At the outset, perhaps an illustration is in order. Without scrutinizing, take a quick look at Figure 12.1 and discern how the two arrays of letters differ. Then come back to the text of this chapter.
Figure 12.1 Take a brief look at each of these letter arrays and think about how they differ.
If you followed the instructions, even the briefest of glances will have revealed that these two quite similar images are clearly different. The most obvious difference, visible in a glance, is that the array on the left is somewhat less regular than the one on the right. You might also have gotten a notion that the distribution of letters is different. In all probability, you will not have noticed that the right-hand image has cancer written vertically. If cancer only appeared in regular grids, you would learn to be more suspicious of those grids after some practice. If cancer tended to appear on the left side of such grids, you would learn to direct your attention preferentially to that side.
This is an unnatural example of a completely natural aspect of scene perception. You are walking through a new city. You turn a corner and in a fraction of a second you know that you have stumbled on an outdoor market. Your expertise in such matters tells you whether this is the sort of market you want to explore. That first glimpse probably does not reveal to you the location of the booth that will feed you your lunch, though it may be in plain sight. That will require a bit more scrutiny. That scrutiny will be guided by your understanding of the structure of this scene and of other scenes of this sort. Your attention will be guided to booths serving food as opposed, for example, to trash bins (Biederman et al., 1973; Henderson and Ferreira, 2004; Vo and Wolfe, 2015).
If you are a radiologist, reading this chapter, you will have had the same experience when viewing medical images. On first glance when viewing a radiograph, you will know what sort of image this is. You might know that this is an image that is likely to contain a finding. If there is a finding present, you will not have found it yet, but you may have a hunch that the image is likely to be worth some effort. Your understanding of human anatomy and of the reason for the exam will guide your deployment of attention within that image.
12.1 The Gist of the Image
In all of these cases, the first glimpse has revealed the gist of the image. Gist is a useful but imprecisely defined term. (For a table describing the variety of usages in the visual cognition literature, see Koehler and Eckstein (2017).) As we have seen, finding “cancer” in an array of letters requires the deployment of some form of focused attention to a region of space. The act of recognizing any object in a scene filled with other objects requires directing attention to that object. There are exceptions to this rule. For instance, if there was a single, red letter in Figure 12.1, you might be able to report on its presence without deploying attention to that red object. However, if you needed to specify the identity of the red letter, attention would need to be deployed (Maljkovic and Nakayama, 1994). Using attention to select the object permits its features to be “bound” so that the observer can appreciate the relationship between those features (Roskies, 1999; Treisman, 1996, 1998; von der Malsburg, 1981) (e.g., his shirt is blue and his hair is red, rather than the other way around). When the observer first sees a scene, its features are unbound (Wolfe and Bennett, 1997; Wolfe and Cave, 1999) and its objects are not explicitly recognizable (Evans and Treisman, 2005).
Nevertheless, it is not as if attention really was a “spotlight” (Posner, 1980) where inside the beam we see and outside we do not (Crick and Koch, 1990; O’Regan, 1992). As Crick (1984) says, “In this metaphor, the searchlight is not supposed to light up part of a completely dark landscape but, like a searchlight at dusk, it intensifies part of a scene that is already visible to some extent.” When you first glanced at the letter arrays of Figure 12.1, the entire figure was visible “to some extent.” Moving beyond the realm of spotlight metaphors, we need to ask what it means, in this case, to be “visible to some extent.”
An observer can extract a rich impression of a scene in a time far too short to permit selective attention to more than a handful of objects (Biederman, 1972; Oliva, 2005; Potter and Faulconer, 1975). Some of this information is “statistical.” In a single glimpse (< 200 ms, with a backwards pattern mask that immediately follows presentation), observers can estimate statistics of groups or “ensembles” of objects (e.g., we can estimate the average and variance of properties like color, motion, size, orientation) (Alvarez, 2011; Ariely, 2001; Brady et al., 2017; Chong and Triesman, 2003). They can categorize natural scenes (e.g., this is a beach, an urban close-up) (Greene and Oliva, 2008, 2009) and identify the presence of types of object like animals (Drewes et al., 2011; Li et al., 2002; Thorpe et al., 2001). Note, however, that when observers extract this sort of semantic information from a scene after a brief exposure, they are doing so imperfectly and without necessarily knowing where the animal is or what animal it might be (Evans and Treisman, 2005). Indeed, the ability to extract image statistics may be closely related to the ability to infer the presence of an animal or a beach. These higher-level decisions may be based on raw image statistics (Oliva, 2005; Oliva and Torralba, 2006) or access to unbound disjunctive intermediate features (Evans and Treisman, 2005; Freedman et al., 2002; Sigala and Logothetis, 2002).
In a real-world scene, those raw image statistics also provide information about the layout of the scene (Greene and Oliva, 2008; Ross and Oliva, 2010; Sanocki and Epstein, 1997). The gist of the scene will include information about whether you are looking at an open or closed space, for example. A brief glimpse of the scene will guide the subsequent deployment of attention, in part because the observer will know where the surfaces in the image lie (Castelhano and Henderson, 2007). Thus, in a search for humans in scenes, observers will preferentially fixate on or near the ground plane because, if there are people, that is where they are likely to be (Ehinger et al., 2009). Importantly, some of this gist processing must be learned. While the system may come equipped with an ability to assess the average orientation of lines in a scene or even to gain an impression of the layout of the surfaces, it can hardly come with built-in definitions of the gist of a bookstore or a gym.
To summarize, as Fei-Fei et al. (2007) say, “within a single glance, much object- and scene-level information is perceived by human subjects.” That initial glimpse produces a representation that includes basic statistical information that can, in turn, give rise to information about the structure and content of the scene. In many cases, the mapping of basic statistical information to specific meanings will be learned as we are exposed to thousands of exemplars of different types of scenes throughout our lives. Moreover, in all but the briefest of exposures, there will be time for one or more deployments of selective attention to specific locations/objects in the field. This will permit a small number of specific objects to be identified, adding them to the gist (Wolfe et al., 2011).
Radiologists are experts with the object class of medical images. As such, their visual systems will become tuned so that it produces this gist representation to this different set of stimuli. Even though they are not “natural,” medical images can be thought of as scenes. The mechanisms that allow us to recognize the gist of the gym will also allow a radiologist to extract some meaning from the first glimpse of an X-ray or magnetic resonance imaging image. As we will see, medical image perception research suggests that experts can use this initial gist to guide attention toward targets and to classify whole images, presumably on the basis of their global image statistics.
12.2 Guiding Attention: Kundel, Nodine, and their Followers
In a classic, early paper in medical image perception, Kundel and La Follette (1972) measured the eye movements of a wide range of observers as they searched normal and abnormal chest X-rays. The core finding was that the eye movements of experts were different than those of novices and trainees. Experts needed fewer fixations to find the targets and their pattern of fixations was different (Figure 12.2). This basic pattern has been replicated many times (Bertram et al., 2013; Krupinski, 1996; Nodine et al., 1996)
Figure 12.2 Eye movements change as radiologists become more expert. Notably, radiologists appear to learn where not to look.
If an expert is going to make fewer eye movements than a novice, that must be because that expert has developed a better idea about what parts of the image need to be fixated in order to find relevant information. Kundel and Nodine have stressed the importance of what they call “holistic recognition” (Kundel, 2007) during the first second or so after an image is presented to the radiologist. Just as the gist of a real-world scene guides the eyes in routine search tasks, the learned structure of the medical image comes to guide the eyes and attention in a search through a medical image. To quote Kundel (2007),
Clearly much of what happens in perception precedes exhaustive visual scanning of the image. Recordings of the location of the initial few fixations of a group of nine mammographers and mammography trainees showed that on about half of the images the mammographers jumped right to the cancer whereas most of the trainees only jumped to the cancer in 20% of the images.
If observers were simply searching through a medical image in the manner that one reads a page of text (or some other orderly manner), then the percentage of targets found should be a linear function of the amount of the image that had been examined and, thus, a linear function of the amount of time spent on the image. On average, by the time you searched 50% of the image, you should have found 50% of the detectable targets. The data make it clear that this is not the case. Figure 12.3 shows the nonlinear rise in performance as a function of time. The right part of Figure 12.3 presents the number of cases completed as a function of time. The dashed line shows the rate over the first 20 seconds and it can be seen that the rate falls off as time goes on. Experts find more targets in the early portion of the search.
Figure 12.3 Curves showing that the rate of target discovery slows over time. The left figure shows this effect within the first second. The right figure shows the effect over many seconds.
Kundel and Nodine (1975) decided to look at the information available in the earliest moments of search by asking observers to assess ten normal and ten abnormal lung X-rays with only a 200-ms exposure to the images. Their experts could classify70% correctly after that limited glimpse of the image. With unlimited time, they were almost perfect, but clearly quite a lot of information was available well within the first second. Carmody et al. (1981) systematically varied the exposure duration and mapped out the rise in this initial information over the course of the first half-second of exposure. Most of the success was obtained within 250 ms (Figure 12.3 left). Not all the information was available in the initial glimpse. Notice that, for the least visible stimuli, performance asymptotes at just 40% after 500 ms. Performance would rise if the observers were free to scrutinize the images, but even with difficult cases, there is substantial information in the first few hundred milliseconds.
The Carmody et al. (1981) data come from search for lung nodules. The Nodine et al. (2002) figure shows mammography data. In both cases, Kundel and Nodine (2004) propose a similar account of the search process. To use the version in Kundel and Nodine (2004), the first step is a global analysis of the image over the course of “a few hundred milliseconds” – 700 ms or so in Kundel et al. (2008). Next, the results of that global step are subject to “foveal verification.” Swensson (1980) formalized this basic idea into his “two-stage detection model,” in which a “final decision stage must logically follow some earlier stage of visual search, during which that particular feature of the pattern was selected for attention and specific consideration.” Swensson noted the similarity between his idea and Neisser’s (1967) distinction between “preattentive” and “attentive” processes. In fact, Swensson’s work is a precursor of subsequent work on the guidance of attention by preattentive and/or nonselective aspects of processing (Drew et al., 2013). We will briefly review that concept here.
12.3 Guidance
To recognize an object or an image feature, it is generally necessary to attend to that location. Often that involves foveating the location, but it is possible to covertly attend away from the point of fixation (Grindley and Townsend, 1968). Indeed, attention to object or locations away from the point of fixation must be happening all the time. If you are searching for one type of letter in a field of many letters, you can only foveate three or four letters per second. However, if the letters are big enough to escape from acuity and crowding limitations, you can process 20–40 letters per second (Wolfe, 2003).
Under normal viewing conditions, attention probably visits several locations in a “useful (or functional) field of view” (UFOV) around a fixation (Sanders and Houtmans, 1985). This UFOV is an important concept if, for example, one is concerned with looking at an entire image. Since the point of fixation is just that – a point – it is important to have a measure of how much of the scene around that point is being effectively processed (for a radiologic example, see Ebner et al., 2016).
How should the eyes and the metaphorical spotlight of attention be deployed? If one is looking for lung nodules, for example, it would be wasteful to simply deploy attention at random or even in an orderly, lawnmower-like sweep, back and forth across the image. It would make more sense to deploy attention to small, white, round elements in the image. Our ability to do this is illustrated with a nonmedical example in Figure 12.4. Your goal is to find the letter, T. First, look for a T in the left panel. Next, search the right panel for a T that you are told is on a gray, vertical rectangle. It is likely that the second search will be more efficient than the first because, on the left, you will have had to resort to an unguided sampling of items until you stumble on the target. In the second search, on the right, your search will be guided to gray, vertical, rectangular objects. You are not going to waste attention on white, round, or tilted items.
Figure 12.4 Guided search (Wolfe, 1994). Look for a T on the left. On the right, look for a T on a vertical, gray rectangle.
Figure 12.4 is just a demonstration. Your experience of which search is easier might not correspond to the description just offered. In the unguided search on the left, there is no reason that you might not have gotten lucky and selected the T with your first or second deployment of attention. This would make the time required for the unguided search as fast as that for any guided search. However, if you were tested on hundreds of trials of this sort, the data would show that the guided search was more efficient (Wolfe et al., 2010).
Not every visual property of the target item can guide attention. There is a limited set of perhaps one to two dozen guiding attributes (Wolfe and Horowitz, 2017). These “preattentive features” (Treisman, 1985) are available in that initial period of what Kundel and Nodine (2004) called “global analysis.” Treisman, in her influential feature integration theory (Treisman and Gelade, 1980), describes the preattentive stage of processing as happening in parallel across the whole field. Her second stage was serial scrutiny of items, akin to the “foveal verification stage” of Kundel and Nodine (2004). Egeth et al. (1984), followed by Wolfe and colleagues, brought in the idea that processing in the preattentive stage could guide the subsequent, serial stage (Wolfe, 1994, 2007; Wolfe et al., 1989); and Wolfe stressed the idea that attention could be guided by multiple features at the same time (e.g., vertical, gray, and rectangle) (Nordfang and Wolfe, 2014).
It is convenient but misleading to think about these stages in strictly sequential terms as if preattentive processing (global) ends at the moment that attentive (foveal verification) begins. If a radiologist is looking for lung nodules, the preattentive information about small, white, and round is available at the outset to guide the first deployments of attention, but it will remain present to continue guiding subsequent deployments for as long as the radiologist cares to keep searching.
Kundel and Nodine (2004) describe another form of guidance. They envision it as a separate stage in their model. After the “few seconds” that are occupied by global processing and foveal verification, they propose a “discovery search” phase. This is also guided search, but it is guidance to “places with a high probability of finding the object specified by the task.” As a trivial example, if you are looking for lung nodules, you need not look outside the lung. An expert would guide her attention not only to the lung, but to the parts of this lung most likely to contain nodules. As with feature guidance, the radiologist is making expert use of a form of guidance we use in more mundane tasks continuously. If you are searching for a toaster in a friend’s unfamiliar kitchen, you will direct your attention to “places with a high probability of finding” a toaster: the kitchen counter, not the stovetop, the ceiling, or the floor. This is known as “scene guidance” (Biederman et al., 1982; Henderson and Ferreira, 2004; Wolfe and Horowitz, 2017).
Kundel and Nodine (2004) make this a later stage in processing but, as noted above, scene layout information is available very early in processing and can be shown to guide the earliest fixations (Castelhano and Henderson, 2007). The Kundel and Nodine model, and indeed, most early models of search, capture the critical aspects of guided search, provided we do not insist on a linear series of nonoverlapping steps. In the first moments of perception, information becomes available that allows attention and the eyes to be deployed in a nonrandom way. Attention will go to places where targets are likely to be and to objects or locations that show the basic features of the target.
Kundel and Nodine (2004) propose one final stage: a “reflective stage” during which the radiologist makes “difficult decisions about ambiguous features.” This can take tens of seconds per image and is an aspect of search that has not been much studied in the laboratory. Most basic visual attention research has used targets that are either trivial to identify once attention is deployed to the right spot (is that a T or an L?). Sometimes more ambiguous stimuli are used (e.g., targets of low, near-threshold contrast), but little in the basic research field has tried to capture the sort of complexity that would face a neuroradiologist trying to determine if anything in the head computed tomography could account for the patient’s headache.
12.4 Global Gist Signals
As discussed at the outset, when you take a quick look at the letter arrays of Figure 12.1, you immediately know something about the gist of each scene. For instance, you know that one is regular while the other is not. As you scrutinize the images, you can identify specific letters but you see and continue to see something everywhere. You do not just see the contents of the spotlight of attention. One way of modeling this is to propose that there are two pathways to visual awareness (Drew et al., 2013; Wolfe et al., 2011). One is a selective pathway that allows observers to attend to and identify objects. The other is nonselective, providing some visual experience across the entire visual field (e.g., “spatial envelope” of an image (Oliva and Torralba, 2001) and the summary statistics, mentioned above). The nonselective pathway makes global gist information available to the observer.
If that observer is a radiologist, what can be done with that information? We get a hint from anecdotal reports in which clinicians say that sometimes, when they first encounter an image, it just “looks bad,” even though the observer does not know why.
This suggests that there might be a gist of abnormality in, at least, some classes of images. This is not an outlandish thought. To return to an earlier example, as you walk through the door, you might have the immediate impression that, not only is this a bookstore, but it is an unusual bookstore. It is also possible that anecdotes about these initial hunches are merely selective memory, after the fact. One could imagine that there may be a bias to remember instances when these hunches turn out to be true, while forgetting times when they are not (Tversky and Kahneman, 1983).
In order to determine if there is a perceptual basis for these reports, Evans et al. (2013) showed radiologists normal and abnormal bilateral mammograms for exposures of 250–2000 ms. These brief presentations were followed by a white outline mask of the image. Observers were asked to localize that abnormality on the outline and then to rate their decision confidence about the image on a 0–100 analogue scale running from abnormal to normal. Fifty-five radiologists were tested on a set of 100 images. Half contained a subtle mass or architectural distortion. No calcifications were used because the ability to detect a bright white dot in a brief exposure was not particularly interesting in this case.
Rating scale data can be used to derive receiver operating characteristic (ROC) curves, as shown in Figure 12.5. Chance performance would fall on the hit = false alarm diagonal. Radiologists showed above-chance performance for detecting these subtle abnormalities at all stimulus durations (d ’ = ~1.4). In fact, the duration had little effect in this study. A 250-ms glimpse is just about as good as 2 seconds during which the observer could make seven to eight fixations.
Figure 12.5 Average receiver operating characteristic data for judging the abnormality of a bilateral mammogram, presented for each of five stimulus durations. The ability to discriminate normal from abnormal cases is similar at all durations and is reliably above chance.
Importantly, radiologists were, however, unable to localize the abnormalities in these images. Regardless of their degree of confidence that the patient should be recalled for further examination, localization performance was at chance. On the other hand, these results are not evidence that screening mammography can be done in a quarter of a second. The “expert” square in the upper left of Figure 12.5 shows the approximate performance of radiologists performing screening exams in the normal manner. Obviously, d ’ is much higher (~2.9).
The ability to extract information from a global / nonselective glimpse of a medical image is not limited to mammograms. In a similar experiment, cytologists were shown Papanicolaou smear images from cervical cancer screening for brief periods. These are essentially unstructured collections of thousands of cells on a microscope slide. Experts could sort stimuli into normal and abnormal at above-chance levels (~ d ’ = 1.2), though again, they were unable to localize the pathology. Some global aspect of the image statistics or the texture of the stimulus is telling experts that the stimulus is or is not abnormal.
What is the nature of the signal? If the signal is well classified, it can be exploited. For example, it could be used to train either radiologists or computer algorithms. Evans et al. (2016) repeated their experiment with low- and high-pass filtered stimuli. As shown in Figure 12.6, radiologists are sensitive to a global gist signal that seems to be present in the fine detail of the fibrous structures of the breast tissue seen in the high-pass image. Performance with a high-pass filtered image is just about as good as performance with the full unaltered image. In contrast, the “blobs” of the low-pass image are far less detectable at the 500-ms exposure duration. This is interesting since the standard hypothesis would be that the coarser, low-frequency information would dominate in brief exposures (Navon, 1977; Oliva and Schyns, 1997), but this was clearly not the case. Localization remained poor across all conditions. Moreover, density – a known risk factor for cancer (Zheng et al., 2012) – was not correlated with performance.
Figure 12.6 Sample images and data showing the results of filtering the mammograms. The global gist signal is more detectable in the higher spatial frequencies than in the lower. Performance with the high-pass filtered images is comparable to the unfiltered condition. Dashed lines represent empirical receiver operating characteristic curves for each observer, derived from the rating scale data. The thicker, solid line is the average result.
Another candidate source of the signal is a distortion of the normal symmetry between the two breasts. Humans are good at detecting symmetry (Wagemans, 1997) and asymmetry can be a strong indicator for developing breast cancer (Scutt et al., 2006; Zheng et al., 2012). However, this does not appear to be the basis for the performance of radiologists when they are detecting the gist of breast cancer. As shown in Figure 12.7, radiologists do perfectly well at the gist detection task when they are presented with just a single breast image. Indeed, performance (d ’ = 1.2) is not much different than what is obtained with a pair of images. Radiologists may use symmetry between two breasts as an important sign in normal mammography, but it is not the signal that allows for classification of mammograms after a half-second of exposure (although in clinical practice where viewing times are longer it is, especially with architectural distortion, an often-used signal).
Figure 12.7 Top, the signal is present in a single breast. It is also detectable in the contralateral breast (bottom): the breast with no lesion. Dashed lines represent empirical receiver operating characteristic curves for each observer, derived from the rating scale data. The thicker, solid line is the average result.
The lower panel of Figure 12.7 reveals what might seem like a counterintuitive finding from the same experiment. Radiologists were able to discriminate normal from abnormal even when images were taken from the contralateral breast of a woman with breast cancer. The signal appears to be weaker (d ’ = 0.6) but it is clearly reliable, as can be seen by noting that the ROC curves for all of the individual observers (dashed lines) lie above the chance diagonal. Since no lesion is present in the image, it is clear that performance cannot be based on a lucky fixation on a mass. In a related experiment, small but statistically significant signals were found when the stimuli were square sections of parenchyma that did not contain an abnormality, regardless of whether the sections came from the ipsilateral or contralateral breast (Evans et al., 2016).
The presence of a signal in the contralateral breast tells us that the global gist signal probably does not arise from the effects of overt cancer in the breast tissue. Rather, these findings suggest that something else about being a patient with or at risk for cancer is correlated with the appearance of the parenchyma.
Converging evidence for this view comes from computer-based texture analysis. For example, Gierach et al. (2014) found that a Bayesian artificial neural network algorithm can be trained to discriminate tissue from women who have a BRCA1/2 mutation status that puts them at higher risk of cancer. As with the global gist measure, this measure is not related to the measurement of density (Li et al., 2014). Presumably, the genetic make-up of the patient has an influence, not only on the probability of developing a cancer but on the structure, and thus the appearance, of the tissue. Those genetic effects will not be restricted to the breast that has the cancer. They can be manifest in the contralateral breast as well (Wang et al., 2017).
If the global gist signal in mammography is not tied to the visible presence of cancer and if it could be related to the patient’s underlying predisposition to develop cancer, it could be an indication of risk before any cancer is visible.
Figure 12.8 shows evidence that the global gist might, indeed, function as a novel biomarker for cancer risk, detectable in women prior to the time that they develop visible signs of the disease (Schill et al., 2017). The figure shows data from nine British radiologists. They viewed 58 bilateral mammograms that had been acquired 3 years prior to the mammograms that had revealed visible and actionable cancer. Thus, in this version of the experiment the “abnormal” cases were “normal” mammograms from patients who would later develop breast cancer. These cases were randomly mixed with 58 normal mammograms (no disease for 3 years after the images were acquired). As in the previous experiments, each bilateral mammogram was presented briefly (500 ms) and masked with the outline of the same mammogram. Participants were asked to rate the likelihood of abnormality of the images on the 0–100 scale. Rating-scale data were converted to ROC curves and d ’ was calculated. The ability to distinguish normal from abnormal (cancer priors) was small (d ’ = 0.2) but statistically significant (p < 0.001). Again, it can be seen that the ROC curves of the individual observers lie above (albeit, not far above) the chance line. Neither results from a few salient cases nor breast density can account for this ability to classify images at above chance in patients who would not develop visible signs of cancer until 3 years. These findings raise the possibility that a global gist signal could be a clinically useful tool. If detected by an expert or extracted by an algorithm, it might serve as an early-warning sign, identifying women who should be followed more closely than the others in their cohort. Indeed, this signal may be related to the texture measures that are being investigated by computer scientists using convolutional neural network methods (Kallenberg et al., 2016; Nielsen et al., 2014).
Figure 12.8 A small global gist signal can be found in images acquired 3 years before the diagnosis of cancer. Dashed lines represent empirical receiver operating characteristic curves for each observer, derived from the rating scale data. The thicker, solid line is the average result.
12.5 Gist in General
This chapter has focused on a global gist signal in mammography (with a brief mention of a similar signal in chest radiographs and cervical cancer screening). This simply reflects the current state of research in this area. There is no reason to think that there are no other gist signals present in other sets of medical images. After all, gist processing is a routine part of normal visual perception and it is likely that it is a routine part of normal medical image perception. In any domain where you develop visual expertise, you probably develop some learned gist expertise for the stimuli in that domain. Any regular at a sports bar will be able to glance at the TV and will know, at the very least, what sport is being played. Any radiologist will know where in the body an image comes from. With more expertise, more information will come out of the initial glimpse at a stimulus. You may have an instant hunch as to whether this restaurant will suit your needs. That hunch may not be perfect but you will probably beat chance. Radiologists will have similar hunches about stimuli in their domain of expertise. It should not surprise us that we may be unable to verbalize the reason for the hunch. Most perceptual processing goes on “under the hood” without our conscious access to the processes that got us to the answer. You infer a three-dimensional world from two-dimensional images on your retinas with only the dimmest idea of how this is accomplished. You hear speech as a succession of discrete words, even though the sound stream lacks dividing moments of silence. This is what Helmholtz (1924) dubbed “unconscious inference.” The semantic aspects of gist processing are, perhaps, best thought of as another of these occasions where the mind makes meaning out of the current stimulus based on its extensive prior experience.
It is worth noting that there is no guarantee that the meaning we make is the right meaning. The world of bias and stereotype, for example, also makes use of a set of snap judgments that can have a decidedly gist-like feel to them. It may well be that some rapid decisions about medical images are just as flawed as a snap judgment about a job applicant, based on race or gender. Scientific research can help us to determine if a hunch about a medical image is a valuable inference or just a leap to a premature conclusion. If an inference is meaningful, it can be exploited in the service of better patient care. That might mean making clinicians aware of the circumstances in which a hunch may be based on useful underlying information. Alternatively, it could suggest signals that can be exploited by computer algorithms to guide more effective computer-aided detection systems.
References










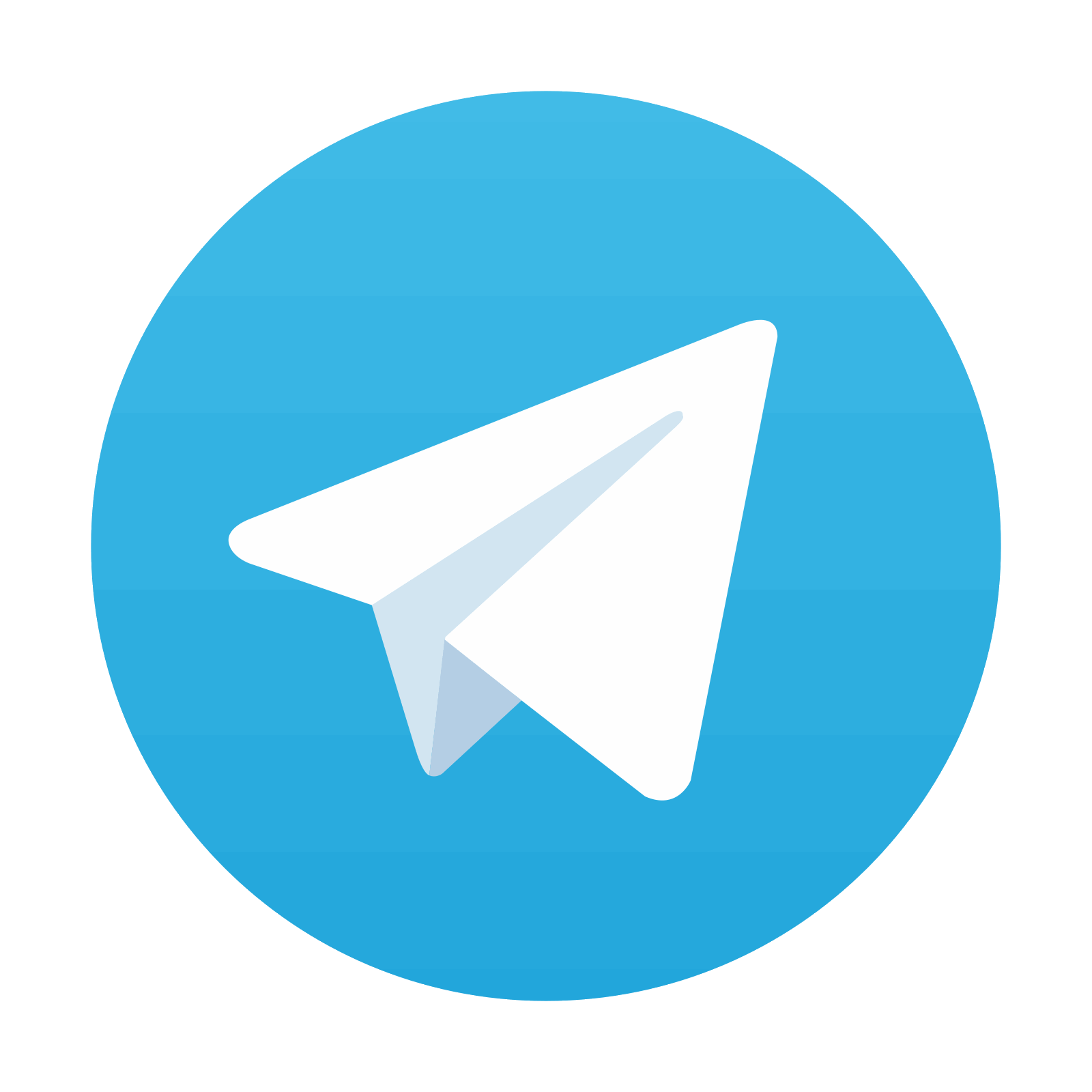
Stay updated, free articles. Join our Telegram channel

Full access? Get Clinical Tree
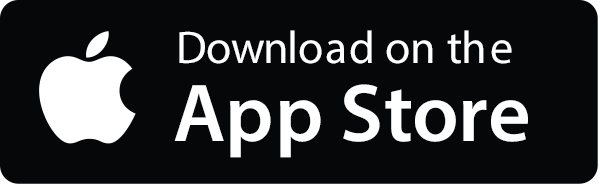

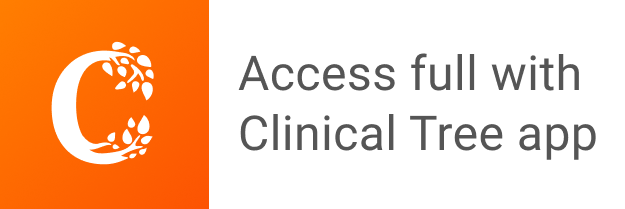