25.1 Introduction
As noted in other companion chapters in this book, computer-aided detection (CADe) is a form of radiology practice in which the radiologist makes a clinical diagnosis by interpreting the images with the assistance of a CADe computer, which analyzes the images independently, and detects and marks potential abnormalities in the image. The premise of CADe is that the CADe computer, and this form of radiology practice, can help radiologists improve diagnostic performance by reducing the number of missed cancers that the radiologist could have detected, which the CADe computer would help the radiologist detect. Several of the preceding chapters discuss CADe in various aspects; in this chapter, we focus on the common evaluation studies that assess the clinical effect of CADe.
It is important to evaluate the clinical effect of CADe. This evaluation is needed to verify the premise of CADe that it benefits clinical practice and it benefits the performance of radiologists. More importantly, this evaluation is needed to clearly indicate that the benefit of CADe outweighs any “cost” associated with its use. There is a financial cost from CADe because it represents an advanced technology. But there are also other costs that may be more important. At the present time, CADe computers invariably mark false positives as they mark cancers. Radiologists can easily dismiss most of the CADe computer false positives, but it takes time for radiologists to review the false-positive marks. If radiologists do not dismiss all of the CADe computer false positives, then additional diagnostic work-up studies will become necessary, without yielding cancer diagnosis in the end. Such CADe-prompted false-positive diagnostic studies are an important clinical concern. Furthermore, the assurance of improved diagnostic performance that radiologists and patients may feel from the use of CADe is justified only if CADe lives up to its promise of helping radiologists detect cancers. A false assurance, if the improvement in diagnostic performance did not materialize, would be dangerous.
To evaluate the clinical effect of CADe is a challenging endeavor. This is true for most new imaging technologies but perhaps more so for CADe because it is not simply a new imaging modality which can be compared straightforwardly with the conventional imaging modality. CADe is a technology that enhances the conventional imaging modality (e.g., conventional screening mammography) and, because of that, one must compare the conventional imaging modality against the conventional imaging modality plus CADe.
That the conventional imaging modality appears in both sides of the comparison complicates the evaluation studies. In addition, to evaluate CADe, one must consider several distinct aspects involved in the practice of interpreting images with the assistance of CADe: the CADe computer, the radiologist, and the interaction of the radiologist with the CADe computer. There are several types of common evaluation studies, which we will discuss in this chapter. These are: (1) studies of the clinical potential of CADe; (2) laboratory observer performance studies; (3) clinical “head-to-head” comparisons; (4) clinical “historical control” studies; and (5) randomized controlled clinical trials.
25.2 Some Common Types of CADe Study
25.2.1 Studies of Clinical Potential
For CADe to help radiologists detect cancer, the CADe computer must be able to detect cancer on its own. Further, the CADe computer must be able to detect cancer that radiologists miss. Without these capabilities, the CADe computer cannot possibly help the radiologist. However, these capabilities are only an indication that the CADe computer could help – it does not necessarily mean that the CADe computer will help. For the CADe computer to help a radiologist detect cancer, the radiologist must first miss the cancer, then the CADe computer must detect the cancer, and finally the radiologist must recognize the cancer once the CADe computer points it out. Therefore, the ability of a CADe computer to detect cancers that radiologists tend to miss is a necessary – but not sufficient – condition for CADe to help radiologists detect cancer.
It is nevertheless important to test a CADe computer to see whether it can detect cancers that radiologists tend to miss. This is the first necessary test of the CADe computer before it advances to the clinical arena. Historically, this was also an important test for the field of CADe research; this test demonstrated the potential of CADe at a time when it was uncertain in the scientific and medical community whether it was at all possible for a computer to be good enough to help the well-trained and sophisticated specialist observer – the radiologist – in a complex image perception and interpretation task. This test provided a reason for CADe to be later evaluated in clinical studies.
To demonstrate this potential benefit, researchers concentrated on cancers that have been missed by radiologists in routine clinical practice. A cancer is missed if it is present in a mammogram but the interpreting radiologist did not recognize the lesion as abnormal or did not consider the lesion suspicious for cancer, and the cancer is detected subsequently after the screening examination. Because it is easier for one to see the cancer once it is pointed out by someone else, it is also common to require that not only the cancer is visible retrospectively in the mammogram but also a panel of experienced radiologists consider, retrospectively, that the lesion is suspicious enough that the original interpreting radiologist should have acted on it and recalled the patient for additional imaging study. Using mammograms containing missed cancers, researchers showed that CADe computers were indeed able to detect many of those cancers in the mammograms where the original interpreting radiologist failed to recall the patient for additional imaging study. These studies provided tangible evidence of the potential for the CADe computer to help radiologists detect cancer.
The design of this type of study is straightforward. It involves the assembly of a missed-cancer image database and the analysis of the images with a CADe computer. The most demanding task is to assemble the image database because it is not easy to identify a large number of cancers that are clinically missed. After such cases are identified, a panel of expert radiologists usually reviews and assesses the appropriateness of the cases for the study to the best of their ability.
The main outcome of this type of study is how many missed cancers the CADe computer can detect. It is not uncommon for a CADe computer to detect about half of missed cancers. A second important outcome is how many false positives the CADe computer also detects as it detects the cancers. If the CADe computer makes several detection marks in each image, then it is possible for missed cancers to be marked by chance rather than by actual computer detection – the greater the number of computer marks per image, the greater the possibility of a cancer being marked by chance. Large numbers of false-positive computer marks are undesirable also because they indicate that the computer detection results are nonspecific and radiologists must review many computer false-positive marks before finding a cancer.
Researchers must decide before the study the scoring criteria to consider a CADe computer mark as a “hit” on a cancer. For obvious reasons, the computer performance is inversely related to the scoring criteria: the computer will appear to detect more cancers with a set of lax scoring criteria and detect fewer cancers with a set of stringent scoring criteria, all without actually changing the computer technique or its performance. If the criterion is that the center of a computer-detected object be within a specified distance from the center of a lesion, then obviously the greater the specified distance, the more likely computer detections will be scored as true positives, and vice versa. It is difficult to come up with a rule that evaluates the computer detection performance entirely objectively and prevents chance detections from being counted as true positives. It is common to require that a computer detection overlaps with a lesion for the computer mark to be counted as a true positive. It is also helpful if a radiologist reviews the computer marks to decide whether each computer mark is a true positive.
An example of a study of the clinical potential of CADe is an article by Warren Burhenne et al. (2000). In this study, the researchers analyzed 1083 biopsy-proven breast cancers and found 427 cases had a prior mammogram. A panel of radiologists evaluated these prior mammograms and determined that 115 mammograms could be considered as missed cancer cases. Of these “missed” cancers, the CADe computer marked 89 cancers, or 77%. Therefore, this study demonstrated a potential for the CADe computer to help radiologists detect breast cancer in mammograms earlier.
25.2.2 Laboratory Observer Performance Studies
To test whether a CADe computer can help radiologists detect cancers, radiologists must be involved in the test so that the scenario in which the CADe computer helps a radiologist detect cancer can be actually observed in the test. One can conduct such a test in a controlled, laboratory environment, known as a laboratory observer performance study.
The purpose of a laboratory observer study is to measure the performance of observers in the interpretation of a specific kind of image or, alternatively, to compare observer performance in the interpretation of two (or more) kinds of images. In the case of CADe, an observer study compares the observer performance in interpreting a specific kind of image (such as mammograms) alone versus interpreting the same images with the assistance of a CADe computer. Both the observer performance in interpreting the images without and with the CADe computer assistance are measured in the experiment, and a comparison is made between the two. Laboratory observer performance studies are commonly analyzed with receiver operating characteristic (ROC) analysis; although not necessarily required, an observer study almost invariably implies an ROC study. ROC analysis provides a fundamentally meaningful depiction of the observer performance by simultaneously examining sensitivity, specificity, and the tradeoffs that are available between them. In the last 20 years, ROC analysis has been developed successfully to address many complex issues in the measurement and the comparison of observer performance with statistical validity, great attention to experimental details, and improved efficiency. For an introduction to ROC analysis, the reader should consult other chapters in this volume and the reviews by Wagner et al. (2007) and Metz (1978, 1989).
In a laboratory observer performance study, one tries to replicate as closely as possible the clinical conditions in which images are interpreted. One also tries to control the experiment to eliminate as much as possible distractions and uncertainties that are part of clinical practice. To facilitate ROC analysis, the experimenter must know in every case the diagnostic truth with respect to the diagnostic task being studied, but this knowledge is withheld from the observers to simulate the condition of clinical image interpretation. The general design of a laboratory observer study includes the collection of a set of images and the selection of observers, having the observers interpret the images both with and without the CADe computer assistance in a carefully designed, controlled environment during which data required for ROC analysis are collected, and subsequently performing ROC analysis on the collected data. In the following, we describe these components in more detail.
For a laboratory observer study to replicate the conditions of clinical image interpretation, the images should be representative of those encountered clinically. However, in many situations this is neither practical nor desirable. Take breast cancer, for example. Because the cancer prevalence is extremely small (about 5 cancers per 1000 women screened in an average-risk screening population), to ensure that a study includes a sufficient number of cancers, the study must also include a much larger number of noncancer cases, causing the study to become extremely large. This is a particularly difficult problem for studies that address screening (asymptomatic) patient populations. For diagnostic examination (i.e., the follow-up or work-up imaging studies after an abnormality is identified in screening) patient populations, although cancer prevalence is greater, this is still a problem because the number of noncancer cases is still several times that of cancer cases. In a study with small cancer prevalence, much of the observers’ time will be spent on reading cases that are not cancer. Further, although the total number of cases is large, the statistical power of the study is determined primarily by the smaller number of cancer cases, rather than the larger number of noncancer cases.
For these reasons, laboratory observer performance studies usually do not randomly sample clinical cases. Instead, it is common to enrich the cancer prevalence in an observer study such that there are disproportionately more cancer cases compared with routine clinical practice. A common practice is to balance the numbers of cancer and noncancer cases about equally because in this way they both contribute equally to the statistical power and, therefore, improve the efficiency of the experiment. However, a fundamental question arises when one artificially increases the number of cancer cases: does the change in cancer prevalence affect observer performance and decision making? It is important to realize that the ROC curve does not explicitly depend on the disease prevalence, i.e., the ROC curve is invariant as one changes the disease prevalence. An intuitive explanation for this is that the innate diagnostic ability of the observers is not affected by the disease prevalence.
To prove this assertion experimentally is extremely difficult because small cancer prevalence requires extremely large studies. Nevertheless, studies have been done with progressively smaller disease prevalence that showed little effect of the disease prevalence on the ROC curve (Gur et al., 2007). However, questions still remain of whether the clinical decisions that radiologists make are affected by the change in cancer prevalence. For example, radiologists strive in screening mammography to detect as many breast cancers as possible while simultaneously maintaining a reasonably low percentage of patients recalled for additional imaging study, knowing that a great majority of the recalled patients do not have breast cancer. If the cancer prevalence is artificially changed substantially, then a radiologist will not be able to maintain both identical sensitivity and identical recall rate in the observer study as in routine clinical practice. Under those conditions, how radiologists change their decision making in the observer study and how those changes affect their sensitivity, or recall rate, or both, is not clear.
Similar to case selection, the observers who take part in an observer study also should be representative of those who interpret the images clinically. In the USA, radiologists must meet the requirements of the Mammography Quality Standard Act (MQSA) to interpret mammograms clinically. It is therefore common to use that requirement as a threshold to select observers in observer studies. A key component of the MQSA is that a radiologist must interpret a minimum of 960 mammograms in a 2-year period, or 480 mammograms per year. During the early development of CADe, it was common for highly experienced radiologists to serve as observers. However, because the number of highly experienced radiologists is small and they interpret only a small fraction of the total clinical cases, it is also important that observer studies include radiologists from a broader pool of practice styles and interpretation skill levels. Subsequent observer studies often include community radiologists as observers.
Many study design details of how observers interpret images can potentially bias the results when comparing two imaging modalities in a laboratory observer performance study. For example, it is conceivable that radiologists perform better in interpreting an image simply by spending more time on it. It is also possible that if a radiologist always interprets one imaging modality first and then, immediately after, interprets the second imaging modality, then the performance measured from interpreting the second image modality is likely the result of interpreting both imaging modalities, because the information that the radiologist has attained from the first imaging modality remains present as the radiologist interprets the second imaging modality.
One can design the experiment in such a way that the effects of these potential biases tend to cancel each other rather than accumulate during the course of the study. A popular study design, sometimes referred to as the counterbalanced study design, can be summarized as follows. Divide the cases into two comparable halves and divide the observers into two comparable groups. The first group of observers will read the first half of cases in imaging modality A and read the second half of cases in imaging modality B. The second group of observers will read the first half of cases in imaging modality B and read the second half of cases in imaging modality A. After all images are read, wait several weeks to discourage the observers’ memory of reading the images for the first time from influencing their reading of the images for a second time. After this memory “washout” interval, all observers read the images for a second time in the imaging modality that they have not read. This study design minimizes potential biases from reading-order effects because such effects tend to cancel between the two halves of the study.
In general, the interval between the two readings is important to ensure that the observers read the two imaging modalities independently. However, observer studies of CADe are special in that the reading of the second modality (images plus CADe assistance) can immediately follow the reading of the first modality (images alone). The reason for this is that that is how CADe may be used clinically: a radiologist interprets the images and then, immediately after, consults the CADe computer results for concurrence or for potential abnormalities that he or she has not noticed. In the spirit of duplicating how cases are interpreted clinically in the laboratory observer performance study, it is reasonable to modify the standard study design specifically for CADe studies, such that each observer interprets all cases twice in a single sitting, and interprets each case first with the images alone and, immediately after rendering a diagnostic opinion, interprets the images again with the CADe computer results present, followed by the rendering of a second, potentially modified, diagnostic opinion. This study design is known sometimes as the “sequential study,” whereas the standard, counterbalanced study design is referred to as the “independent study.”
Observers may behave differently in an independent study compared with in a sequential study. The independent study is designed specifically to discourage observers from remembering, when they interpret an image for the second time, what their impression was when they interpreted the image for the first time. On the other hand, in a sequential study observers know exactly what their impression was from reading the images alone when the CADe computer results are made available to them. Therefore, observers tend to “change their mind” less frequently in a sequential study compared with in an independent study in which they would appear to “change their mind” simply because they could not duplicate their previous impression exactly. This may seem to be an advantage of the independent study given that CADe only helps radiologists if it can persuade radiologists to change their mind toward the correct diagnosis. However, the sequential study can yield greater statistical power in comparing reading images alone against reading images with the assistance of CADe because of improved observer consistency and reduced random statistical noise between the observers’ diagnostic opinions attributed to interpreting the images with and without the assistance of CADe (Beiden et al., 2002). It has been shown that, reassuringly, a study conducted twice with both the independent and the sequential designs produced similar results (Kobayashi et al., 1996).
One of the early laboratory observer performance studies was done by Chan et al. (1990). They selected 60 single-view mammograms, of which 30 mammograms each contained a subtle cluster of microcalcifications and the other 30 mammograms did not contain clustered microcalcifications. Seven attending radiologists and eight radiology residents participated in the study as observers. The results of the observers’ “composite” ROC curves (Figure 25.1) show that CADe statistically significantly (p < 0.001) improved the radiologists’ performance interpreting these mammograms in the detection of clustered microcalcifications.
Figure 25.1 Receiver operating characteristic curves adapted from the observer performance study of Chan et al. (1990) showing statistically significant (p < 0.001) improvement in 15 radiologists’ performance in detecting clustered microcalcifications from interpreting mammograms with the assistance of computer-aided detection (CADe).
25.2.3 Clinical Head-to-Head Comparisons
One way to test in the clinical setting whether CADe helps a radiologist detect cancers is to compare on a case-by-case basis the radiologist’s interpretation of images with and without CADe assistance. We call this the “head-to-head” comparison.
The goal of the head-to-head comparison is to determine the frequency and the specific cases in which CADe helps radiologists detect cancer. To do this, radiologists interpret each case first without the CADe computer assistance and formally record their findings in the case. Then, the radiologists review the CADe computer results and interpret the case again, potentially modifying their findings prompted by the marks of the CADe computer, and formally record a second set of findings (or modify the initial set of findings). The radiologists do so in every case over a period of time, during which cancer cases will be encountered and, hopefully, cases in which CADe helps the radiologists detect the cancer also will be encountered.
Because of low cancer prevalence in screening and because CADe is expected to help radiologists only when they initially miss the cancer, which presumably is infrequent, the head-to-head comparison must go through a large number of cases to accumulate the encounters of cancer cases in which CADe helps a radiologist detect the cancer. This translates into long periods of study. Because of the additional work required to record two sets of findings in each case, this type of study usually accumulates only a limited number of cancer cases.
The advantages of head-to-head comparisons are that CADe is used in the daily clinical practice as it is intended and that one can identify the specific cases in which CADe helps radiologists detect the cancer. However, one could raise the question of whether the performance of radiologists in the study is indeed the same as their ordinary performance outside of the study. The presumption of the head-to-head study is that, when radiologists first interpret a case without the assistance of the CADe computer, they perform in exactly the same way as they do ordinarily outside of the study.
However, there are plausible arguments that claim radiologists might be more – or less – vigilant than usual. Because it is apparent to radiologists that their performance interpreting the cases without the assistance of the CADe computer will be compared with the performance of the CADe computer, radiologists may feel pressured to compete with the CADe computer and, consciously or subconsciously, be more vigilant than usual in detecting abnormalities. On the other hand, because the radiologists know that any abnormality missed in their initial interpretation has a chance of being caught by the CADe computer and by their second read of the images, radiologists may be less vigilant in their initial interpretation than usual. It is commonly accepted that CADe computers can detect clustered microcalcifications in mammograms with high sensitivity (in excess of 90%). Therefore, it may seem reasonable to some radiologists to do only a cursory search for calcifications on their own and rely on the CADe computer to find other subtle calcifications, which the radiologists would then interpret after the CADe computer marks them. If radiologists operate in this way in a head-to-head comparison study, then their performance reading the images alone will not be the same as their performance without the assistance of CADe.
An example of the clinical head-to-head comparison was done by Freer and Ulissey (2001). Their study lasted 12 months and included 12,860 screening mammograms and two radiologists. Each mammogram was initially interpreted without the assistance of CADe, followed immediately by a re-evaluation of areas marked by the CADe computer. They reported an increase in the number of cancers detected of 19.5% (from 41 cancers detected without the assistance of CADe to 49 cancers detected with the assistance of CADe) and a corresponding increase in the recall rate from 6.5% to 7.7%.
25.2.4 Clinical Historical Control Studies
Another way to test in the clinical setting whether CADe helps radiologists detect cancer is to compare radiologists’ performance interpreting some cases without the assistance of CADe against their performance interpreting some other cases with the assistance of CADe. In this way, radiologists avoid having to interpret and record the findings twice in every case.
One way to implement such a study efficiently is to compare the performance of a group of radiologists after they have begun to use CADe against their historical performance before they began to use CADe. In this way, the radiologists act as their own controls (hence the term “historical control”). However, such a study does not isolate the effect of CADe alone; any change in the radiologists’ performance is the summary result of whatever has changed between the two historical time periods, including the use of CADe, but also including any other changes such as radiologist staffing changes, improvements in their interpretation skills, and any underlying changes in the patient population. Therefore, such a study is sensible only for well-established practices with a stable patient population and a stable radiologist staff, for which the assumption would appear plausible that little else has changed except for the addition of CADe during the study.
In addition to the requirement of stable practice, detailed and high-quality audit of practice data is also critical to a historical control study. At a minimum, the audit data should show the total number of patients undergoing an imaging study, the number of cancers diagnosed as a result of the imaging study, the number of false-positive imaging findings (e.g., a recall for diagnostic breast imaging study in a patient who does not have breast cancer), and the frequency of unintended downtime of CADe in the study. More detailed audit data at the level of individual radiologist or individual cases are desirable. Aside from maintaining a high-quality audit, the study is not different from routine clinical practice in other ways. Every patient, every radiologist, and every case are entered into the study as the clinical imaging study is performed in its routine fashion. Therefore, the study provides a good snapshot of the clinical performance.
An example historical control study is the Gur et al. (2004) study. This study included the screening mammography studies during 2000, 2001, and 2002 interpreted by 24 radiologists at an academic medical center and its satellite breast imaging clinics. The control arm included 56,432 screening mammograms interpreted without the assistance of CADe before the installation of the CADe system and the study arm included 59,139 screening mammograms interpreted with the assistance of CADe after the CADe system was used consistently in their clinical practice. The authors found a similar cancer detection rate with and without the assistance of CADe and also similar recall rates. The cancer detection rate was 3.49 without the assistance of CADe and 3.55 with the assistance of CADe per 1000 screening mammograms. The recall rate was 11.39% without the assistance of CADe and 11.40% with the assistance of CADe.
25.2.5 Randomized Controlled Clinical Trial
The randomized controlled clinical trial offers perhaps the most rigorous design of clinical studies. The historical control study achieves high efficiency in taking an accurate snapshot of clinical performance with the tradeoff of not being able to isolate the effect of one particular change – the use of CADe – from other longitudinal changes during the study. In comparison, the randomized controlled clinical trial overcomes this shortcoming by taking snapshots of clinical performance with and without the assistance of CADe at the same time. To do this, a scheduled imaging study is assigned randomly with equal probability to either the study arm or the control arm. Radiologists interpret cases assigned to the study arm with the assistance of CADe and interpret cases assigned to the control arm without the assistance of CADe. Each radiologist should interpret an equal number of cases with and without the assistance of CADe. In this way, the clinical performance with and without the assistance of CADe can be compared directly because the patient populations are comparable, the radiologists are comparable, and, except for the assistance of CADe, the imaging studies are interpreted in a comparable way.
The randomized controlled clinical trial is the gold standard in drug and interventional studies. However, whether the randomized controlled trial is appropriate for CADe studies also depends on cost-effectiveness. A randomized controlled trial is highly demanding on resources. Because every case must be randomly assigned to either the study arm or the control arm, it is not adequate to audit the cases only retrospectively as is commonplace in a historical control study, but every case must be tracked prospectively. And because in almost all cancer screening situations the prevalence of cancer is extremely low, the study must accrue an extremely large number of patients and, consequently, last a long time. The historical control study must also accrue a similarly large number of patients, but because the data are obtained through retrospective audit, there is only minimal added effort to the otherwise routine clinical practice, whereas in the randomized controlled trial the prospective tracking of every case demands a large effort on the part of the investigators. In particular, the random assignment of radiologists to interpret some images with and some images without CADe adds considerable logistic complication to the workflow of radiologists compared with their otherwise routine clinical practice.
Because of the high cost and the complexity associated with randomized controlled trials, the decision to perform such a trial is not made lightly. A number of randomized controlled trials have been done to determine the efficacy of screening mammography for the detection of breast cancer in asymptomatic women. A similar trial (rather than randomized, in this trial every patient was imaged with both imaging modalities) has been done to determine the efficacy of full-field digital mammography as compared with conventional screening-film mammography (Pisano et al., 2005). However, no randomized controlled trial has been done to study CADe.
25.3 What we Have Learned from CADe Studies
25.3.1 Summary of Types of CADe Study
A large number of studies have been done to demonstrate the clinical potential of mammography CADe. Many of these studies were done in the early phase as CADe was being introduced into clinical practice and were done to collect evidence in support of the clinical use of CADe. Table 25.1 lists ten of this type of study. In total, these studies include about 1000 “missed” cancers – cancers not diagnosed in the mammograms studied but diagnosed subsequently. The CADe computer flagged about 50% of these “missed” cancers, with a range of 13–77% reported in the individual studies. Therefore, these studies indicate that the CADe computer can flag about 50% of breast cancers in mammograms before the cancers are diagnosed in routine clinical practice without the assistance of CADe. This finding suggests that there is a great clinical potential for the CADe computer to help radiologists detect breast cancers earlier. However, these studies indicate only the clinical potential – they do not indicate what fraction of the cancers that the CADe computer flags the radiologist recognizes as cancer.
Table 25.1 Studies of clinical potential of mammography computer-aided detection
Reference | Journal | Location |
---|---|---|
te Brake et al. (1998) | Radiology | Netherlands |
Warren Burhenne et al. (2000) | Radiology | Canada, CA |
Birdwell et al. (2001) | Radiology | CA, USA |
Zheng et al. (2002) | Academic Radiology | PA, USA |
Brem et al. (2003) | American Journal of Roentgenology | PA, USA |
Karssemeijer et al. (2003) | Radiology | Netherlands |
Destounis et al. (2004) | Radiology | NY, USA |
Ikeda et al. (2004) | Radiology | CA, USA |
Ciatto et al. (2006) | Breast | Italy |
Skaane et al. (2007) | American Journal of Roentgenology | Norway |
Several observer studies have been done to study the effect of mammography CADe on radiologists’ performance in detecting breast cancer in screening mammograms. Table 25.2 lists eight of these studies. All of these studies reported improved performance of the radiologists when they used the assistance of CADe compared with their unaided performance. Although there is the possibility that the literature is biased toward publishing studies with positive findings and, therefore, there could have been studies that did not find a statistically significant difference in performance that were not published, we are unaware of any such null-result studies. Given the large effort required to conduct these observer performance studies, such null-result studies would have been rare if they existed.
Table 25.2 Laboratory observer performance studies of mammography computer-aided detection
Reference | Journal | Location |
---|---|---|
Chan et al. (1990) | Investigative Radiology | IL, USA |
Kegelmeyer et al. (1994) | Radiology | CA, USA |
Moberg et al. (2001) | European Journal of Radiology | UK |
Marx et al. (2004) | European Journal of Radiology | Germany |
Alberdi et al. (2005) | British Journal of Radiology | UK |
Taylor and Given-Wilson (2005) | British Journal of Radiology | UK |
Gilbert et al. (2006) | Radiology | UK |
Taplin et al. (2006) | American Journal of Roentgenology | WA, USA |
Several clinical “head-to-head” comparisons of radiologists’ performance in detecting breast cancers in screening mammograms with and without the assistance of CADe have been done. Table 25.3 lists seven of these studies. In all, these studies include about 60,000 screening mammography cases, from which the radiologists detected 319 cancers without the assistance of CADe, and detected 31 additional cancers with the assistance of CADe. These results amount to an increase in sensitivity of 9.7% with a concurrent increase in recall rate of 12.5%. The individual studies reported a range of increase in sensitivity from 4.7% to 19.5%, and a range of increase in recall rate from 8.2% to 25.8%. Note that, because of the extremely low breast cancer prevalence in the average-risk screening population, the total number of cancers and the number of cancers detected because of the CADe computer assistance are small even with a total number of 60,000 screening mammograms.
Table 25.3 Clinical head-to-head comparisons of mammography computer-aided detection (CADe)
Reference | Journal | CADe | Location | Practice |
---|---|---|---|---|
Freer and Ulissey (2001) | Radiology | R2 | TX, USA | Community |
Helvie et al. (2004) | Radiology | In-house | MI, USA | Academic |
Birdwell et al. (2005) | Radiology | R2 | CA, USA | Academic |
Khoo et al. (2005) | Radiology | R2 | UK | Program |
Dean and Ilvento (2006) | American Journal of Roentgenology | iCAD | CA, USA | Private |
Ko et al. (2006) | American Journal of Roentgenology | iCAD | MA, USA | Academic |
Morton et al. (2006) | Radiology | R2 | MN, USA | Academic |
Four historical control studies have been conducted (Table 25.4). These are large studies. In total, these studies include about 228,000 screening mammogram cases read with the assistance of CADe and 687,000 screening mammogram cases read without the assistance of CADe – the large imbalance is due, mostly, to the Fenton et al. (2007) study, which included disproportionately more cases read without the assistance of CADe than the cases read with the assistance of CADe.
Table 25.4 Clinical historical-control studies of mammography computer-aided detection (CADe)
Reference | Journal | CADe | Location | Practice |
---|---|---|---|---|
Gur et al. (2004)1 | Journal of the National Cancer Institute | R2 | PA, USA | Academic |
Cupples et al. (2005) | American Journal of Roentgenology | R2 | SC, USA | Community |
Fenton et al. (2007) | New England Journal of Medicine | R2 | USA | Community |
Gromet (2008) | American Journal of Roentgenology | R2 | NC, USA | Community |
1 See also Feig et al. (2004).
The results of these studies do not agree. The Gur et al. (2004) study reported an increase in the cancer detection rate of 1.7% and an increase in the recall rate of 0.1%. A subsequent reanalysis of that study by Feig et al. (2004) of a subset of radiologists excluding those who were high-volume readers found a 19.7% increase in the cancer detection rate and 14.1% increase in the recall rate. The Cupples et al. (2005) study reported a 16.3% increase in the cancer detection rate and an 8.1% increase in the recall rate. The Fenton et al. (2007) study reported a statistically nonsignificant 1.2% increase in the cancer detection rate and a 30.7% increase in the recall rate. The Gromet (2008) study reported a 1.9% increase in the cancer detection rate and a 3.9% increase in the recall rate.
These disparate results raise questions and have prompted confusion and debate over the clinical benefit of CADe. The Fenton et al. (2007) study offers the most extreme position, in concluding1 that “the use of computer-aided detection is associated with reduced accuracy of interpretation of screening mammograms.”
25.3.2 The Relations Between Laboratory Observer Studies and Historical Control Clinical Trials and Why They may Find Different Results
The disparate findings in the historical control trials contradict the consistency in the positive findings from laboratory observer performance studies. Why does CADe, a new technology that has been tested extensively in laboratory observer studies and has consistently produced strong evidence of decision-making benefits, fail to produce the same consistent results in large clinical trials? Are these inconsistent trial results valid grounds to question whether CADe is clinically beneficial? If CADe is clinically beneficial, what are its clinical effects and how can we measure those beneficial effects consistently and unambiguously?
These are important questions that are not easy to answer. Of the several types of study that we have described, we focus on the differences between the laboratory observer studies and historical control clinical trials because a laboratory observer study is probably the most rigorous study that can be done in the laboratory environment outside of the realm of clinical practice and the historical control clinical trials are by far the largest clinical studies (it is difficult for head-to-head comparison studies to achieve a similarly large size).
There are important differences between laboratory observer studies and clinical trials and there are important differences between the data analyses of these studies. Laboratory observer studies often use ROC analysis, sensitivity, and specificity as the fundamental performance metrics. The sensitivity and specificity can be calculated in an observer study because the study uses only cases of known diagnosis. For all practical purposes, sensitivity and specificity cannot be calculated in a clinical trial because the diagnostic “truth” in each and every case – required to calculate sensitivity and specificity – is not known.
In clinical trials the performance metrics most often calculated are the cancer detection rate and the recall rate. The cancer detection rate is the number of cancers detected in a particular cohort of patients. The cancer detection rate in breast cancer screening is approximately 4–5 cancers detected per 1000 average-risk women screened. The recall rate refers to the fraction of patients recalled for additional diagnostic studies after the initial screening study resulted in an abnormal finding. The recall rate for screening mammography ranges from a few percent to over 10%, even approaching 20%. The cancer detection rate and the recall rate are related to the sensitivity and specificity through the cancer prevalence, which is an unknown quantity. Therefore, one cannot calculate sensitivity and specificity from cancer detection rate and recall rate, nor vice versa.
25.3.2.1 The Cancer Detection Rate is not Expected to Increase
The premise of using the cancer detection rate as one of the primary endpoints in a clinical trial is based on the expectation that if CADe helps radiologists detect cancers then the cancer detection rate will increase. However, that expectation may be flawed. Cancer is relatively rare in the average-risk asymptomatic population and the cancer prevalence is generally stable over time. The prevalence of breast cancer in the average-risk, asymptomatic population is approximately 5 per 1000 women and is believed to be stable over time (Jemal et al., 2008). If we ignore, for a moment, the plausible assertion that cancer prevalence is approximately constant over time, then if CADe helps radiologists detect more cancers, it would be plausible to expect the cancer detection rate to increase. However, if we take into account that cancer prevalence remains approximately constant over time, then the effect of CADe helping radiologists detect more cancers on the cancer detection rate becomes more complicated, and the effect varies depending on at which time point we look at the cancer detection rate.
Initially, when CADe is introduced into clinical practice, if CADe helps radiologists detect more cancers, then the cancer detection rate will be greater compared with the baseline cancer detection rate without the assistance of CADe. However, in the next screening round, because CADe has already helped radiologists detect some cancers that normally would not have been detected already (in the last screening round) had CADe not been used, the number of cancers that normally could have been detected in the current screening round would be smaller compared with the conventional practice without the assistance of CADe. If CADe again helps radiologists detect some cancers that they normally would not have detected, then the total number of cancers detected – i.e., the sum of the smaller number of cancers that radiologists normally would have detected without the assistance of CADe and the number of cancers that CADe helps radiologists to detect – might be similar to the number of cancers that radiologists normally would detect without the assistance of CADe.
Over time, the cancer detection rate probably does not change substantially compared with the baseline cancer detection rate without the assistance of CADe, even as CADe helps radiologists detect cancers that they are normally not able to detect without the assistance of CADe. But an important difference is that the cancers that radiologists detect because of the assistance of CADe are detected earlier than they normally would have been without the assistance of CADe. This is analogous to the benefit of screening mammograms, which primarily help to detect breast cancers early when they can still be treated effectively, rather than detecting more of them. Nishikawa (2006) has studied these dynamics in detail with stochastic modeling and has found quantitative evidence that the cancer detection rate might not change substantially as CADe helps radiologists detect more cancers.
25.3.2.2 The Cancer Detection Rate is Difficult to Measure
When the cancer detection rate does increase, it is entirely another challenge to measure that increase accurately. In an average-risk asymptomatic population, the prevalence of breast cancer is approximately 5 per 1000 women, and the cancer detection rate with mammography is somewhat smaller than that, perhaps 4 per 1000. It should be clear that the statistical uncertainty in measuring these small rates is quite large, especially when the total number of cancers is small. The binomial statistics indicate that if the expected cancer detection rate is 4 per 1000 women, then the standard deviation in the observed cancer detection rate is expected to be 2 per 1000 women for a cohort study of 1000 women, 0.9 per 1000 women for a cohort study of 5000 women, and 0.6 per 1000 women for a cohort study of 10,000 women. Often, this problem is recognized and a common remedy is to combine the cases interpreted by several radiologists to calculate the aggregate cancer detection rate of a group of radiologists instead of calculating the cancer detection rate of each individual radiologist, because the uncertainty in the calculated cancer detection rate decreases as the total number of cases becomes larger. However, what may not be obvious is that, when comparing two cancer detection rates, e.g., when comparing the cancer detection rates with and without the assistance of CADe, the uncertainty is greater than the uncertainty of the individual cancer detection rates. This is illustrated schematically in Figure 25.2.
Figure 25.2 Illustration that the aggregate cancer detection rate of five radiologists reduces the uncertainty in the cancer detection, but calculating the difference between two cancer detection rates results in greater uncertainty. Bottom: Aggregate cancer detection rate calculated from five radiologists based on data in Jiang et al. (2007; also shown in Figure 25.3). Data shown as circles are calculated directly from Jiang et al. (2007) and data shown as crosses are postulated with an increase of one additional cancer detected per 1000 screening mammograms by every radiologist. Top: The difference in the two cancer detection rates. The mean of the difference is 1/1000, as is postulated. Note the greater uncertainty associated with the difference of the cancer detection rates. PDF, probability density function.
In addition to this statistical uncertainty, measurement of the cancer detection rate is influenced by variability in the performance of radiologists – whereas some radiologists are able to operate at a relatively high cancer detection rate and maintain the recall rate at a reasonably low level, other radiologists operate at a smaller cancer detection rate and are often compelled to operate at a relatively high recall rate. This variability is well known and it is generally agreed that large variability in radiologists’ performance in the interpretation of screening mammograms exists, although accurate quantification of the extent of it is often difficult (Beam et al., 1996; Elmore et al., 1994; Schmidt et al., 1998). Interradiologist variability compounded with statistical uncertainty increase the uncertainty in the measurement of the cancer detection rate and the measurement of the difference between two cancer detection rates (with and without the assistance of CADe).
How large is the uncertainty in the measurement of the cancer detection rate? Jiang et al. (2007) calculated the single-radiologist cancer detection rate based on the clinical practice data collected by the Breast Cancer Surveillance Consortium (Ballard-Barbash et al., 1997) in seven US regional registries: the Carolina Mammography Registry, Chapel Hill, NC; the Colorado Mammography Project, Denver, CO; the New Hampshire Mammography Network, Lebanon, NH; the New Mexico Mammography Project, Albuquerque, NM; the San Francisco Mammography Registry, San Francisco, CA; the Vermont Breast Cancer Surveillance System, Burlington, VT; and Group Health Cooperative, Seattle, WA. The data cover the period between January 1, 1996 and December 31, 2002, and include 510 radiologists, each of whom read at least 500 mammograms within the study during the study period. A total of 2,289,132 screening mammograms, and 9030 screen-detected breast cancer cases, are included in the study.
Analysis of these data showed that the average single-radiologist cancer detection rate was 3.91 cancers per 1000 screening mammograms, with a standard deviation of 1.93 cancers per 1000 screening mammograms (Figure 25.3). The range of the cancer detection rate was 0.25–13.75 cancers per 1000 screening mammograms. Clearly, there are large variations in the single-radiologist cancer detection rate calculated from this large data set that covers a broad cross-section of the clinical screening mammography practice in the USA.
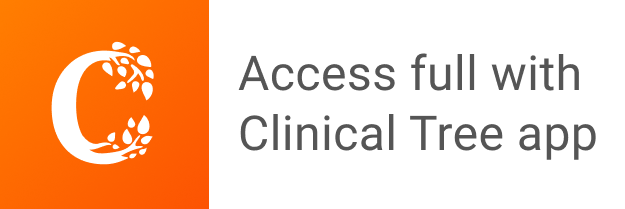