TP = test positive in diseased subjects
FP = test positive in nondiseased subjects
FN = test negative in diseased subjects
TN = test negative in nondiseased subjects
T+ = abnormal test results
T- = normal test results
D+ = diseased subjects
D- = nondiseased subjects
STATISTICS
Incidence = number of diseased people per 100,000 population annually
Prevalence = number of existing cases per 100,000 population at a target date
Frequency = number of times an event occurred; often graphically represented in histograms
Mortality = number of deaths per 100,000 population annually
Fatality = number of deaths per number of diseased
Sensitivity
= ability to detect disease
= probability of having an abnormal test given disease
= number of correct positive tests / number with disease
= true positive ratio = TP / (TP + FN) = TP / D+
• D+ column in decision matrix
◊ Independent of prevalence
Specificity
= ability to identify absence of disease
= probability of having a negative test given no disease
= number of correct negative tests / number without disease
= true negative ratio = TN / (TN + FP) = TN / D-
• D- column in decision matrix
◊ Independent of prevalence
Accuracy
= number of correct results in all tests
= number of correct tests / total number of tests
= (TP + TN) / (TP + TN + FP + FN) = (TP + TN) / total
◊ Depends much on the proportion of diseased + nondiseased subjects in studied population
◊ Not valuable for comparison of tests
Example: same test accuracy of 90% for two tests A and B
Positive Predictive Value
= positive test accuracy
= likelihood that a positive test result identifies disease
= number of correct positive tests / number of positive tests
= TP / (TP + FP) = TP / T+
• T+ row in decision matrix
◊ Dependent on prevalence
◊ PPV ↑ with ↑ prevalence for given sensitivity + specificity
◊ PPV ↑ with ↑ specificity for given prevalence
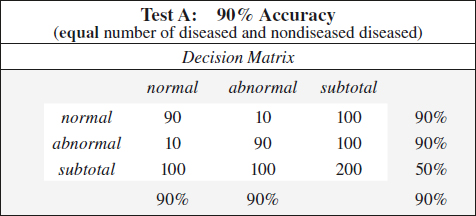
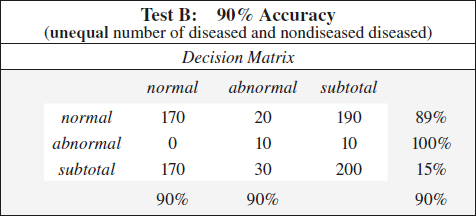
Negative Predictive Value
= negative test accuracy
= likelihood that a negative test result identifies absence of disease
= number of correct negative tests / number of negative tests
= TN / (TN + FN) = TN / T-
• T- row in decision matrix
◊ Dependent on prevalence
◊ NPV ↑ with ↑ prevalence for given sensitivity + specificity
◊ NPV ↑ with ↑ sensitivity for given prevalence
False-positive Ratio
= proportion of nondiseased patients with abnormal test result
• D- column in decision matrix
= FP / (FP + TN) = FP / D-
= 1 – specificity = (TN + FP – TN) / (TN + FP)
False-negative Ratio
= proportion of diseased patients with a normal test result
• D+ column in decision matrix
= FN / (TP + FN) = FN / D+
= 1 – sensitivity = (TP + FN – TP) / (TP + FN)
Disease Prevalence
= proportion of diseased subjects to total population
= (TP + FN) / (TP + TN + FP + FN) = D+ / total
◊ Sensitivity + specificity are independent of prevalence!
◊ Affects predictive values + accuracy of a test result
Example:
Test A, C, D: 90% sensitivity + 90% specificity
Bayes Theorem
= the predictive accuracy of any test outcome that is less than a perfect diagnostic test is influenced by
(a) pretest likelihood of disease
(b) criteria used to define a test result
Receiver Operating Characteristics (ROC)
= degree of discrimination between diseased + nondiseased patients using varying diagnostic criteria instead of a single value for the TP + TN fraction
= curvilinear graph generated by plotting TP ratio as a function of FP ratio for a number of different diagnostic criteria (ranging from definitely normal to definitely abnormal)
Y-axis: true-positive ratio = sensitivity
X-axis: false-positive ratio = 1 – specificity; reversing the values on the X-axis results in an identical “sensitivity-specificity curve”
Use: variations in diagnostic criteria → reported as a continuum of responses → ranging from definitely abnormal to equivocal to definitely normal ← based on subjectivity + bias of individual radiologists
◊ A minimum of 4–5 data points of diagnostic criteria are needed!
Difficulty: subjective evaluation of image features; subjective diagnostic interpretation; data must be ordinal (= discrete rating scale from definitely negative to definitely positive)
Interpretation:
◊ ↑ in sensitivity leads to ↓ in specificity!
◊ ↑ in specificity leads to ↓ in sensitivity!
◊ Most sensitive point is the point with the highest TP ratio
→ equivalent to “overreading” by using less stringent diagnostic criteria (all findings read as abnormal)
◊ Most specific point is the point with the lowest FP ratio
→ equivalent to “underreading” by using more strict diagnostic criteria (all findings read as normal)
◊ Does not consider disease prevalence in the population
◊ The ROC curve closest to the Y-axis represents the best diagnostic test
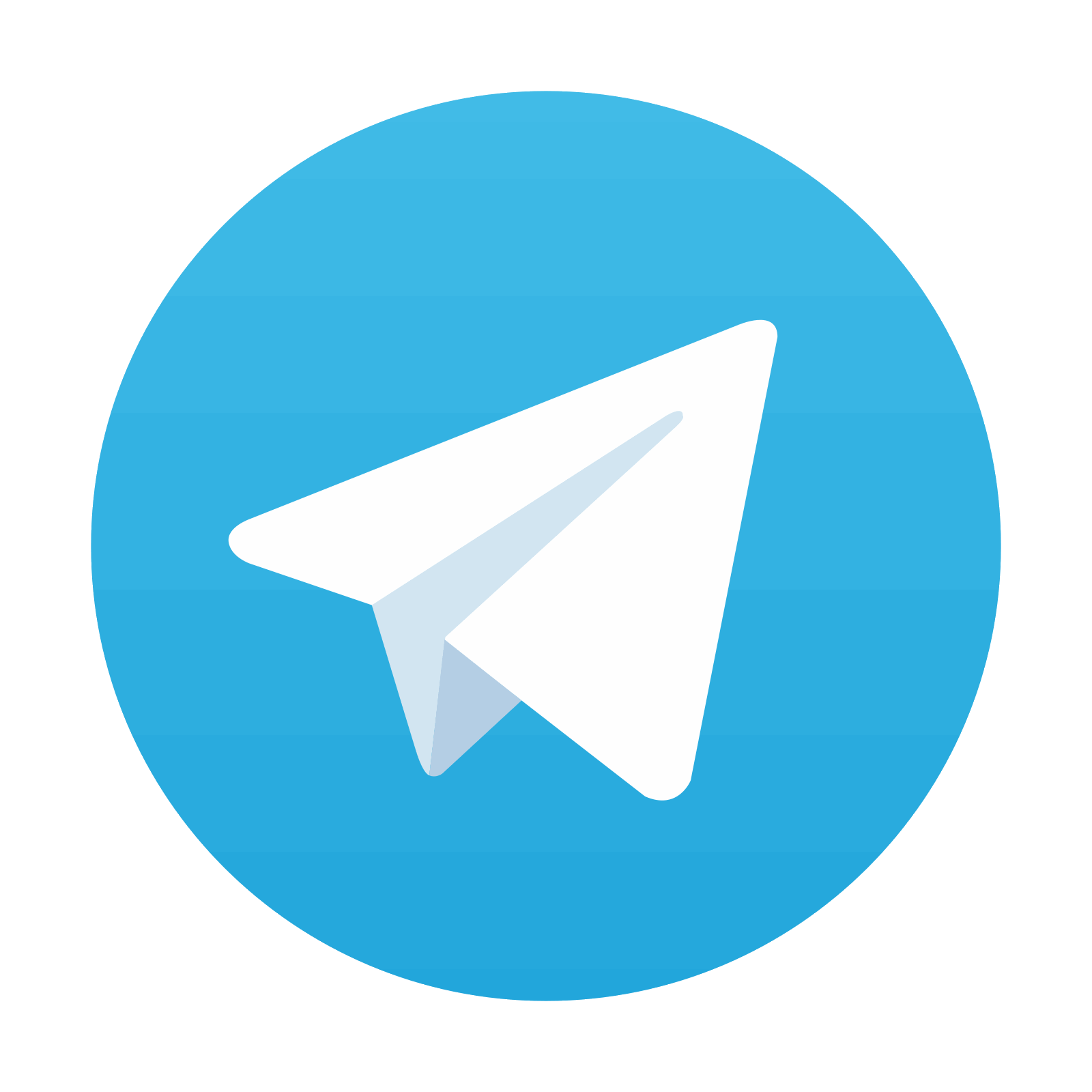
Stay updated, free articles. Join our Telegram channel

Full access? Get Clinical Tree
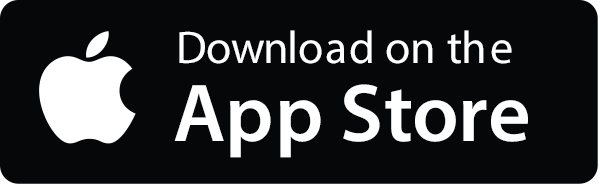
