Fig. 1
The EEGLAB environment for electrophysiological signal processing is the center of a growing framework of tools developed and released by researchers at the Swartz Center for Computational Neuroscience (SCCN) at UCSD. These include software for synchronized multimodal recording (SNAP, LSL, XDF), MoBILAB, an object-oriented toolbox for analysis and visualization of multimodal data, the HeadIT data and tools resource with its associated tools (HED, ESS, etc.), and a growing set of toolboxes that operate as EEGLAB plug-ins (AMICA, DIPFIT, NFT, MPT, SIFT, BCILAB, etc.). MEEG is a new plug-in developed by the authors for analysis of MEG and MEEG (synchronized MEG plus EEG) data
Other tools. EEGLAB is the center of a growing ecosystem of open source software tools (Fig. 1) that have been released by researchers at the Swartz Center for Computational Neuroscience at UCSD (sccn.ucsd.edu). These include the Human Electrophysiology, Anatomic Data, and Integrated tools (HeadIT) data archive and resource (headit.org), with its system for tagging uploaded studies (Experimental Study Schema (ESS) (Bigdely-Shamlo et al. 2013a), Hierarchical Events Descriptors (HED) (Bigdely-Shamlo et al. 2013b) and a cross-platform system for synchronized collection of data from EEG and many other devices (Lab Streaming Layer (LSL), code.google.com/p/labstreaminglayer) plus an extensible, XML-based data format (Extensible Data format, XDF; code.google.com/p/XDF) and a Python-language scripting framework for controlling simple or very complex experimental paradigms (SNAP).
MoBILAB. An object-oriented environment for analysis of multimodal data collected under the mobile brain/body imaging (MoBI) paradigm, MoBILAB (sccn.ucsd.edu/wiki/Mobilab_software) can export EEG data to EEGLAB for further analysis, and may in the future become our primary platform for developing and sharing multimodal data analysis methods, since the EEGLAB EEG data structure has limited support for different channel types and assumes all data to be recorded at the same sampling rate. For MEG/EEG data recorded at the same rate this is not much of an inconvenience, as EEGLAB provides a channel type variable that allows functions to perform EEG analysis and/or MEG analysis of the respective data channel subsets based on their specified channel types.
EEGLAB plug-ins. The growing range of EEGLAB plug-ins have been previously described (Delorme et al. 2011). Plug-ins released by SCCN itself include advanced Adaptive Mixture ICA (AMICA) for identification of maximally independent brain sources with artifact rejection (Delorme et al. 2012; Palmer 2006), the DIPFIT toolbox implementing source dipole fitting tools by Robert Oostenveld from Fieldtrip (fieldtrip.fcdonders.nl), the Neuroelectromagnetic Forward Head Modeling Toolbox (NFT) for creating detailed boundary element model (BEM) or finite element model (FEM) head models (Akalin Acar and Makeig 2010), the Measure Projection Toolbox (MPT) for cross-subject source-level analysis using measure projection (Bigdely-Shamlo et al. 2013c), the Source Information Flow Toolbox (SIFT) for calculation and visualization of multivariate causal source dynamics in both event-related and continuous data (Delorme et al. 2011), and BCILAB, a complete toolbox for building, running, and statistically evaluating brain-computer interface (BCI) models (Kothe and Makeig 2010). At least 20 other plug-in tools and toolboxes have been released by other research groups; these are listed on a wiki page (sccn.ucsd.edu/wiki/EEGLAB_Plugins). A facility for automated updating of listed plug-ins to new versions from within EEGLAB is planned for EEGLAB v13.
The MEEG plug-in. EEGLAB now includes an MEEG plug-in (sccn.ucsd.edu/wiki/MEEG) that expands the ability of EEGLAB users to import and analyze MEG and dual-modality MEEG (concurrent MEG and EEG) datasets, thereby opening a range of novel data analysis techniques for use by the MEG community. MEEG data handling within EEGLAB is tightly coupled to Fieldtrip, allowing the EEGLAB data structures to be readily imported from and exported to Fieldtrip. Both the EEGLAB environment and the MEEG plug-ins are ongoing efforts that we hope other MEG users and methods developers will contribute to. The MEEG developers remain open to partnering with other methods developers to share capabilities between MEEG and other MEG toolboxes.
Data and experiment types supported. In addition to standard EEG data types, EEGLAB now supports the loading of MEG and MEEG data through its integration of the Fieldtrip fileio module. Individual data files can be imported as individual EEGLAB data sets, or multiple runs can be combined into a single dataset using realignment to a common sensor orientation. In addition, the new MEEG plug-in enables EEGLAB to import and export a range of Fieldtrip data structures, including raw and epoched data, as well as independent component analyses, so that EEGLAB processing can begin after partial analysis in Fieldtrip, or can be exported, allowing Fieldtrip to be used for additional processing. EEG recording systems provide a single scalar value per sensor location, in contrast to the wider variety of MEG sensor types. The scalar model easily accommodates magnetometer and radial gradiometer systems, but requires either magnetometers or the magnitude of the planar gradient to be chosen (e.g., for Yokogawa system data sets).
Source localization. ICA decomposition enables the profitable use of dipole-based inverse methods because of the characteristic resemblance of many MEG, EEG, or also MEEG independent component scalp maps to the projection of a single equivalent dipole, allowing them to be well-fit by a single equivalent dipole model (or, in some cases, to a dual-dipole model with symmetric location constraints) (Delorme et al. 2012). The DIPFIT toolbox in EEGLAB implements equivalent dipole model fitting tools by Robert Oostenveld from Fieldtrip (fieldtrip.fcdonders.nl). Dipole fitting tools have been integrated in the Neuroelectromagnetic Forward head modeling Toolbox (NFT) (Akalin Acar and Makeig 2010). These plus some novel distributed source localization methods will be put into a toolbox paralleling NFT, to be called the Neuroelectromagnetic Inverse Source modeling Toolbox (NIST).
Processing data from multiple subjects or sessions. EEGLAB supports across-subject analysis via a STUDY structure that points to a set of similar EEG datasets forming an experimental study. Currently, these datasets are typically epoched datasets (sets of data epochs similarly time locked to one or more sets of experimental events). EEGLAB Study software can prepare and store a user-specified set of continuous (power spectrum) and event-related (ERP, ERSP, ITC, etc.) measures for each dataset and help the user to separate these measures into conditions, sessions, and/or subject groups. Typically, each dataset is associated with an ICA decomposition and a list of ‘brain’ components to study, each with an equivalent dipole model. The Study functions can then prepare a pair-wise distance measure between components based on component dipole (and/or scalp map) and specified measure distances. Users then can cluster the components using at least three clustering methods, and can compute statistical contrasts across subjects/sessions using either parametric (Gaussian) or non-parametric (bootstrap) statistical methods. Clustering scalp channel signals, though less advised, is also supported.
Currently, users can create and process one or more 1 × N or N × M statistical designs for a given Study. Thus, for example, given 5 different event-related measures for each subject in an experiment, the user can specify Conditions 1–4 as forming a 2 × 2 design, and/or can also compare Conditions 2 versus 5 in another design, without needing to duplicate the STUDY structure and its associated measure files. Both within-subject and across-subject variable types are supported.
As in practice the range of experimental designs is much wider (than NxM), EEGLAB and some EEGLAB toolbox developers are now working with Cyril Pernet of the University of Glasgow to incorporate his LIMO toolbox into the core of EEGLAB study processing. It supports parametric and non-parametric statistics for a much wider range of designs (gforge.dcn.ed.ac.uk/gf/project/limo_eeg) (Pernet et al. 2011).
Measure projection. An alternate approach to component clustering is taken in the Measure Projection Toolbox (MPT) (Bigdely-Shamlo et al. 2013c). This toolbox focuses on comparing component source dynamics for a single measure at a time (for example, ERPs) based on the location of the equivalent source dipole in a template brain. Each component dipole location is replaced by a 3-D Gaussian blur (representing location probability) and, after populating the template brain with source dipoles across a potentially large number of subjects, two operations are applied voxel-wise (that is, template brain voxel-by-voxel). First, brain regions in which local dipole measures agree are identified, forming a measure consistency subspace. Next, voxels in this subspace are clustered using affinity clustering to form voxel domains with distinct measure time courses. Here the concept of measure domains in the template brain volume replaces the discrete component clusters produced by the default EEGLAB study processing. Users may choose either or both paths to use to characterize their study data.
CSA clustering. Arthur Tsai of Academica Sinica, Taiwan, has recently developed an advanced approach to study source clustering (Tsai et al. 2013). This applies spatiotemporal ICA decomposition using EMSICA (Tsai et al. 2006) to EEG (or as readily, MEG) data from its projection back onto to the oriented subject cortex, modeled from a subject MR head image. The cortical surface models are then inflated and co-registered using tools available in Freesurfer (Fischl et al. 1999). Finally, source clustering across subjects is performed in the 2-D cortical surface-aligned space rather than in 3-D template brain space (as in MPT and EEGLAB Study functions). A CSA (Cortical Surface Alignment) EEGLAB plug-in is envisaged that will allow users to perform this potentially more accurate analysis when MR head images are available for the individual subjects in an EEG or MEG study.
2 MEEG Data Decomposition: An Empirical Data Example
For example purposes, we will illustrate the capabilities of the MEEG plug-in and other EEGLAB features using a simultaneously recorded multimodal (MEEG) MEG plus EEG dataset (Bledowski et al. 2012) that is jointly decomposed, in a single AMICA decomposition, to extract independent components accounting for both MEG and EEG data streams. The validity of the decomposition is based on the assumed linearity of the underlying electric and magnetic components of the electromagnetic field generated by the effective generators of the scalp-recorded (EEG) potentials and (MEG) flux. We use the NFT toolbox to create an EEG and MEG head model and use it to fit equivalent dipole models to the resulting independent component (IC) scalp maps. We focus here on describing the relations between MEG signal and EEG signal projections of the resulting ICs, including a first statistical examination using ICA of the degree to which radial EEG sources (as determined by an equivalent dipole model) are also visible in MEG.
Data loading and preprocessing. The epoched CTF dataset included time series data from 269 radial gradiometers (3rd-order synthetic) plus 56 EEG channels. Five separate runs from the same recording session were imported and merged into a single EEGLAB dataset of size 325 channels by 580 k time points. The MEEG toolbox enabled the selection of alignment across runs of the MEG data (e.g., projection onto the average across-run gradiometer locations using Fieldtrip ft_megrealign) as well as the choice (when appropriate) of synthetic gradiometer order. Field contributions from external sources were removed by computation of third order gradients using contributions from reference sensors (Fife 1999). The resulting EEGLAB dataset included 324 channels and 136 6-s data epochs. These data were down sampled from 1200 to 600 Hz, and the EEG channels were average referenced. One EEG channel was dropped following these procedures to keep the data full rank.
Artifact detection and rejection. A range of artifact rejection options are available in EEGLAB, both automated and interactive data rejection or cleaning, as well as ICA-based artifact rejection. For the dataset used here, epochs containing large artifacts had previously been rejected based on visual inspection.
Independent Component Analysis. The MEEG data were analyzed using AMICA to find independent components across the modalities. ICA in general proceeds from the observation that the signal measured at any sensor is a linear mixture of multiple sources within the brain (Makeig et al. 1996). The goal of the algorithm is to learn an unmixing matrix across all channels that results in a complete decomposition of the data into maximally independent components (ICs). In single-modality MEG or EEG data, many ICs have dipolar patterns of projection onto the sensors (Delorme et al. 2012
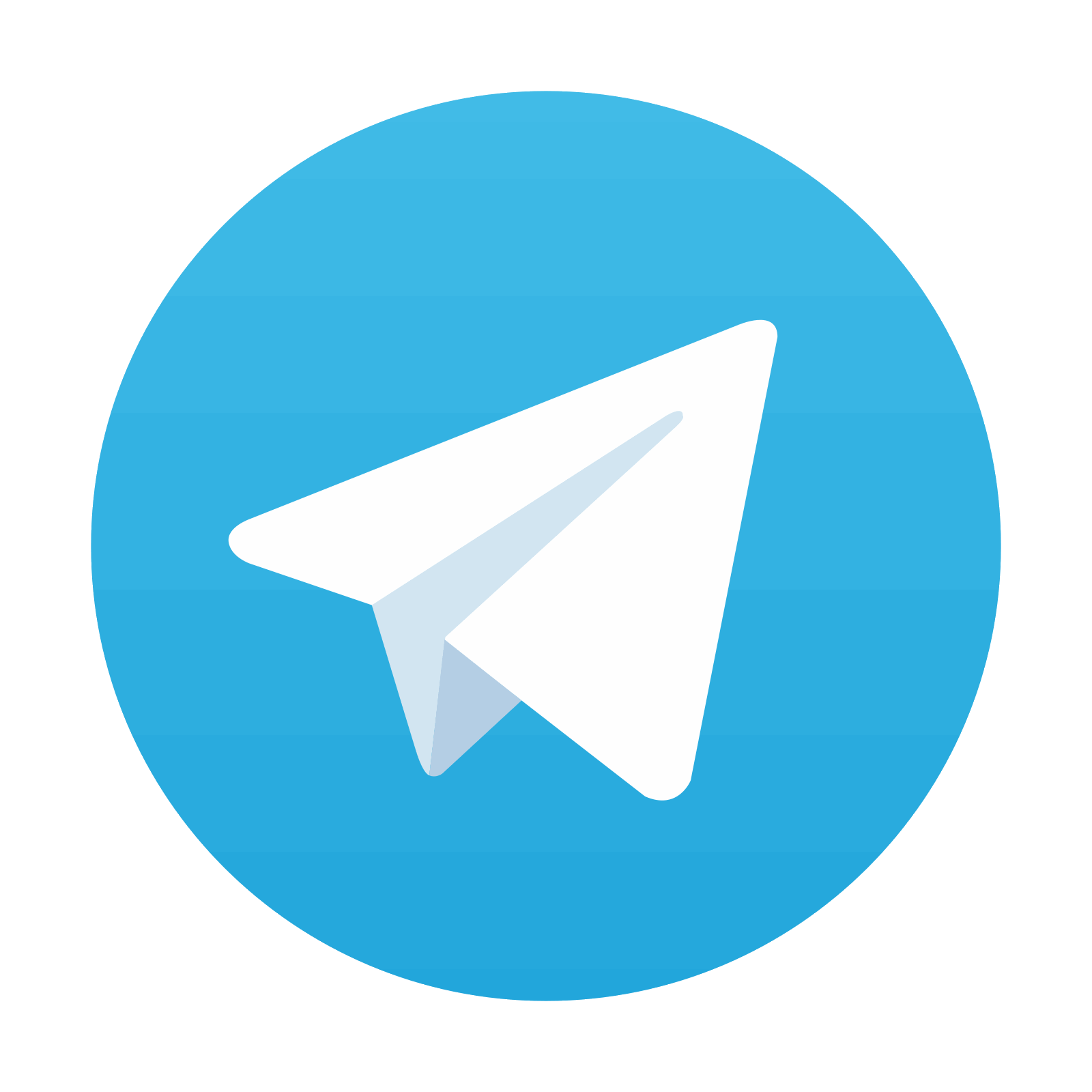
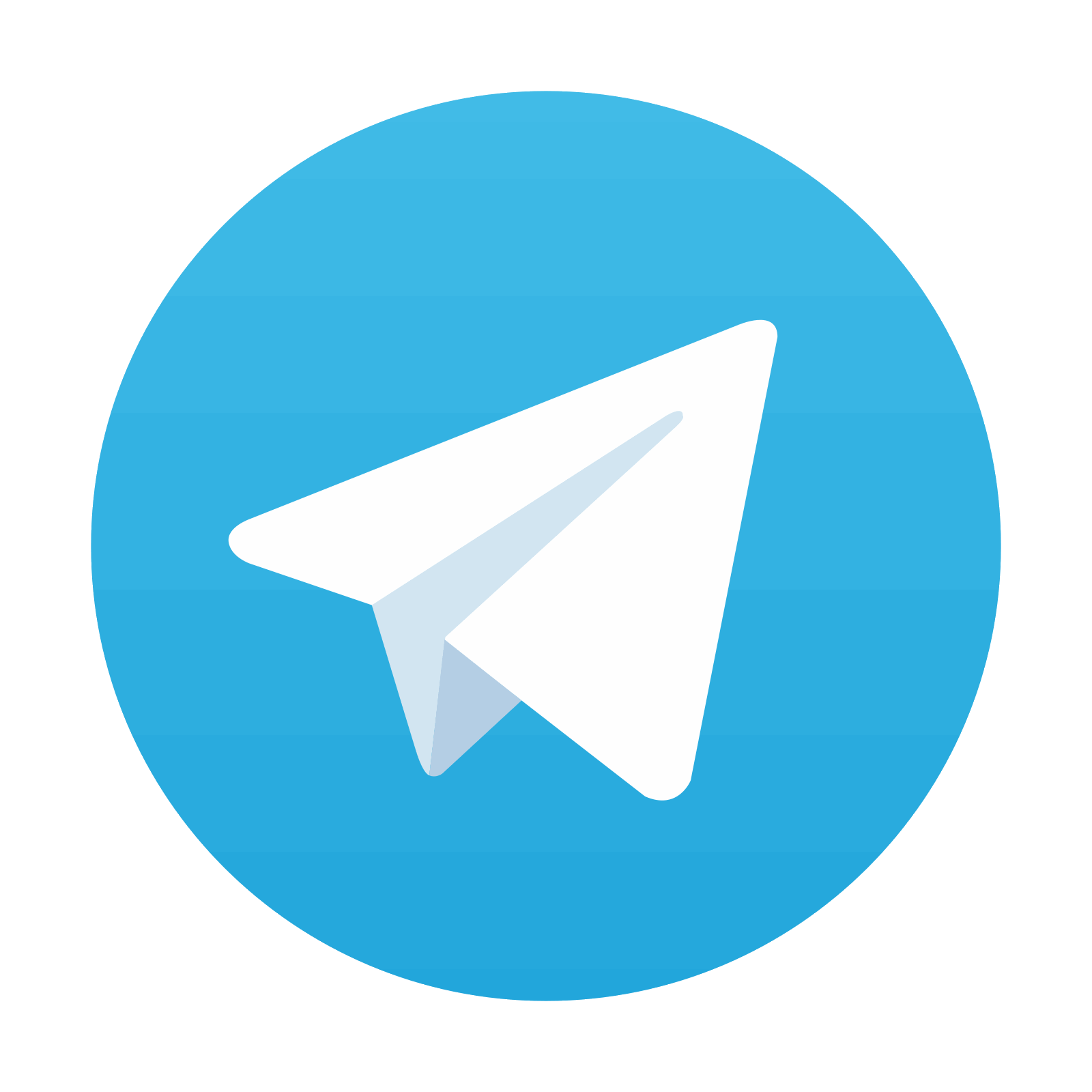
Stay updated, free articles. Join our Telegram channel

Full access? Get Clinical Tree
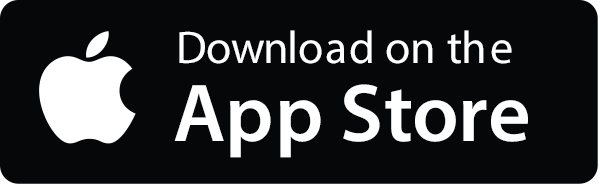
