33.1 Role of Perception in Pathology
The practice of pathology is largely dependent on visual inspection of a deceased human body (e.g., autopsy, forensics), patient specimens (e.g., gross pathology), or microscopic material examined using a microscope (e.g., surgical pathology, cytopathology, hematology). Hence, academic pathology departments are generally organized into two divisions: (1) anatomic pathology, which includes surgical pathology, cytopathology, and autopsy pathology, and (2) clinical pathology, which includes chemistry, clinical microbiology, hematology, cytogenetics, genomics/molecular, and toxicology. There are also numerous subsection specialties within pathology, with as many as 20 or more organ-based pathology subsections ranging from cardiac to neuropathology. This diversity leads to significant diversity in the types of task a pathologist engages in, but at a core level there are key similarities. A pathologist’s ability to identify, classify, and interpret (i.e., perceive) gross and/or microscopic pathologic findings using visual information influences his or her ability to accurately make a diagnosis. Pathologists sometimes also use touch for diagnoses (e.g., firmness of a resected lung with pneumonia on palpation).
For over a century, pathology has been based on the use of light microscopy for medical diagnoses, using visible light and magnifying lenses to examine specimen details. The objective lenses in microscopes are characterized by two parameters: magnification (2× to 100×), and numerical aperture (0.14–0.7). Of particular interest for today’s pathologist is the role of whole slide imaging (WSI) in digital pathology, as it has recently been approved for primary diagnosis in surgical pathology for slides prepared from formalin-fixed tissue (Food and Drug Administration, 2017), and the evidence for its utility is substantial (Bashshur et al., 2017).
WSI consists of two processes (Farahani et al., 2015; Webster and Dunstan, 2014): the first utilizes specialized hardware (scanner) to digitize glass slides generating a large representative digital image (i.e., “digital slide”); and the second employs specialized software (i.e., virtual slide viewer) to examine these digital slides on a workstation (i.e., computer with a monitor) (Figure 33.1). Both light (conventional) and digital microscopy present numerous opportunities for perceptual research.
Figure 33.1 A typical whole slide imaging workstation. On the left is the slide scanner and on the right the display monitor showing the scanned slide.
When making a visual diagnosis pathologists need to: (1) recognize relevant pathologic features (e.g., found by screening) from irrelevant findings (e.g., background distractors), and then (2) render an accurate diagnosis that relies upon their visual expertise, attention, knowledge, access to an expert/consensus-developed reference, and experience (Bussolati, 2006; Carney et al., 2016; King, 1967). Experience alone is often insufficient for trainees to become experts at interpreting and diagnosing pathology images. Although pathology is often considered the gold standard in terms of diagnosis via other modalities and techniques (e.g., radiology), errors can and do occur (Harrison et al., 2017; Nakhleh et al., 2016; Perkins, 2016; Woolgar et al., 2014).
Making a diagnosis is the epitome of a problem-solving strategy, and Pena and Andrade-Filho (2009) proposed a model that encompasses four domains of the pathologic diagnostic process: (1) cognitive (which is guided in part by perception); (2) communicative (e.g., report creation); (3) normative (e.g., empirical knowledge); and (4) medical conduct (e.g., consequences of a diagnosis in the management of a case). The cognitive domain involves more than just perceptual processes, as it also entails attention, memory, search, pattern recognition, hypothesis creation, and verification. When pathologists examine a case (e.g., unfixed biopsy specimen, glass slide tissue section, whole slide digital image) they seek specific informational cues (e.g., growth pattern, cellularity, necrosis) to formulate a diagnosis. They may need to collect more information (e.g., perform special stains or other ancillary tests), looking for further familiar diagnostic cues. With this additional information the pathologist confirms the final case diagnosis. With experience, pathologists learn to fine-tune and improve their diagnostic skills.
Modern healthcare depends greatly on the perception and interpretation of medical images (Beam et al., 2006). In pathology, technological advances since 1998 have introduced WSI scanners that are capable of digitizing (scanning) entire glass slides to generate high-resolution digital (virtual) slides. WSI has ushered in a plethora of novel clinical, education, and research applications in pathology. This includes the ability to use virtual microscopy to study (e.g., track eye movements or mouse actions) pathologists’ navigation precision and efficiency, interpretative process, and diagnostic ability (Roa-Pena et al., 2010). For example, tracking pathologists using WSI allowed researchers to conclude that individuals who spend more time fixating on relevant regions of interest (ROIs) tend to demonstrate higher diagnostic accuracy (Brunyé et al., 2017).
This chapter covers the role of perception in pathology and deals with specific tasks such as screening and interpretation, the influence of physical variables such as color and image quality, as well as the impact of distractors in the diagnostic process. The role of technology in perception issues related to pathology, including eye tracking, digital imaging, and augmented/virtual reality, is also addressed.
33.2 Viewing and Search Tasks
How does a pathologist make a diagnosis? To quote Bussolati (2006), there are four steps involved: “(1) to look, (2) to see, (3) to recognize and (4) to understand.” In this context to look is related to the largely unconscious visual search component that is responsible for slide exploration. To see and recognize are perceptual components that gather and process relevant information, and to understand represents the cognitive component that assembles all the available information into a diagnostic decision. In this framework we can predict that when pathologists look at a slide and know what the diagnosis is, their exploration strategy should be economical and focused, as they “look,” “see,” and “recognize” the relevant pathologic findings for the disease process that is present. On the other hand, when the diagnosis eludes them, search should be effortful and information pursued in detail at different magnification levels. In the former case, it can be said that the pathologist engaged in “pattern recognition” (where the disease process is recognized almost instantly, based upon prior examples seen); whereas in the latter case, two possible strategies could be used to explain the pathologist’s behavior: (1) multiple branching or arborization, which is a reduction of the diagnostic process from a large number of possible alternatives; or (2) exhaustive search, that involves the collection of all possible data before generation of a diagnostic hypothesis (Pena and Andrade-Filho, 2009).
Do pathologists consciously decide whether they are going to engage in pattern recognition or in exhaustive search upon viewing a new case? This is an interesting question that, to our knowledge, has not been directly explored in the literature. Models of medical image perception and interpretation (see, for example, Nodine and Kundel, 1987) propose that upon image presentation, the pathologist acquires a general idea or gist of the image (see Chapter 12 for a general discussion of gist). If this general idea fits a pre-established schema held in memory from previous experiences, the pathologist will likely engage in pattern recognition, namely, he/she will search for specific areas of the slide and arrive at a diagnostic conclusion in most cases without visually inspecting most of the slide. On the other hand, if no immediate recognition of the disease process occurs, the pathologist will engage in a more thorough search of the image, and in this situation arborization or exhaustive strategies may be employed.
Another interesting question is whether these different reasoning strategies are related to the task the pathologist is carrying out, namely, whether the specimen being examined is a screening case or a diagnostic case. Again, to our knowledge no studies have been carried out to examine this question directly, but the process of screening (e.g., a Pap test slide) seems to lend itself naturally to an elaborate search strategy; whereas the diagnostic process (e.g., determining whether a specimen has lung cancer, what type and stage it is) may or may not engage pattern recognition in the same way. This would depend on the abnormal signs of the disease (e.g., size and shape of cell nuclei, cell arrangement) being present in specific locations, and whether they can be disembedded from the rest of the tissue material at slide locations where information is contained, in such a way that the brain can match the current information with what is stored in memory. If disease is spread out or no immediate signs of disease are detected, arborization or exhaustive search will likely be used.
As indicated by Roa-Pena et al. (2010), navigation of a pathology specimen involves two strategies: scanning and examination at various magnifications. With the first look at an image, the pathologist acquires a gist of the image properties (e.g., type of stain, whether any obvious disease process is present), followed by selection of ROIs for further examination using various (low, medium, high) magnification. In general, much of the literature suggests that ROI selection is based upon a single criterion (i.e., areas that contain diagnostic information), but Roa-Pena et al. (2010) suggest that the time spent analyzing ROIs and the areas that attract the attention of multiple pathologists should also be added to the definition of what constitute ROIs.
Clearly, the selection of ROIs required to adequately examine slides is critical in diagnostic decision making. Recently, efforts have been devoted to determine diagnostic ROIs automatically using deep learning and other computer-based techniques in a variety of different tissue types. For example, Gutierrez et al. (2011) used a supervised model inspired by the human visual system that replicated the activities carried out by the human visual system in the first 100 ms of exposure to the slide, simulating the work of visual cortex areas V1, V2, and V4. They compared their model with two popular models in the computer vision literature, and found higher sensitivity and specificity, particularly at higher magnifications (×20, ×40).
On the other hand, Romo et al. (2011) used a training scheme with ROIs that had been selected by pathologists as being diagnostically important and those that had not been selected. In this case the algorithm was evaluated by calculating its precision and recall, where precision was defined as the percentage of estimated areas identified as relevant, and recall as the percentage of relevant areas that were correctly estimated. This algorithm performed better for identification of diagnostically relevant ROIs than a random algorithm and one that is commonly employed in the computer vision literature. Even so, the average precision of the algorithm was only 0.55, with the average recall being 0.38.
A different approach was taken by Mercan et al. (2016), using bag-of-words to create “visual words” and hence a dictionary for automatic ROI selection. These investigators obtained a classification accuracy of 74% when the dictionary was comprised of 40 “visual words,” but noted that reducing the dictionary to 30 words came at a steep cost, with accuracy dropping to 46%. The advantage of this type of algorithm is that it allows for insights into the pathologists’ cognitive processes. For example, the authors reported that the main difference between the dictionary with 30 words from the one with 40 words was the description of epithelial cells, but it turned out that these descriptions are critical in the pathologists’ task of finding diagnostically relevant areas in breast specimens. Thus, the authors were able to gain insight into the importance of epithelial cells in this task.
An interesting question in the definition of ROIs is the degree of agreement among pathologists that a given area contains relevant diagnostic information. Roa-Pena et al. (2010) found that, while the four pathologists in their study tended to cover only 66% of the tissue present, they agreed on average in 70.5% of the areas covered. This agreement was dissimilar for different types of slides. For example, in a slide containing endomyometrium tissue, pathologists only covered 48%, but agreed in 97% of the areas that attracted visual attention.
This is in agreement with Pantanowitz et al. (2012), who showed that cytopathologists tended to cover significantly smaller amounts of cellular area on a slide at higher magnification levels (average 65.1% at low, 33% at medium, 2% at high magnification), but agreement in the areas covered tended to increase with magnification level (average 77.6% for low, 67.7% for medium, 96.5% for high magnification). This is significant because it shows that, for the cases evaluated in both studies, diagnostic information can be found in specific areas, and pathologists concur to a high degree about where those areas are located.
Using breast specimens, Nagarkar et al. (2016) studied the importance of agreement among ROIs identified by pathologists and the ultimate concordance of their diagnoses. They created a test set for which an independent group of three pathologists marked consensus ROIs important for diagnosis. A different group of pathologists was then asked to mark diagnostically significant ROIs on these cases. It was found that, when percent overlaps between the marked ROIs and the consensus ROIs were classified into five ordinal categories, for each incremental change in percent overlap diagnostic agreement increased by 60%. Perhaps more than any other study, this suggests that there are areas that contain diagnostic information that pathologists can agree upon. This implies that, in some cases, exhaustive search is not necessary because relevant diagnostic information may often be restricted to certain identifiable ROIs. This raises the possibility of using computer-based analysis and classification tools to find and identify these areas, thus reducing the pathologists’ workload and diagnostic uncertainty.
33.3 Role of Color in Pathology
When conducting perception and observer performance research that involves color, it is important to have at least a basic understanding of color perception from the perspective of basic physiology (Oyster, 1999). The retina contains rods and cones which are light-sensitive cells that receive light photons, undergo a chemical transformation, and transmit electrical energy that acts on nerve cells that then transmit signals to the visual cortex and related brain systems. Rods, about 115 million of them, are mostly in the retina periphery, and are responsible for lower resolution and motion perception, and peripheral vision. Being motion detectors, their temporal response is faster than cones, allowing for rapid detection and orientation of the eyes to changes in scenes.
Cones (about 6.5 million) are located in the central or foveal region of the retina and are responsible for fine, high-resolution detail perception. Essentially this corresponds to the direction of gaze or attentional focus. Of importance here is that cones are responsible for color perception. There are three types of cones, each sensitive to a distinct range of wavelengths: S, M, and L, sensitive to short (blue), medium (green), and long (red) wavelengths, respectively.
Two further key aspects of color need to be considered that are important not only for the pathologist viewing images (via light and/or digital microscopy), but also for the development and implementation of automated image processing and analysis tools for use with WSI (Badano et al., 2015; Webster and Dunstan, 2014). The first is color accuracy or a system’s ability to produce exact color matches between input and output (Shrestha and Hulsken, 2014). The second is color consistency (Bautista et al., 2014) or a system’s ability to provide data that are identical or at least very similar to the color perceptual response of the human visual system. The latter is more difficult since color perception is a complicated issue.
One popular option is to use the International Color Consortium (ICC) device profiles that provide a standardized architecture, profile format, and data structure for color management and data interchange between different imaging devices. These profiles incorporate characterization data for color-imaging devices, plus data tags and metadata that detail transforms between native color spaces of a device and a device-independent color space. Computers use these color management software tools to provide consistent and perceptually meaningful color reproduction for input devices, output devices, and color image files.
It is important to note that there is significant variability in the color of pathology images at almost every stage of the imaging process, from glass slide preparation and staining, imaging (i.e., image acquisition and processing), and transmission to the display device (Badano et al., 2015; Clarke and Treanor, 2017; Revie et al., 2014). Color variation may even occur before imaging (e.g., tissue procurement, fixation, processing, sectioning, staining) that is often highly operator- (or laboratory instrument-) dependent. With WSI, additional variations ensue during slide scanning (digitization) and after imaging (display) that are addressable using color calibration techniques (see Badano et al. (2015) for a thorough summary and recommendations).
Methods to standardize color have been proposed for WSI acquisition and display, but in general they have not been methodically validated or evaluated with respect to their impact on diagnostic interpretation performance (Silverstein et al., 2012). Yagi, for example, has been developing a variety of techniques for color validation and optimization for a number of years. One such technique employed by Yagi and her collaborators takes two standard slides that are scanned and displayed by a given imaging system (Bautista et al., 2014; Yagi, 2011); one slide is embedded with nine filters with colors purposely selected for hematoxylin and eosin (H&E)-stained WSIs, and the other is an H&E-stained mouse embryo. Displayed images are then compared to a standard to identify any inaccurate display of color.
Displays and possible color characterization tools have also been investigated (Cheng et al., 2012; Kimpe et al., 2014; Saha et al., 2010). For example, Kimpe et al. (2014) examined whether WSI appear different on diverse display systems, as well as whether differences result in variances of perceived contrast of clinically relevant features. They used a set of four WSI and displayed them on three display systems. The visibility data revealed that images do look different on different display systems and if they are located in areas that contain clinically relevant features. In particular, the results also showed that perceived contrast of clinically relevant features was influenced by the display system. The authors also examined calibration methods and found that calibrating to the Digital Imaging and Communications in Medicine grayscale standard display function (DICOM GSDF) gave slightly worse results than standard red, green, blue (sRGB), and that a new method color standard display function (CSDF) performed better than both DICOM GSDF and sRGB.
The transition to viewing WSI on computer displays raises a number of important perceptual and ergonomic questions with respect to interpretation efficiency and accuracy (Krupinski, 2010; Pantanowitz et al., 2011; Weinstein et al., 2012). Although there are a number of studies considering color and display quality, there are very few on whether it impacts diagnostic performance. One study (Krupinski et al., 2012) used a calibration, characterization, and profiling protocol to assess just this question. Physical characterization of displays calibrated with and without the protocol revealed high color reproduction accuracy with the protocol. The observer study used a set of 250 breast biopsy WSI ROIs (half malignant, half benign) and showed them to six pathologists, once using the calibration protocol and another using the same display in its “native” off-the-shelf uncalibrated state. Receiver operating characteristic area under the curve showed that calibrated performance was 0.8570 and uncalibrated was 0.8488 (p = 0.4112). However, in terms of interpretation speed, mean time to render a decision with the calibrated display was 4.895 seconds and uncalibrated 6.304 seconds (p = 0.0460). This suggested that there was a slight advantage diagnostically when using a properly calibrated and color-managed display as well as a significant potential advantage in terms of improved workflow. Clearly much more work needs to be done regarding color in pathology interpretation.
33.4 Resolution and Magnification
With microscopic pathology, image resolution (quality), magnification (digital zooming), and focus (depth perception) can all influence visualizing pathology features in specimens (Bashshur et al., 2017). When looking at an object (e.g., cells or tissue on a glass slide) through a light microscope, the pathologist sees a “virtual” image of that object, perceived to be some distance away in the microscope’s observation tube. With the adoption of digital pathology, it is important to be aware that the appearance of an image on a digital display can be different from the traditional light microscope view. In particular, the perceived size and quality of pathologic findings may vary in digital slides on computer monitors (Sellaro et al., 2013). There are several reasons for this, related to various parameters of WSI, including: (1) the objective lens’ magnification and numerical aperture used by the microscope in a WSI scanner; (2) resolution (size and number of pixels) of the digital camera’s sensor; and (3) resolution (size and amount of pixels) of the monitor used to view the image.
The numerical aperture of the microscope objective is more important for image quality than magnification. Numerical aperture is the measure of an objective to resolve fine specimen detail. Higher values of numerical aperture produce a more highly resolved image and allow smaller structures to be visualized with greater clarity. Magnification, on the other hand, changes the apparent size of an object. Increasing the magnification often makes it easier to see certain objects. However, increased magnification need not simultaneously increase resolution. Using oil immersion between an objective and the slide coverslip increases the optical resolving power of a microscope. For this reason high-magnification, oil immersion digital pathology platforms have been shown to be better suited for microbiology applications and yield interpretations on par with glass slide evaluations when looking for microorganisms (Rhoads et al., 2016). With WSI, greater zooming behavior has been shown to predict a tendency to overinterpret certain cases (Brunyé et al., 2017). The magnification of an image on a computer screen is not only influenced by the scanner (image acquisition), but also by the workstation (display) and how far the pathologist viewing the image is sitting from the screen. In general, the further away the pathologist sits, the smaller the image will appear.
As more pathologists adopt digital pathology, it is important to appreciate that traditional glass microscope image quality monikers may no longer apply (e.g., microns/pixel instead of scan magnification). Also, better understanding is needed to appreciate what, if any, impact image resolution and magnification have on a pathologist’s viewing experience and workflow.
33.5 Image Compression
By their very nature, virtual slides are very large images. As Kalinski et al. (2009) determined, one Z (focal)-layer of a virtual three-dimensional (3D) slide (i.e., a virtual 2D slide) with an original specimen size of 0.5 × 0.5 cm and digitized at a resolution of 0.23 nm per pixel would generate an image sized 1.4 Gb, or 12.6 Gb for the entire 3D slide if it was scanned with nine focal planes. Thus, data compression is a necessity in virtual microscopy, as storage of such large images is expensive and transmission can be costly and slow unless their file size is reduced.
One major issue with image compression in virtual microscopy is that it is impossible to determine a priori level of compression that fits the need of all clinical applications (Krupinski, 2009). Similarly, there is debate over the use of lossy versus lossless compression, where the latter produces larger images but offers no loss of potential diagnostic information, and the former produces smaller images, but depending on the choices made at the time of algorithm application, may lose some diagnostic information.
Kalinski et al. (2011) argue that lossless compression (using JPEG2000) is not required for diagnostic virtual microscopy. They arrived at this conclusion by having three pathologists blindly examine gastric biopsy slides that were either compressed in a lossless (1:1) or lossy manner (with rates from 5:1 to 20:1). The slides may or may not have contained Helicobacter pylori gastritis. As the authors found no differences in performance between lossy and lossless slides, they concluded that lossless compression is unnecessary. Since this study only evaluated one type of tissue and one type of finding (H. pylori), it is difficult to infer whether these results can be generalized to all tissue types and findings.
Kalinski et al. (2009) went a step further and sought to determine, in this same task, how much lossy compression could be applied without a corresponding loss in diagnostic image quality. They found that image compression of up to 75:1 produced images that were still of acceptable levels, but that at 200:1 blurry artifacts could be seen at high magnifications, which would be unacceptable to pathologists.
Krupinski et al. (2012) examined the use of model observers in the evaluation of compression of ROI in breast slides. They used JPEG2000 compression (8:1 to 128:1) and found a significant decrease in performance as the compression ratio increased. When evaluating the performance of human observers with compressed slides, they found that pathologists could differentiate benign from malignant entities at an almost constant rate (measured by area under the receiver operating characteristic curve) up to a compression level of 32:1, but further compression significantly degraded performance. It is important to note that, while image compression may not hinder visual interpretation by a pathologist (human), loss of data that occurs with image compression can markedly influence the data output of image analysis performed by a computer algorithm (Pantanowitz et al., 2017).
Konsti et al. (2012) evaluated the effects of image compression and scaling on the performance of automated computer algorithms that analyzed immunohistochemical stains and automated tumor segmentation. Using two tissue microarray slides containing samples of breast cancer and two containing samples of colon cancer, the authors reported a good agreement between the algorithms’ results with lossless compression and lossy compression of ratios up to 1:25. As these studies show, lossy compression is viable in digital pathology, but the appropriate compression ratio is task-dependent, as no one-size-fits-all solution has yet been established.
33.6 Distractors
Pathologists may become distracted by overt or subtle stimuli within their field of vision or surrounding environment. Distractors could affect perception and thereby divert pathologists from reaching a correct diagnosis. With microscopy, the viewing field of a light microscope is typically considered to be large enough to minimize interference from peripheral visual stimuli. In contrast, the visual angle created by virtual microscopy (viewing WSI on a monitor) is believed to make pathologists more vulnerable to visual interference. Employing a variant of a visual distraction paradigm (the Eriksen flanker task), one group of investigators tested this by determining whether the accuracy and speed of interpreting cytology images on a central target screen were affected by images and data displayed on neighboring monitors (Evered et al., 2016). They found no effect of visual distraction on diagnostic accuracy by peripheral distractors.
With “busy” fields of view pathologists may have difficulty finding rare diagnostic cells. This phenomenon is believed to be exacerbated using WSI. In one study where WSIs were used for teleconsultation, WSI evaluation misclassified a mixed-cellularity Hodgkin lymphoma case compared to glass slide evaluation (Wilbur et al., 2009). The authors aptly point out that inflammatory conditions, particularly those requiring meticulous searching at high magnification, may be more difficult to interpret in WSI format.
Some features (e.g., contrast, color) may cause greater distractions than others. For example, when investigators intentionally manipulated digital images of Pap test slides using Photoshop (e.g., adjusted brightness, contrast, red–green–blue color, and luminosity), this significantly affected diagnostic interpretation (Pinco et al., 2009). The authors accordingly suggested that care needs to be taken when digital images are used in pathology, to specifically ensure that their digital alteration does not affect diagnosis.
33.7 Digital vs. Glass Slide Pathology
There have been numerous studies comparing diagnostic accuracy and/or concordance of glass slides (optical modality) examined under a light (conventional) microscope versus WSI (digital modality). A recent review by Bashshur et al. (2017) summarized the bulk of literature on this topic, as do a number of other reviews (Farahani et al., 2015; Meyer and Pare, 2015; Webster and Dunstan, 2014). This chapter highlights a few exemplar studies to illustrate how such investigations have been conducted.
Hufnagl et al. (2016) compared diagnostic accuracy between telepathology (using a remote robotic microscope) and conventional frozen-section interpretation for 81 routine breast surgery specimens. They found that diagnostic concordance was 95.1% overall, with 2 cases in each mode that resulted in uncertain diagnoses. They also recorded interpretation time and found that it took an average of 9 minutes with conventional and 17 minutes with telepathology, highlighting the challenges associated with digital viewing, especially using remote robotic systems.
Campbell et al. (2014) compared WSI and light microscopy for breast needle biopsies, examining the effects of image capture magnification (×20 vs. ×40) and monitor type on accuracy: standard, noncalibrated desktop monitor (17-inch, 1.3-megapixel (MP) flat screen) vs. a DICOM-compliant, diagnostic-grade, color-calibrated monitor (30-inch, 4-MP flat screen). Four pathologists reviewed 85 breast biopsies (92 parts, 786 slides). Complete concordance between the two modes of viewing was reached in 31% of cases. There were 3% discordant opinions involving 9 cases. The remaining 66% were concordant with clinically insignificant differences, and when the two concordant categories were grouped together, overall concordance rates were 97.1%.
Overall, the bulk of the published evidence assessing the impact of using WSI for interpretation indicates that diagnostic concordance for glass vs. digital reads was observed in more than 90% of cases in most studies. However, agreement tends to be higher (i.e., diagnostic concordance) for higher-grade cancers and cases where there is overall less ambiguity. Moreover, concordance varies by type of pathology. The type of display used can also impact performance, but potentially more in terms of efficiency rather than efficacy. Of note, inter- and intra-reader variability exists with both conventional and WSI viewing. Reported difficulties have occurred due to limitations in visualizing nuclear detail of cells, mitotic figures, microorganisms, and complex cytopathology. When WSI cases were deferred to light microscopy in these studies, reasons typically included the need for additional clinical information and poor-quality images.
33.8 Influence of Expertise: Diagnostic Accuracy and Visual Search Strategies
The nature of expertise (see Chapter 11), the importance of training (see Chapter 31), and performance evaluation (see Chapters 22 and 23) have all been described in detail in other chapters, so we refer readers to them for additional background. Here, we highlight a few studies concerning the nature and development of expertise in pathology, especially as they pertain to WSI. Compared to radiology, there have been relatively few eye position recording studies in pathology, although the results to date are very similar in both areas. This is not surprising as, in both radiology and pathology, diagnostic decisions are rendered based on search and visual examination of image data. The images in both fields are generally quite complex, making it necessary to scan images with high-resolution foveal vision after an initial gist impression of the image content.
A critical part of clinical reasoning in pathology is based upon acquisition and processing of visual information. In Bussolati’s (2006) model of diagnostic processing, the first two components pertain to this, namely, “to look” and “to see.” Eye tracking allows for monitoring of pathologists’ visual attention, which can lead to insights into their cognitive processing of an image (Brunyé et al., 2017). Although this technology has been used widely in radiology, it has only recently been used in pathology, perhaps because of the many difficulties associated with tracking the eyes in a 3D environment (Venjakob and Mello-Thoms, 2016). Perhaps because of this, many eye position recording studies in pathology do not allow observers to zoom or pan, and thus depart significantly from clinical practice. Nonetheless, these studies have yielded valuable insights into the nature of the decision-making processes used by pathologists and trainees when reading digital slides.
For example, in one of the earliest studies, Tiersma et al. (2003) suggested that diagnoses based on images tend to lead to a high degree of interobserver variation. According to these authors,
inter-observer variation may arise at three levels, which are not only influenced by previous knowledge and experience, but also depend on applied diagnostic criteria. The first level concerns the visual search of the image, the second level involves the interpretation of the perceived visual information, and finally, the third level concerns the way the collected information is combined to reach a diagnosis (Tiersma et al., 2003).
In this context, eye position tracking allows for an investigation of the differences at the first level of Tiersma et al.’s model. Using eye tracking it has been found that two types of search strategies could be observed when pathologists studied cervical intraepithelial neoplasia: (1) a “scanning” strategy where many areas of the slide were fixated on for a brief amount of time; and (2) a “selective” strategy in which only a few selected areas attracted visual attention for prolonged periods of time. This is remarkably similar to the “scanning and drilling” strategies reported by Drew et al. (2013) of radiologists reading volumetric images.
To determine if these strategies also applied to pathology, Mercan et al. (2017) carried out a study in which 87 pathologists examined 60 digital breast slides. They confirmed the use of scanning and drilling, where scanning “involves maintaining a particular zoom level while searching relatively broad regions of interest,” and drilling involves “restricting a search to a region of interest and zooming in to high magnification levels” (Mercan et al., 2017). They found that pathologists engaged in both strategies. In particular, they tended to use more scanning when reading the initial cases and progressively moved to more drilling as they became comfortable with the interface.
The authors hypothesized that scanning could potentially be a less efficient and effective search strategy because it requires a higher cognitive load than drilling. Pathologists using scanning had to remember larger portions of the slide that they were panning, whereas those using drilling only had to recall the areas that had been “drilled” at high magnification, which were likely to be areas of saliency. However, the authors cautioned that this study was not designed to measure the cognitive load of each strategy, and hence such hypotheses should be taken with caution. They found no differences in diagnostic accuracy of pathologists who used scanning versus those who used drilling.
Another groundbreaking early study was by Krupinski et al. (2006). This study presented breast WSIs to pathologists and pathology trainees, and asked them where they would zoom if they could zoom in on only three locations. Eye position was recorded (Figures 33.2 and 33.3), and the results showed that pathologists were not only faster at viewing these breast images, but that their saccades (jumps between locations of fixations) were also significantly longer than residents’. Interestingly, pathologists did not always choose to magnify an area where they had previously fixated, suggesting a larger visual span of the image.
Figure 33.2 Observer searching digitally displayed whole slide imaging as eye position being recorded.
Figure 33.3 Typical eye position pattern of a senior-level resident searching a digitally displayed whole slide imaging. Each circle represents a fixation, with the size reflecting dwell time (larger = longer dwell) and the lines representing saccades.
A few years later Krupinski et al. (2013) published a follow-up study where they monitored the development of pathology expertise by eye tracking using the same group of pathology residents at the beginning of the year for each of their four residency years. The study showed that trainees’ search patterns progressed from resembling those of medical students (in year 1) to being more closely aligned with those of expert pathologists (in year 4). In addition, search times decreased, in conjunction with a decrease in the time spent on the areas deemed to be diagnostically significant, and saccades became more purposeful. The percent of locations chosen that were not diagnostically relevant was reduced from about 4% in year 1 to 0% in year 4. As pointed out by the authors, as residents develop expertise they seem to be able to quickly acquire relevant information about the image faster with each passing year, and the ROIs that they fixate on are more likely to contain truly diagnostic information.
Brunye et al. (2014) also investigated the relationship between eye movements and visual expertise. In a clever setup they used a saliency algorithm to designate the most salient area in a digital breast slide, and asked an expert pathologist to pick another relevant diagnostic area in the slide such that the two areas did not overlap. They then had novice, intermediate, and expert pathologists visually scan the slides while their eye movements were recorded.
The authors reported that novices tended to be guided by bottom-up saliency, fixating initially in the salient areas picked up by the algorithm, instead of the diagnostic areas. Experts, on the other hand, rarely fixated on the salient areas, suggesting a top-down control of fixations probably due to cognitive schema held in memory. These cognitive schema filter out irrelevant information from the image and guide the experts’ attention to relevant diagnostic areas of the slide. Novices, on the other hand, do not have such schema formed yet, and tend to be guided by salient features in the image. Often such features are not diagnostically relevant, and this may cause difficulties in their diagnostic processes.
Nodine and Mello-Thoms (2010) proposed that experts “detect-then-search,” namely, they capture diagnostically relevant information from the image through the use of cognitive schema, then search for information to confirm or dismiss the diagnostic hypotheses formed. In contrast, novices “search-then-detect,” that is, they absorb large quantities of information from the image before the formulation of diagnostic hypotheses. Most of this information is not diagnostically relevant, and it may lead to enlargement of the diagnostic tree, which may become unruly and difficult to prune into a correct final diagnosis (or differential).
Pathologists’ experience level is a critical factor in diagnosing less common diseases, such as atypia and ductal carcinoma in situ, relative to benign and invasive breast cancer (Brunyé et al., 2017). In one study, pathologists who spent their time fixating mostly within the diagnostically relevant areas of a slide (as opposed to looking around) tended to arrive more often at the correct diagnosis. Interestingly, these authors reported that pathologists were more likely to overcall a case with increased zooming behavior, and they linked this to findings that suggest that observers tend to make erroneous guesses the longer they examine an image (Christensen et al., 1981; Chun and Wolfe, 1996).
Since eye position tracking is so difficult to carry out, even when using virtual slides and digital microscopes, some researchers have investigated whether proxies could be used to determine where the pathologists’ visual attention was at any given point in time. Raghunath et al. (2012) correlated pathologists’ eye position with mouse cursor movements, and found that the cursor was a good predictor for position in the x direction, with a mean difference of 4 pixels; but in the y direction the mean difference tended to be larger, about 37.5 pixels. These authors hypothesized that pathologists may place the mouse cursor above or below the area where they are fixating but not exactly on it, in order not to interfere with the structural integrity of the scene by obscuring some elements.
In contrast, Molin et al. (2015) used a strategy already used in radiology and many other areas, called “thinking aloud” (Dunckner, 1945; Littelfair et al., 2012), and asked pathologists to think aloud while diagnosing slides, and recorded their navigation strategies through a digital microscope interface. All the pathologists in this study had significant experience with virtual slides. The authors found that there were basically six types of strategies employed in navigating the slides: three were related to panning, and the other three related to zooming. From these, three strategies were not possible using a conventional microscope, namely, panning in the thumbnail (which depicted a low-magnification view of the entire slide), and zooming in and dip-zooming in the main view. In this study zooming in was defined as “an increase in magnification, directed or undirected on a specific image feature,” and it occurred 20.7% of the time, whereas dip-zooming was “zooming-in on a specific image feature followed by zooming-out,” and it occurred 8.7% of the time (Molin et al., 2015).
Treanor et al. (2009) have also tracked trainees and expert pathologists as they examined virtual slides, by recording their navigation with a digital microscope interface. These authors reported that, while trainees made no search errors (i.e., they did not fail to inspect relevant areas containing diagnostic information), they failed to properly assemble the gathered information into an appropriate diagnosis in 39.5% of cases. In 8% of errors, there was also a failure to identify a diagnostic feature that was important for the correct interpretation of the case. These observations are in line with the findings of Crowley et al. (2003), who found that intermediates (defined as second- and third-year pathology residents) committed 3.6% errors of feature identification, and in 32% of the cases they failed to assign a proper meaning to the gathered information.
Finally, Mello-Thoms et al. (2012) examined search patterns of pathology residents and fellows when reading a case set of dermatopathology digital slides. By recording all actions taken on the viewport, they developed a static representation of the dynamic search strategy used by the pathologists, allowing for side-by-side comparison of slide exploration between different observers. They reported that statistically significant differences were found in the trainees’ slide exploration when the final diagnosis was correct versus when it was incorrect. In the former case, the search strategy was economical, with pathologists focusing on a small number of areas from low to high magnification, suggesting that they were engaging in pattern recognition. When the diagnosis was incorrect, pathologists tended to engage in an expensive search strategy in which the slide was explored thoroughly at different magnification levels. In this case, it is possible that pathologists were using either arborization or exhaustive search, and for the particular cases and observers used in this study, it tended to lead to incorrect decisions.
33.9 Emerging Technology
In digital pathology, image analysis is being increasingly used to extract meaningful information from images. This has introduced the promise of computer-aided diagnosis using either traditional image algorithms or deep machine learning with convolutional neural networks (Bhargava and Madabhushi, 2016). It is recognized that pathologist interpretation using glass or digital slides is limited by intra- and interobserver variability, as well as human limitations related to perception of dimension, size, and color.
Akin to the well-known Edward Adelson checker shadow illusions, investigators have shown that computers outperformed humans when assessing HER2 staining of tissues (Vandenberghe et al., 2017). This was related to perceptual differences in assessing HER2 expression due to high HER2 staining heterogeneity.
Compared to pathologists performing manual tasks (e.g., quantification), image analysis has been shown to yield better accuracy (precision) and standardization (more reproducible results), especially for intermediate categories and complex scoring systems (Stålhammar et al., 2016). Further studies are anticipated to better understand how machine vision differs from human perception, for the purpose of developing more efficient “perceptive machines” (Privitera and Stark, 1998).
Mixed reality technologies, including augmented reality and virtual reality, either supplement or completely replace the real world with computer-generated data to facilitate user interaction via one’s natural senses (Figure 33.4). These technologies (e.g., Oculus Rift, HoloLens) have begun to be explored for use in healthcare, primarily for medical education and simulation training. Such head-mounted devices have been tested in an attempt to overcome current complaints from users of WSI that navigation of digital slides is slow and tedious, especially with input devices such as a computer mouse (Farahani et al., 2016). Pathologists also complain that when viewing a digital slide on a computer monitor, they lose the benefit of peripheral vision they are used to having when looking at a glass slide with a conventional light microscope. Preliminary studies have shown that using the Oculus Rift to view and navigate pathology WSI in a virtual environment is feasible for diagnostic purposes. It also facilitates viewing and manipulation of 3D images. However, further technologic advances (e.g., improving image resolution, minimizing motion sickness-like side-effects) and larger clinical studies to validate these wearable tools are required.
Figure 33.4 Pathologist using the Oculus Rift virtual reality system to screen and interpret Papanicolaou test digital images.
33.10 Conclusion
Given that perception can significantly influence diagnostic accuracy and efficiency, it is important to recognize the factors that can potentially alter perception. Moreover, these influences need to be addressed when training pathologists, establishing environments in clinical practice to perform diagnostic work, and designing digital pathology systems.
For example, as noted by Bashshur et al. (2017), the up-and-down focusing of light microscopes during specimen examination is a tool during slide scanning and visualization. The original dynamic robotic telepathology systems used by pathologists offered complete robotic remote control of up-and-down focusing on histopathology slides. The autofocus feature of most newer WSI systems is an incomplete solution, as substituting a single optical plane representation of a histopathology slide provides only a partial solution. WSIs have a relatively shallow depth of focus with a 40× lens, meaning that potentially more than 80% of the volume of a tissue section might be excluded from viewing. While modern scanners with Z-scanning (multiplane) capability are being developed, more perceptual and ergonomic research is required.
As this chapter illustrates, there are numerous topics amenable to image perception research in pathology, especially in the context of WSI and telepathology. In some cases the issues are quite similar to those found in radiology, but other topics such as color, WSI display, image navigation, and depth of focus are quite unique to this specialty. The techniques available to researchers interested in these topics are varied (e.g., eye tracking, diagnostic concordance among modalities) and have been shown repeatedly in both pathology and radiology to reveal significant information about the perceptual and cognitive mechanisms underlying image interpretation. As we continue to develop and refine WSI technologies and its applications, improve ergonomics, and incorporate computer-based image analysis and deep learning techniques into WSI interpretation, perception research will continue to be relevant.
References





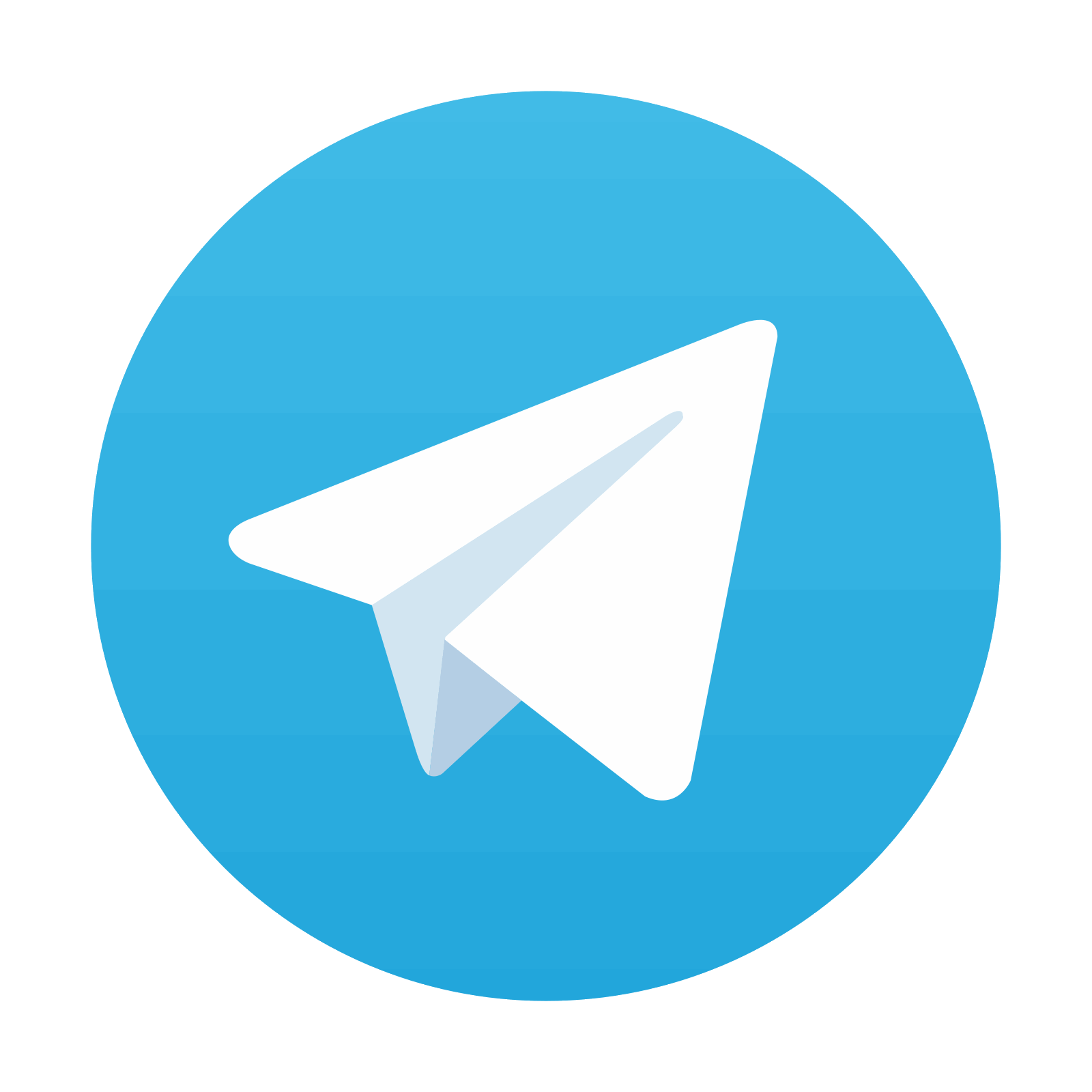
Stay updated, free articles. Join our Telegram channel

Full access? Get Clinical Tree
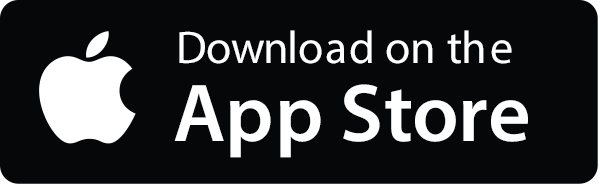

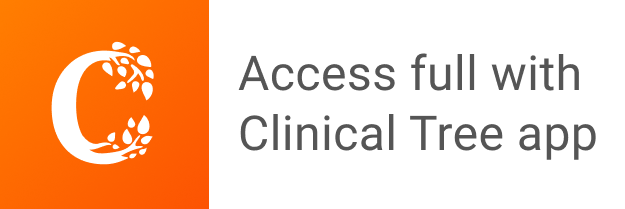