where



This parameter has been widely used in research for several decades, although it has been often misused. As a result [12], some concerns about this reliability measure. First, it must be remarked that this measure is a characteristic depending on the scores obtained in a particular sample of patients. It is not good practice to make statements about the reliability of an instrument under all circumstances, based exclusively on the value of Cronbach’s alpha. Besides, the set of selected biomarkers must represent a unidimensional construct so that the analysis of the internal consistency makes sense. For that reason, this coefficient should not be used if it is suspected that biomarkers represent a multidimensional structure. Finally, the value of Cronbach’s alpha depends on the number of biomarkers being studied. It appears that we may increase its value through the addition of new biomarkers to the instrument. Thus, this general reference of achieving a minimum value of 0.7 to ensure reliability may be distorted when large sets of biomarkers are examined. In fact, values of the Cronbach’s alpha greater than 0.95 may point out to redundancy between biomarkers. The usual situation where we may find such high values for this measure is unidimensional scales with too many items correlating and overlapping among them. Consequently, the recommendable values for this measure should range among 0.7 and 0.95 to ensure internal consistency [39].
An alternative method for assessing internal consistency is examining inter-item correlations, based on the correlations of each item to the scale as a whole, omitting that item. Minimum correlations of 0.20 are expected to be obtained among biomarkers in order to conclude that they are internally consistent. For dichotomous items, Kuder and Richardson [30] suggested an alternative coefficient as the equivalent of Cronbach’s alpha.
9.3.2 Observer Variation
Like internal consistency, observer variation constitutes an important source of variability in reproducibility studies. It may be caused by technical failures, misinterpretation of data, or skipping abnormalities while taking measurements for biomarkers. Measurements made by different observers usually present a high degree of variability due to the presence of bias between them. In contrast, measurements made by the same observer are more similar [3]. Meanwhile, the measurement errors associated to each observer can also have different standard deviations, if one of the observers is likely to obtain more precise measurements. The consequence in these circumstances is a higher intraclass correlation coefficient (ICC) within the same observer, when compared to the ICC corresponding to different observers.
The intraclass correlation coefficient (ICC) represents the correlation between one measurement of a biomarker (either a single rating or a mean of several ratings) and another measurement for that biomarker, but under different conditions [18, 37]. The ICC coefficient ranges from −1 to 1, like the Pearson correlation coefficient, and is defined as a ratio of true variance versus observed variance. However, the Pearson coefficient measures the similarity of the relative measures taken by two observers, whereas the ICC indicates the average similarity of the patients on both ratings.
The first step in calculating the ICC is to identify our research interests as regards to the role of the observers in the clinical trial. This coefficient refers to a family of analysis of variance, and there are three possible approaches for assessing observer variation accordingly (Cases 1, 2, and 3) [37]. In the first approach, we consider that each biomarker is measured by different observers (Case 1). Secondly, we can consider that a particular group of observers take measures for each biomarker (Case 2). Finally, following the third approach, all possible observers take measures in each biomarker (Case 3). These different approaches are specified in the mathematical formulation of the ICC coefficient.
If the study is designed according to Case 1, a one – way ANOVA is the most appropriate statistical model. In this analysis, total variability is decomposed into a Between – targets Mean Square (BMS) and a Within – targets Mean Square (WMS). On the contrary, if the study is designed as a Case 2 or 3 analysis, the within – target sum of squares is partitioned into a between – Judges Mean Square (JMS), referred to observers, and a Residual Mean Square (EMS). As mentioned, the only difference between both Cases is the assumption that observers are randomly sampled (Case 2) or fixed (Case 3).
For determining the effect of different observers in the variability of biomarkers, Case 1 is not interesting, as it does not allow the study of observer variation. If our interest lies in a particular selection of observers (Case 2), we should consider that biases between them are constant. Then, we assume that the effect of observers on the biomarker is fixed. Assessing differences between observers is essential for the interpretation of the results in most studies and will be an appropriate approach in most of studies. In these cases, allow for a random subject effect and fixed observed effects. Thus, a two-way mixed effects model will be adequate for obtaining the ICC.
Those who need to draw inferences about a wider population of observers should consider that biases between observers are constant. Perhaps the origin of these biases is inherent to the method itself, or the practitioner is interested in extrapolating his (or her) conclusions to other studies about the same clinical outcome. At that point, we consider that the observers in our study are a random sample of all potential observers from a larger population, and we assume that observers have random effects on the biomarker. In these cases, a two-way random effect model seems more appropriate, as random subject effects and random observer effects are both allowed [4]. However, the practitioner must beware the need for greater number of measurements (at least higher than two per observer) for analyzing the random effect of observer variation.
Differences between the measurements taken by different observers can be easily assessed for continuous data, as shown. In contrast, when data are categorical the question becomes more difficult to assess. Landis and Koch [31] suggested a set of tests for interobserver bias as generalized kappa-type statistics. However, Brennan and Silman [6] warned about the difficulties of dealing with data in categorical form. A more pragmatic approach based on raw data is recommendable, rather than simplistically calculating the χ2 statistic.
9.3.3 Test–Retest Reliability
Test–retest reliability assesses whether a biomarker yields the same results on repeated measurements separated by a lapse of time, provided that the rest of the conditions of the clinical trial have not changed as regards the clinical endpoint being measured. This analysis allows the researcher to distinguish between a true change in the biomarker and another one occurring on the basis of chance or systematic bias. A generic interval between 2 and 14 days is the recommendable length of time that should elapse between both measurements [39]. This period of time is large enough for not being able to recall the biomarkers’ previous measurements. However, it is not so long that actual changes in the clinical output may have occurred.
The use of Pearson correlations for test–retest reliability, or regression analysis, is now considered outdated as it can seriously exaggerate the impression of reliability [2]. It has been also argued that the statistical strength of the association between two measurements may not be due exclusively to agreement (Bland and Altman 1986). In fact, a change of units of measurement would not affect correlation, but it could have a significant effect in the assessment of agreement. Results from two repetitions of a measurement may correlate highly but be systematically different. For this reason, the intraclass correlation coefficient is usually a more appropriate indicator of test–retest reliability. Another alternative is to examine intrasubject variation in a graphical representation, as suggested by Bland and Altman [6], where differences in the measurements of a biomarker are plotted against the mean of both measurements.
9.3.4 Method Comparison
Whereas the ICC is commonly used for analyzing observer variation and test–retest reliability, the Bland and Altman plot tends to be largely applied for comparing two methods of measurement of the same biomarker. This plot is used for examining whether two methods of measurement are so similar that one of them could accurately replace the other [5]. This graphic plots on the x-axis the mean of a patient’s measurements using both methods
, against the difference between both methods
on the y-axis. Notice that we should always subtract the same method’s measurement from the other’s
in order to obtain consistent results.



This approach provides the bias and the limits of agreement for the bias. In simple terms, the bias is the overall mean difference between both methods of measurement, whereas the standard deviation of this bias is the estimate of the error. It is important to make a first inspection of the plot, in order to identify visible relationships between this bias across the x-axis and the difference of measurements on the y-axis. If the variability of the paired differences is uniform along the range of measurements, we may estimate the limits of agreement.
These limits of agreement are marked in the Bland and Altman plot using dashed lines and represent a range of values in which the “true” agreement will lie for approximately 95 % of the sample. In other words, the limits of agreement provide an interval within which 95 % of future differences between methods is expected to fall. The hypothesis of zero bias can be statistically examined by a paired t-test of the measurements from each method. However, the bias and limits of agreement must be additionally assessed from a qualitative perspective. If both fall within the lower and upper cut-offs defined by the practitioner as satisfactory, both methods may be used interchangeably. In contrast, if bias exceeds at least one cut-off, there may be an over- or underestimation of the true clinical endpoints measured through each method. Consequently, in these cases both methods cannot be considered identical and should not be used interchangeably [23, 27].
After checking the assumption of normal distribution for paired differences, we can compute the mean and standard deviation of the paired differences using the following expression:
If the paired differences are normally distributed, we may compute the standard error of the limits of agreement as
where n represents the number of subjects.

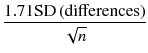
On the contrary, whenever a relationship between the bias and the differences in the measurements using different methods is found in the plot, a transformation (of the raw data) may be successfully employed. However, in those cases the limits of agreement will be asymmetric and the bias will not be constant. For instance, it is a common problem to find that the variability of the differences increases when the value being measured is larger. This problem can be solved taking the difference in the logarithm of the methods [4].
9.4 How Well Do Biomarkers Represent the Constructs Being Measured?
In the previous section, we discussed different approaches for examining reliability. However, a reliable biomarker does not involve its validity. The point is this: the reliability of a biomarker indicates the stability of measures under changing conditions, whereas validity is defined as the extent to which a biomarker measures what it intends to measure [29]. This point bears repeating because many biomarkers have been suggested to be valid indicators of some particular clinical outcomes, based on their values for the ICC or the Bland and Altman plot.
However, in the search for credibility, there is a tendency to accept almost any biomarker with high reliability coefficients, as an appropriate biomarker of a particular clinical outcome. Additional analysis of validity must be performed in those cases, as the description of the biomarkers needs to confirm the link between the clinical outcome and the biomarker. The following section will describe different types of validity, according to the approach of the analysis.
9.4.1 Content Validity
The content validity defines the degree to which a sample of items constitutes an adequate definition of the construct to be measured. In other words, it refers to how adequately the selected items (or the selected biomarkers) cover the topics that were specified in the scope definition of the instrument.
The content validity also includes face validity, which is particularly focused on the clinical credibility of a measure, thanks to its clarity and completeness with regard to the research area. There is a general consensus that content validity is largely a matter of expert judgment. Frequently, patients and experts are asked to critically review the content validity of an existing instrument, through formal focus groups, cognitive interviews, and, occasionally, tests of linguistic clarity [32]. Thus, a high number of composite indicators have been defined, in order to reflect the degree of agreement between experts. A widely used measure for reporting content validity is the content validity index (CVI) [33]. However, this index may be computed through two alternative methods, based on the universal agreement among experts, or an average between the item-level CVIs, sometimes leading to different conclusions [35]. Thus, statements about the content validity of an instrument should be based on exhaustive conceptualizations of constructs, well-written items, and carefully selected and trained experts regarding the underlying constructs and the rating task to be developed [12, 32].
9.4.2 Construct Validity
A construct is a theoretical concept, which is intended to be measured through a biomarker, or set of biomarkers. In the field of health and clinical sciences, the constructs of interest are often described as clinical outcomes. Generally, constructs are defined as latent variables, such as major depression or distress. These constructs are usually measured through several manifest variables such as patient outcomes, reported by the patient or observed by the clinicians.
Confirmatory factor analysis (CFA) provides empirical evidence of the convergent and discriminant validity of theoretical constructs, while adjusting for measurement error [7]. Convergent validity refers to the evidence that theoretically similar, or overlapping, constructs are highly correlated, while discriminant validity focuses on the lack of correlation between indicators of theoretically distinct constructs. The most elegant model for addressing the simultaneous study of convergent and discriminant analysis is the analysis of the multi-trait–multi-method (MTMM) matrices, as described by Campbell and Fiske [8].
9.4.3 Criterion Validity
Criterion validity examines to what extent scores provided by the instrument agree with a definitive “gold standard” reference of measurement in the same field of study. The COSMIN group arrived to the conclusion that no gold standard exists for health-related or patient-reported outcomes. Thus, as it cannot be assured that the comparison with other instrument is really gold, it may be more appropriate to focus on construct validity [33]. On the contrary, if the estimation of criterion validity may be supported by a gold standard reference, the type of data should be considered. If both instruments have continuous scores, correlational studies may offer interesting information. However, if one of the instruments has a dichotomous scale, the AUC provided by the ROC curve constitutes a more appropriate method [36].
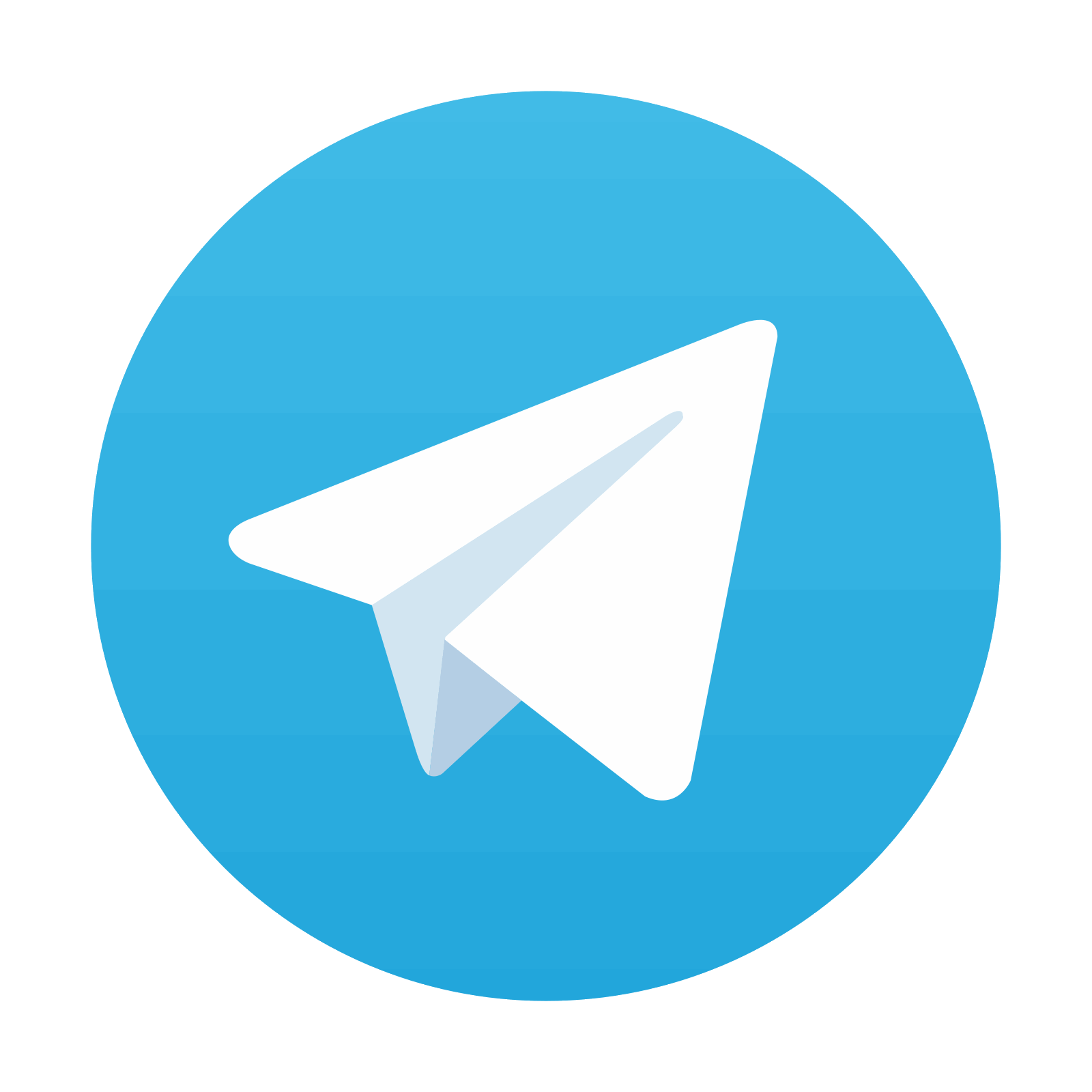
Stay updated, free articles. Join our Telegram channel

Full access? Get Clinical Tree
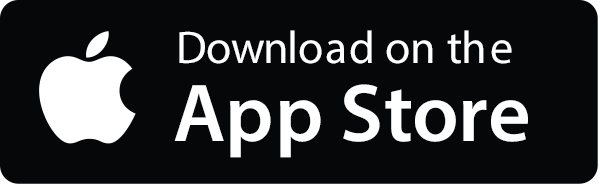
