There are many technical and nontechnical steps involved in a successful clinical functional MRI (fMRI) scan. The output from scanning and analysis can only be as good as the input, so task instruction and rehearsal are the most important steps during an clinical fMRI procedure. Properly pre-processed data significantly affects statistical analysis, which has a great impact on image interpretation. Even though there is general agreement on how to process clinical fMRI data, such as algorithms for head motion detection and correction, the theory and practicalities associated with data processing remain complex and constantly evolving.
Key points
- •
Task instruction and rehearsal are the most important steps during an fMRI procedure.
- •
Data processing steps must be taken to optimize the signal that is associated with specific functional tasks and to minimize any noise-related signal.
- •
Pre-processed data significantly affects statistical analysis, which has a great impact on image interpretation.
- •
Algorithms for head motion detection and correction, the theory and practicalities associated with data processing remain complex and constantly evolving.
Introduction
Since its discovery in the early 1990s, functional MRI (fMRI) has been used to study brain function and to advance clinical care. , One well-established application of fMRI in the clinical setting is in neurosurgical mapping for patients with brain tumors near eloquent cortical areas. The main goal of neurosurgery is to maximize tumor resection while sparing important brain structures near the tumor, which relies on the proper identification of eloquent cortical areas. However, interestingly, there is considerable anatomic variation in the human brain between individuals, and the presence of a tumor can lead to cortical plasticity that recruits cerebral areas for tasks that are not originally associated with that region. As a result, the location of eloquent cortical areas adjacent to a tumor cannot be accurately defined by simply examining a structural brain image. In addition, one must be careful to acknowledge the existence of neurovascular uncoupling (NVU) that may also influence the blood-oxygen-level-dependent (BOLD) fMRI signal and can lead to false negative results (see separate article elsewhere in this issue). The goal of clinical fMRI is to preoperatively identify important functional areas associated with specific functional tasks critical in the patients’ daily life, such as hand movement and language function. , , For example, when a lesion presents in the vicinity of important areas like sensory motor or language areas, surgeons may request an fMRI scan to know how close the regions of activation are located near the lesion to best plan the surgery, including for intraoperative brain mapping or awake brain surgery ( Fig. 1 ).
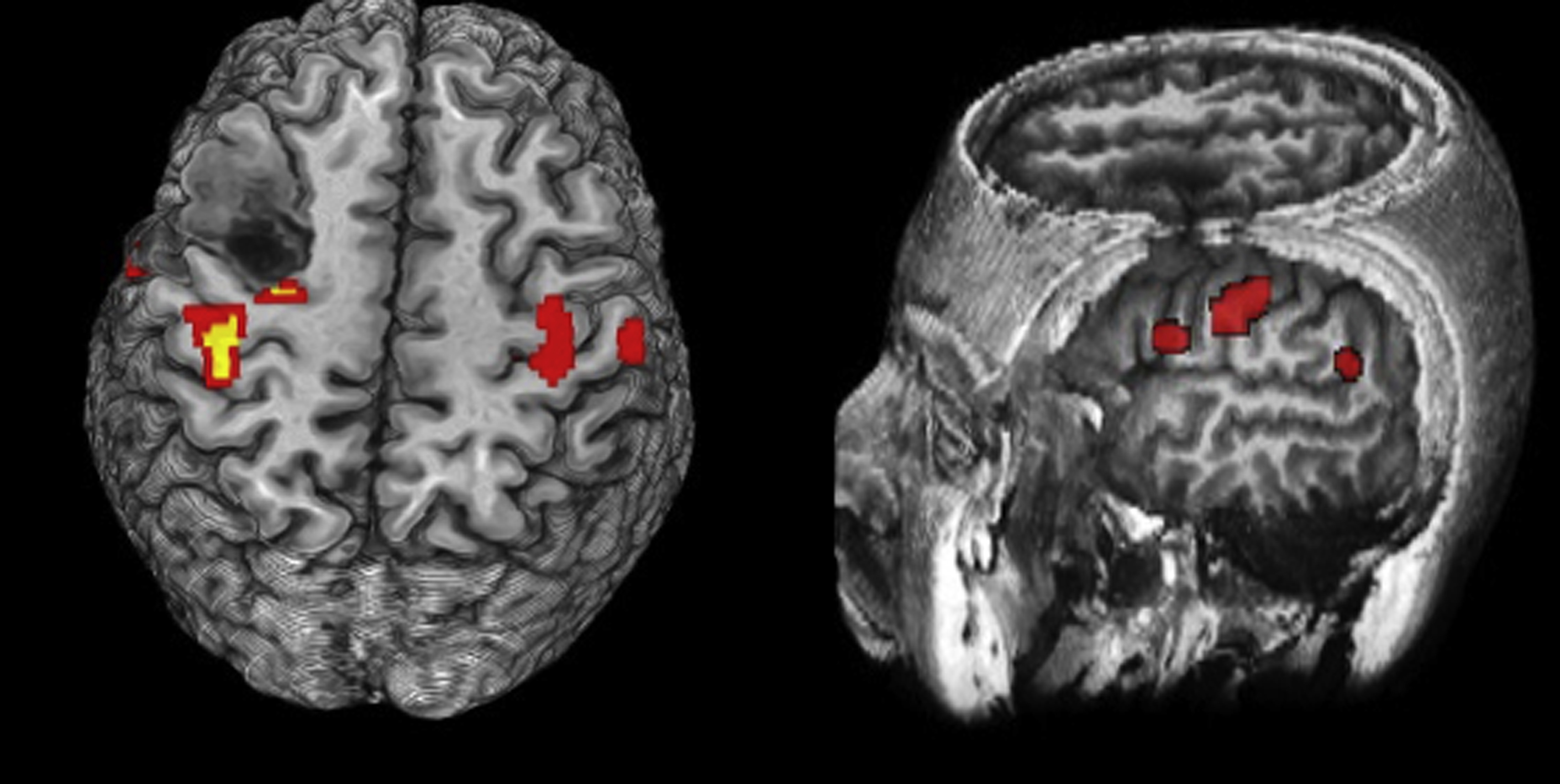
Workflow in clinical functional MRI
General Workflow
To obtain successful fMRI maps for presurgical planning, there are several important steps involved. The following diagram shows the typical workflow for a clinical fMRI procedure ( Fig. 2 ).
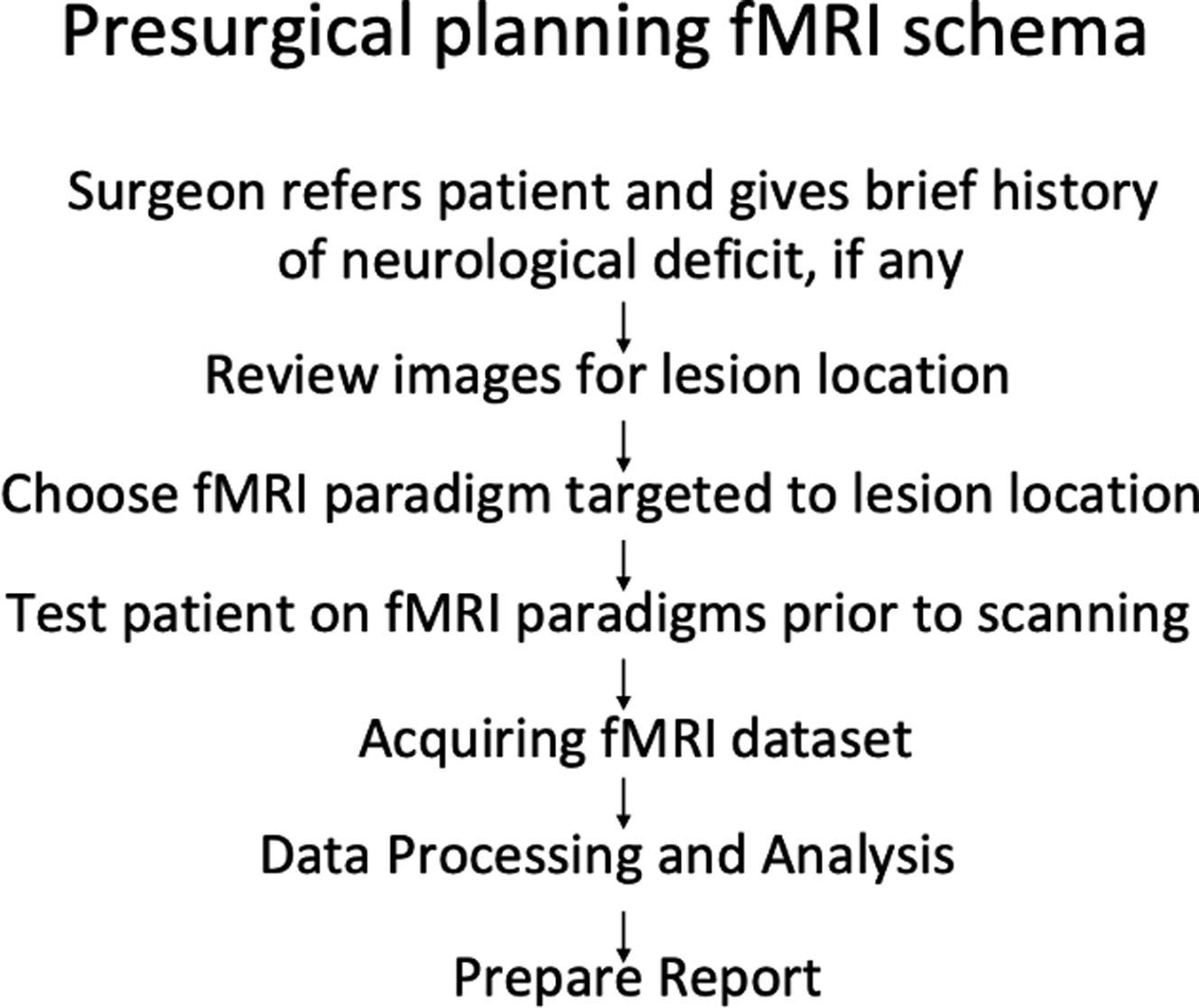
First, the neurosurgeon refers the patient to the fMRI team. The presence of any neurologic deficits should be noted to help guide the fMRI team with the lesion location. The fMRI team will also determine the lesion location by examining any previous images of the patients’ lesion, if available, and account for its potential growth or spread. Based on the lesion location, specific fMRI paradigms are prescribed to elucidate the nearby and/or affected cortical areas via task function.
On the day of the scan, the fMRI team begins by instructing the patient regarding the functional tasks that the patient will perform in the scanner. During the scan itself, the patient’s performance is monitored using the available real-time pre-processing software in the MRI suite. Current modern scanners incorporate some capability to perform t -test or Z-score analysis on a voxel-by-voxel level in real-time. This quick, real-time review of the fMRI activation will allow the fMRI team to have knowledge about the quality of the data acquisition, guiding the team to either continue with the scan or to re-conduct the scan. The completed fMRI and anatomic dataset can then be transferred to an off-line computer for pre-processing and statistical analysis. A neuroradiologist then reviews the functional activation maps generated, and a report is written for the neurosurgeon.
Task Instruction and Monitoring the Task Performance
The importance of following 2 procedures before any scanning is conducted cannot be overstated: task instruction and monitoring task performance . In clinical fMRI, the patient’s task instruction and rehearsal before the scan are of utmost importance and more critical than the image processing itself. The success of a fMRI scan also mainly depends on the subject’s task performance: even if you have the highest quality MRI scanner, you cannot generate a good fMRI scan if the subject’s task performance is poor. Task performance is especially important when the scan is used for presurgical planning because the patients may have neurologic deficits related to their lesion.
The subject’s task performance is also directly related to how well the subject understood the task instruction. Before a subject is scanned, a fMRI specialist instructs the subject about the functional tasks that will be performed in the scanner. During this procedure, the specialist should be able to gauge the subject’s performance level as they rehearse the task before going to the scanner. For example, hand or foot weakness can be evaluated when rehearsing motor tasks. If the subject shows very weak hand or foot motor strength, the fMRI specialist may need to make a decision to change the paradigm from a self-controlled task to an externally controlled stimulation for sensory motor task. For language function, several assessments including the Boston Naming Test can be performed to assess for any language deficits. If the subject has a language deficit, then it would be better to apply several different types of language paradigms because one language paradigm could be easier than another for the subject, and different paradigms may elicit different brain activations that can be interpreted together as a whole for general language function. Careful paradigm selection according to the patient’s condition, proper instruction, and practice of the paradigms prior to scanning also reduces difficulties with head motion artifacts and poor or inconsistent task performance. These nontechnical considerations during an fMRI scan can often be overlooked, but they remain vital for a successful clinical fMRI scan.
Image pre-processing
Purpose of Functional MRI Data Processing
After acquiring the fMRI data, fMRI data processing is conducted to maximize the signal and filter out the noise that is associated with the task performance ( Fig. 3 ). This process involves reducing the artifactual signal in the voxel time course that is, not part of the subject’s functional task performance. When this process is finished, there is improved detectability of statistically significant activation clusters that can be used to generate functional maps for presurgical scanning. fMRI data processing is generally conducted through several, essential steps including image quality assessment, head motion correction, spatial smoothing, and linear trend removal.
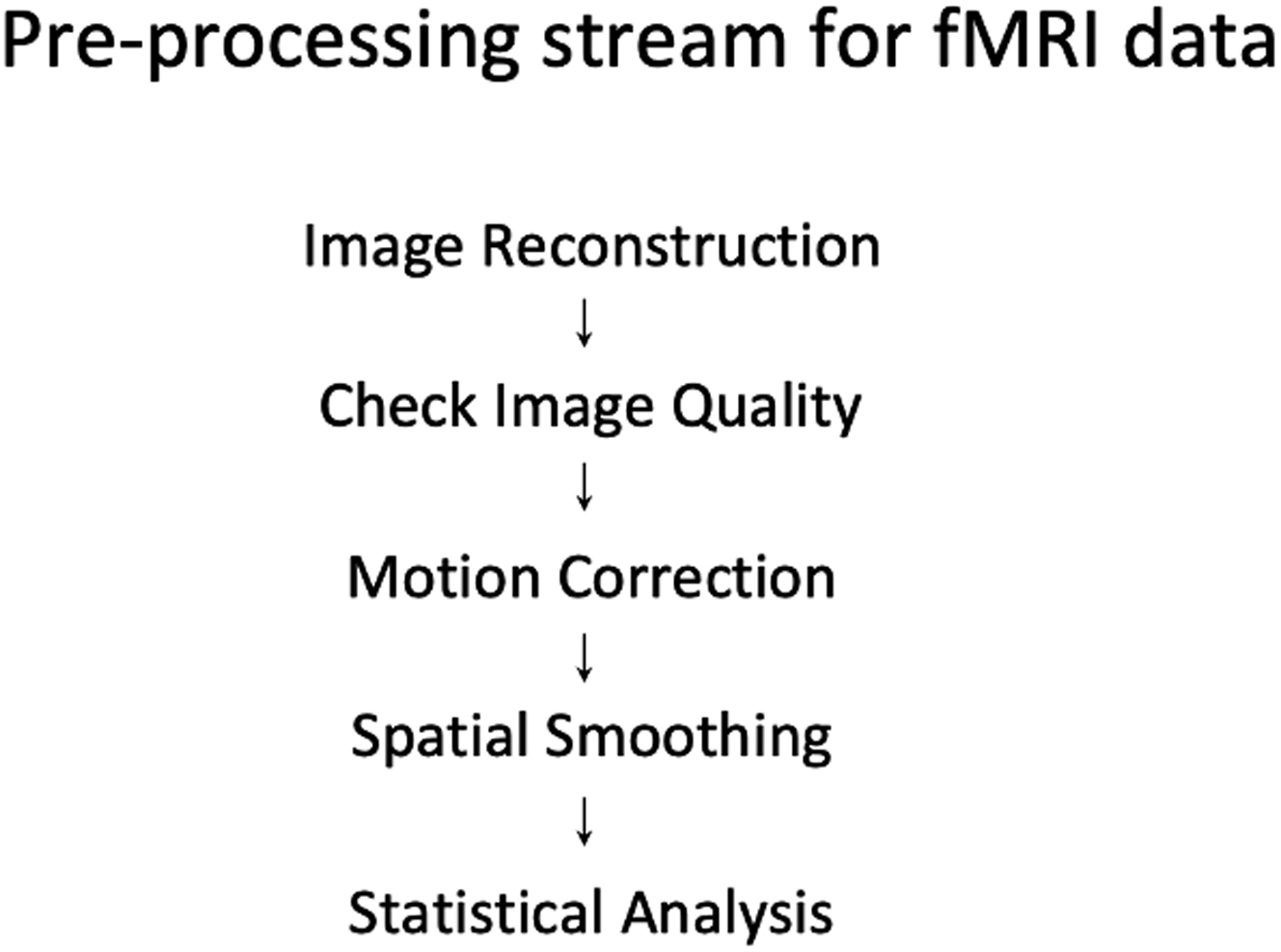
Normally, the fMRI data is processed using commercial or freely downloadable software (eg, FSL and AFNI , ). Commercial software is designed to be user-friendly and provides automatic or manual pre-processing tools. However, the fMRI data obtained from patients occasionally needs more complicated and patient-specific image processing steps that cannot be handled by the pre-determined automated processes found in commercial software. Therefore, additional advanced software may be used to tackle complicated issues including randomly-occurring artifactual images, severe head motion, and incorrect task performance.
Image Quality Assessment
After the raw fMRI data are transferred for data processing, it is essential to carefully review the images and time courses, especially the areas where activation is expected. Any sources of artifacts and noise-related abrupt signal change in the data should be identified and removed or minimized. Performing these image quality checks consistently throughout the pre-processing steps significantly improves the final images used for clinical interpretation.
Functional MRI Artifacts
The main artifacts in fMRI are caused by susceptibility, which are due to local magnetic field inhomogeneity. Common sources of these artifacts are at air-tissue interfaces such as the ear and nasal canals, which can cause signal loss in the auditory cortex and frontal lobe, respectively. There are other potential sources of susceptibility artifacts including the sphenoid sinus in the inferior frontal cortex and geometric distortion due to scanner Bo field inhomogeneity. There are also subject-related artifacts including those caused by the movement of brain parenchyma and the subject’s motion due to heart rate, respiratory rate, and bulk head motion. Cardiac pulsation artifacts can appear as large as a few percent signal change of BOLD contrast. Bulk head motion can cause voxels on the periphery of the brain to appear activated. Reviewing the images in “real-time mode” and checking temporal variations in the time series often allows for identifying problematic volumes or slices that should be removed before further processing steps ( Fig. 4 ).
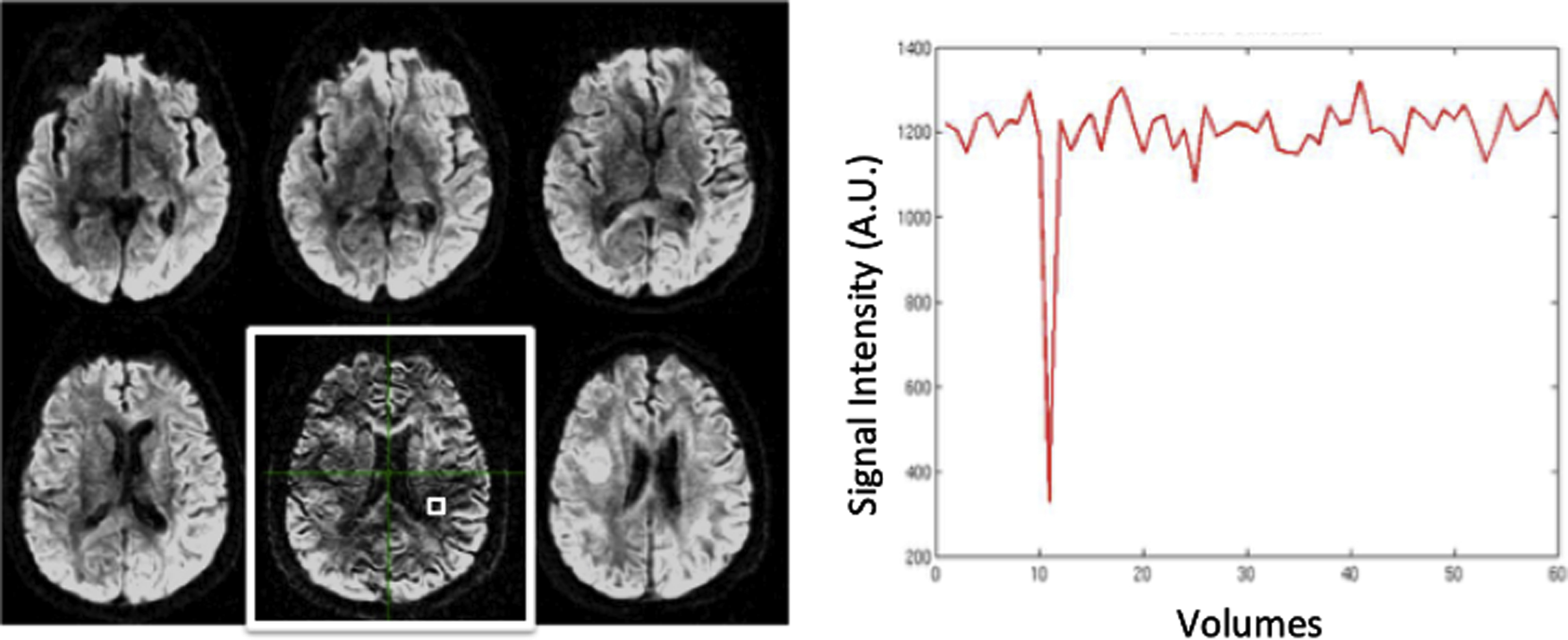
Head Motion
Motion is a major problem for fMRI studies, for it is the greatest cause of fMRI examination failure. In clinical fMRI, patients tend to show more frequent unintended head and body movements due to their underlying, neurologic condition than healthy control subjects. Motion is also more easily prevented than corrected. Therefore, patients must be specifically instructed how head motion can degrade the image quality and may lead to a repeated scan. In the scanner, ensuring maximal patient comfort can decrease the chance of motion. In addition, vocal feedback and encouragement right before each scan will help to minimize motion artifacts.
As task-related BOLD signal percentage changes are small (on the order of single-digit percentage changes) and because data analysis is based on signal variation in the voxel time series, even small head motion can heavily confound statistical analyses. Motion can occur during different paradigms and in different directions; leg motion for foot motor tasks can cause head movement in the inferior-to-superior direction, while the tongue motor task cause shifts in head placement.
Motion during fMRI can be of 2 kinds: randomly timed motion or stimulus-correlated motion . Randomly timed motion (that is not correlated with the stimulus task) can block real activity by increasing false negatives in the activation map. False negatives may increase when the random motion occurs during the baseline volumes where no task-induced activation should be present. On the other hand, stimulus-correlated motion creates false positives, as voxels of higher signal intensity can be shifted into surrounding areas of the activating region that are originally occupied by other voxels of lower signal intensity. If 2 neighboring voxels differ in intrinsic brightness by 15%, then a motion of 10% of a voxel dimension can result in a 1.5% signal change which is comparable to the BOLD signal percent change during stimulus activation at 1.5 T. Stimulus-correlated motion is particularly problematic because most software programs do not have a tool to separate true activity from activation due to head motion. Stimulus-correlated motion can be especially severe in areas lying near the high-contrast boundaries in the brain ( Fig. 5 ). Percent signal change of the voxels due to stimulus-correlated motion is typically much higher than that of the true activated voxels. The percent signal change could be used as a threshold to remove such false positive voxels.
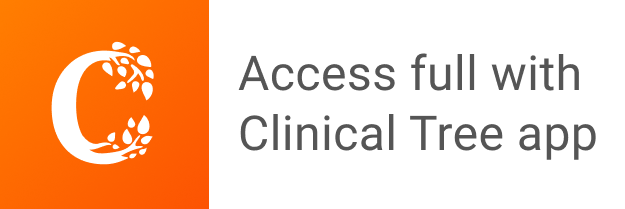