumer-grade digital camera.
All of these image-based clinical applications have a number of common features. In every case, clinicians rely on the optimal presentation of the image data in order to render a complete and accurate diagnostic decision. However, no matter what the image acquisition and display technology, abnormalities can be missed or misinterpreted, and the very important question is: how do we avoid such errors? It is certainly possible to improve the imaging systems or develop new systems for imaging and analysis that will provide new and important information that was not available with traditional methods. In radiology improvements are continuously being made and new technologies and tools developed (e.g., quantitative imaging; Larue et al., 2016; Yankeelov et al., 2016). The virtual slides in pathology are now being scanned at multiple depths, recreating virtually the way that pathologists would focus on different planes of interest within a traditional specimen. Automated image analysis and interpretation schemes (i.e., computer-aided detection and diagnosis (CAD and CADx)) are being used extensively in radiology and are being adapted to pathology, dermatology, and other image-based specialties (Emre et al., 2007; Li and Nishikawa, 2015; Mete et al., 2007; Rodriguez et al., 2016). What may eventually have an even greater impact, not only in imaging but in healthcare in general, is the multitude of deep learning techniques being developed as decision support tools (Lee et al., 2017; Miotto et al., 2017; Shen et al., 2017).
However, technology development is not the ultimate answer to eliminating medical image interpretation errors. For example, although the “threat” of artificial intelligence may seem real to some in healthcare and in radiology in particular (Komorowski and Celi, 2017; Recht and Bryan, 2017), clinicians still need to review the images and see, recognize, and interpret the information that is there and render a decision that will affect patient treatment and care. We need to understand not only the images and the technologies used to acquire and display them but also the interpreter of those images – the clinician. As the clinical environment changes and more and more various types of images become a part of the patient record, this becomes even more critical. This chapter reviews some of the basic issues associated with medical image perception research, especially in the context of radiology, but with examples from other areas of medical imaging as well.
8.1 Vision Basics
The human visual system has been studied for thousands of years and we know much about it in terms of physiology, neural connections to the brain, and where in the brain information gets processed. This chapter will not describe the visual system or vision theories in great detail (see Forrester et al. (1996) for an excellent overview), but some aspects are relevant to medical image perception. At a very basic level there are two main aspects of vision that are important for interpreting most medical images, especially radiographs – spatial resolution and contrast resolution. Surprisingly with respect to vision, clinicians in image-based specialties often do not get regular eye exams (Quaghebeur et al., 1997) and very few observer performance studies in the past even asked them to verify that their vision was 20/20 or corrected to 20/20 (although more do now). Of interest is that in some subspecialties, such as interventional radiology, eye disease (e.g., cataracts) may be an occupational hazard (Junk et al., 2004).
Spatial resolution, or the ability to see detail, is highest at the fovea and declines rather sharply as one moves out toward the peripheral regions of the retina. In terms of visual acuity, it drops by about 75% at about 5° from the center of the fovea (Figure 8.2). This is a reflection of the distribution of the rods, which are responsible for sensing contrast, brightness, and motion, and the cones, which are responsible for fine resolution, spatial resolution, and color. The rods (about 115 million in total or 30,000/mm2) are located mainly in the retina’s periphery, while the cone population increases toward the macula. In the fovea itself there are only cones and they number about 6.5 million in total or 150,000/mm2. Also contributing to these resolution differences is the fact that in the fovea there is a convergence of rods and cods on the next layer of visual cells, called the bipolar cells. The ratio of cones to bipolar cells in the fovea is essentially 1:1. In the periphery each bipolar cell receives input from 50–100 rods. This means direct transmission of a single signal versus a combination of multiple signals into one neural pathway.
Figure 8.2 Schematic of the extent of high-resolution foveal vision and peripheral vision where the central foveal part represents the useful visual field that must be directed across an image during search to fixate on image detail.
The acuity or resolving power of the eye depends on physiological factors as well as the physical separation between the stimuli to be discriminated, the wavelength of the light, the illumination of the background on which the stimuli appear, and the level of dark/light adaptation of the viewer. The dependence of visual acuity on contrast (and vice versa) may be even more important. By testing observers’ ability to discriminate a thin white line against a uniform background illumination, it has been found that the limits of resolution are about 0.5 minutes of arc. Due to diffraction effects, detecting this thin white line depends on the liminal brightness increment (lbi) or the point at which the bright and dark diffraction rings at the edges of the line are detected. If these rings are not different enough from the background, they will not be detected and neither will the line. Sinusoidal grating patterns of dark and light lines (where the average luminance is the same but the contrast between the lines differs) can be used to measure the lbi in a more direct and practical manner. The grating frequency (cycles per degree) and acuity, defined as 1/grating frequency, characterize grating discrimination. Quite a few psychophysical studies have been done with this method, and have shown that contrast sensitivity peaks in the mid spatial frequency range around 3–5 cycles/degree. A number of other factors also affect contrast sensitivity, including luminance, the direction of the grating lines, the grating frequency, line width, motion, dark/light adaptation, and wavelength.
These spatial and contrast resolution limits can be readily characterized using discrete, generally geometric stimuli against fairly uniform backgrounds with classic psychophysical paradigms. With medical images the targets are not simple geometric shapes, the backgrounds are generally very complex, and the clinicians rarely know the type of target lesion they are looking for or where it is located a priori. Thus it is a bit simplistic to say that an abnormality of a given size and contrast (as rendered on a given display) should be detected if it falls within the limits of spatial and contrast resolution. Medical image perception is more complicated.
8.2 Visual Search
It is clear that spatial resolution is greatest at the fovea, and what this means for perception research is that images need to be searched or scanned with the fovea, especially if small, low-contrast objects are going to be detected. There are some abnormalities that can be detected with peripheral vision (Kundel and Nodine, 1975), but normal anatomy (or structured noise) can significantly decrease detection the further away they are located from the axis of gaze (Kundel, 1975). Peripheral vision contributes to the guidance of foveal vision (i.e., the effective visual field) to abnormalities, but this is not the main mechanism for detection (Kundel et al., 1991). Search is required to cover an image adequately with foveal vision, so research into how radiologists search images is an active area of interest (see Van der Gijp et al. (2017) for a good review).
Tuddenham and Calvert in 1961 carried out one of the first studies of search patterns in radiology. Radiologists were given a flashlight with an adjustable diameter and were asked to move the beam across a series of paper-based radiographic images as they searched for lesions. They adjusted the light’s diameter to illuminate only the area that was required for accurate and comfortable interpretation. It was found that there is a significant difference between readers in their search patterns, and that readers tend to be rather nonuniform in their coverage. This nonuniformity in coverage might be a significant source of error.
The first study in radiology to record eye position of radiologists was by Llewellyn-Thomas and Lansdown (1963). It demonstrated, in a more rigorous way, that search patterns are unique to an individual and nonuniformly cover the image. A number of studies have been done since then using eye position analysis as a tool for understanding how the radiologist searches images and why errors tend to occur. The technology has changed, of course, but eye position recording is still used as a tool to observe and understand the perceptual processes involved in medical image perception. Most of the eye-tracking systems are based on the same general principles. They typically use a low-level infrared light source that is reflected off the pupil and cornea back into a digital camera that samples this signal every 1/60 second. Figure 8.3 shows a typical observer setup for an eye position recording system using a head-mounted system.
Figure 8.3 An observer setup to record eye position during a search task. The optics of the recording system are located on the headband above the observer’s eyes. The visor reflects the infrared signal into and back out of the eyes to a digital camera also located above the eyes.
Since the system is calibrated to the scene (image) it is possible to correlate visual dwell information with locations in the image being viewed. Most of the head-mounted systems achieve at least 1° of accuracy (spatial error between true eye position and computed measurements) and many remote systems are equally accurate, although some are slightly less but still quite useful. Both types of system can use a magnetic head tracker in addition to the optical system to track any movements that the observer makes and incorporate these data into the eye-tracking record. Without a head tracker it is often necessary to restrict the observer’s range of motion with a headrest or at least a specified “box” within which the observer can move from side to side and closer/farther away from the display. In studies with clinicians this does introduce a rather unnatural situation since in the clinic they do tend to change viewing and seating positions quite often.
The raw x, y location data generated during search are typically processed using both spatial and temporal thresholds to sum the raw data into more meaningful units (Nodine et al., 1992). These thresholds can vary and are to some extent determined by the stimuli, the task, and the observers. Typically, the raw x, y coordinate data are transformed, using these thresholding algorithms, into fixations that generally last a minimum of 250 ms. Figure 8.4 shows a typical eye position recording output file.
Figure 8.4 Typical output file from an eye position recording trial. Column 1 shows the temporal order of the fixations, column 4 shows the individual fixation durations, and columns 7 and 8 show the x, y locations of the fixations.
The fixations can be gathered together using spatial thresholds into clusters of fixations. This is often useful when determining how long someone looks at a given location. By adding in return visits to a given location (i.e., after scanning somewhere else in the image), cumulative clusters can be calculated to give a more accurate picture of the total time spent fixating a location. This is important in medical imaging because it is useful to know whether or not someone actually fixated a lesion. Figure 8.5 shows a typical search pattern of a radiologist searching for nodules in a chest image.
Figure 8.5 Typical eye position pattern of a radiologist searching a chest image for nodules. Each small circle represents a fixation and the lines show the order in which they were generated. There is a nodule in the lower left lung with a single fixation on it. This single fixation was all the experienced radiologist needed to report the nodule’s presence.
These types of search patterns are not unique to the radiologist’s task of searching for lesions in X-ray images. The search patterns of pathologists searching virtual slides for cells indicative of cancer (Figure 8.6) are quite similar.
Figure 8.6 Typical eye position pattern of a pathologist searching a virtual slide for cells indicative of breast cancer. Each small circle represents a fixation and the lines show the order in which they were generated.
One of the underlying assumptions in recording eye position is that observers are not only directing high-resolution foveal vision to areas within the image, but they are directing their attentional and information-processing resources there as well to extract and process information about the scene in order to render some sort of decision about it. Even when observers look at works of art (Figure 8.7) they are directing their perceptual and attentional resources to locations within the image that are interesting and/or informative – in this case to decide if the artwork is aesthetically pleasing or not (Locher et al., 2007).
Figure 8.7 An observer scanning a work of art (Edo’s The Wave) in order to decide if it is aesthetically pleasing.
Figure 8.8 shows the eye position pattern of a radiologist searching for nodules in a patient with only one lung (the right lung is missing). Information-processing theory provides the basis for the current interpretations of visual search data such as these (Buetti et al., 2016; Crowley et al., 2003; Haber, 1969; Krupinski et al., 1998; Nodine and Kundel, 1987; Nodine et al., 1992; Spoehr and Lehmkuhle, 1982). The initial glance at an image results in a global impression of the image that includes the processing and recognition of content such as anatomy, symmetry, color, and grayscale. The information gathered in this global impression is compared with information contained in long-term memory that forms the viewer’s cognitive schema (or expectations) of what information is in an image. In some cases, the target of the search “pops out” in this global impression and the viewer makes a quick decision (Drew et al., 2013; Wolfe and Horowitz, 2017).
Figure 8.8 Eye position pattern of a radiologist searching for nodules in a patient with only one lung. Since there is no relevant information in the right lung area (missing lung), the radiologist does not generate many fixations on that side. There is a nodule under the left clavicle that was fixated but not reported (false negative).
If there is no relevant information at some locations within the image (if there is no lung there can be no lung nodules), there is little need to direct high-resolution foveal gaze and attention to those areas. Thus the radiologist recognized within a split second of the image coming into view that there was no right lung present. This split-second recognition is related to what is known as the global percept, in which a massive amount of parallel processing occurs within the visual system, characterizing the image, its general layout, major features, and boundaries. This early stage of scene perception takes place in less than 250 ms. The information processed in this global percept guides the subsequent search of the image with high-resolution foveal vision, where the extraction of feature details from complex image backgrounds takes place.
The early studies in medical image perception focused on trying to determine why errors were made – especially when lesions that were missed initially could often be easily detected when viewed another time. It is estimated that the miss rate in radiology is about 20–30% (false negatives) with a false-positive rate of about 2–15% (Bird et al., 1992; Muhm et al., 1983; Robinson, 1997).
To some extent, false positives are easier to understand than false negatives from a perceptual point of view. False positives often occur because the overlaying anatomic structures can mimic disease entities. A vessel on end or an ambiguous nipple marking can easily be mistaken for a nodule. For many false positives, there is clearly something in the image that attracts attention and leads to the false impression that a lesion exists. This is not always the case, however, because sometimes there does not seem to be anything clearly identifiable in the image but a lesion is still reported. Kundel et al. (1989) called these two types of false positives common and sporadic. Common ones tend to be reported by more than one person and are associated with longer dwell times. Independent ratings of common false positives indicate that they indeed have more lesion-like features. Sporadic ones tend to be reported by only a single radiologist, are associated with few or no lesion-like features, and tend to have less visual dwell associated with them.
False negatives are slightly more complicated, but probably more important to understand in terms of why they occur. Once technical reasons (e.g., patient positioning or exposure) are eliminated, the cause of such errors most likely resides in the reader (Lee et al., 2013; Siegal et al., 2017; Taylor, 2017; Waite et al., 2017a, 2017b). Tuddenham and Calvert (1961) suggested that lesions may be missed due to inadequate search of images and significant interobserver variability in search strategies. Kundel et al. (1978) followed up on these ideas and used eye position recording to classify types of errors made during search. Again, a key assumption is that the eye position system records the axis of gaze and visual dwell is a reflection of information processing and allocation of attention and visual processing resources (Nodine et al., 1992).
Thus, false negatives have been classified into three categories (Kundel et al., 1978) based on how long they are dwelled on or fixated. About one-third of false-negative errors fall into each category. The first error category is known as a search error because the observer never fixates the lesion with high-resolution foveal vision and thus cannot begin to process the information at that location in the image. Since there are no fixations directed here and no positive report of the lesion’s presence, it is also assumed that the lesion was not detected in the global percept.
The second type of error is called a recognition error, because these lesions are fixated with foveal vision, but not for very long. Inadequate time spent fixating reduces the likelihood that lesion features will be detected or recognized.
Decision errors comprise the third group and these occur when the observer fixates the lesion for long periods of time, but either does not consciously recognize the features as those of a lesion or actively dismisses them. In Figure 8.8 there is a nodule located about 2 cm under the left clavicle. The radiologist fixated it but did not report it. The dwell time was less than 1000 ms (a typical cutoff point in chest viewing) so the false negative was considered to be a recognition error. The dwell time cutoff between recognition and decision errors may vary as a function of the type of image and type of lesion, but these types of errors have been noted in chest (Kundel et al., 1978, 1989), bone (Hu et al., 1994; Krupinski and Lund, 1997), and mammography images (Krupinski, 1996; Nodine et al., 2002).
Fixations are not the only relevant eye position parameters used in understanding the perception of medical images. How the observer gets from one place to the next in an image is important as well. Scan paths represent saccadic eye movements or movements of the eyes around a scene, locating interesting details and building up a mental “map” representing the scene. Again, the eyes move so that small parts of the scene can be centered in the fovea where visual acuity is the greatest. A number of studies of perception in radiology and pathology image interpretation pay special attention to eye movements (e.g., saccades), based on the hypothesis that eye movements may reflect meaningful differences in the cognitive abilities of novice and expert pathologists (Bertram et al., 2013, 2016; Kok et al., 2015; Krupinski et al., 2006).
In the Krupinski et al. (2006) study, medical students, residents, and experienced pathologists viewed a series of virtual slides on a color (9 Mpixel) liquid crystal display as their eye position was recorded, with the task being to indicate where they would like to zoom to get a higher-resolution view of the image. The residents and medical students generated about one-third of all fixations with equal frequency on their first, second, and third preferred zoom locations, but the pathologists generated significantly fewer fixations (26%) on their first selected preferred zoom location and significantly more (44%) on the second preferred zoom location. This is consistent with the pathologists’ ability to identify preferred zoom locations in their peripheral vision.
In terms of the saccades, the pathologists had significantly longer saccades (mean 0.500 second) than the residents who, in turn, had significantly longer saccades (mean 0.244 second) than the medical students (mean 0.129 second). The pathologists generally fixated on a location and then made a long saccade to another fixation location and had few repeat fixations and small saccades around the same location. The residents and medical students had long saccades between locations as well, but had more short saccades and hence more fixations within a given area.
Total scan path distance (in degrees of visual angle) was also calculated by summing all of the saccade distances or the lines between each fixation (Figures 8.5–8.7). The pathologists had shorter total scan path distances (mean 63.21°) than the residents (mean 176.37°) or the medical students (mean 205.74°). The saccades were also analyzed to examine whether there were differences in the number of saccades generated per image searched as a function of experience. There were, with the pathologists generating the fewest saccades followed by the residents, who were followed by the medical students, who had the most saccades per image. There were also fewer saccades generated on the malignant cases than on the benign cases for all three groups.
In the 2016 Bertram et al. study, it was observed that in the presence of radiographic lesions (abdominal computed tomography (CT) scans), saccade lengths of specialists (minimum of 2 years of experience in abdominal radiology) shortened more than those of advanced (> 2 years’ residency) and of early (< 2 years’ residency) residents. For all subjects, higher detection rates correlated with greater reduction of saccade length in the presence of lesions. This study was based on an earlier study by this group (Bertram et al., 2013) comparing radiologists, CT radiographers, and psychology students, showing similar results.
8.3 Using Visual Search to Study the Reading Environment
Eye position recording as a tool to understand medical image perception has been used to study other aspects of viewing than just errors. Chapter 11 discusses the body of data that we have on the impact that expertise has on visual search, perceptual processing, and decision-making accuracy.
From a purely perceptual standpoint, a couple of points regarding displays are warranted here. The Digital Imaging and Communications in Medicine 14 (DICOM-14) grayscale standard display function (GSDF)(Blume, 1996) was devised in part to provide a basis for providing similarity in grayscale perception for a given image when displayed on different display systems that may not have the same luminance. The emphasis on luminance and proper calibration of display monitors is important because it has been found that luminance levels drift over time (Evanoff et al., 2001; Groth et al., 2001; Parr et al., 2001). One study (Evanoff et al., 2001) monitored display parameters (including maximum and minimum luminance levels) of 98 monitors used over a 5-day period for the oral portion of the American Board of Radiology exam meeting and found that there was considerable luminance drift in even that short a period of time. It is clear that without regular calibration it is possible that these changes in luminance could affect detection and search performance (Krupinski et al., 1999b).
However, there is a significant amount of flexibility in the human visual system and it may be that those with more experience in radiology may actually become more perceptually sensitive to the crucial parameters of visual analysis required for detecting lesions in radiographic images (Sowden et al., 2000). In one study (Siegel et al., 2001) that assessed performance using displays with degraded image quality, there were no significant differences in sensitivity (although specificity varied a bit more), but the differences in performance were smaller for dedicated chest radiologists compared to general radiologists. It may take significant changes in display parameters to affect performance of experts significantly because of perceptual learning or adaptation.
The importance of perception and its dependence on the display medium are obviously crucial. In fact, the DICOM-14 display standard for digital radiology is based on the idea of perceptual linearization (Blume, 1996; Johnston et al., 1985; Pizer, 1981a, 1981b). Perceptual linearization capitalizes on the capabilities of the human visual system (i.e., threshold contrasts and just-noticeable differences (JNDs)) to optimize displays by producing a tone scale in which equal changes in digital driving levels yield changes in display luminance that are perceptually equivalent across the entire luminance range. This means that equal steps in brightness sensation represent equal steps in acquired image data, and studies have shown that it does matter (Blume, 1996; Johnston et al., 1985; Pizer, 1981a, 1981b).
One study (Krupinski and Roehrig, 2000) examined detection and search performance for detecting masses and microcalcifications in mammograms using a perceptually linearized DICOM-calibrated display versus a nonperceptually linearized display calibrated using the Society for Motion Picture and Television Engineers (SMPTE) pattern. It was found that there was statistically increased detection accuracy with the perceptually linearized display. Eye position recording revealed significantly more efficient visual search patterns with the perceptually linearized display. The total viewing times, time to first fixate the lesions, decision dwell times, and number of fixation clusters generated during search were decreased with the perceptually linearized display. Proper calibration of the digital display in terms of luminance and tone scale clearly impacts efficient and accurate perceptual performance.
There is no one-size-fits-all configuration for display monitors in medical image interpretation, and it varies for each clinical specialty, but understanding visual search with different configurations might help optimize reading environments. For example, one recent study compared a single 8 MP versus two side-by-side 5 MP displays for mammogram interpretation (Krupinski, 2017). It measured diagnostic accuracy, reading time, number of times the readers zoomed/panned images, and visual search.
Six radiologists viewed 60 mammographic cases, once on each display, with eye tracking on a subset of 15 cases. There was significant difference for viewing time, with 8 MP taking less time (62.04 vs. 68.99 s) than dual 5 MP. There was no significant difference in zoom/pan use (1.94 vs. 1.89 times per case). Total number of fixations was significantly lower with 8 than 5 MP (134.47 vs. 154.29), but time to first fixate lesion did not differ and total time spent on lesion did not differ (8.59 vs. 8.39). Of most interest was that the number of times readers scanned between images was significantly fewer with the 8 MP display (6.83 vs. 8.22). Overall, the single 8 MP display yielded the same diagnostic accuracy as the dual 5 MP displays – in other words, the lower resolution did not influence the readers’ ability to detect and view the lesion details.
From the eye position study it appears that the gain in efficiency is not due to detecting or spending any different time on the lesions, but rather results from not having to scan back and forth from image to image as much. This may be due to the presence of the bezels around each of the two 5 MP displays creating a physical separation between the two images, while the single 8 MP display has the two images abutting each other without anything between them.
8.4 Color Vision
Medical image perception as applied to radiology is rather unique since radiology is essentially monochrome while most other clinical specialties that use images use color images (for a good review of the role of color in pathology, see Clarke and Treanor (2017)). It is possible to add color to radiographic images to bring out certain details (e.g., color Doppler ultrasound (Beebe et al., 1999; Blaivas, 2002)), but the initial rendering is in shades of gray.
It is interesting to note, however, that even with pseudocolor images, it may be important to utilize certain calibration color scales versus others, as the scale used can impact perceptual/diagnostic performance. For example, in a recent study, Chesterman et al. (2017) studied the detectability of color differences using a medical display calibrated to CIEDE200-based color standards display function (CSDF), the DICOM GSDF and the typically used the standard red, green, blue (sRGB) method. Error when interpreting a rainbow color scale was lower for CSDF than for DICOM GSDF in an initial study, and there was a significant improvement in detecting color differences with CSDF compared to DICOM GSDF and a trend toward improved detection compared to sRGB. Interestingly, in an earlier study the heated object scale yielded performance equivalent to grayscale and better than the rainbow and a number of perceptually linearized scales (Hong and Burgess, 1997). Differences in results may be due to the nature of the tasks, targets, and backgrounds used. Although it did not use clinical images or a diagnostic task, the authors suggest that the results may be beneficial in quantitative imaging such as positron emission tomography standardized uptake value, quantitative magnetic resonance imaging, and CT and Doppler ultrasound.
In another study, it was found that color scale actually impacted perceived eye fatigue, with yellow (versus gray, blue, red, green) yielding the lowest fatigue and yellow and green yielding the highest detection performance (Ogura et al., 2017).
Color may also be used when merging/registering images from different modalities to highlight the differences between images as an indication of disease or other changes (Kather et al., 2017; Rehm et al., 1994). Color is useful in medical image perception because the human visual system relies quite heavily on color perception. In fact, from an evolutionary perspective color may be more important for survival than spatial resolution (Chaparro et al., 1993)! Data from Levkowitz and Herman (1992) indicate that color has a dynamic range of at least 500 JNDs. Grayscale has a dynamic range of only about 60–90 JNDs – clearly more restricted. It’s not that simple, however, because the visual system has lower spatial resolution in the color channels than in the luminance channels. In radiology at least, increasing dynamic range by going to color may actually be confounded by color luminance dependencies having a significant effect on contrast perception (Granger and Heurtley, 1973; Mullen, 1985). In other clinical specialties, where color is the rule rather than the exception, this not really an issue.
An interesting point to consider when discussing medical image perception and color images is that there are common deficiencies in color vision that might have to be considered when moving to color displays and developing methods to calibrate them. Thus, when conducting studies where color may be a consideration, a simple color vision assessment of participants should be carried out using, for example, the Ishihara color test set (Krupinski et al., 2014; www.colour-blindness.com/colour-blindness-tests/ishihara-colour-test-plates/). Very basically, color vision is based on the fact that there are three sets of cones in the retina. Each is sensitive over a range but has peak sensitivity at a specific wavelength: short at 419 nm (blue), medium at 531 nm (green), and long at 558 nm (red). There are relatively few short cones so overall photopic sensitivity is due largely to summed medium and long cones (555 nm). Perceptually, color is characterized by three properties. Saturation is the depth or purity of colors and is related inversely to the number of different incident wavelengths. Hue or color name (e.g., red) is a function of the wavelength(s) reaching the eye’s photoreceptors. Brightness is the intensity of the color and is roughly proportional to the amplitude of the incident wavelength(s).
Color may be more relevant to pathologists, ophthalmologists, dermatologists, and other clinicians who utilize true-color images compared to radiologists who use grayscale and pseudocolor images only. Color vision deficiencies fall into two categories – acquired or inherited. One common acquired deficiency that occurs with age is the growth of cataracts, which not only affect acuity but lead often to a yellowing of the lens (xanthopsia), resulting in the lens absorbing blue and green, making perception in this range difficult. Corneal edema as a result of allergies, infections, and even contact lenses can result in the perception of halos and rainbows, especially around bright light sources (e.g., display devices). Age-related macular degeneration affects about 23% of people over 65 and is a loss of cones, resulting in a loss of both acuity and color perception. Migraines (and sometimes regular headaches) can cause a central blind spot (scotoma) in which colored zig-zags (like twisted pieces of yarn) shimmer or flash, without any actual external stimulus. Chromatopsia results in a distortion of color in which the scene is either infused with an illusory blue tint (cyanopsia) or an illusory yellow tint (xanthopsia). Clearly, if the diagnostic task requires proper perception of color within a medical image, these degradations in perceptual processing of color could affect diagnostic accuracy.
Much more common than the acquired color deficiencies are inherited deficiencies and these too can be important in the perception of color medical images. There are three types of congenital color vision deficiencies, referred to as monochromacy, dichromacy, and anomalous trichromacy. Most inherited color deficiencies are significantly more common in men than in women. Monochromacy or total color-blindness is the lack of all ability to distinguish colors. This occurs when two or all three of the cone pigments are missing and the result is that color vision is reduced to a single dimension. This is a relatively rare condition. Dichromacy occurs when only one of the cone pigments is absent or not functioning properly, reducing color to two dimensions. Protanopia is caused by the absence of red photoreceptors, making red colors appear dark; and in deuteranopia the green photoreceptors are missing, affecting red–green discrimination. Tritanopia is the rarest deficiency in this category, in which blue receptors are missing.
Anomalous trichromacy is rather common and occurs when one pigment is altered in its spectral sensitivity rather than missing altogether. Protanomaly is an alteration of red receptors (closer to green receptor response), resulting in poor red–green discrimination. Deuteranomaly affects the green receptors and is the most common color deficiency. It affects red–green discrimination, especially in males. Tritanomaly is rare and affects blue–yellow discrimination. There are really no significant studies that have examined color perception or color deficiencies and their impact on diagnostic accuracy in any field of medical imaging.
8.5 Stereoscopic Viewing
Most images (e.g., paintings or photographs), including medical images, are two-dimensional (2D). They generally incorporate cues that lead to the perception of depth (e.g., interposition or linear perspective) but they do not truly incorporate depth information in the sense of seeing 3D objects. Radiographic images are 2D images of 3D objects (the human body) and the third dimension is captured primarily as density differences and structural overlap. Dermatology images try to incorporate depth by acquiring one image of a lesion straight on and another from the side (McKoy et al., 2016). Ophthalmology captures multiple views of the retina as well. In all of these cases, however, the clinician takes the 2D information and with knowledge of human anatomy “sees” the third dimension. The perception or interpretation of depth is done primarily in the mind of the clinician. Digital imaging, however, presents a unique opportunity for viewing medical images in 3D, potentially leading to better localization and characterization of lesions.
With proper acquisition devices and advances in display technologies, 3D is becoming much more feasible than in the past in many areas of medical imaging. The question of course is: does it help? Some observer model-based investigations suggest it might (Douglas et al., 2016; Reinhold et al., 2017) and even holography is becoming a reality clinically (Bruckheimer et al., 2016). Stereotactic biopsy systems, for example in mammography or performing other procedures (Moll et al., 1998), have been shown to improve the accuracy and reduce the time spent performing these interventions. One study (Rosenbaum et al., 2000) that used rotating 3D images presented on a stereoscopic display showed that readers tended to have increased confidence in their perception of findings. Getty (2007) has demonstrated similar success with stereoscopic viewing of mammography images in which standard 2D and stereoscopically presented 3D mammography images were presented to radiologists. Diagnostic accuracy was improved, especially for microcalcifications, with the stereo mammograms and a significant number of new lesions were actually detected compared to 2D viewing. It is likely that the improvement was due to better separation of over-and underlying tissues from the lesions.
Manipulating images in a variety of ways to get better views or see things from a different angle also seemed to help in separating normal tissue from lesion tissue. Benign versus malignant characterization was also observed and attributed to the fact that the stereo images allow the observers to follow the extent of the lesions through the normal tissue more effectively and to visualize lesion characteristics obscured by overlying tissues in 2D images. There have been few studies in other medical imaging areas on potential benefits of 3D over 2D images, especially in education and training (De Faria et al., 2016; Murakami et al., 2017).
8.6 Perception and Visual Fatigue
A later chapter will discuss this topic in more detail, but it is worth mentioning in this more general context of perception as well. Clinicians in all specialties are viewing more medical images of all sorts (along with text data) from computer displays on a regular basis. Radiologists and pathologists are doing so at an extraordinary rate given the fact that image viewing comprises close to 100% of what they do. This long-term viewing of images at a relatively close distance for hours on end may however have a negative side. Computer vision syndrome (CVS) is a repetitive strain disorder characterized by eye strain, blurred vision, double vision, dry eyes, tired eyes, headaches, neckaches, and backaches (Rechichi et al., 1996; Takahashi et al., 2001; Yunfang et al., 2000). According to the Occupational Safety and Health Administration (OSHA), about 90% of US workers using computers for more than 3 hours per day suffer from some form of CVS. Significant effects include perceptual errors (OSHA, 2017), performance errors, decrease in reaction time, fatigue, and even burnout.
Up to about 15 years ago one could easily have said this has nothing to do with radiology or other medical image perception tasks, but today it has everything to do with medicine. Radiology in particular has changed from a predominantly film-based specialty to one in which images are read from computer monitors. CVS has not been studied to any degree in medicine, but it has been studied in other areas and the effects observed are likely to be observed in digital-based medical image interpretation as well. The aspect of most concern is the effect on perception and performance, since these two phenomena comprise the basics of what medical image perception is all about – viewing a diagnostic image and rendering a diagnostic interpretation.
Short-term visual fatigue has been often noted as one of the main symptoms of CVS, but there are long-term effects that could affect perception as well. Prolonged and repeated computer use can lead to vision problems (e.g., induced myopia or asthenopia) that are likely to need correction (Mutti and Zadnik, 1996; Sanchez-Roman et al., 1996). The cause of these problems seems to be the amount of effort required for vergence and accommodation responses with respect to fixating the computer display. Decreased vergence accuracy at far distances (Tyrrell and Leibowitz, 1990) occurs with prolonged viewing and it is even more pronounced as task and stimulus complexity increase (Watten et al., 1994). This is especially relevant for medical imaging because interpreting medical images is a very complex task both perceptually and cognitively, and the stimuli themselves are very complex and vary considerably from case to case.
Objective factors can be monitored to determine if display users are fatigued and should take a break or stop altogether. Measures such as pupil diameter, eye movement velocity, dark focus of accommodation, and width of focal accommodation can be measured relatively easily to determine someone’s relative state of fatigue and the results fed back to the viewer to act upon (Chi and Lin, 1998; Murata et al., 2001). Two factors that can affect fatigue in medical image viewing are display luminance and room illumination. Both medical-grade and off-the-shelf displays have higher and higher luminance values, and at least in radiology high luminance is associated with better diagnostic accuracy and more efficient visual search. There may be limits to how high we can go, however, in luminance before it starts to induce fatigue.
One study (Saito and Hosokawa, 1991) with rather simplistic stimuli showed that increased display luminance correlated highly with increased fatigue and the impact was lessened when display luminance and room illumination were kept constant. The less often the eyes had to dark- or light-adapt to changing light intensities from either the display or room lights, the less fatigue and effects on visual reaction time were observed. Other factors also can impact fatigue levels, including ambient noise (Takahashi et al., 2001), the height of the display (Sotoyama et al., 1996; Turville et al., 1998), experience with computers (Czaja and Sharit, 1993; Krupinski et al., 1996b), and even user age.
Most of the factors are easily controlled for in the digital reading environment once users are aware of them. From a perceptual standpoint, room design, design of the immediate area clinicians work in, and the specifications of the display (e.g., luminance, contrast ratio, and pixel size) are all well within the control of the operator and should be calibrated and monitored on a regular basis to promote optimal reading conditions and avoid fatigue and stress.
8.7 Conclusions
There are many other aspects of perception that impact the interpretation of medical images. The aspects considered in this chapter, however, provide those interpreting medical images and those running observer studies with an idea of the types of things they should be concerned with. Many things need to be considered in the optimization of medical image perception, including basic visual system physiology, the display, the room, the images, the task, and especially the clinician. Even something as simple as how often a clinician needs to take a break from viewing to avoid fatigue and decrease the probability of making errors is important to understand. The problem is not a static one that will be solved with “the” study on medical image perception.
Technology changes continually. The cathode ray tube was replaced by the liquid crystal display, and true 3D projection displays, microdisplays, and even virtual-reality displays (Farahani et al., 2016; King et al., 2016) are being explored. Film has been essentially replaced by digital acquisition and display in radiology and glass slides are being transformed into virtual slides that no longer require a light microscope to view in pathology. Digital images of the skin, the eyes, and many other organs are being acquired with simple digital cameras and transmitted around the world for quick and accurate interpretation. Each of these medical imaging applications presents new challenges to clinicians’ perceptual and cognitive systems. Our continued exploration of medical image perception and the way the clinicians interact with the medical image will always be important.
References













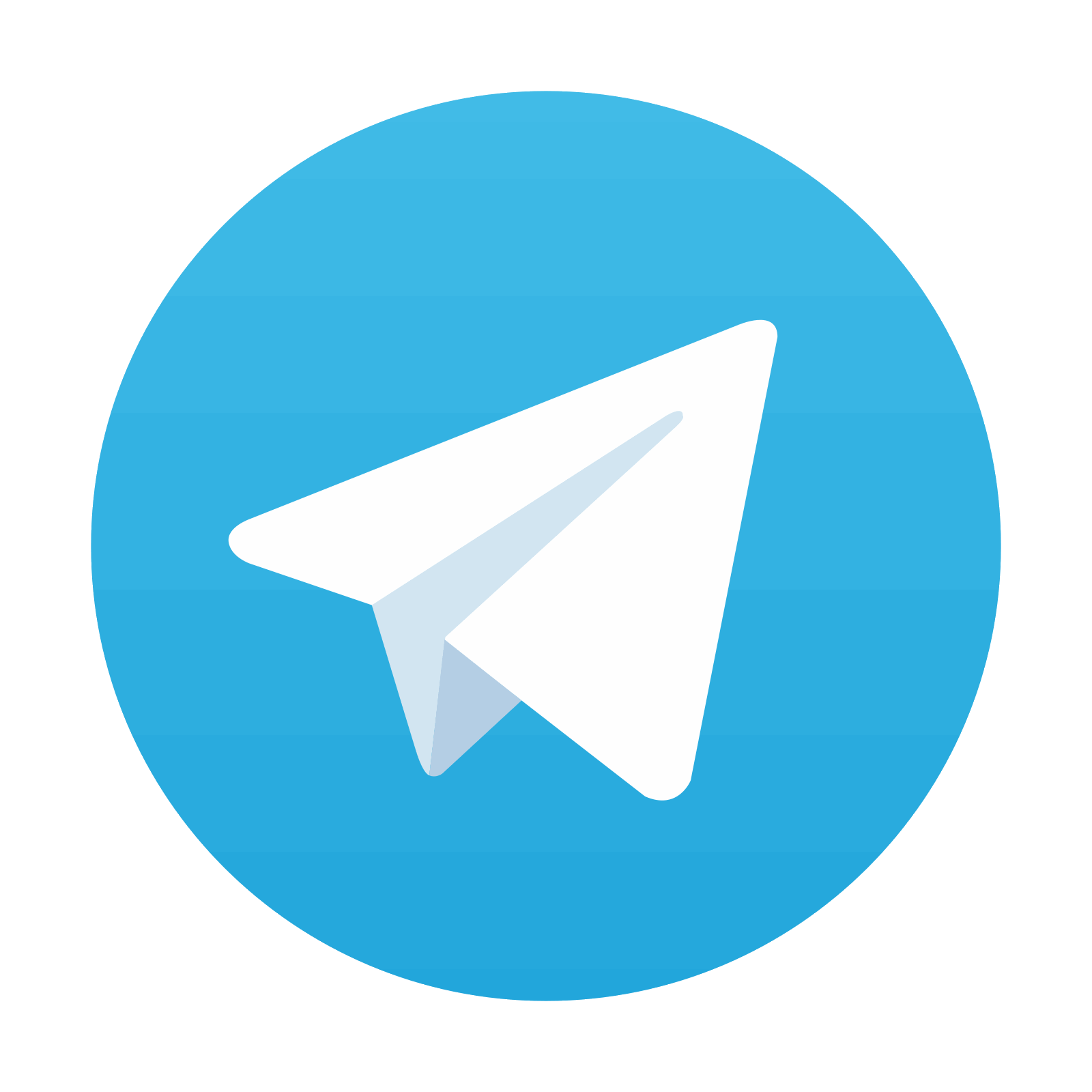
Stay updated, free articles. Join our Telegram channel

Full access? Get Clinical Tree
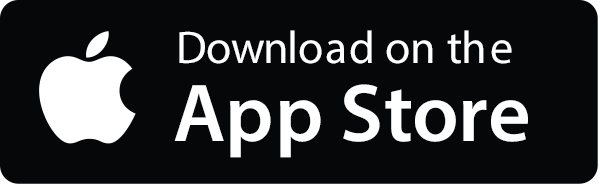

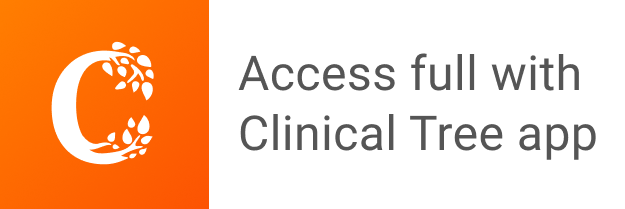