Artificial intelligence (AI) and informatics promise to improve the quality and efficiency of diagnostic radiology but will require substantially more standardization and operational coordination to realize and measure those improvements. As radiology steps into the AI-driven future we should work hard to identify the needs and desires of our customers and develop process controls to ensure we are meeting them. Rather than focusing on easy-to-measure turnaround times as surrogates for quality, AI and informatics can support more comprehensive quality metrics, such as ensuring that reports are accurate, readable, and useful to patients and health care providers.
Key points
- •
Realizing the potential of AI and informatics requires operational coordination and standardization across the imaging value chain and throughout the field
- •
Radiology quality definitions and metrics should incorporate more than just operational efficiencies as measured by turnaround times
Introduction
A common theme throughout this issue is the promise of artificial intelligence (AI) to impact nearly every step of the imaging value chain, from order placement to scheduling, image acquisition and reconstruction, worklist prioritization, diagnosis and interpretation, and reporting. Taken together, these efforts seek to improve the practice of diagnostic imaging, making radiology departments more precise and responsive, radiologists more accurate and efficient, and radiology reports more relevant and complete, culminating in improved patient outcomes and a more sustainable health care system.
Realizing these potential improvements will require substantially more strategic and operational coordination than in the past, as the various elements of the AI-enabled imaging value chain depend on and support upstream and downstream elements. Ensuring each component is based on nonproprietary standards, such as HL7 and DICOM, can mitigate some of the complexities of these intertwined workflows but still requires robust governance processes to identify deficiencies and react to change.
Measuring the improvements presents yet another challenge, in part because defining quality in radiology is complicated, in part because some elements of quality, such as diagnostic accuracy, are difficult to generalize, and in part because there are often competing interests, each with a different definition of quality.
Perhaps the most commonly cited definition of quality in the radiology literature is from Hillman and colleagues who stated:
Quality is the extent to which the right procedure is done in the right way at the right time, and the correct interpretation is accurately and quickly communicated to the patient and referring physician.
This definition of quality provides a good starting point and highlights the many links on the value chain that impact quality. This definition leaves open to interpretation or task-specific definition certain concepts relevant to AI in medical imaging, such as what “interpretation” means in this context, what constitutes “results,” and what format “communication” takes. For example, population-health approaches to mining historical computed tomographic (CT) scans for disease risk factors, such as osteopenia or coronary artery calcium, are opportunities to extract value from historical diagnostic imaging studies, but our traditional mode of generating formatted plain text reports that are filed away in the electronic medical record (EMR) seems inadequate and antiquated for such a workflow with discrete data output. Many similar examples are conceivable, some that would exist outside of a traditional radiology report and some that may be generated in the context of a narrative report but, nevertheless, should be logged in a structured database for more efficient retrieval and processing. The infrastructure to create and store discrete data, either embedded within or in addition to plain text reports, is not yet widely adopted, but this is an area of active development.
Kruskal and Larson provide a general definition of value that is more malleable to the changing state of AI-driven radiology services: “The concept of value simply boils down to understanding the needs and desires of those whom an organization serves, and continuously working to improve how well those needs and desires are met”. This conception of value puts the onus on each radiology group to identify their customers and work to understand their needs, and then develop the metrics and process controls to meet and exceed those needs consistently.
AI, and informatics tools more generally, are critical not only to driving quality improvement throughout the value chain but also in organizing and analyzing the data required to demonstrate that quality improvement.
Operational quality improvement
Informatics tools can improve both the accuracy and efficiency of operational workflows, including some tasks that precede image acquisition or reporting. One such task, the bane of existence for residents and fellows across the land, is examination protocoling—reviewing orders for diagnostic imaging studies, including free text examination indications as well as structured and unstructured data hiding in the nooks and crannies of the EMR, and then deciding on the appropriate series of patient preparatory and image acquisition steps to best address the clinical question. Machine learning methods that analyze free text examination indications and structured metadata, such as demographics, ordering service, and patient location, can automatically protocol neuroradiology MR imaging examinations with high accuracy. Examination indications, however, are often incomplete or even discordant with contemporaneous clinical notes from the same providers, highlighting the data quality chasms that still litter the EMR landscape and confound these clinical decision support and automated protocoling efforts. Successful and generalizable automated protocoling solutions will require highly integrated electronic health records (EHRs) that are actively designed to facilitate the accurate capture and transmission of clinical data, and to be successful those solutions will likely need access to a comprehensive data set, including EMR notes.
The LOINC/RSNA Radiology Playbook is the joint product of efforts by the RSNA and the Regenstrief Institute to standardize terminology of radiology procedures, which is a key prerequisite for the development of AI tools that could influence radiology operations upstream of image acquisition in a generalizable way. The ongoing development of these standardized terminology resources is essential, and yet adoption of the playbooks seems to be lacking based on the fewer than 10 PubMed references to either the LOINC/RSNA Radiology Playbook or its predecessor, the RadLex Playbook, in the nearly 10 years since the RadLex Playbook was first referenced in 2012.
Turnaround time (TAT), whether measured from order placement to final report, or examination complete to final report, is, for better or worse, one of the most common operational quality metrics in radiology. TAT expectations are built into most professional service agreements and are easy key performance indicators for hospital executive dashboards. With a mature informatics infrastructure, TATs between any steps of the process ( Fig. 1 ) can be easily calculated from readily available timestamps.

More complicated metrics can be informative but require a nuanced understanding of and accounting for workflow. For example, academic radiology departments must balance workflow priorities of the medical center with their obligations to support resident education and autonomy. At my institution, senior residents work independently overnight generating preliminary interpretations for patients in the emergency room, which are then finalized the following day by an attending. Our Quality and Safety (Q&S) Committee defined a metric to ensure that we are reviewing those overnight preliminary reports in a timely manner—we set a target that 80% of preliminary reports should be finalized by 9:30 am on weekdays and by noon on weekends. With this specific guidance from the Q&S Committee, our informatics group then developed a report for that metric for the bimonthly Q&S Committee meeting that distinguishes resident preliminary reports by radiology specialty, time of day, weekend versus weekday, and cross-sectional imaging versus radiography to address specific concerns of the various sections. This is an example of a radiology group working with the organization to identify a specific need (timely finalization of overnight preliminary reports) and then developing a metric to monitor how well we are meeting that desire. The metric is informative and specific while preserving section chief autonomy in deciding how to construct a schedule and workflow priorities to meet the desired performance goal. An example report is shown in Fig. 2 . Importantly it is the health care organization—not the informatics group—that determines what is valuable . It is sign of disorganization and a recipe for operational confusion to expect the informatics group alone to both define what is valuable and measure it—that process needs to be driven by department and organizational leaders.
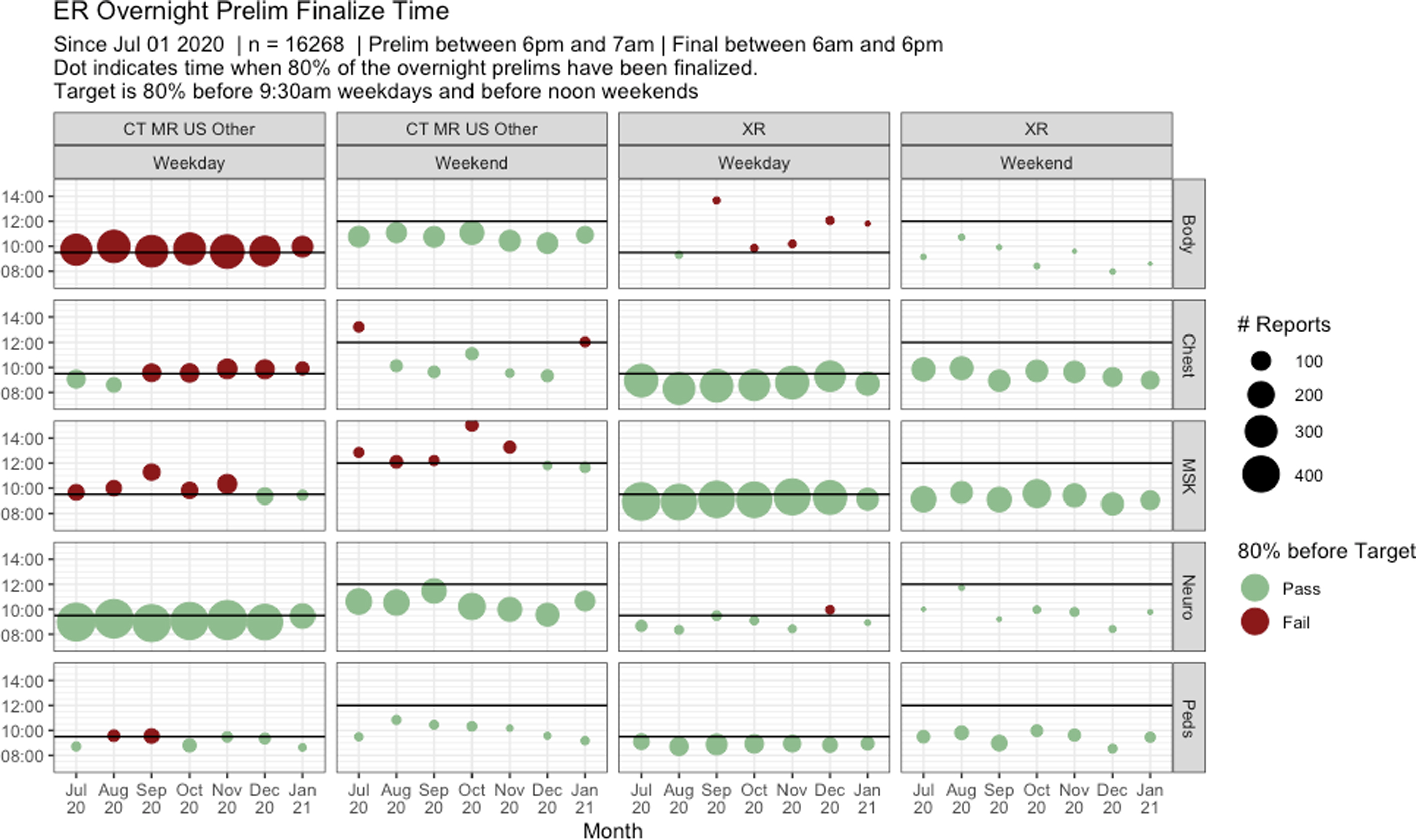
Realizing the potential opportunity for AI and informatics tools to significantly impact the examination ordering, scheduling, and protocoling components of the imaging value chain will require a common and standardized terminology for those examinations and their composite steps. The LOINC/RSNA Radiology Playbook provides that structure but needs broader acceptance and adoption.
Report quality improvement
Eberhardt and Heilbrun derive the following radiology report value equation by extending the common health care adage that value equals quality health outcomes divided by cost, and defining accuracy , utility , clarity , conciseness , and timeliness as the dimensions of radiology report quality:
ReportValue(RV)=(Accurate+Useful)∗(Clear+Concise+Timely)Cost
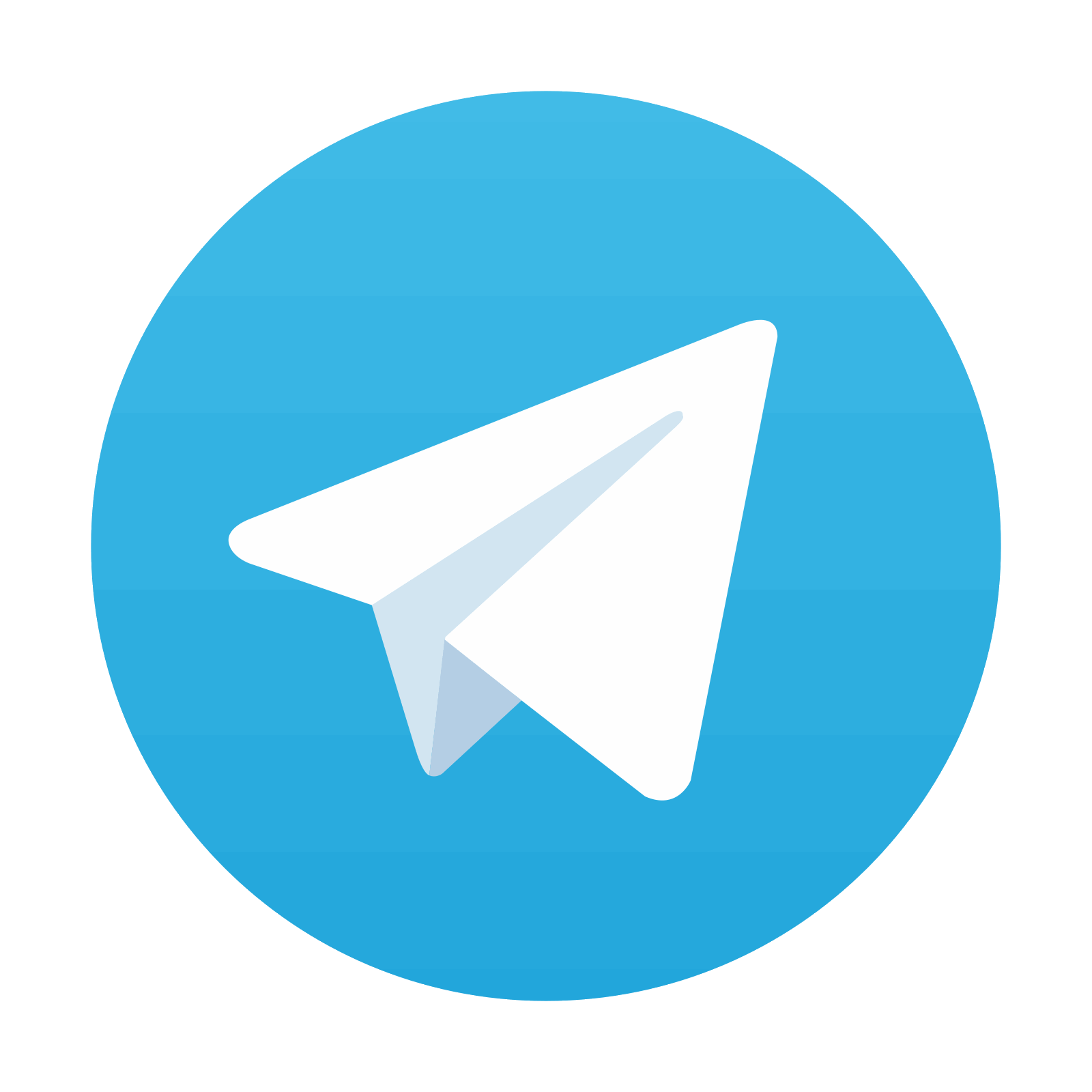
Stay updated, free articles. Join our Telegram channel

Full access? Get Clinical Tree
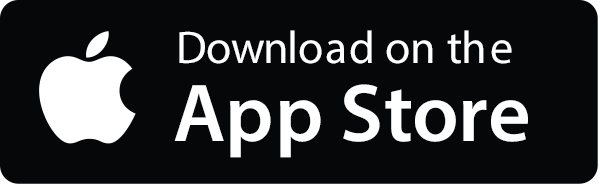

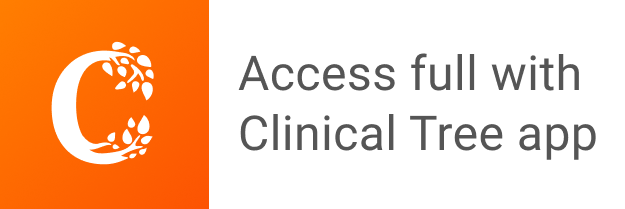