23.1 Overview
Early diagnosis of breast cancer results in a 97% survival rate. However, to achieve this survival rate and, even more importantly, to achieve zero deaths from breast cancer in the near future, we must significantly reduce the 30–40% of breast cancers that fail to be diagnosed. In 2011–2012 the National Breast Cancer Foundation, the Royal Australian and New Zealand College of Radiologists, and the Australian Government provided funding to allow the University of Sydney to implement and establish BREAST (Breast Screen Reader Assessment Strategy), a research infrastructure focused toward transforming breast cancer diagnosis.
The BREAST platform has helped to better our understanding of types of missed cancers, impact of novel imaging technologies, and radiologist characteristics and practices that promote accurate diagnoses. To date, the infrastructure has supported nearly 30 research projects involving clinics across all Australian states and territories; 20 PhD students, international research partnerships across four continents with universities such as Harvard Medical School, Fudan University, National Cancer Centre in Vietnam, and National University of Singapore; and 50 publications and presentations.
With 800,000 mammography studies performed in Australia annually and 1 million new breast cancer cases being reported each year globally, the impact of radiologic misdiagnoses on public health is a hugely important issue. In 2011, a study showed that 44% of lesions in test cases were missed by 116 Australian and New Zealand breast imaging readers (Reed et al., 2010). In addition, the median level of sensitivity was below 70% (Rawashdeh et al., 2013b). It was data such as these that led to the development of BREAST. It is not that Australian readers perform better or worse than anywhere else, although recent evidence shows the former (Soh et al., 2016). We had now established a system of research that highlighted varying levels of performance between individuals and regions.
Since its inception, up to 80% of Australia’s breast imaging radiologists and trainees have engaged with BREAST resulting in a million data entries. Through BREAST workshops and the online platform, up to 720 individuals worldwide have engaged in single experiments – much more than the typical sample size in other studies anywhere in the world. BREAST represents an effective method of engaging enough radiologists to help identify causal agents for human error, and a platform for testing potential solutions. At the time of writing, BREAST has been adopted in Australia, New Zealand, Singapore, Vietnam, and Mongolia.
23.1.1 How Does it Work?
BREAST is a web-based system created by the University of Sydney. The system allows breast screen radiologists (and others) to diagnose sets of mammographic images online in a geographically limitless way with each image interaction being instantly and centrally recorded (Figure 23.1). Specifically, radiologists are asked to look at images in exactly the same way as they would clinically. So each case is displayed using craniocaudal and mediolateral views of each breast and drop-down menus and postprocessing options are presented which typically reflect those found in clinical practice. Each radiologist (independently) then judges each image and marks on each projection wherever he or she sees a lesion and gives each perceived lesion a score. The scoring system used so far reflects the Australian National Breast Cancer Centre Synoptic Breast Imaging Report classification system, which has been endorsed by the Royal Australian and New Zealand College of Radiologists. The current scoring system is on a scale of 2–5, where 2 = benign findings, 3 = indeterminate/equivocal findings, 4 = suspicious findings of malignancy, and 5 = malignant findings. It should be stressed however that any scoring system such as the American College of Radiology’s Breast Imaging Reporting and Data System (BI-RADS) categories can be implemented. If a score of 1 is given (1 = no significant abnormality), or the next icon is chosen, the next case is displayed. Observers can go back to any image or case and correct a previous decision.
Figure 23.1 An individual studying the Breast Screen Reader Assessment Strategy (BREAST) test set.
Since truth is known for each case and image, instant feedback can be given to each radiologist on performance and any diagnostic errors. Once the case sets are completed, all readings are instantly analyzed using behind-the-scene algorithms. Scientists and clinicians are immediately presented with performance values, including receiver operating characteristic (ROC) and jackknife alternate free-response ROC (JAFROC) figures of merit, sensitivity, location sensitivity, specificity, true positives, true negatives, false positives, and false negatives. In addition a reader-specific image file is instantly generated (Figure 23.2) so that correct and incorrect decisions can be examined in detail on each image. All data produced are stored on a central database which promotes subsequent analyses by scientists studying a variety of imaging-based topics.
Figure 23.2 (A) Example of a feedback image presented after the radiologist has judged the image. “Truth” represents the real location of the image, whereas “My Selection” represents where the radiologist thought there was a lesion. In this case, on the left MLO projection, both cancers were correctly located, whereas on the right CC projection the observer failed to locate the cancer. (B) In this example, on both projections, the two cancers have been missed, but the observer has a false-positive interaction in a different part of the image.
In terms of analyzing new innovations and technologies, BREAST provides a solution to a problem encountered by imaging researchers for many decades. Once a breast image is created using novel techniques or methods, bringing together world-leading experts to examine the images is extremely difficult. Local reviews often involve nonexperts in a nonoptimized laboratory setting, which often results in equivocal data with wide standard deviations and inconclusive findings. To address this limitation BREAST has developed an innovative online research portal for mammography and other radiology images which can be extended to pathology. For the first time, high numbers of expert readers can log in and review the cases in their own specialized clinical environment (wherever in the world they are located), resulting in instantly available data to the researchers.
23.1.2 Clinical Impact
Missed breast cancer rates remain stubbornly high at around 30%, even with major technological advances. In all, 30–70% of breast cancers diagnosed at follow-up mammography are retrospectively visible on earlier mammograms, which were originally interpreted as cancer-free. In a review (Australian Government, 2009) of 40–49-year-olds, almost 50% of cancers were missed at screening mammography, meaning that up to half of reported cancers in women enrolled in a screening program present as interval (symptomatic) cases, often resulting in more aggressive treatments.
BREAST is currently attempting to reduce this error rate by translating research outputs into solutions for clinical environments. As the tool was designed by clinicians and each BREAST research project is developed for clinical relevance, the strategy should be of benefit to women. Adherence to this fundamental principle of BREAST is being monitored by our Breast Access and Management Committee, which meets every 3 months and consists of the country’s leading clinicians, including Professor Mary Rickard (pioneer of breast screening in Australia and elsewhere), Dr. Marli Gregory (Clinical Leader of BreastScreen Aotearoa) and Dr. Nalini Bhola (State Radiologist, BreastScreen NSW), as well as consumers and other senior academic and clinical personnel.
Some examples of research useful to the clinic include: a study of Australian radiologists that showed that digital breast tomosynthesis (DBT) improved sensitivity and specificity by 12 and 16% respectively (Alakhras et al., 2015); a second study showed that current understanding of the impact of mammographic density on cancer detection may not be relevant with digital images (Al Mousa et al., 2014b); a third study identified important radiologists’ characteristics/practices needed to optimize diagnosis, which underpin current National Accreditation Standards (Rawashdeh et al., 2013b); and a fourth study demonstrated that radiology accuracy depends on the country of reading (Soh et al., 2016). Some of these outputs will be described in more detail below.
23.2 How Do We Engage Readers?
As mentioned above, due to BREAST’s widespread adoption by radiologists, we have over 1200 readings to date. Any radiology researcher will know that it is a challenge to find radiologists with spare time to read large numbers of images for perception experiments. So how was this achieved for BREAST?
Much of the credit goes to the user-friendly interface of the software involved. Clinicians like to have an instant score reflecting their abilities and the initial software purchased from Ziltron and all subsequent versions of the software designed at the University of Sydney do just that. Ziltron displays immediate performance values the instant a reader completes a test set (Figure 23.3). Also an image-by-image review of the observer’s interaction with the cases is immediately available. These highlight any errors made, thus allowing effective reflection on the reader’s part. It should be stressed that, whilst radiologists can compare their performance for any test set with other radiologists who have completed the same set, this is done in an anonymized way so that radiologists can only identify themselves. Neither of the two directors of BREAST (Lee and Brennan) can associate scores with specific individuals.
Figure 23.3 Screenshot of the type of information instantly available to the reader once he or she has completed the test set. ROC, receiver operating charactereistic.
We source readers using two main methods. The first is through our online platform where individuals anywhere in the world can register with the BREAST team. Once a login is arranged, readers can access and complete a test set. Approximately 44% of readings have been done this way. Secondly we bring the test set with primary workstations to radiology and breast cancer conferences and set up a schedule where attendees at the conference can complete a test set. This is highly popular and usually the BREAST team are booked out from early in the morning to late in the evening with four parallel sessions running at the same time. Roughly 56% of readings are performed at these meetings.
Participation is also promoted by the awarding of continuing medical education points for completing a test set. This is provided by the Royal College of Australian and New Zealand Radiologists and in one state in Australia and in New Zealand completing one test set per year is a mandatory part of professional registration.
One of the most exciting elements of BREAST is its implementation in other countries. In particular, large proportions of the radiologists in Singapore, Vietnam, and Mongolia have read at least one BREAST test set. This not only provides insights into areas of improvement in training or educational regimen, it also facilitates a better understanding of observer behaviors and diagnostic efficacy when judging images from women of various ethnic origins where size, density, and overall morphology of the breast can vary substantially.
23.3 Some of the Research Outputs
23.3.1 Radiologist Characteristics
Throughout this textbook the reader will become increasingly aware of the importance of image perception on diagnostic efficiency. In particular it has been argued that perception factors may account for up to 60% of all radiologic errors (Brem et al., 2003). There are a range of perceptual parameters that are responsible, such as inadequate image searching, failed lesion recognition, and faulty decision making (Kundel et al., 1978). A key agent that impacts upon all these parameters is the radiologist and the experiences that he or she possesses.
Researchers have looked at this and have come up with conflicting findings. It has been argued that fellowship training is an important factor (Elmore et al., 2009); however, others have suggested that experience measured in terms of years since registration, years reading mammograms, and hours of mammography reading performed each week is more important (Miglioretti et al., 2007; Reed et al., 2010). Interestingly, with regard to volume of reading, a number of countries have stated minimal values for number of cases that should be read over specified periods. This ranges from 960 per 2 years to 5000 per year while in Australia the stated value is 2000 per year (Kan et al., 2000). However even the literature here is unclear, with evidence (Barlow et al., 2004; Committee, 1997; Miglioretti et al., 2007; National Accreditation Committee, 1994; US Department of Health and Human Services, 1997) showing no association between performance and reads per year, in contrast to the strong relationship shown more recently by Reed et al. (2010). The need to define precise and accurate national accreditation standards to maintain best practice requires clearer direction.
To address this BREAST researchers attempted to establish the key characteristics (if any) that determined best diagnostic performance. Since data on a large number of readers were available (n = 116) who had read the same test set, it was possible to break down readers into various experiential categories by numbers of readings per year – less than 1000, 1000–5000, and greater than 5000.
The study (Rawashdeh et al., 2013b) then looked at a number of radiologist characteristics and demonstrated that, when all radiologists were grouped together, positive relationships were evident between performance and number of years of mammogram reading, number of years qualified as a radiologist, and annual number of readings (Table 23.1). However specific results that were different from this overall pattern were noted for individual categories of radiologists. In particular, for the radiologists with the lowest annual readings (<1000/year), performance was negatively correlated with number of years qualified as a radiologist and number of years reading mammograms. This inverse relationship between performance and experiential factors was not shown for the other two groups of radiologists and instead, for those who read more than 5000 cases per year, highest performance was positively associated with the number of years qualified as a radiologist, hours reading per week, and number of years reading mammograms. The latest finding was also relevant to the category of radiologists who were reading 1000–5000 cases per year.
Table 23.1 The association of the performance of radiologists with different characteristics and experiences. Emboldened values suggest a significant relationship, as shown using Spearman statistics (Rawashdeh et al., 2013b)
JAFROC | Sensitivity | Specificity | |||||
---|---|---|---|---|---|---|---|
r | p-value (≤) | r | p-value (≤) | r | p-value (≤) | ||
Age | Less than 1000 | –0.47 | 0.06 | –0.36 | 0.15 | –0.18 | 0.47 |
1000–5000 | 0.01 | 0.92 | –0.11 | 0.39 | 0.07 | 0.56 | |
More than 5000 | 0.11 | 0.56 | –0.19 | 0.25 | 0.02 | 0.22 | |
Years of qualification | Less than 1000 | –0.65 | 0.004 | –0.22 | 0.18 | –0.41 | 0.09 |
1000–5000 | 0.24 | 0.059 | 0.08 | 0.51 | 0.2 | 0.12 | |
More than 5000 | 0.40 | 0.01 | –0.06 | 0.72 | 0.38 | 0.01 | |
Years of mammogram reading | Less than 1000 | –0.69 | 0.002 | –0.33 | 0.18 | –0.38 | 0.12 |
1000–5000 | 0.01 | 0.88 | 0.01 | 0.99 | 0.11 | 0.37 | |
More than 5000 | 0.47 | 0.002 | 0.01 | 0.94 | 0.33 | 0.03 | |
Total number of mammograms per year | Less than 1000 | 0.06 | 0.79 | 0.04 | 0.85 | –0.18 | 0.49 |
1000–5000 | 0.29 | 0.03 | 0.21 | 0.09 | 0.04 | 0.73 | |
More than 5000 | 0.06 | 0.68 | 0.07 | 0.63 | 0.35 | 0.03 | |
Hours per week reading mammogram | Less than 1000 | –0.3 | 0.24 | 0.07 | 0.78 | –0.33 | 0.18 |
1000–5000 | –0.10 | 0.4 | 0.11 | 0.37 | –0.10 | 0.43 | |
More than 5000 | 0.46 | 0.003 | 0.09 | 0.56 | 0.46 | 0.003 |
JAFROC, jackknife free-response receiver operating characteristic.
Emboldened values indicate statistically significant findings.
This work shows the value of having infrastructures that yield high numbers of readings such as BREAST. In the past, where readings involved only a limited number of radiologists, researchers did not have the freedom to allocate readers to specific groupings. Previous studies that failed to demonstrate relationships between numbers of readings and performance could therefore be due to an aggregation of results where findings for one group of radiologists, which were directly opposed to findings for another radiologist grouping, were being concealed. Another interesting discovery of this BREAST work was the finding that radiologists who read less than 1000 cases per year performed worse the longer they had been reading mammograms and the longer they had been qualified as a radiologist. Clearly the stated position: “I have been reading mammograms for 30 years and therefore must be good” is a questionable one.
23.3.2 What are the Breast Cancer Appearances That Radiologists are More Likely to Miss?
It is accepted that detecting breast cancer within mammography is a difficult task for either humans or machines. The varying nature of cancer appearances in lesion brightness, size, and shape, and the fact that these are superimposed on a heterogeneous anatomic background present a significant radiologic challenge and relatively high missed cancer rates (Vyborny et al., 2000a). With the drive for earlier detection of breast cancers and the importance of reducing missed cancers in mammography, we need to better understand the image characteristics of difficult-to-detect cancers so that educational strategies or machine learning algorithms can be optimized.
With regard to brightness and size of lesions, much work has been done highlighting that missed cancers mainly occur within breasts that are more dense, with lesions that present with developing or low density (Bassett, 1997; Bird et al., 1992; Goergen et al., 1997; Majid et al., 2003) or when cancers are smaller in size (Bassett, 1997; Goergen et al., 1997; Huynh et al., 1998). This highlights the importance of sufficient attenuation if the contrast of the lesion is to be sufficiently high to facilitate detection. However there is a paucity of other information around overall lesion shape (Pohlman et al., 1996; Rangayyan et al., 2000; Shi et al., 2008), which is surprising as radiologists rely on shape characteristics such as spiculations to identify malignant lesions and give an opinion on the aggressiveness of a cancer (D’Orsi and Kopans, 1993; Jain, 2000; Jiang et al., 1998; Shen et al., 1994; Sickles, 1989; Strickland and Hahn, 1996).
To address this deficiency, one BREAST work (Rawashdeh et al., 2013a) aimed to establish if a range of shape features of a lesion impacted upon the level of detection. In this study 129 readers looked at 20 cases which consisted of single and multicentric masses in 16 and 4 cases respectively, resulting in a total of 24 cancers. These cancer cases were dispersed within a larger test set of 60 cases. Each cancer was then given a difficulty rating, which was calculated by dividing the number of readers that spotted that cancer by the total number of readers (n = 129). So, for example, if one cancer was identified by 43 radiologists, the difficulty rating would be 0.34 (43/129). The difficulty ratings were then correlated against shape descriptors such as area, perimeter, lesion elongation, and lesion nonspiculation. Other features such as lesion texture, brightness, and breast characteristics were also studied in this study, and details for those can be found in the full paper.
The results showed that detection of cancers was significantly positively associated with area, perimeter, lesion elongation, and lesion nonspiculation. Whilst the relationship with size is not a surprise, the new information regarding elongation and spiculation offered new insights into the types of cancers that were most likely to be missed. This means that the more rounded or more spiculated the cancer is, the less likely radiologists are to decide that it is definitely a cancer. Perhaps this is because these cancers are more difficult to visualize, or there could be other factors at play. What the work clearly showed is that radiologists are less likely to report these appearances as cancerous lesions.
This work also showed that geometric parameters such as shape and size were better able to predict if a cancer would be identified, compared with lesion brightness and texture, even though quantifiable parameters such as signal-to-noise and contrast-to-noise ratios are often used to judge image quality. This once more supports the idea that psychophysical measures do not always serve as a good surrogate to diagnostic efficacy.
In conclusion this work suggested that certain shape features will determine how well a cancer is identified regardless of its contrast or brightness. This finding should have important implications for radiology training regimen and automated detection systems. Further work is now required, particularly using eye-tracking technologies, to determine if this decreased diagnostic efficacy is more related to the visibility of the lesion or the radiologists’ decision processes.
23.3.3 Effect of Radiologists’ Experience on Breast Cancer Detection and Localization Using Digital Breast Tomosynthesis
DBT is proving to be a promising technology for diagnosing breast cancer. At the time of writing, many studies have shown that using this technology enhances both sensitivity and specificity, particularly when used as an adjunct to conventional mammography (Figure 23.4). Whilst large-scale studies in Europe (Ciatto et al., 2013; Gilbert et al., 2015; Lang et al., 2016) and the USA confirmed these benefits, certain features around its efficacy were unclear, particularly around the level of reader experience that was required to accrue maximum benefit from this new technology. This deficiency in the literature was once again the result of inadequate numbers of readers of varying experience being available to participate in reading experiments.
Figure 23.4 The digital breast tomosynthesis image on the right shows more easily the cancer (arrow) compared with the traditional mammographic image (left).
In 2013, BREAST facilitated an observer-based assessment of DBT (Alakhras et al., 2015) which involved 50 cases, including 27 cancers and 26 radiologists (Figure 23.5). Based on previous DBT experiences the readers were allocated to three groups: those with no experience; those with only workshop experience; and those with clinical experience. Readers were asked to judge the cases, firstly with mammography alone and then with mammography and DBT, and score their confidences on a 1–5 scale. The results showed that all radiologists regardless of their experience showed an improvement in diagnostic performance once DBT was available. The percentage improvements in JAFROC figure-of-merit scores for the no, workshop, and clinical experiences were 15.3%, 22.1%, and 20.9% respectively. These values are summarized in Table 23.2.
Figure 23.5 Radiologists in Darwin, Australia, undertaking the digital breast tomosynthesis observer study.
Table 23.2 Various scores for the different radiologist groupings for digital mammography(DM) and DM + digital breast tomosynthesis (DBT) (Alakhras et al., 2015)
ROC AUC | Sensitivity | Specificity | Location sensitivity | JAFROC FOM | ||
---|---|---|---|---|---|---|
No DBT experience | DM | 0.682 (0.051) | 0.630 (0.167) | 0.652 (0.174) | 0.484 (0.141) | 0.603 (0.120) |
DM + DBT | 0.775 (0.071) | 0.704 (0.204) | 0.826 (0.131) | 0.547 (0.140) | 0.695 (0.042) | |
p-value | 0.004 | 0.235 | 0.008 | 0.031 | 0.016 | |
Workshop experience | DM | 0.680 (0.071) | 0.630 (0.111) | 0.652 (0.153) | 0.453 (0.117) | 0.621 (0.094) |
DM + DBT | 0.790 (0.063) | 0.704 (0.074) | 0.783 (0.066) | 0.594 (0.062) | 0.758 (0.063) | |
p-value | 0.004 | 0.013 | 0.013 | 0.009 | 0.004 | |
Clinical experience | DM | 0.681 (0.095) | 0.649 (0.139) | 0.718 (0.120) | 0.469 (0.078) | 0.632 (0.071) |
DM + DBT | 0.789 (0.107) | 0.704 (0.102) | 0.718 (0.337) | 0.563 (0.156) | 0.764 (0.190) | |
p-value | 0.042 | 0.073 | 0.888 | 0.016 | 0.031 |
JAFROC FOM,jackknife free-response receiver operator characteristic figure of merit; ROC AUC, area under the receiver operator characteristic curve.
Emboldened values indicate statistically significant findings.
These data demonstrate the benefit of DBT to all radiology clinicians working in breast imaging, despite the level of previous DBT experience. It is also valuable to note that the benefits are noted for a variety of metrics, including both specificity and lesion sensitivity.
23.3.4 The Impact of Architectural Distortion on Breast Cancer Detection with Digitally Acquired Images
Presentations of cancers as architectural distortions are a well-reported challenge with mammographic imaging. They are described as the most difficult appearance to identify and interpret and are the most commonly missed abnormality (D’Orsi et al., 1998; Homer, 1997; Knutzen and Gisvold, 1993). These types of lesion appearances are characterized by distortion to the normal tissue with the cancer itself often not being visible. They often present with lines radiating out from a central point and bear a strong similarity to normal anatomic features such as Cooper’s ligaments (D’Orsi et al., 1998; Knutzen and Gisvold, 1993; Rangayyan et al., 2010). Whilst architectural distortions represent only about 6% of all abnormal lesions (Yankaskas et al., 2001), it is estimated that they are responsible for up to half of all missed cancers with mammographic screening (Bird et al., 1992; Burrell et al., 1996; Digabel-Chabay et al., 2004; Huynh et al., 1998). In addition 60% of biopsied lesions appearing as architectural distortion are malignant, 80% of which are invasive tumors (Baker et al., 2003; Broeders et al., 2003). To date computer-aided diagnosis systems have offered few solutions (Bargallo et al., 2013; Doi et al., 1999; Giger, 2000; Giger et al., 2001; Sampat et al., 2005; Vyborny et al., 2000b), and the need to promote human detection of these types of lesions is clear.
Almost all the evidence to date around the difficulties of detecting cancers appearing as architectural distortion is based on film screen mammography (Burrell et al., 1996, 2001; Yankaskas et al., 2001). Limited data are available on whether the situation has improved with digital acquisitions and it is possible that postprocessing tools such as windowing and zooming may have ameliorated some of the previously recorded difficulties. The aim of the following study was to establish if architectural distortion still presented a challenge to radiologists following the introduction of digital mammography.
The work (Suleiman et al., 2016a) involved 41 experienced radiologists, 21 of whom were from Australia whilst the remaining 20 were from the USA. Each observer looked at 30 digitally acquired mammography cases, of which ten were normal, ten displayed cancers with the appearance of architectural distortion, whilst the remaining ten had cancer but with an appearance other than architectural distortion. The results showed that the ability of the radiologists to detect the architectural distortion cases was significantly less than that of the other cancer cases, suggesting that this type of cancer appearance remained a significant challenge despite the technological switch to digital acquisition (Table 23.3). This finding was relevant for both groups of readers. The resemblance of this lesion appearance to normal tissue remained and it was clear that the availability of facilities to alter contrast and magnification made little difference. Therefore, the solution to the problems associated with architectural distortion most likely lies in implementing efficient educational strategies. Educational programs that incorporate systems such as BREAST could present comprehensive and interactive images of this lesion type and provide instant feedback, thus enabling learning.
Table 23.3 Location sensitivity of radiologists with a group of images containing cancers with architectural distortion compared with images with cancers of a nonarchitectural distortion appearance (Suleiman et al., 2016a)
Reader type | Architectural distortion | Nonarchitectural distortion |
---|---|---|
Australian readers | 0.65 (0.13) | 0.82 (0.08) |
US readers | 0.61 (0.13) | 0.83 (0.09) |
All readers grouped together | 0.63 (0.13) | 0.83 (0.09) |
23.3.5 The Impact of Mammographic Density on Diagnostic Efficacy When Images are Digitally Acquired
The female breast is made up mainly of fibroglandular and adipose tissue, and due to the differing X-ray attenuation of these tissues the former appears relatively bright on an image as opposed to the latter, which appears darker on a mammographic image. If a breast is made up predominantly of fibroglandular tissue and hence appears mammographically bright, the breast is said to be dense. In recent years the pathologic implications of high-density breast have received much attention, particularly since those women from Westernized populations who have high density are understood to have an up to six times increased risk of presenting with breast cancer, compared with women with low-density breasts (Boyd et al., 1995, 1998).
This also has a radiologic implication (Figure 23.6). Since fibroglandular tissues and cancer both appear bright on a mammogram, dense regions have the potential to reduce the visualization of cancers. This effect has been well reported in the literature for film screen-based technologies with workers showing associations between higher density and higher rates of missed (Bird et al., 1992; Chiarelli et al., 2006) and interval cancers (Ciatto et al., 2004; Mandelson et al., 2000), supported by data highlighting that sensitivity can drop from above 80% to below 30% for women with mammographically dense breasts (Buist et al., 2004; Carney et al., 2003; Cawson et al., 2009; Kolb et al., 2002; Mandelson et al., 2000; Rosenberg et al., 1998). Previous work was predominantly performed in the analogue era, when little was known about the impact of digital technology on this inverse density/diagnostic efficacy relationship. We used BREAST to investigate whether our understanding of the obscuring nature of increasing density on breast lesion detection persisted when images were digitally acquired.
Figure 23.6 Two mammographic images. The one on the left represents a relatively low-density breast, whilst the one on the right appears more dense.
This work (Al Mousa et al., 2014b) involved 14 radiologists who were classified into two groups depending on whether they read more or less than 2000 mammographic cases per year. Each observer looked at 150 images consisting of low- and high-density cases and 75 of these images contained cancer. The results showed that, when cancer was superimposed on dense tissue, the whole group of radiologists and those radiologists who read more than 2000 cases per year were better able to detect the cancer for the higher- compared with the lower-density images (Table 23.4). This not only challenges what was previously known about the radiologic impact of increased density, it also seems to defy logic. Why would increased bright regions in a breast image enhance the detection of cancers that are of a similar brightness compared to images where a bright cancer positioned against a low-density background offers more contrast?
Table 23.4 Impact of mammographic density on diagnostic efficacy when the cancer was overlaying the dense regions of the breast1
Radiologist group | Assessed parameter | Low-density images | High-density images |
---|---|---|---|
All radiologists | Location sensitivity | 50.0 (20.84) | 59.1 (38.64) |
Specificity | 77.0 (25.68) | 76.3 (38.16) | |
JAFROC FOM | 0.63 (0.1) | 0.68 (0.13) | |
Higher-reading radiologists | Location sensitivity | 55.6 (12.5) | 81.8 (20.64) |
Specificity | 71.62 (25.68) | 80.27 (35.83) | |
JAFROC FOM | 0.69 (0.08) | 0.77 (0.19) | |
Higher-reading radiologists | Location sensitivity | 41.7 (25.01) | 47.7 (27.27) |
Specificity | 77.03 (39.19) | 76.32 (34.87) | |
JAFROC FOM | 0.61 (0.13) | 0.65 (0.14) |
1 Median values across the observers are given for each assessed parameter and the interquartile ranges are shown in parentheses. Jackknife free-response receiver operating characteristics (JAFROC FOMs) are shown (Al Mousa ete al., 2014b).
Further BREAST work helped to answer this question (Al Mousa et al., 2014b). Using eye-tracking technologies, seven expert radiologists assessed 149 mammography images and various behavioral qualities were recorded, including dwell time, number of fixations, and time to first fixation. This part of the work showed that more dwell time was evident as well as higher numbers of fixations when cancers were located within dense, compared with less dense, regions of the breast. In addition time to fixation on a cancer was longer when the cancer was not overlaying a dense region. This points to greater and more immediate visual attention in dense regions of the image. Whilst this behavior may have been evident with film screen technology it offered little advantage in the absence of postprocessing tools such as windowing and contrast enhancement which of course are available with digital acquisition.
The potential explanation was: because highly dense breasts have a higher risk of breast cancer, expert radiologists who saw such breasts therefore gave the images more attention. This extra attention, coupled with the availability of digital image manipulation, allowed the observers to identify cancers more readily in higher- rather than low-density image regions. If this explanation is valid, we should now see a reduction in interval and later-presenting cancers within women having high mammographic density. This work is ongoing.
23.3.6 Can Test Set Data Reasonably Describe Actual Clinical Reporting in Screening Mammography?
Thousands of radiology observer studies have been performed to test new technologies, new techniques, or simply to better understand reader behavior with medical images. To satisfy reviewers’ expectations when trying to publish research in peer-reviewed journals, experiments are designed to minimize the effects of any confounders that might arise and therefore conditions are strictly controlled. Ambient light is maintained at optimum conditions; primary workstations are calibrated throughout the experiment to make sure they adhere to standards such as the Gray Scale Display Function; rooms have very low background noise and the possibility of unwelcome intrusions are removed; help is instantly at hand if any issues arise with image displays; the task given to the radiologist is often oversimplified so that analyzable data are provided; prevalence of disease cases are often enhanced compared with real clinical situations to promote robust statistical treatments; and prior images are often not available. All these conditions are to some extent quite removed from clinical reality and are typical of the conditions when readers study test set cases such as that contained within the BREAST program. The question therefore remains: do radiologists’ performances under these almost artificialized conditions in any way reflect the way the same radiologists would perform in a real clinical situation?
To establish if performance measured when reading BREAST in any way reflected performance when the same radiologists interpreted images in clinical centers, we designed a study which involved ten expert radiologists (Soh et al., 2013, 2014). Each reader was asked to interpret 200 individual-specific cases under three conditions (outlined below), but the important thing here was that all radiologists had interpreted each of their specific cases over the previous 5 years in their own clinical center. In other words, they were asked to interpret in a controlled test set environment images that they had already interpreted in a clinical setting as part of their routine practice. The 200 cases consisted of 10 true positives, 20 false positives, 160 true negatives, and 10 false negatives and these categories were defined by how each reader diagnosed each case in the clinic.
In the test set environment, the radiologists were allocated in groups of five to two of the following three conditions; each reading was separated by a period of 4 months:
A. reading the test set in the radiologist’s normal clinical reporting environment, whilst making available prior images;
B. reading the test set in a typical laboratory setting, commonly used for BREAST work that had the same ambient lighting and workstations as the radiologist would find in the clinical environment, with prior images being provided;
C. reading the test set in a laboratory (as in the previous condition) but without prior images.
Performance in the test set environment was assessed using a region-of-interest figure of merit (RoI FOM), side-based sensitivity and specificity and for each radiologist the level of agreement of these results with how they originally interpreted the results in the clinical environment were compared using a Wilcoxon matched-pairs signed rank test. In addition the level of agreement between the confidence scores provided in the clinical setting and in each of the above three conditions was assessed using a Kendall’s coefficient of concordance. The results showed no significant differences between the clinical and test set readings for sensitivity and specificity and 11 (out of 15) differences for the RoI FOMs (Table 23.5). All readers’ confidence scores for all conditions showed significant levels of agreement with the clinical situation.
Table 23.5 Region of interest figure of merit (RoI FOM), sensitivity, and specificity values for the clinical and experimental reads are shown for conditions A, B, and C. In the last two columns the levels of agreement between the confidence scores for the clinical and test set reads are shown using the Kendal W value and p-values, the latter demonstrating consistent agreement (Soh et al., 2013)
Comparison and reader no. | ROI FOMs | Sensitivity (%)1 | Specificity (%)1 | Kendall W2 | |||||
---|---|---|---|---|---|---|---|---|---|
Actual clinical reporting | Test set condition | p-value | Actual clinical reporting | Test set condition | Actual clinical reporting | Test set condition | W value | p-value | |
A | |||||||||
1 | 0.77 (0.65, 0.90) | 0.84 (0.72, 0.96) | … | 58.82 | 70.59 | 89.07 | 87.98 | 0.63 | <0.01 |
2 | 0.73 (0.61, 0.84) | 0.76 (0.64, 0.88) | … | 50.00 | 55.00 | 88.89 | 92.22 | 0.72 | <0.001 |
3 | 0.74 (0.62, 0.86) | 0.74 (0.62, 0.86) | … | 52.63 | 52.63 | 88.76 | 88.76 | 0.73 | <0.001 |
4 | 0.79 (0.66, 0.92) | 0.95 (0.88, 1.02) | <0.05 | 62.50 | 93.75 | 89.13 | 84.24 | 0.72 | <0.001 |
5 | 0.74 (0.62, 0.86) | 0.94 (0.86, 1.01) | <0.01 | 52.63 | 89.47 | 88.76 | 93.85 | 0.71 | <0.001 |
Median | 0.74 | 0.84 | 52.63 | 70.59 | 88.89 | 88.76 | 0.72 | ||
B | |||||||||
1 | 0.77 (0.64, 0.89) | 0.72 (0.59, 0.85) | … | 58.82 | 52.94 | 89.07 | 91.26 | 0.64 | <0.01 |
2 | 0.74 (0.62, 0.86) | 0.86 (0.75, 0.96) | … | 50.00 | 73.68 | 88.89 | 91.11 | 0.65 | <0.01 |
3 | 0.74 (0.62, 0.86) | 0.85 (0.74, 0.95) | … | 52.63 | 73.68 | 88.76 | 89.44 | 0.76 | <0.001 |
4 | 0.79 (0.66, 0.92) | 0.91 (0.82, 1.01) | <0.05 | 62.50 | 87.50 | 89.13 | 84.78 | 0.78 | <0.001 |
5 | 0.74 (0.62, 0.86) | 0.88 (0.78, 0.98) | … | 52.63 | 78.95 | 88.76 | 88.76 | 0.73 | <0.001 |
Median | 0.74 | 0.86 | 52.63 | 73.68 | 88.89 | 89.44 | 0.73 | ||
C | |||||||||
1 | 0.80 (0.66, 0.93) | 0.73 (0.60, 0.87) | … | 62.50 | 50.00 | 89.13 | 89.67 | 0.68 | <0.01 |
2 | 0.72 (0.61, 0.84) | 0.85 (0.75, 0.95) | … | 50.00 | 80.00 | 88.89 | 68.33 | 0.64 | <0.01 |
3 | 0.73 (0.61, 0.84) | 0.78 (0.66, 0.90) | … | 50.00 | 60.00 | 88.83 | 89.44 | 0.72 | <0.001 |
4 | 0.77 (0.64, 0.90) | 0.86 (0.75, 0.97) | … | 58.82 | 76.47 | 89.07 | 87.43 | 0.70 | <0.001 |
5 | 0.73 (0.61, 0.85) | 0.88 (0.78, 0.98) | <0.05 | 50.00 | 80.00 | 88.89 | 85.00 | 0.69 | <0.001 |
Median | 0.73 | 0.85 | 50.00 | 76.47 | 88.89 | 87.43 | 0.69 |
Note – The middle descriptive value is presented as the median. Data in parentheses are 95% confidence intervals.
1 No significant differences.
2 Based on confidence score.
The overall message appears to be that clinical and test set performance are in reasonable agreement; however, it should be noted in particular that the sensitivity in the test set environment can be higher than the test set (thus impacting on the RoI FOM scores). This suggests that in the test set environment readers are more likely to spot the cancer compared to the clinic. The possible explanations for this may be around increased prevalence of the disease within the test set, readers being unrestricted by clinical audit expectations to reduce the number of women recalled in a test set environment, and radiologists simply getting better at their job since the test set readings were performed up to 5 years after the clinical reads.
This study was also followed up by a further test whereby performance metrics collected by BREAST were compared with the actual clinical audit values generated by 20 radiologists (Soh et al., 2015). For example, audit metrics such as rates of detecting cancers, recall rates, and percentage cancer read that were not recalled were compared with the BREAST metrics. Again overall good correlation was shown using Spearman’s rho, where significant agreement was shown for BREAST’s FOM and sensitivity scores with the audit data, although it was noted that the test set’s specificity data showed poorer correlation. This specificity result is most likely due again to the reduced need to restrict numbers of “recalled women” in a test set environment.
Nonetheless the overall results from both these studies are encouraging and, whilst some caution needs to be applied, the level of agreement shown between test set performance and clinically based data supports the work done using reading strategies such as BREAST and PERFORMS (Scott and Gale, 2006).
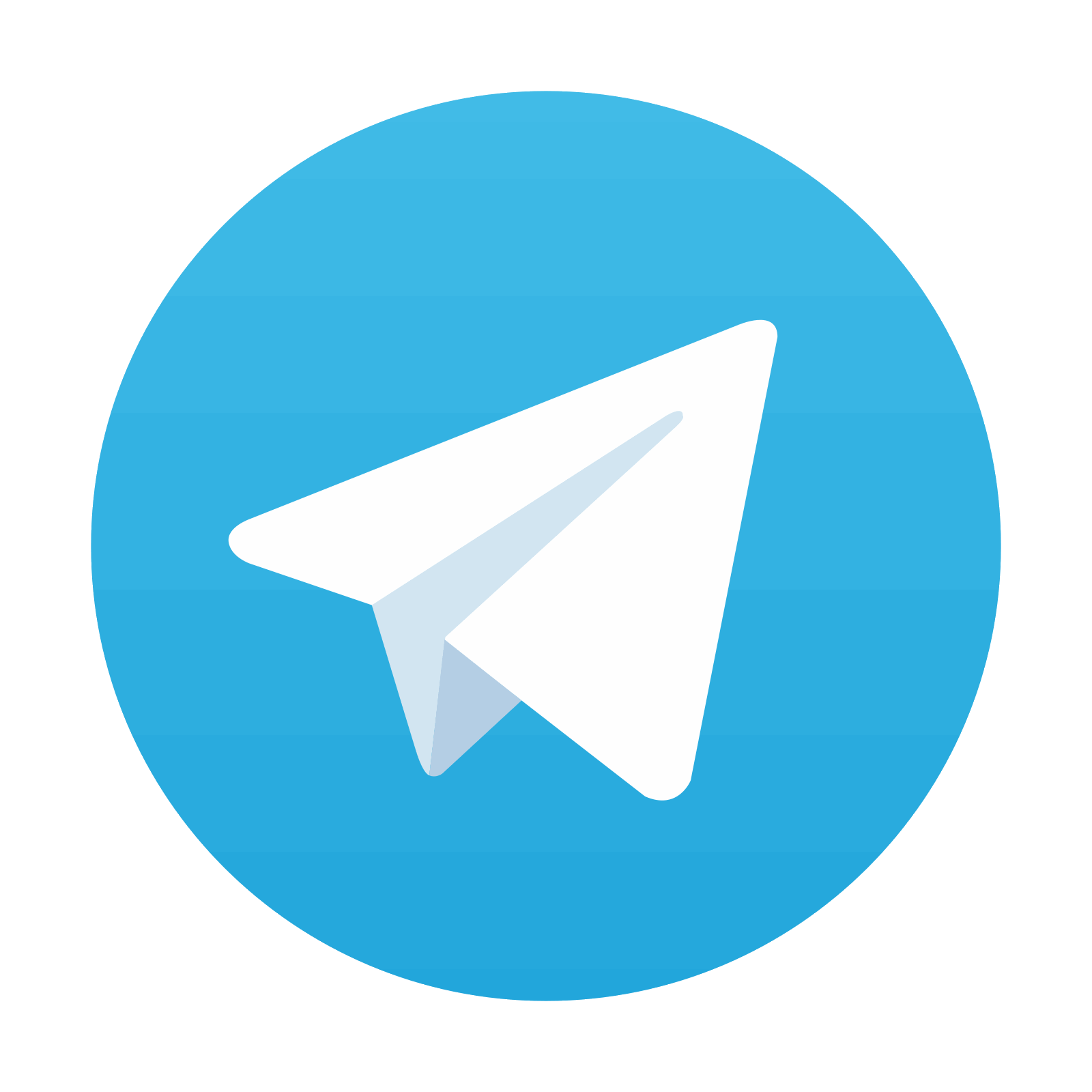
Stay updated, free articles. Join our Telegram channel

Full access? Get Clinical Tree
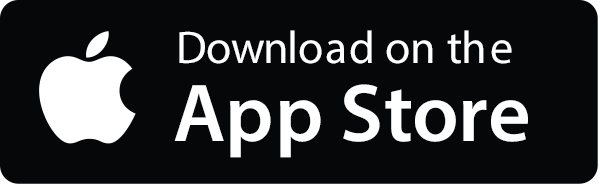

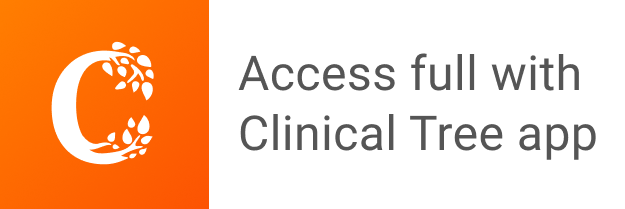