Fig. 5.1
Original image (Exudative maculopathy)
From observing Fig. 5.1 the Exudates are seen as yellow patches on the retina. Figure 5.2 is the resultant image of the proposed method. But here the Exudates are not clearly visible. Thus Intensity adjustment is carried out to extract the features in a visible way as shown in Fig. 5.3.
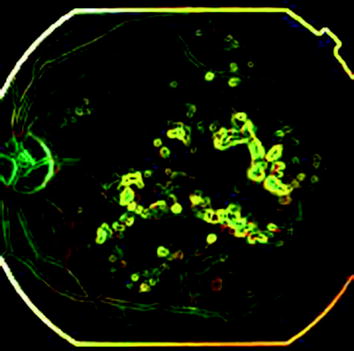
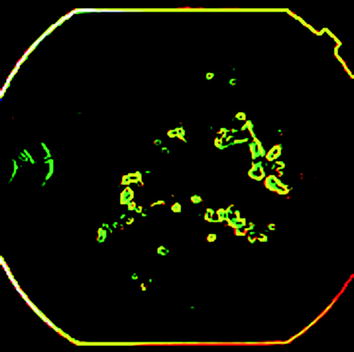
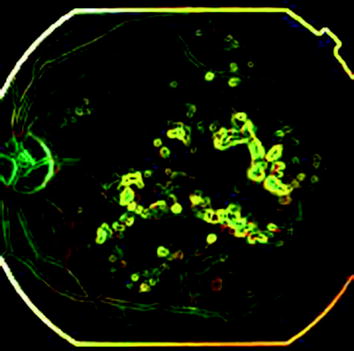
Fig. 5.2
Edge detection (Gradient filter technique)
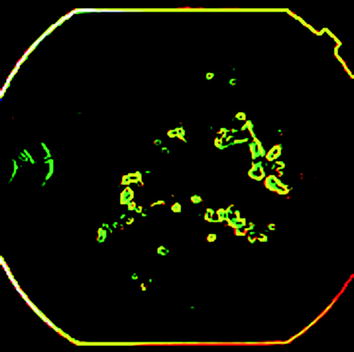
Fig. 5.3
Intensity adjusted image
5.2 Detection of Maculopathy Using Artificial Neural Networks
The area covered by the exudates in the four regions (R1, R2, R3 and R4) are considered as parameters for identifying the severity in the maculopathy images. The input data for the ANN Classifier is the total number of white pixel which indicates the exudates in each region.
In normal and non-CSME images, the R1 and R2 values are zero due to the black area on the foveola and fovea regions. Whereas there are R1 values in the minority of the CSME images and R2 values in all CSME images as compare to other images due to the number of white pixels exist in these regions.
In majority of the CSME images, the R3 and R4 values are higher as compare to non-CSME images due to the area tends to be white. Thus the number of white pixels is more in this class. In normal image, the values are zero due to black area on the parafovea and perifovea regions.
5.3 Results and Test Performance
A data base of 90 samples is used for training and testing the classifiers. These samples are divided into 90 % training and 10 % for testing. The performance measures such as sensitivity, specificity and positive predictive accuracy are used to evaluate the performance of the systems.
The sensitivity, specificity and positive predictive accuracy are computed as below
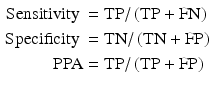
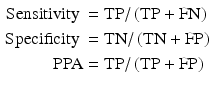
5.4 Normal Retina
From Fig. 5.4c all the areas of macula have no white spots which are exudates. So this image is a healthy retina with no macular part affected.
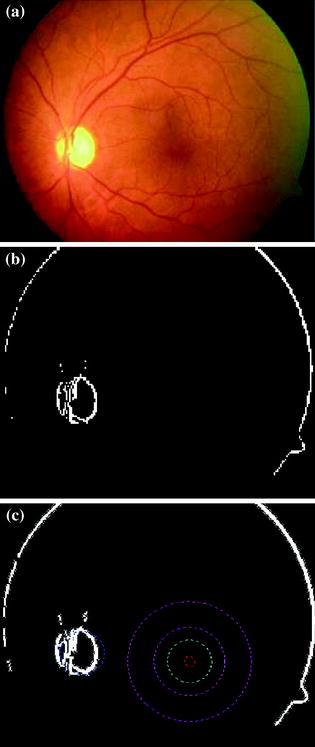
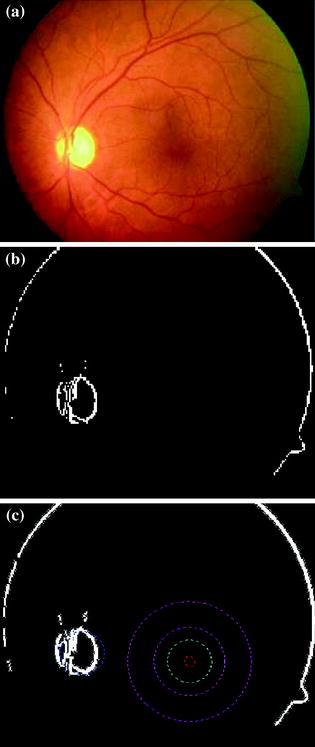
Fig. 5.4
Normal retina: a Input image. b Segmented image (Kirsch segmentation). c Graded Image (Artificial neural network)
5.5 Non-Clinically Significant Macular Edema (Non-CSME)
Some Exudates are present in the Macular area, but only the outer most part of macula is affected, so from Fig. 5.5c the Image has Non-Clinically Significant Macular Edema (Non-CSME).
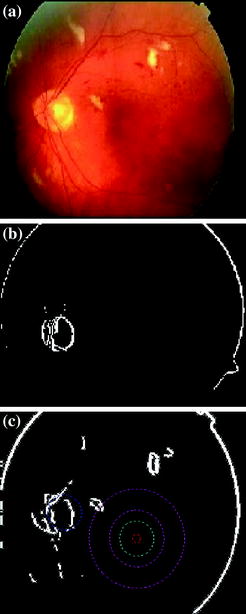
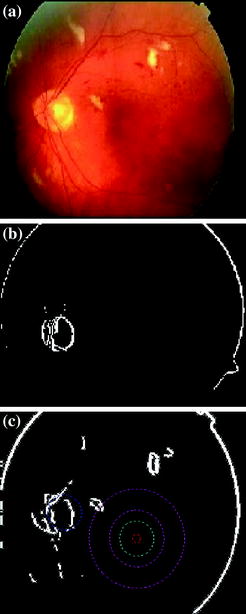
Fig. 5.5
Non-clinically significant macular edema: a Input image. b Segmented image (Kirsch segmentation). c Graded image (Artificial neural network)
5.6 Clinically Significant Macular Edema (CSME)
More Exudates are present in the Macular area, two parts of macula are affected severely from Fig. 5.6c. The Image is having Clinically Significant Macular Edema (Non-CSME). Since the exudates count is more.
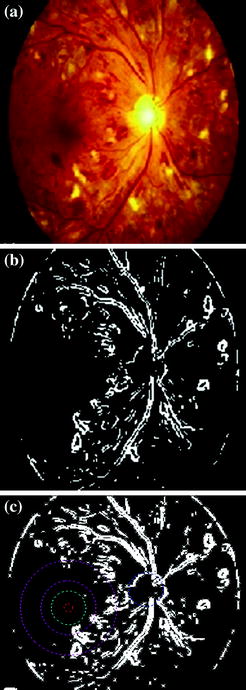
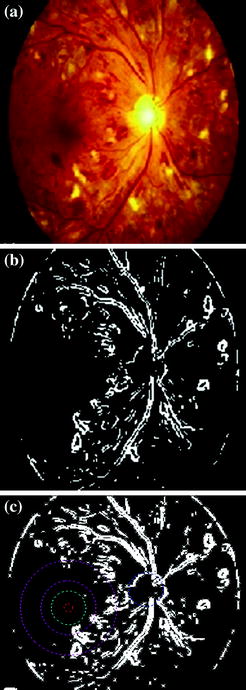
Fig. 5.6
Clinically significant macular edema: a Input Image. b Segmented image (Kirsch segmentation). c Graded image (Artificial neural Network)
Diabetic maculopathy is resulted from the prolonged diabetes retinopathy and is a leading cause of blindness. It occurs when the retinal blood vessels are damaged and the exudates leakage area increases, deposit very close to the fovea. Hence, an automatic system for identification of normal, Non-CSME and CSME fundus eye image is proposed. The features from the raw images are extracted using image processing techniques, and fed into the feed-forward neural network classifier for classification. We have concluded that feed-forward neural network classifier perform better with an accuracy of more than 96 % of correct classification, sensitivity of more than 96 % and specificity of 100 %. The accuracy of the system can further be improved using proper input features such as microaneurysms and Haemorrhages, and the size of the training data.
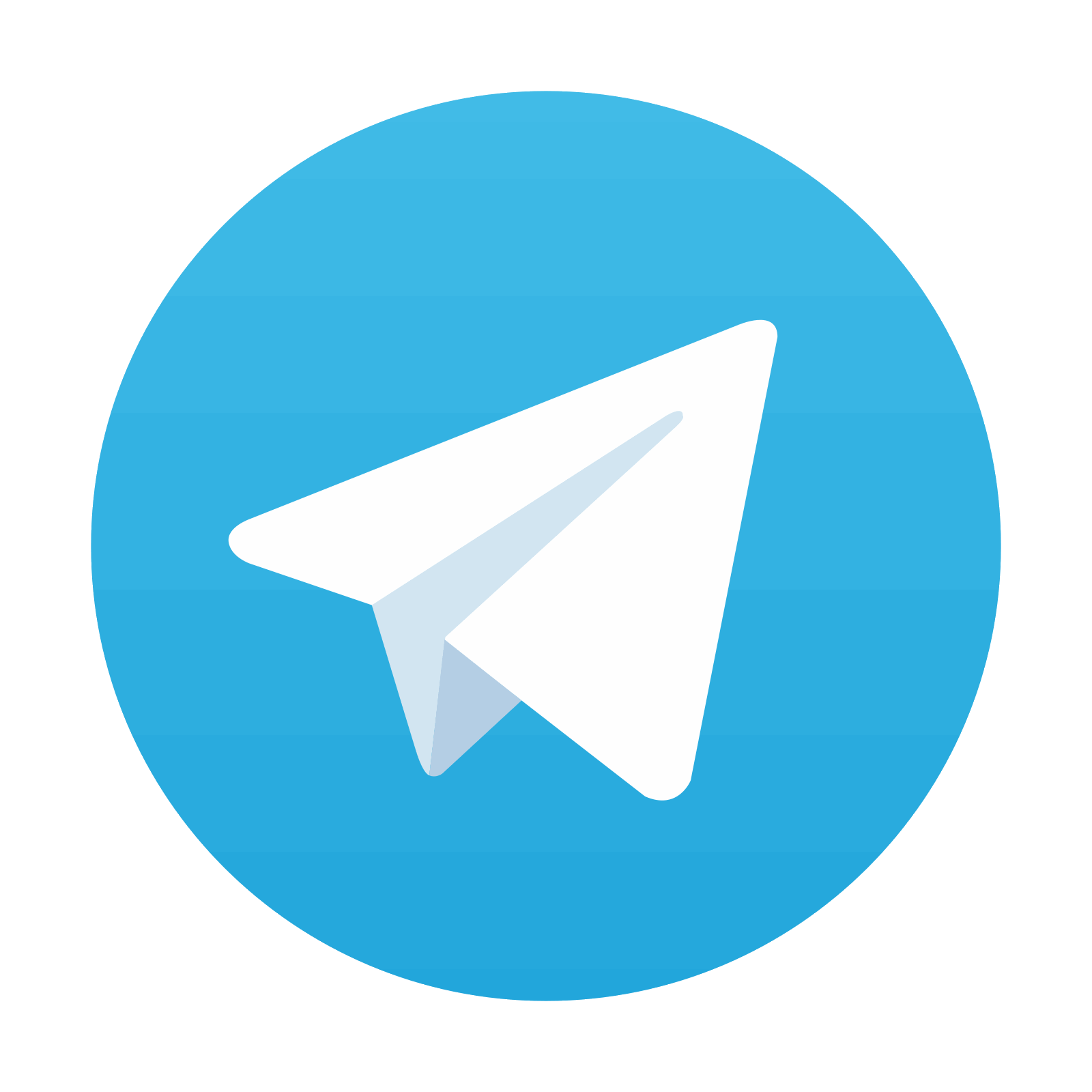
Stay updated, free articles. Join our Telegram channel

Full access? Get Clinical Tree
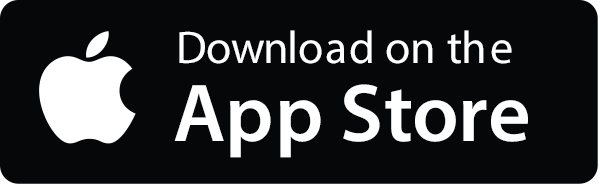
