, and the primary unknowns are the extracellular potential and the transmembrane potential
. Hence, the intracellular potential reads
. For all
with
such that, for all
with
,
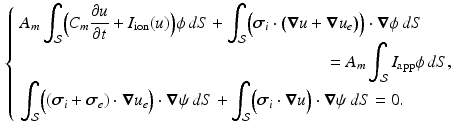
(1)






![$$\begin{aligned} {\varvec{\sigma }}_{i, e} = \sigma _{i,e}^t {\varvec{I}} + (\sigma _{i,e}^l - \sigma _{i,e}^t) \bigl [ I_0(\theta ) \varvec{\tau }_0 \otimes \varvec{\tau }_0 + J_0(\theta ) \varvec{\tau }_0^\perp \otimes \varvec{\tau }_0^\perp \bigr ], \end{aligned}$$](/wp-content/uploads/2016/09/A339585_1_En_46_Chapter_Equ2.gif)
(2)




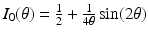
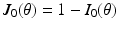

Table 1.
Conductivity parameters (all in
) and maximal conductance
in the different atrial areas (all in
) with RT = regular tissue, PM = pectinate muscles, CT = crista terminalis, BB = Bachman’s bundle, FO = fossa ovalis



![]() | ![]() | ![]() | ![]() | ![]() | ![]() | ![]() | ![]() | ![]() |
---|---|---|---|---|---|---|---|---|
![]() | ![]() | ![]() | ![]() | 7.8 | 11.7 | 31.2 | 46.8 | 3.9 |
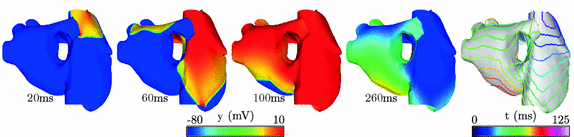
Fig. 1.
Atrial electrical depolarization and corresponding synthetic front data
2.2 Data of Interest
We assume in this work that the patient-specific depolarization front is measured, as is the case when isochrones are available. From a mathematical standpoint, the measurement procedure can be modeled by considering that, for a particular solution of (1) denoted by
and associated with patient-specific parameters and initial conditions, we have at our disposal the time evolution of the front

defining
as a threshold value characterizing the front, and the already traveled-through region is given by
– that essentially take two different values inside and outside the traveled-through region. Our objective is to use the image sequence
to reconstruct the target solution
, in a context where the initial conditions and some physical parameters are uncertain.
Get Clinical Tree app for offline access


(3)



3 Estimation Methodology
3.1 Sequential Estimation Principles
We consider a general dynamical system
, where y is the state variable, A the model operator, and
some parameters of interest. In this abstract setting, we consider a specific target trajectory
, where
is a known a priori whereas
is unknown, and assuming the same type of decomposition for the parameters
. We further assume that we have at our disposal some indirect measurements of the target trajectory represented by the observation variable z(t). Our estimation problem consists in reconstructing the solution
from the data z(t).







As a prerequisite to any estimation strategy, we must be able to define – at each time – a similarity/discrepancy measure
between the data z and the state variable y. When
vanishes, the state is exactly compatible with the data. By contrast, when
is non-zero, the data indicate that
.




To achieve our estimation objective, we adopt a so-called sequential strategy where we define an observer system – also known as sequential estimator system – as a new dynamical system of the form
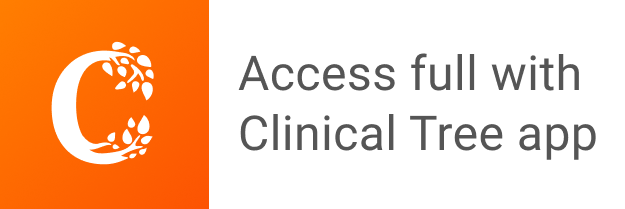