Harit Kapoor, Seema Kapoor, Meenakshi Bothra We are learning the language in which God created life. Bill Clinton Radiogenomics as the name might suggests has something to do with two widely different branches of medicine, the radiology and genomics which try to correlate the imaging features of diseases with the underlying genetic alterations responsible for the disease pathophysiology. It is a very recent concept with most of the literature focussed on cancer diagnosis and management. As we are aware that cancer is largely a genetic disease with the latest research involving the cancer management focussed on identifying targeted therapies according to the genetic makeup of the tumour. Also, with completion of human genome project in 2003 and various technological advances, there has been a discovery of various molecular biomarkers identified by gene expression profiling which are used for diagnosis, identification of tumour subtype, targeted therapy and prognostic evaluation. However, these genetic analyses require invasive procedure such as biopsy or surgical excision, are costly and not always readily available. Furthermore, molecular analysis performed via these invasive procedures may not give adequate information as the sample is being taken from a very small area of heterogenous tumour resulting in sampling errors. On the other hand, diagnostic imaging is an integral part of management of cancer patients, is non-invasive and more readily available as compared to molecular diagnostics (genomics). Therefore, the field of radiogenomics attempts to explore the potential relationships between the genetic make-up of tumour (genotype) with the various imaging patterns seen on diagnostic imaging (imaging phenotype) in order to develop non-invasive surrogate biomarkers for gene expression. The hallmark of cancer is unchecked cell proliferation which at genetic and molecular levels is attributed to processes such as genomic instability, mutations, accumulation of genetic and epigenetic modifications in the DNA. These genetic mutations have been shown to be predictive of survival and response or resistance to treatment. Furthermore, in addition of chemotherapy and radiotherapy, recently targeted therapy has become a mainstay in treatment of cancers. These targeted therapies target molecular pathways in tumour cell biology blocking action of enzymes, tumour-associated proteins and receptors, etc., therefore, the knowledge of various molecular pathways and associated mutations or genetic alterations modifying these pathways leading to unchecked cell proliferation make way to develop therapies targeting the specific alterations. With the availability of various biomarkers due to knowledge of genetic profile of tumours have made significant impact of diagnosis of cancers, but they need an invasive procedure such as biopsy or surgical excision. Radiogenomics aims to provide non-invasive biomarkers by identifying imaging signatures associated with particular genetic alterations. As mentioned in the above paragraphs, the term “Radiogenomics” is most often used to refer to the correlation between the imaging phenotype and genomic characteristics which include various patterns of gene expression, gene mutations and other genome-related characteristics. However, the term radiogenomics has also been used to refer to relationships between the genetic make-up of patient and his response to radiation therapy, in the context of radiation toxicity. Finally, the terms radiogenomics and radiomics are often confused, but they have a different meaning. Rather than evaluating a relationship between genome and imaging phenotype in radiogenomics, radiomics is concerned with the methodology used in such analyses which specifically include extraction of various qualitative features using various computer algorithms. “Radiomics” is defined as the analysis of imaging data through the use of specific algorithms aimed at identifying quantitative features otherwise not identifiable with the simple visual analysis, in order to create enhanced data models for improving medical decision support. In-depth details of the process of radiomic analysis is complex involving a lot of mathematics, however a brief conceptual overview of the process is presented to familiarize the reader with this topic. Broadly, two distinct phases are involved in radiomics which are feature identification phase and model development phase. Feature identification refers to describing those imaging features which are not seen on simple visual assessment, through use of various computer algorithms. The whole process further involves three steps which are firstly image acquisition, database creation and data selection. After the creation of imaging data base, the investigators identify and segment a particular volume of interest (VOI) which can be done manually or semi-automatically. Lastly, for a given VOI, a texture analysis is performed with the use of dedicated software. We know that medical image consists of a group of pixels or voxels (in 3D) representing the discrete shades of grey. In simple terms, texture analysis is nothing but mathematical analysis of differences in grey scale of pixels/voxels of the image to extract various quantitative features which include semantic and agnostic features. Semantic features are the geometrical features of the selected VOI such as size, volume, surface area, spiculations and vascularity. The more mathematically complex agnostic features are further divided into first-order statistics (describe distribution of pixels inside a single voxel), second-order statistics (describe inter-relations between voxels and provide information about heterogeneity of the lesion) and higher-order statistics (which extract repetitive and non-repetitive patterns in the image by imposing filter grids). Finally, all the radiomic data coupled with other non-radiomic data such as clinical, genomic and histologic data can be used to develop artificial intelligence-based predictive computer models which after being validated can be used to predict the genotype of a tumour, overall survival and response to treatment. The Cancer Genome Atlas (TCGA) project which started in 2005 having the catalogue of genetic mutations responsible for various cancers along with The Cancer Imaging Archive (TCIA) harbouring a large archive of medical images of various cancers including CT, MRI and histopathology will serve as a useful tool in further radiogenomic research. The most common and fatal primary brain neoplasm is glioblastoma which has dismal outcomes and poor prognosis despite decades of effort to improve patient survival. The mean survival is 1 years and 5-year survival <5% which could be attributed to extensive molecular and genomic heterogeneity. GBM is characterized by features such as local invasion and diffuse infiltration into the surrounding brain tissue with associated cellular proliferation and necrosis, marked angiogenesis, genomic instability, resistance to apoptosis, and therefore associated with a high likelihood of recurrence, poor response to treatment and morbidity. Therefore, radiogenomics research in brain is primarily focussed on glioblastoma. The initial radiogenomics research in brain evaluated the use of imaging features to predict the molecular subtypes which have been assessed through genomic profiling through TCGA and are associated with different tumour outcomes and progression patterns. Texture features extracted from MRI, tumour shape and oedema associated with tumour on T2-FLAIR images have been shown to predict molecular subtype belonging to one of the four groups: classical, neural, proneural or mesenchymal. More recently, stratification into one of the core pathways according to p53, RTK/RAS/PI K, and RB signalling alterations showed a better correlation with outcomes. Van Meter et al. (2006) demonstrated the presence of intra-tumoural spatial variations with respect to genetic expression in the lesion with the poorly enhancing and low perfused core having an elevated rate of expression for hypoxia-induced genes, whereas the peripherally enhancing tumour edge having an increased expression of genes involved in proliferation and invasion. Diehn et al. demonstrated the gene expression profiles were different in contrast-enhanced and non-enhancing tissues of the tumour. Furthermore, the genes responsible for proliferation were associated with the tumour mass effect and genes involved in hypoxic process showed correlation with contrast enhancement on T1W MRI images.
1.37: Radiogenomics: Insights into novel tool for cancer diagnosis and management
Introduction
Importance of genetic profile in cancer
Definitions
Radiomic pipeline
Applications of radiogenomics in common cancers
Brain
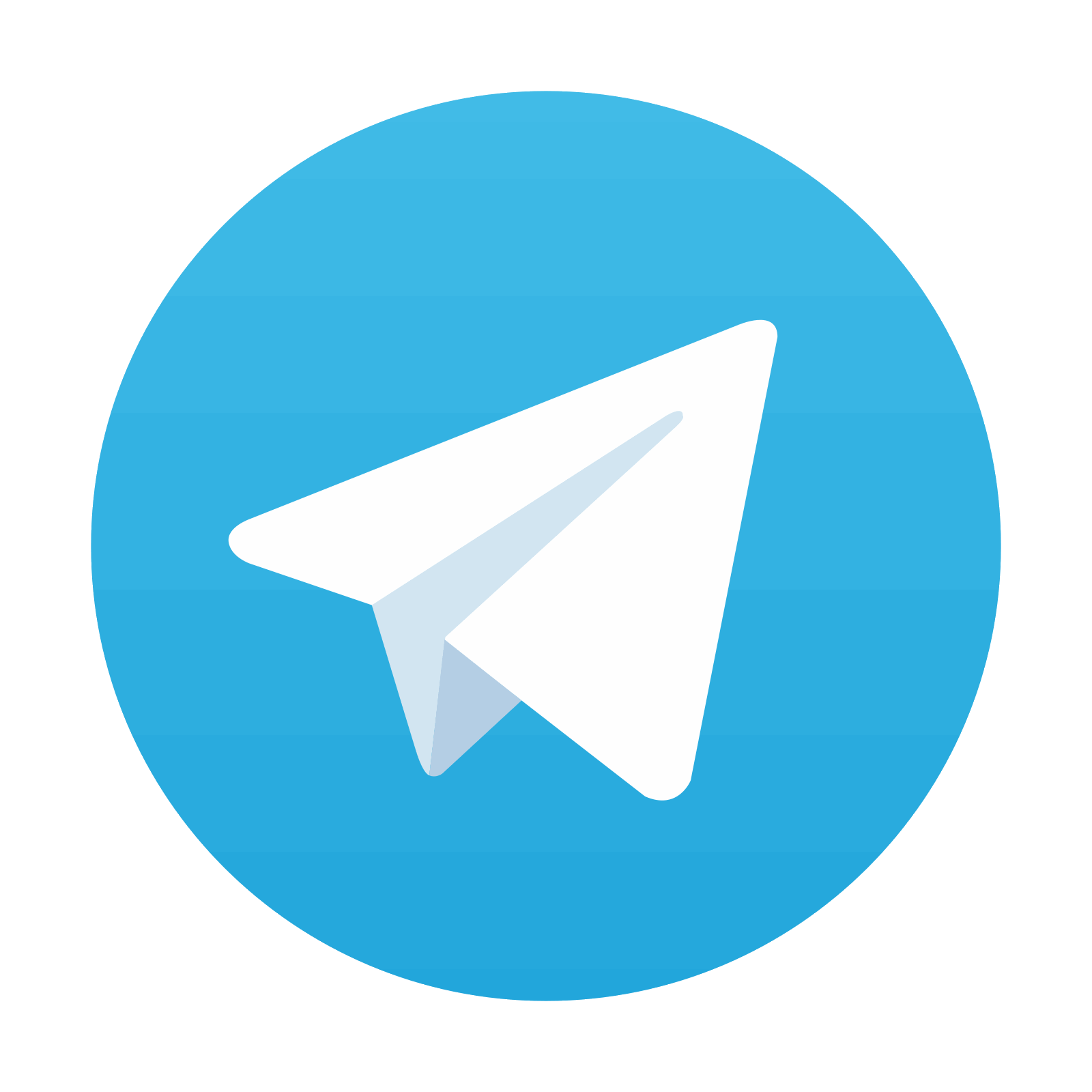
Stay updated, free articles. Join our Telegram channel

Full access? Get Clinical Tree
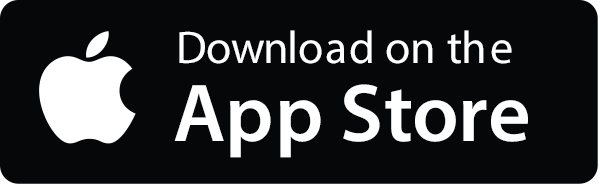
