Imaging biomarker
Organ, disease
Metric
Reference
DWI:
ADC, nADC
Hepatic metastases
CVADC: 10.1 %
CVnADC: 8.3 %
Deckers et al. [3]
DCE-CT:
Arterial flow, blood volume, permeability
Gastroesophageal junction cancer
ICCAF: 0.88
ICCBV: 0.89
ICCPERM: 0.91
Lundsgaard Hansen et al. [4]
DWI:
ADC, D, D*, f
Hepatic metastases
CVADC: −14.7 to 13.8 %
CVADChigh: −15.5 to 9.46 %
CVf: −75.3 to 241 %
CVD*: −89 to 2120 %
CVD: −20.8 to 25.3 %
Andreou et al. [5]
DCE-MRI:
Ktrans
Kep
Ve
Vp
Renal cell carcinoma
ICCKtrans: 0.686
ICCKep: 0.906
ICCVe: 0.764
ICCVp: 0.657
Wang et al. [6]
T1 relaxometry
Liver
CVT1liver: 0.9–2.5 %
Aronhime et al. [7]
DWI:
ADC, f, D, D*, fD*, DDCa, a, DDCk, kurtosis
Pediatric tumors
CVADC: 3.3 %
CVf: 41 %
CVD: 2.5 %
CVD*: 35.1 %
CVfD*: 38.1 %
CVDDCa: 4.3 %
CVa: 3.5 %
CVDDCk: 6.1 %
CVK: 52.7 %
Jerome et al. [8]
Particularly in MRI, a significant breakthrough has been achieved in the last decades where significant imaging biomarkers have been developed for functional information assessment. Diffusion-weighted imaging (DWI) and diffusion tensor imaging (DTI) give an insight in the complexity of the tissue environment via the motion of water molecules. Dynamic contrast-enhanced (DCE) and dynamic susceptibility contrast (DSC) imaging techniques, acquired after intravenous administration of specific contrast agents, depict tissue perfusion and the microvascular environment. Magnetic resonance spectroscopy (MRS) is conducted for measuring the concentration of several biochemical metabolites in the tissue, reflecting unique information on its chemical composition.
10.2 Road for Biomarker Development
A significant number of imaging biomarkers are presented in the literature as potential indicators of biological processes and for monitoring the response to therapy. However few of these are used routinely in the daily clinical practice failing to replace the established histological “gold standards” [9]. This issue relies on the fact that integrating imaging biomarkers into the clinical practice requires a stepwise well-structured procedure in which a couple of criteria must be first followed. As reported in [10], these criteria underline the importance of a biomarker to (a) provide accurate, precise, and feasible measurements, (b) be associated with a clinical end point, and (c) perform in a specific context of its proposed use. In the context of developing biomarkers in general, the Institute of Medicine broadly classifies these criteria into three distinct but interrelated areas: the analytical validation, qualification, and utilization. Qualification and utilization are major parts for assessing the clinical impact of a biomarker, whereas analytical validation plays the most important role in determining the technical performance of it.
Analytical validation is a prerequisite step before qualification and utilization as it provides the concepts and methods for evaluating the validity and the performance characteristics of a biomarker from the technical perspective. The analytical validation process is not limited to accuracy metrics such as the sensitivity and specificity but the measurement of efficacy and effectiveness. Once technical performance is ensured for a potential biomarker, clinical questions need to be answered. Sensitivity and specificity of a potential biomarker in a human population and its cutoff points for establishing clinical decision-making systems and many other areas that are out of the technical scope of this chapter are major concerns that need to be addressed. Thorough studies for qualification and utilization are presented in [10–12].
The subsequent chapters will give an overview of the analytical validation criteria and address the computational models and measurement systems required for assessing the ability of a biomarker to meet the validation criteria. When met, established proofs of efficacy and effectiveness of imaging biomarkers will facilitate the use of these in drug development, therapy assessment, and patient care.
10.3 Proof of Efficacy and Effectiveness Through Analytical Validation
On the one hand, the proof of efficacy enrolls the necessity to assess the ability of a biomarker in measuring tissue characteristics from an acquired image reproducibly, reliably, and accurately. On the other hand, the proof of effectiveness is mainly involved in analyzing the ability of a biomarker to be clinically relevant, thus to be consistent as a potential indicator for clinicians, physicians, and drug developers to make proper and informed decisions. The Quantitative Imaging Biomarkers Alliance (QIBA) [13], organized by the Radiological Society of North America (RSNA), devotes its attention to the development of technical performance analysis methods and metrics in response to the aforementioned concerns for efficacy and effectiveness. As reported in [14] and [15], this technical infrastructure, provided by the QIBA Metrology Working Group, was grouped into three primary validation fields: measurement bias and linearity, reproducibility, and repeatability.
10.3.1 Standardization: A Prerequisite Before Validation
A major challenge to the biomarker validation is the lack of standard approaches to data gathering, analysis, and representation. Technical issues in standardization impair consistency, accuracy, repeatability, and reproducibility of a candidate biomarker, thus making the validation a difficult process. Two main categories related to standardization are highlighted in [12]: (a) the standardization of image acquisition and (b) the standardization of image analysis. The first category reflects the large variety of ways in which images of the same sequence are acquired. In case of DWI-MRI, optimization of the b-value distribution demonstrated improved accuracy and repeatability of the derived parameters from four mathematical models [16]. The second category refers to the mathematical framework in which the images are analyzed, visualized, and presented quantitatively. A representative study in [17] highlights the substantial influence of a region of interest (ROI) size and position into the quantification of the perfusion characteristics of DCE-MRI data.
To overcome these issues, standardized acquisition protocols and image analysis framework need to be addressed, strengthening reproducibility across different conditions. In case of DWI-MRI data, a thorough study in [18] underlines the standards required for stakeholders to conduct multicenter studies, evolving diffusion imaging biomarkers to be clinically useful with significant impact on drug development and patient treatment. Several consensus studies have been held on the standardization of perfusion MRI [19], PET, and CT [20].
10.3.2 Bias and Linearity
The ultimate goal of a potential imaging biomarker is to provide unbiased measurements in order to gain insight into the pathophysiology of a patient and to contribute significantly in the design of therapeutic trials. Accuracy and bias are often used synonymously [21] and in general describe the difference between a measurement and the true value of the same examined object. To this understanding, bias of an imaging biomarker can be evaluated either at cross-sectional studies in which the biomarker is measured at one time point or during a longitudinal study where changes are measured over multiple time points.
In both studies, inherent in the estimation of bias is to know the true value of the examined object. In case of in vivo imaging studies applied to patients, determining bias implies a challenging process because tissue characteristics and structural changes due to a disease (i.e., necrosis, fibrosis, cellularity, vasculature network architecture, etc.) are linked indirectly to their related imaging biomarkers [22] and gold standards from histopathology are necessary. Alternatively, validated tissue-mimicking materials (i.e., phantoms) can be well-defined references replacing real data for assessing bias [23].
Bias measurement typically begins with the qualitative representation of the measured value(s) against the referenced, otherwise the true or expected value, in a single plot. This visualization can be enhanced with confidence bounds that reflect the variability of the multiple measures and additional boxplots with outliers plotted. Quantitative assessment of bias includes metrics for estimating the squared difference between a measurement and the true value and thresholds based on agreement intervals varying within the 95 % of the total differences.
Linearity is a crucial indicator for estimating the change between the measurements and the true values over the range of true values. In other words, linearity can be measured in longitudinal studies and when combined with analysis of bias can potentially provide quantitative information which is directly proportional to the true value change. A single scatterplot depicting a pairwise analysis of measured values of a biomarker versus its true values will have a slope equal to one and intercept at zero when there is no bias and perfect linearity over the range of true values. With known linearity degree of an imaging biomarker in a longitudinal study, measurement interval limits are determined, ensuring that its measurements reliably indicate clinically important true changes. Simple regression analysis can be also followed to describe the statistical relationship between the multiple measurements of a biomarker and its true values over the range of them.
10.3.3 Reproducibility
Reproducibility refers to test conditions, assessing the same imaging biomarker at short intervals, when studies are conducted using different experimental conditions. These experimental conditions include multiple measurements of the same imaging biomarker but with different vendors, measuring systems, operators, and locations that may compromise the reproducibility of the results. A reproducibility study is said to be valid when at least one of the above criteria is met, and a potential biomarker is robust enough and reproducible when repeated measurements with no variation of the same subject are provided under diverse conditions.
Measurements in reproducibility studies can be derived from both synthetic and real data. Synthetic data can be retrieved from phantoms that mimic the tissue characteristics [24, 25], whereas real data can be provided by single lesion of a patient or a group of patients with similar characteristics (i.e., individuals with same disease) [26–29]. However, patient’s comfort and safety are crucial aspects that limit the ability to perform multiple repeated studies. Radiation exposure in CT and PET and the use of intravenous injection of contrast agents in DCE-MRI and DSC-MRI are typical features that limit the number of scans in a reproducibility test. On the other hand, phantoms often fail to illustrate the complexity and characteristics of the human tissue, thus leading to overestimated measurements for reproducibility [15].
A statistical analysis framework is required for assessing reproducibility qualitatively and quantitatively. Once a reproducibility experiment has been performed, useful statistics act as indicators of the variability between the different measurements. A technical representation of the reproducibility metrics is analytically outlined in [30]. Based on [30], reproducibility is often measured by two statistical metrics: the concordance correlation coefficient (CCC) and the reproducibility coefficient (RDC). Pairwise qualitative assessment is given by scatterplots and Bland-Altman plots. Moreover, distribution analysis based on histograms, Q-Q plots, and boxplots is an important tool to visually inspect the agreement between the biomarker estimates delivered from different conditions.
10.3.4 Repeatability
The potential ability of an imaging biomarker to act as an indicator of a biological process and for monitoring the response to therapy can be severely influenced by the lack of reproducibility and repeatability. These two terms are commonly confused, with varying degrees of consistency on their terms found in the literature [14]. Repeatability tests, often named as test-retest studies (Fig. 10.1), refer to the variation in repeat measurements of the same imaging biomarker under identical conditions. In contradiction to the experimental conditions in a reproducibility test, measurements are taking place under the same vendors, measuring systems, operators, and locations. A crucial prerequisite in repeatability tests is the short period of time required for measurements to be taken, in order to ensure that changes in the biomarker are not caused by inherent technical or physiological variation.
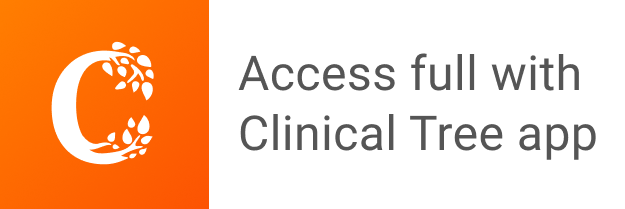