Fig. 1
Schematic diagram showing neural systems and flow of information involved in the control of food intake and regulation of energy balance. Internal regulatory circuitry using neural and hormonal feedback on hypothalamus and brainstem is shown at the bottom (dark grey boxes). Sensory and cortico-limbic brain areas used for processing information from the environment are shown in the upper half (light gray boxes). The extensive influence of circulating and neural internal feedback signals on sensory processing and cortico-limbic systems concerned with reward, emotion, learning and memory is emphasized (dashed lines) (Figure with permission from Shin et al. 2009)
2.1 Physiology of Homeostatic Control of Food Intake
Most adults are characterized by a relatively stable body weight, even if daily food intake and energy expenditure (in the form of basal metabolism, adaptive thermogenesis, and physical activity) underlie huge variations. The balance between energy intake and expenditure is regulated by a complex physiological system with the hypothalamus as a key structure for regulating appetite by integrating signals from central and peripheral pathways (Suzuki et al. 2010; Mayer and Thomas 1967).
The hypothalamic network involved in the control of feeding and energy metabolism is composed of interconnected neuronal populations located in the arcuate nucleus, ventromedial nucleus, paraventricular nucleus, dorsomedial nucleus, and lateral hypothalamus (Saper et al. 2002; Berthoud and Morrison 2008; Dietrich and Horvath 2009). This network receives peripheral signals that can be divided into anorexic (reduction of food consumption) and orexic (increase of food consumption) signals. These signals encode the amount of circulating nutrients, such as glucose, fatty acids, or the amount of stored energy and are conveyed by hormones like insulin, leptin, and ghrelin with their concentrations directly connected to food intake, amount of adipose tissue, or gut peptide concentrations (Cummings et al. 2001; Woods and Seeley 2000). In addition, these peripheral signals can also be conveyed by direct activation of the brainstem by gut peptides via vagal afferents or by arcuate nucleus neurons with the ability of sensing energy-rich nutrients directly (Suzuki et al. 2010). These peripheral signals are then integrated and the information is encoded by neuropeptides and other neurotransmitters (Meister 2007) connected to circadian rhythm, thermoregulation and arousal (Saper et al. 2002). In principle, the output of the hypothalamic network is two-fold. For one, the hypothalamic network exhibits projections to sympathic and parasympathic nuclei innervating the endocrine organs and the gastrointestinal tract (Williams et al. 2001) to send feedback signals to the periphery. Secondly, the hypothalamic network is interconnected with cortical and mesolimbic control circuits controlling food reward to initiate or terminate food intake (Hommel et al. 2006; Kampe et al. 2009).
2.2 Cognitive Elements of Eating Behavior
Food intake, however, is not regulated by this homeostatic system alone. For actual behavioral activation to pursue food intake, integration of information from other systems like sensory, reward, and cognitive networks is required. This results in complex interactions between the systems, which so far are not well understood.
For a long time in evolution, food and especially high fat, energy-dense, palatable food was scarce. Thus, consumption of such high palatable food had clear homeostatic and social benefits. As a result, reward-driven or “hedonic” feeding has developed. Besides increased motivation for obtaining highly palatable food, hedonic feeding is also characterized by the consumption of this food beyond current needs and saving it in form of fat (Berthoud 2007).
Reward-associated feeding is regulated by a complex reward circuitry, involving interactions between several signaling systems, including opioid, dopaminergic, and cannabinoid systems (Wynne et al. 2005). In hedonic feeding, a differentiation between “liking”, the sensory pleasure from eating a palatable food, and the motivational process of “wanting” (an incentive value is attributed to a food stimulus) has been suggested (Berridge 2007). As a neurophysiological basis for liking of palatable food, opioid neurotransmission in the nucleus accumbens has been discussed (Kelley and Berridge 2002). Opioate antagonists reduce food palatability in man (Yeomans et al. 1990) and reduce palatable food intake in animals and their affective facial expressions in response to tasting palatable food (Pecina and Berridge 2005). Wanting is thought to be mediated by the mesolimbic system including dopaminergic projections from the ventral tegmental area to the nucleus accumbens (Kelley and Berridge 2002) and hyperdopaminergic mice have a higher motivation to consume palatable food without changes in the pleasantness perceived for it (Pecina et al. 2003).
Interactions between the homeostatic and hedonic networks have been addressed in several studies, but the picture is far from being complete. Cabanac (1971) showed that sweetness is rated less pleasant after subjects had ingested glucose syrup. Other studies were also able to show that subjective palatability is different in the fed and in the fasted state (Berridge 1991). Therefore, the nutritional status of a subject can influence the rewarding effects of food. Besides direct action of leptin, insulin, and ghrelin in the hypothalamic structure, they have also been shown to influence neurons in the ventral tegmental area, nucleus accumbens, and amygdale (Kampe et al. 2009, Shin et al. 2009). For instance, leptin can inhibit firing of dopaminergic neurons and feeding-induced dopamine release in the nucleus accumbens (Fulton et al. 2006; Hommel et al. 2006). Ghrelin on the other hand, stimulates firing of dopaminergic neurons and dopamine release in the nucleus accumbens (Abizaid et al. 2006). Functionally, also direct neuronal connections between hypothalamic, cortical, and mesolimbic circuits exist (Hommel et al. 2006). The neurons in the arcuate nucleus project to the insular and anterior cingulate cortex via midline thalamic nuclei, while neurons in the lateral hypothalamus project to the shell of the nucleus accumbens and the cerebral cortex (Kampe et al. 2009).
In our modern society with high energy food easily accessible, hedonic feeding would promote a positive energy balance in the long run. However, most individuals stay at a relatively stable body weight. Consequently, additional mechanisms of executive control and decision making in the control of eating behavior have been suggested (Appelhans 2009). Considered in the context of evolution, cognitive control of hedonic feeding is a valuable mechanism allowing for conservation of food during periods of anticipated food shortage (Polivy and Herman 2006) or in social interactions. The second aspect is still traceable in our modern society. In company of others, individuals match their food intake to those around them, regardless of hunger or satiation (Herman et al. 2003).
Cognitive control of eating behavior is considered to involve networks responsible for cognitive control of behavior in general. The primary neuroanatomical basis for self-regulation and executive function is the prefrontal cortex (PFC). The PFC is considered to exert top-down control over automatic cognitive and affective processes to inhibit responses to environmental cues with unfiltered emotionally driven behaviors (Miller and Cohen 2001). Regarding inhibition of hedonic feeding, the dorsolateral region of PFC (DLPFC) is activated after ingestion of a meal (Gautier et al. 2001; Tataranni et al. 1999). It seems that prefrontal cortex is important for inhibition of hedonic feeding; its interplay with the homeostatic system, however, is not clear.
Finally, sensory processing channels allowing detection and interpretation of environmental food cues interact with and are modulated by these neural systems involved in control of eating behavior. In many cases, food is first perceived by the visual system. The visual stimulus of food signals availability and provides information about palatability and therefore, is a key factor in the initiation of a meal (Cornier et al. 2007). Not only viewing of real food, but also viewing of food pictures has been shown to activate distributed networks of brain regions involved in food categorization and also in reward evaluation, such as amygdale and orbitofrontal cortex as well as the hypothalamus (Berthoud 2004; Rolls 2005; Cornier et al. 2007).
In tasks assessing attention allocation, visual selective attention is preferably directed towards food items (Nummenmaa et al. 2011). Activity in these networks and the degree of attention allocation is highly dependent on motivational states, either by the motivational significance of external cues or by the intrinsic current need state. For instance, only the attention capture effects of food items, but not non-food items, as well as the memory performance for visually presented food items is strongly modulated by an individual’s hunger level (Mogg et al. 1998; Morris and Dolan 2001; Piech et al. 2010). Furthermore, in an electroencephalographic (EEG) study, Stockburger et al. (2008) showed that hunger state modulated brain potentials selectively to food pictures. Food specific correlations between regions involved in processing of visual food cues with states of hunger and calorie content of the food cues were also found by means of functional magnetic resonance imaging (fMRI) (Porubska et al. 2006; Siep et al. 2009; Fuhrer et al. 2008; Cornier et al. 2007). It appears that the hunger state induces a change in the salience of food stimuli as need-related cues.
3 Role of Insulin in Eating Behavior
Although individual systems of eating behavior control have been described to some detail, interactions between them are still rather elusive. Manipulation of a key hormonal signal and investigation of its effects on cognitive elements in eating behavior might shed some light on these interactions in humans.
3.1 Cerebral Insulin Function
As previously described, one of the key signals is the hormone insulin, which regulates the metabolism of carbohydrates, lipids and amino acids. Insulin interferes with the metabolism of all three types of nutrients; however, the most important function of insulin is the regulation of glucose metabolism in peripheral tissues. Since glucose uptake in neurons is independent of insulin, the central nervous system has long been considered an insulin-independent tissue. However, insulin receptors are expressed all over the brain with high concentrations in the olfactory bulb, the primary olfactory cortex, the limbic and paralimbic system and the hypothalamic nuclei (Baskin et al. 1987; Unger et al. 1991; Schulingkamp et al. 2000).
Currently, it is well established that insulin affects eating behavior and metabolism by targeting the hypothalamic nuclei (e.g. ventromedial nucleus, arcuate nucleus) (Woods et al. 1979; Schwartz et al. 2000; Benoit et al. 2002). Furthermore, insulin is an essential neuronal growth factor and stimulator of neuronal protein synthesis during development of the central nervous system (Heidenreich and Toledo 1989; Robinson et al. 1994; Choi et al. 2005; Chiu and Cline 2010). Insulin has also been shown to co-regulate neurotransmitters and/or their receptors including norepinephrine (Boyd et al. 1985; Masters et al. 1987; Figlewicz et al. 1993; Apparsundaram et al. 2001), acetylcholine through glucose utilization (Lechin and van der Dijs 2006), glutamate receptors (Man et al. 2000; Plitzko et al. 2001; Mielke et al. 2005; van der Heide et al. 2005), and GABA-ergic transmission (Wan et al. 1997; Ma et al. 2003; Vetiska et al. 2007). The majority of these insulin effects on neurotransmission are localized in the hippocampus, prefrontal cortex, and hypothalamus.
Thus, it is not surprising that an important role for insulin in high-level cognition, memory, and synaptic plasticity has been suggested. Animal studies demonstrated that rats which were injected intracerebroventricularly with insulin after a training task showed increase in performance if tested for retention in comparison to control animals (Park et al. 2000). Improvement of memory function by insulin administration was also shown in humans (Craft et al. 2000; Benedict et al. 2004).
3.2 Cerebral Insulin Resistance in Humans
For the investigation of insulin effects on cognitive elements of eating behavior, there are in general two ways for manipulating insulin levels and action in the human brain. One approach is to raise insulin levels in the body either by a hyperinsulinemic euglycemic clamp, which increases the plasma concentration of insulin without changing the glucose level, or by administration of intranasal insulin. The advantage of intranasal insulin is that it raises insulin concentration in the cerebrospinal fluid without relevant absorption in the systemic blood circulation as it enters the brain via the olfactory nerve (Illum 2000; Born et al. 2002). The other approach is to study eating behavior in a model with impaired insulin function. Obesity is associated with peripheral insulin resistance. In addition, the deletion of central insulin receptors in rats and mice is accompanied by hyperphagia and obesity (Bruning et al. 2000; Obici et al. 2002).
In a recent MEG study, we investigated insulin action in resting state networks/spontaneous brain activity to evaluate association of cerebral insulin resistance and BMI in humans. Spontaneous brain activity is characterized by the presence of more or less regular oscillations in various frequency bands (δ = 1–4 Hz, ϑ = 4–8 Hz, α = 8–12 Hz, β = 12–30 Hz, γ > 30 Hz). These oscillations are generated by specific brain areas and networks and are quite stable at rest. Any input to the system and/or information exchange between spatially separated areas is accompanied by power/and or phase synchronization in specific frequency bands (Schnitzler and Gross 2005). This type of information transfer seems to be affected in different neurological disorders, such as Alzheimer’s disease or schizophrenia (Uhlhaas et al. 2008, Stam et al. 2009), as well as in type 1 diabetes (van Duinkerken et al. 2009) and in obesity (Olde Dubbelink et al. 2008). We hypothesized that alterations in insulin signaling may be one cause of dysfunction in oscillatory networks in obese subjects.
Tschritter et al. (2006) used a hyperinsulinemic euglycemic clamp to assess cerebrocortical insulin effects in resting state and modulation by body weight. For lean subjects, we observed an increase in power in theta and beta band for an increase in plasma insulin concentration. For obese subjects, on the contrary, no effect of insulin on beta activity was observed and even a decrease in theta activity was evident. Furthermore, the insulin-induced changes in theta and beta activity were closely correlated with BMI and percent body fat. Regarding peripheral insulin sensitivity, these changes were positively correlated with insulin sensitivity of glucose disposal. In summary, this means that cerebrocortical insulin action was positively correlated with peripheral insulin sensitivity and negatively with measures of obesity.
Results of this study indicate that an increase in insulin levels in the brain has an effect on resting state brain networks and that this effect is altered in obese subjects. Therefore, we suggest that obese subjects are not only characterized by peripheral, but also by central insulin resistance. This insulin resistance is most likely associated with overeating and the development of obesity and is of special interest when investigating insulin effects on networks involved in eating behavior control.
4 Modulation of Neural Networks Related to Control of Eating Behavior by Insulin and Obesity
Stingl et al. (2010a) explored the effects of intranasally administered insulin on the small world dynamics of resting state magnetoencephalographic brain activity. Insulin induced subject-specific changes of the weighted path length in the theta band were observed. This change again showed a statistically significant positive correlation with the body mass index of individual subjects supporting the hypothesis of cerebral insulin resistance in obese individuals.
Weighted path length is a measure of global interconnectedness of a network and its global efficiency. This confirms that insulin is a strong modulator of global communication of the brain networks involved in satiation and the control of eating behavior. However, this approach is not suitable to elucidate which networks or how the communication between these networks is modulated by insulin.
In general, these networks have a highly complex pattern of correlated activity of multiple brain areas with interconnectivities and existing feedback loops. In this respect, a method with high temporal resolution is advantageous for evaluation of function or malfunction of individual components of these networks. Based on the low sensitivity of MEG for activity in homeostatic control areas, MEG research has to focus on cognitive elements of eating behavior including brain areas involved in sensory processing and categorization and higher-order brain areas involved in executive functions.
4.1 Visual Processing and Categorization
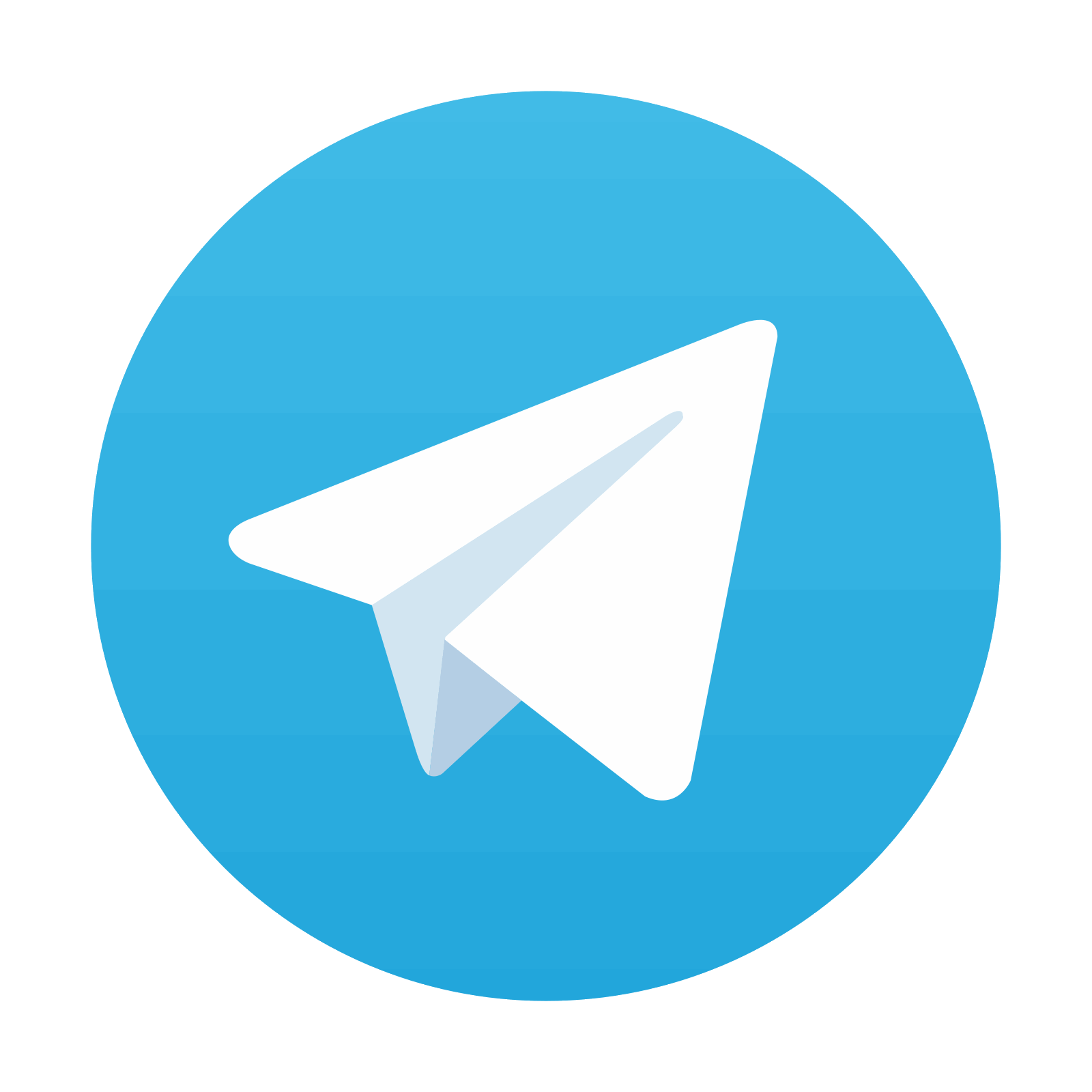
Stay updated, free articles. Join our Telegram channel

Full access? Get Clinical Tree
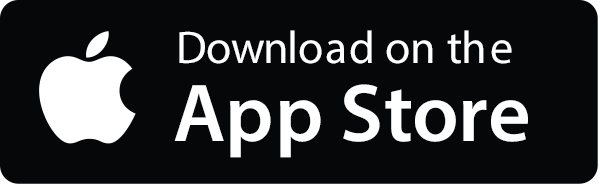
