div class=”ChapterContextInformation”>
14. Image Processing: What Is Needed and Unique for Vessel Wall Imaging?
Keywords
MRIVessel wall imagingImage processingVessel morphologyAtherosclerosisIntroduction
This chapter focuses on the image processing needs for vessel wall imaging (VWI). While general image processing techniques can be applied to VWI, there are many unique aspects which need special attention. First, the size of vessels typically presents small targets for imaging, allowing only several pixels of coverage for a vessel wall. Second, vessel wall metrics extend beyond size and thickness to tissue composition. Third, comprehensive analysis of tissue composition requires processing of multimodality, multi-contrast, and/or contrast-enhanced data, which requires image registration. Finally, physicians are not yet well trained in reviewing VWI, although this is rapidly changing.
The Need for Image Processing
As established in previous chapters, vessel wall imaging (VWI) is capable of identifying and quantifying diseases of vasculature in both large and small vessels. Most commonly these are atherosclerosis, aneurysm, and Moyamoya, but the tools provided by imaging are applicable to other pathologies. Following image acquisition, image processing methods can be employed to identify significant locations and quantify disease extent and severity. This chapter will focus on image processing for vessel wall morphology and characterization of atherosclerosis, which remains the primary motivation for vessel wall imaging and the focus of the majority of image processing methods. Applications in other vascular diseases generally use methods originally developed for atherosclerosis.
While imaging acquisition methods are key to visualizing wall morphology and atherosclerotic plaques, image processing methods, most commonly applied after acquisition, are needed to identify plaques at risk of rupture, identify and analyze plaque components, and provide accurate measurement of plaque size, volume, morphology, and characteristics. They also enable rapid analysis of plaques and may provide us with automated methods of risk analysis for future vessel wall rupture or ischemic events.
As vessel stenosis measurements alone have been shown to be insufficient for accurate diagnoses of all plaques at risk of future events [1], the concept of “vulnerable plaques” (VP), i.e., plaques at higher risk of rupture or event due to constituents, morphology, or other factors, has emerged [2–4]. A series of foundational studies have established the corresponding appearance of these VP features on images [5]. A study by Underhill et al. [6] in particular provides a concise, comprehensive framework for risk analysis in the carotid artery and identifies the features of atherosclerosis which image processing methods should focus on. These are briefly summarized below.
As we know from previous chapters, the development of atherosclerotic plaques is complex [7]. Plaques may not only grow into the vessel lumen causing stenosis (narrowing), of the vessel, but also expand outward from the vessel lumen without impinging on luminal flow, a process referred to as “outward remodeling” [1]. Remodeling and its subsequent increase in overall plaque burden are thought to be important risk factors in cardiovascular events. Specific morphological measures that have also been shown to be associated with VP are plaque thickness (measured cross-sectionally from the thickest point of lumen to the outer wall boundary); normalized wall index (NWI), calculated as wall area divided by total vessel area and a measure of total plaque burden along the length of the vessel; and plaque eccentricity, a measure of the shape or “concentricity” of the plaque [8]. This concept has long been applied to coronary arteries [9]. Image processing is essential in providing efficient tools for identification and accurate measurement of these morphologies. Challenges for these morphological measurements include effective and accurate identification of luminal and outer wall boundaries—which may not be apparent because of flow artifacts, motion, and poorly marked border between vessel wall and surrounding tissues.
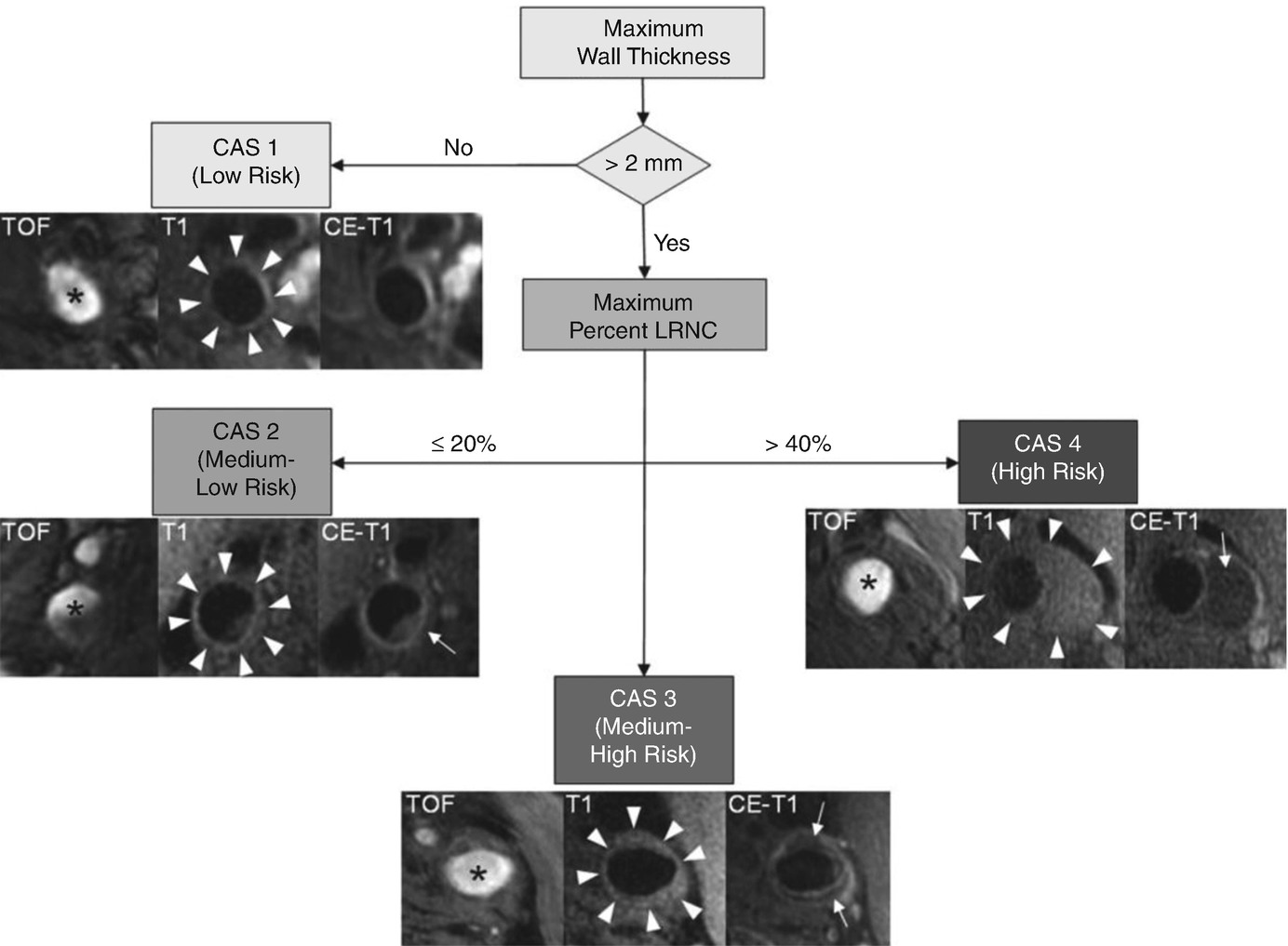
Flow diagram of carotid artery scoring system from Underhill et al. Subjects with a maximum wall thickness >2 mm require additional evaluation. Further categorization of lesions can be determined by the size of the maximum percentage LRNC. Examples of matched cross-sectional images from three contrast weightings (TOF, T1WI, and CE-T1WI) for each category are provided. Images corresponding to CAS 1 (maximum wall thickness <2 mm) are from the left common carotid artery of a 69-year-old man imaged at MSU. The plaque in the CAS 2 (maximum percentage LRNC, ≤20%) example is from the right internal carotid artery of a 63-year-old man imaged at UW. There is a small LRNC (arrow) present on postcontrast imaging. Of note, there are also flow artifacts visible in the lumen—common artifacts in images distal to the bifurcation. The lesion in CAS 3 (maximum percentage LRNC, >20% and ≤40%) is from the left common carotid artery of a 65-year-old man imaged at AH. A noticeable LRNC (arrows) is present in both the anterior and posterior arterial walls. An example of a large LRNC (arrow) without IPH in the left common carotid artery of a 64-year-old man imaged at PLA is shown for CAS 4 (maximum percentage LRNC, >40%). Arrowheads indicate the outer wall boundary; asterisk indicates the lumen. (Reprinted with permission from Underhill et al. [6])
Plaque enhancement patterns during and after contrast application improve tissue contrast and are associated with plaque inflammation and neovasculature [11–13]. In particular, leakiness of neovasculature is in the vessel wall has been linked with VP [11]. Its measurement requires implementing kinetic modeling into vessel wall imaging to provide quantitative measures of contrast agent uptake and associated risk. Image processing techniques are clearly needed for this analysis.
Furthermore, size, tortuosity, length, vascular pathology, and pressure are different for each vascular bed. There is evidence that atherosclerosis progression and identifiers of VP may vary throughout vascular beds. However, for the purposes of image processing, the key assessments for vessels in terms of disease evaluation remain the same: identify lumen and outer wall boundaries, and then define vessel wall morphology, plaque burden, distribution, and remodeling patterns, and identify regions of interest. Automated identification methods of lumen and outer wall boundaries can prepare image sets and enable plaque analysis and measurement that require human readers to be performed efficiently and relatively quickly.
Current Vessel Analysis Tools and What They Can Provide
Two well-known image processing techniques in vessel wall imaging are calcium scoring by CT and intima-media thickness (IMT) measurement by ultrasound. The first, calcium scoring based on CT imaging of coronary arteries, has shown that higher coronary calcium scores are predictive of patients with advanced coronary atheroma and are at higher risk for rupture [14]. This area has been much studied and confirms the initial findings investigating quantifying coronary calcium levels using CT [15]. The second, IMT as measured by ultrasound, has established that IMT measurements in the carotid artery are directly associated with increased risk of cardiovascular disease and progression [16, 17]. While CT and ultrasound both offer promising biomarkers for vessel disease, this chapter will focus on more recent MRI-based VWI processing techniques, which offer more comprehensive VWI information in multiple vascular beds.
Current and developing processing methods for identification, measurement, and analysis of all these vessel wall conditions are described below. A literature search for “vessel wall image processing” shows that many algorithms and methods have been attempted for vessel wall imaging; currently, however, only two software systems are both prevalent in peer-reviewed literature search and also used in multiple clinical studies and regulated drug trials. VesselMASS RE , developed by Medis, is a commercial software that has been used for vessel analysis in the Multi-Ethnic Study of Atherosclerosis [18, 19]. CASCADE , developed by the University of Washington Vascular Imaging Laboratory [20], is an academic software package which has been used in the ORION study CPC, AIM HIGH [21], and others [22–24].
Techniques for Image Processing
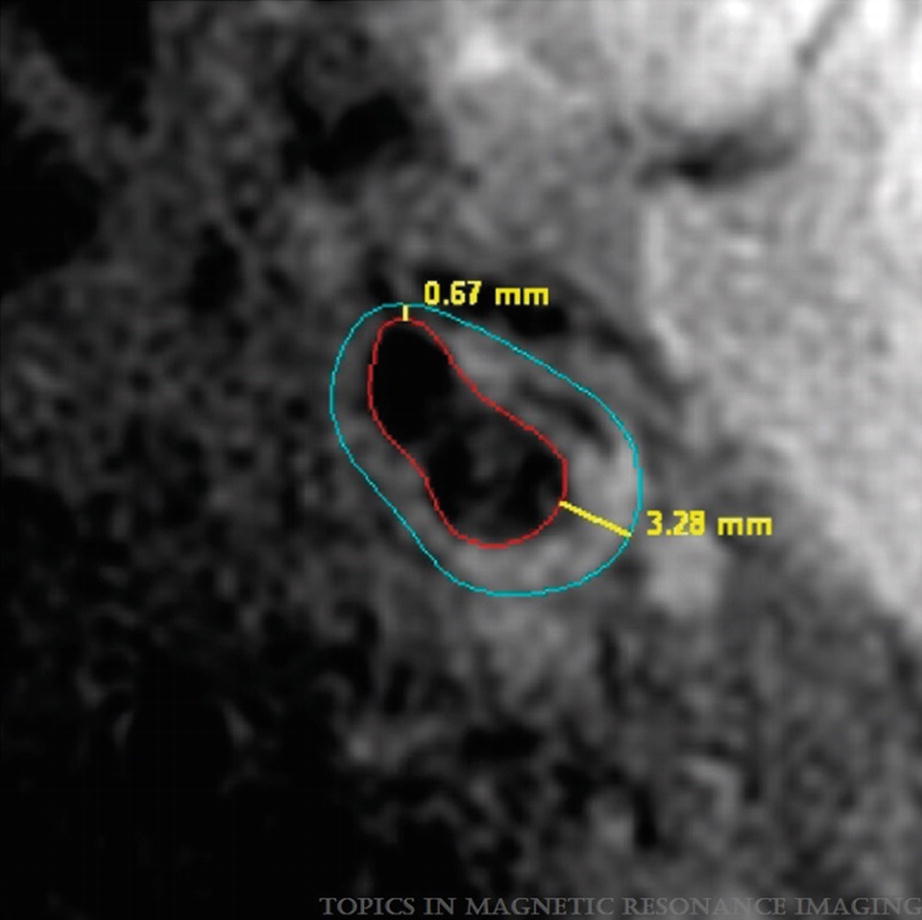
Example of wall thickness measurement by CASCADE showing the minimal and maximal values detected. (Magnetic Resonance Imaging of Carotid Atherosclerosis: Plaque Analysis, Kerwin et al. [20]. Copyright © 2019 Wolters Kluwer Health, Inc. All rights reserved)
In order to achieve accurate vessel wall measurements, relevant parameters for vessel areas must first be captured, namely, area, wall thickness, normalized wall index, and plaque eccentricity, further described below.
Parameters
Area
The capability of MRI to accurately define both luminal and adventitial boundaries for area measurement was first validated by a study that compared maximum wall areas measured from in vivo carotid MR images to images from corresponding ex vivo specimens in 1998 [56] and has since undergone a series of improvements for boundary detection [57], improved acquisition techniques, and establishing intra- and inter-reader reproducibility [40].
Vessel wall area, whether measured from lumen area, wall area, total vessel area, or area of a region of interest or plaque component, is typically measured by a number of pixels present inside the relevant contour. In the case of wall area, interior contours (i.e., the vessel lumen) may be subtracted from the outer wall. Due to vessel remodeling and varying morphology, vessel wall area measurements may represent a more accurate way of capturing total plaque burden and representative risk than clinically used measurements of vessel stenosis. A commonly reported used wall area measurement is normalized wall index, discussed in the following section.
Thickness
Thickness is typically represented as maximum wall thickness (MaxWT) and is defined by the thickest point of the vessel wall in the imaging coverage. Measurements of arterial wall thickness have been shown to be effective at differentiating lesions with and without LRNC. For ipsilateral atherothrombotic risk [25], MaxWT was predictive of ipsilateral ischemic events (HR, 1.6; 95% CI, 1.1–2.3). In a recent cross-sectional study based on over 1000 subjects who had suffered TIA/stroke (CARE II), MaxWT was also found to be a strong discriminator to identify high-risk plaque as compared to stenosis measurement. On the other hand, if one is looking for a marker of generalized CV health/risk, mean wall thickness may also be useful. For example, in Mani et al. [26], subjects with prior MACE had higher mean wall thickness than those without. In MESA [19] the JHU group reported that MRI-measured mean wall thickness was associated with CVD outcomes.
Normalized Wall Index
Normalized wall index (NWI) is calculated as wall area divided by total vessel area (wall area/(lumen + wall)area) ×100% and provides a measure of lesion burden that takes into account differences in the wall area for vessels of different diameters (i.e., the common, bulb, and internal carotid arteries). Saam et al. in a study published in 2007 [8] introduced this concept in carotid VWI and showed that a higher value for NWI is indicative of an artery with greater plaque burden. Since then, many studies have used this parameter in population-based studies [21] in different arteries and vascular beds, such as intracranial and peripheral arteries [27–29], and in comparisons of atherosclerosis burden between vascular beds [28–31].
Eccentricity
Vessel wall eccentricity, a concept initiated in coronary artery atherosclerosis analysis [9], has been implicated as a key feature of VP and has been defined as minimum wall thickness divided by maximum wall thickness [32]. It is introduced based on considerations of vessel wall remodeling and the impact of hemodynamic forces. With 3D vessel wall lumen and outer wall boundaries identified, eccentricity can be easily measured, and revised definitions have been used in a number of studies [9]. A study by Mossa-Basha et al. applied it to intracranial vessels and found it to be an important indicator of atherosclerotic disease diagnosis [33]. Another study found it to be an important factor in looking at plaque distribution and structural stress [34]. A paper by Li et al. found that measures of plaque eccentricity are associated with preserved lumen size and advanced plaque features such as larger plaque burden, more lipid content, and increased calcification in femoral arteries [35].
Plaque Composition
Prior to measuring plaque composition, multi-contrast MR images are obtained, typically including black blood T1W and T2W and bright blood time-of-flight (TOF) sequences [36–40]. After acquisition, images must be registered to account for patient movement and coverage of sequence.
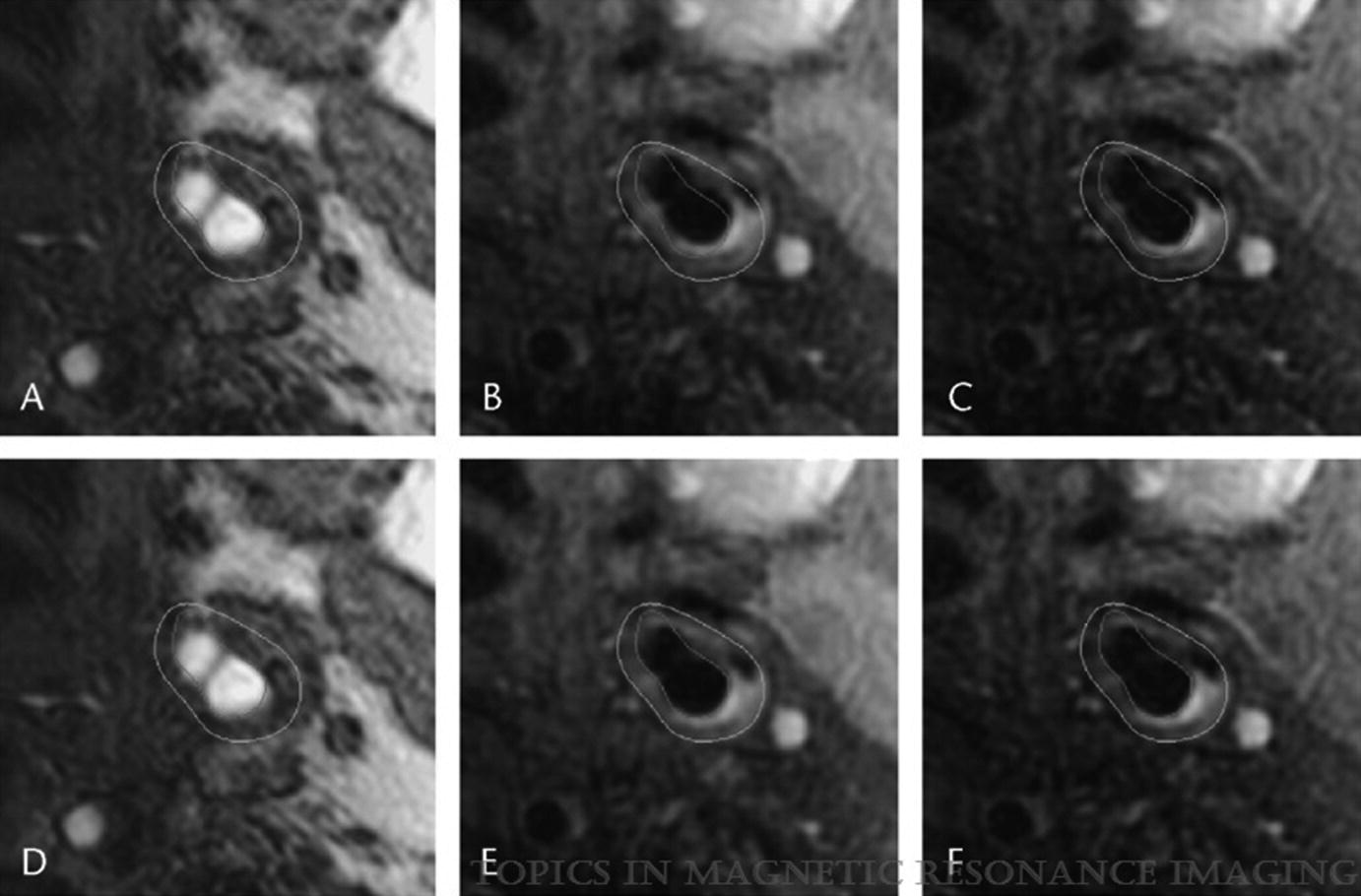
Registration: lumen and wall boundaries mapped to TOF (a), PDW (b), and T2W (c) images show misalignment that is corrected by the registration algorithm (d–f). (Magnetic Resonance Imaging of Carotid Atherosclerosis: Plaque Analysis, Kerwin et al. [20]. Copyright © 2019 Wolters Kluwer Health, Inc. All rights reserved)
Advanced Features
Image Segmentation and MEPPS
A major tool in VWI processing is image segmentation—a method for separating components of the vessel wall or regions of interest seen on imaging for further analysis and measurement. This is a highly challenging area for MRI, as vessel segmentation requires multiple contrast weightings and image registration to accurately capture the parameters presented above. Additionally, human review, or manual segmentation, methods are often time-consuming and require specialized training, making them clinically unviable. While many automatic image segmentation methods exist, few have been evaluated against human reviewers and histological ground truth.
Morphology-enhanced probabilistic plaque segmentation (MEPPS) is an automated image processing tool that was developed to mimic successful segmentation by trained human reviewers [41]. Unlike other segmentation methods, which typically derive segmented regions from the intensity values in different contrast-weighted images, MEPPS uses morphologic distribution information as an important factor. MEPPS was tested in 142 sets of multi-contrast MR images in patients undergoing carotid endarterectomy, and results were compared to the ground truth from corresponding histology of the excised carotid plaques for regions identified as necrotic core, calcification, loose matrix, and fibrous tissue. The results showed correlations (R2) for the histology confirmed areas of 0.78, 0.83, 0.41, and 0.82, respectively. By comparison, manual review by trained reviewers blinded to histological results yielded correlations of 0.71, 0.76, 0.33, and 0.78, respectively.
Subsequently, MEPPS has been used in multiple clinical studies and trials. MEPPS also has the advantages of being relatively simple to use and does not introduce concerns of inter-reader variability. In a study by Kerwin et al., MEPPS was used to evaluate signal feature differences between two field strengths [42] to assess changes in plaque tissue over time [43] and to support the claim of plaque compositional changes in prospective trials of pharmaceutical treatments of atherosclerosis [21, 22, 42–46].
Dynamic Contrast Enhancement and Ktrans
For the vessel image processing parameters listed in section “Introduction”, contrast-enhanced imaging is treated no differently than non-contrast and may be conducted using CASCADE or other tools. For quantitative measures of plaque inflammation and angiogenesis (i.e., neovasculature that may permeate atherosclerotic plaques) however, dynamic contrast-enhanced magnetic resonance imaging (DCE-MRI) coupled with pharmacokinetic modeling must be used. Following imaging with a 2D DCE acquisition protocol, a Kalman filter-based algorithm called the Kalman filtering, registration, and smoothing algorithm is applied to simultaneously filter noise and register image motion. Quantitative DCE-MR parameters related to perfusion characteristics may then be derived. The simplest of these is integrated area under a curve, consisting of the area under the signal intensity versus time curve (AUC) with different durations, a model-free approach that is highly reproducible. This method, however, suffers from a lack of physiologic meaning. Therefore, a more advanced approach using the Patlak model for pharmacokinetic modeling was developed by Kerwin et al. [47] and validated with histology. This method estimates Ktrans (the transfer constant reflecting permeability of neovessels) and vp (the fractional plasma volume) for each pixel by linear fitting. Both Ktrans and vp have been correlated with histological features of inflammation and angiogenesis in multiple studies [11, 48].
Intracranial VWI Analysis
As outlined in the AJNR consensus paper of [49], VWI’s application includes atherosclerosis, vasculitis, Moyamoya disease, radiation-induced arteriopathy, and arterial dissection. Both vessel morphology and tissue signal feature information, especially on postcontrast application, are critical for the accurate diagnosis. The role of image processing, especially 3D-based techniques, is important to assist in the analysis. To date, there has been limited report on specialized analysis packages addressing the special needs for ICA analysis.
The Future of Image Processing Methods in VWI
Vessel wall imaging is still a field going through rapid development in terms of image acquisition techniques and potential areas of clinical and research applications. Imaging processing will play a key role in this development. There are four areas worth consideration.
First is on simplified methods of plaque analysis and with specific targeted applications that can facilitate the clinical adaptation of VWI. We have discussed the usage of several software packages in this chapter, but they are designed more as a research tool for clinical trials with detailed vessel wall morphology and composition analysis as the main purpose. In many situations, effective simple methods may be more useful for clinical applications.
Second is on adopting the advancement in image acquisition techniques. An exciting new trend in VWI is the use of quantitative T1 and T2 mapping techniques for tissue characterization. In some sense, these techniques may reduce the need for image processing. But in reality, tissue maps will always need to be combined with proper imaging processing in order to provide usable data. In other words, image processing may need to be adopted as part of the quantitative imaging techniques.
Third is on the integration of different VWI techniques in imaging processing. The description of image processing techniques in this chapter is focused on MRI-based data acquisition and the needs to address multi-contrast analysis. VWI is a vast field that includes other imaging modalities, such as ultrasound, PET, and CT, each offering different aspects of information on the vessel wall, with different spatial resolution and viewing angles. Being able to integrate these data sets together will provide a comprehensive view of vascular maps. Furthermore, beyond anatomic images, blood flow, vessel wall compliance and elastographic properties can now be assessed by imaging [50–54]. Effective integration of these data with 3D anatomic VWI will also be very useful and exciting.
Fourth is on training of physicians and professionals on how to use analysis tools that will be paramount to advancement of this field.
Finally, we will touch on the role of artificial intelligence (AI). AI provides an exciting opportunity in VWI from image acquisition to image quality improvement and image processing. A recent study by Li et al. [55] introduced an AI-based technique to sort carotid VWI into normal, diseased, or severely diseased categories automatically, within minutes of the original MRI data being acquired. The technique was developed based on over 300 carotid scans in subjects with varying levels of atherosclerosis.
In summary, image processing plays a key role in acquisition, registration, and qualitative and quantitative measurement of vessel wall imaging, without which assessments of vessel wall status and risk would not be possible.