Resting state functional MR imaging methods can provide localization of the language system; however, presurgical functional localization of the language system with task-based functional MR imaging is the current standard of care before resection of brain tumors. These methods provide similar results and comparing them could be helpful for presurgical planning. We combine information from 3 data resources to provide quantitative information on the components of the language system. Tables and figures compare anatomic information, localization information from resting state fMR imaging, and activation patterns in different components of the language system expected from commonly used task fMR imaging experiments.
Key points
- •
Task functional MR imaging is the standard of care for mapping the language system before surgery.
- •
The language system is composed of multiple components and more than one task is typically required to fully localize the language system.
- •
Resting state functional MR imaging can localize the language system but its relationship to task functional MR imaging and anatomic landmarks has not been fully characterized.
Introduction
Localization of language regions using task-based functional MR imaging (T-fMR imaging) is currently considered standard of care before surgical resection of brain lesions that may impinge on these critical areas of the brain (“eloquent cortex”). This information is considered critical for presurgical planning, assessing the risk for morbidity, and consultation with patients and their families. This method is most often used for brain tumor resection, but has become common in numerous other neurosurgical procedures such as epilepsy surgery, brain biopsies, and laser ablation procedures.
Mapping language function accurately is complicated by several factors such as the numerous components of the language system (receptive vs expressive language, memory, reading, listening, and speaking that involve components of vision, hearing, and the motor systems), and the variability of its location across patients even after hemispheric dominance is established. Further complicating the surgical plan is the need to integrate this information between aggressive resection (that can extend life and delay recurrence) and functional preservation (decreasing morbidity), which can differ depending on the exact location of the tumor with respect to different parts of the language system. Some areas of the language system will not recover from resection (eg, Broca’s area), but others may be fully restored after recovery time and therapy (eg, supplementary motor area).
Although no one task can fully characterize the entire language system, a large collection of tasks have been developed as part of research in the field of system neurosciences. These tasks have been designed to activate and map different components of the language system. Owing to time and patient participation constraints, it is necessary to customize the T-fMR imaging examination to the individual patient. The complexity of this task has not gone unnoticed in the research literature, , , , including a white paper from the American Society for Functional Neuroradiology.
The need for patient participation in the task is critical for accurate language mapping. Alternative approaches are needed when a patient cannot participate in the task, such as in cases of confusion, disability, need for sedation, or in young children. One approach that is used by a several sites in such situations is resting state fMR imaging (RS-fMR imaging). Specifically, the multilayer perceptron algorithm has been used successfully for language localization in a large series of patients. This approach does not require patient participation and is able to extract language (and other) maps from calculations of functional connectivity across the brain. Although further studies are needed to fully characterize the accuracy and usefulness of this method in surgery, preliminary studies indicate that it provides a fairly balanced map of the language system and this result would be expected because it is not specific to any one task.
Customization of the T-fMR imaging study should take into account the condition of the patient (their ability to participate in the examination and how long they can lay still in the MR imaging scanner), the location of the tumor with respect to the language system, and the information that can be obtained from different specific language tasks. Several articles , , , have emphasized the need to map multiple language areas for surgical planning. The American Society for Functional Neuroradiology white paper is valuable in providing practical suggestions for task selection.
Several recent technical developments in informatics techniques applied to neuroimaging analysis can help us quantitatively answer the central question of this study: How do the methods of T-fMR imaging and RS-fMR imaging compare in regard to localization of the language system? The tools used in this article include parcellations derived from the MNI152 atlas using FreeSurfer ( surfer.nmr.mgh.harvard.edu ), which has significantly improved our ability to characterize anatomic regions across the brain. The second tool used is the Neurosynth software platform ( www.neurosynth.org ), which provides activation maps from meta-analysis of thousands of T-fMR imaging studies. The third tool we use is a deep learning 3-dimensional convolutional neural network (3D CNN), trained on thousands of normal subjects, which maps the language system using RS-fMR imaging.
Methods
Anatomic Parcellation
Anatomic parcellation makes inferences based on neuroanatomy represented on the Montreal Neurologic Institute atlas, which includes the average of 152 T1-weighted MR imaging scans nonlinearly transformed into Talairach space (MNI152). We applied the recon-all command from FreeSurfer on the MNI152 atlas to generate parcellations. We used FreeSurfer version 6.0.0. For this work, we curated FreeSurfer parcellations to coincide with 10 language-relevant anatomic regions described by Brennan and colleagues. These regions can be roughly divided into 3 groups, from the frontal lobe, the parietal lobe, and the temporal lobe. From the frontal lobe, we defined Broca’s area to comprise the left hemispheric pars opercularis and pars triangularis (FreeSurfer 1018, 1020). The dorsolateral prefrontal cortex (DLPFC) was manually curated by the union of the posterior portion of the rostral middle frontal region (FreeSurfer 1027, 2027), and the caudal middle frontal region (FreeSurfer 1003, 2003). The anterior insula was defined by FreeSurfer indices 1035, 2035, and the supplemental motor area was manually created from the portion of the superior frontal gyrus (FreeSurfer 1028, 2028) obliquely posterior to the RAS coordinate (−18.73, 27.58, 60.63). From the parietal lobe, the angular gyrus and supramarginal gyrus retained parcellations natively defined by FreeSurfer indices 11,125, 12,125, 1031, and 2031, respectively. From the temporal lobe, Wernicke’s area was manually created from the superior temporal gyrus posterior to Heschl’s gyrus. Heschl’s gyrus, the middle temporal gyrus and inferior temporal gyrus retained parcellations natively defined by FreeSurfer indices 1034, 2034,1015, 2015, 1009, and 1009, respectively.
Task Functional MR Imaging Meta-Analysis
The Neurosynth software platform provides automations for parsing the texts of published task fMR imaging (T-fMR imaging) studies, identifying topical words that convey neuroimaging semantics, generating models for documents and their texts, parsing published tables for purposes of extracting task activation coordinates, and aggregating task activation data into visualizable statistical maps of significance. The developers of Neurosynth were motivated by the need to aggregate and synthesize large numbers of T-fMR imaging studies disseminated across peer-reviewed publications. Because T-fMR imaging studies are often underpowered and have high false-positive rates, meta-analyses are useful for obtaining consistent, replicable quantitation with high specificity. , We used Neurosynth to generate automated meta-analyses that mapped a set of cognitive terms, curated from the literature, to task activation maps.
Neurosynth extends the methods of information retrieval, which have enabled modern Internet search engines, to support neuroimaging. We used Neurosynth’s term frequency-inverse document frequency (tf-idf) scheme , to map terms to task activations. Queries of topical terms from a corpus of documents require scoring schemes for accurate query matches. Term frequency is the number of occurrences of a query term per document, evaluated over all documents. Inverse document frequency is log ( N / df ) for N documents and df defined to be the number of documents containing a query term. Inverse document frequency assigns lower scores to query terms having little specificity (eg, “fMR imaging” in the corpus of documents in Neurosynth has high frequency and low information). The product tf-idf improves specificity for query terms occurring many times in a small number of documents (eg, “syntactic” in Neurosynth documents). Neurosynth directly maps tf-idf scores to task activations using activation coordinates parsed from documents. Thereby, we used Neurosynth to generate statistical maps of z-scores for χ 2 tests of significance on terms mapped to task activation coordinates. We used Neurosynth versions deployed at the web portal www.neurosynth.org in the spring of 2020. The active dataset for search queries was version 0.7, released in July 2018. The tf-idf scheme had been applied to the texts of all abstracts from 14,371 peer-reviewed documents published between 2000 and 2018. The active dataset provided 1335 searchable terms and enabled searching 507,891 task activations. We visualized terms mapped to task activations using the hyperbolic tangent of z-scores, which formally yielded correlation coefficients.
Table 1 describes an initial list of topical terms curated from the enumerations of frontal, parietal and temporal foci of task functionality given by Brennan and colleagues, Zacá and colleagues, and Black and colleagues. We pruned this initial list according to the number of studies and number of activations reported by Neurosynth. For similar terms, for example, “semantic,” “semantic memory,” “semantically,” and “semantics,” we selected the topical term with the greatest number of studies and the greatest number of activations. We removed terms with correlation maps that possessed sparse, small clusters. We pruned further after comparing to FreeSurfer regions and resting state networks (RSNs), retaining terms with larger Jaccard and boundary F1 (BF1) similarity measures. Our final selection retained 15 topical terms: “attention,” “hearing,” “language,” “lexical,” “listening,” “naming,” “nouns,” “phonological,” “reading,” “semantically,” “sentence,” “speech perception,” “syntactic,” “verb,” and “words.”
Term | No. of Studies | No. of Activations | fMRI Tasks |
---|---|---|---|
Attention | 1831 | 65,346 | Nonspecific (weak activity) |
Hearing | 124 | 4393 | Passive story listening |
Language | 1101 | 42,749 | Sentence completion Antonym generation Rhyming |
Lexical | 331 | 14,271 | Sentence completion Antonym generation Rhyming |
Listening | 250 | 9819 | Passive story listening |
Naming | 179 | 7361 | Silent word/verb generation Object/category naming Noun–verb association |
Nouns | 100 | 4434 | Silent word/verb generation Object/category naming Noun–verb association |
Phonological | 377 | 17,844 | Sentence completion Antonym generation Rhyming |
Reading | 521 | 21,842 | Sentence completion Reading comprehension |
Semantically | 122 | 4241 | Silent word/verb generation Object/category naming Noun–verb association |
Sentence | 307 | 11,204 | Sentence completion Antonym generation Rhyming |
Speech Perception | 97 | 3178 | Passive story listening |
Syntactic | 169 | 5369 | Silent word/verb generation Object/category naming Noun–verb association |
Verb | 127 | 5079 | Silent word/verb generation Object/category naming Noun–verb association |
Words | 948 | 38,353 | Sentence completion Antonym generation Rhyming |
Mapping Language with Deep Learning
Deep learning computational models learn detailed representations of complex data and have been highly successful in visual object recognition. Deep learning models comprise simple nonlinear modules that are composed in layers and other organizing structures. Model parameters learn the features of data in hierarchical layers of abstractions, such that higher level layers amplify features that are discriminating and suppress features with irrelevant variations. Compared with other machine learning methods, deep learning requires minimal domain-specific engineering, building internal representations directly from large amounts of available data and making use of extensive training computations. Multilayer perceptrons have successfully classified RSNs from RS-fMR imaging. We made full use of the benefits of deep learning by using a 3D CNN with 3 and 5 cubic convolutions, 49 layers, and 3 dense blocks in a densely connected architecture. The 3D CNN was implemented in Matlab R2019b ( www.mathworks.com ).
The 3D CNN was trained to classify brain regions belonging to a priori assigned RSNs. Training made use of normal human RS-fMR imaging data (n = 2795) from the Harvard-MGH Brain Genomics Superstruct Project as well as from additional studies in progress at Washington University. These are detailed in Table 2 and include the Alzheimer’s Disease Research Center, the Dominantly Inherited Alzheimer’s Network, and the HIV Program at the Division of Infectious Diseases. Each subject had approximately 14 min of RS-fMR imaging data (gradient echo echoplanar imaging with 3000 m TR, 3 mm cubic field of view), which were denoised, motion corrected, low-pass filtered and adjusted with global signal regression using methods previously described . RSNs were identified from 300 spherical regions of interest (ROI), which had previously been identified on a meta-analysis of T-fMR imaging. The choice of ROIs is detailed in Gordon and colleagues. From the 300 ROIs we extracted a subset belonging to 9 RSNs that are associated with the language system. These include the ventral attention network (VAN), cingulo-opercular network (CON), auditory network (AUD), default mode network (DMN), parietal memory network, fronto-parietal network (FPN), salience network (SAL), dorsal attention network (DAN), and medial temporal network (MTL). Of note, the 300 ROI parcellation does not have a separate language network; however, it includes the language system as a major component of the VAN. For each of the RSNs, an output map from the 3D CNN estimated the probability that voxels belonged to the RSN. Multiple (n = 268,000) example sets were generated from the data and then divided into training (n = 187,600) and validation (n = 80,400) sets. Outputs of our 3D CNN were native to a standardized atlas customized for scanners and preprocessing workflows at our institution and we linearly transformed these outputs to MNI152.
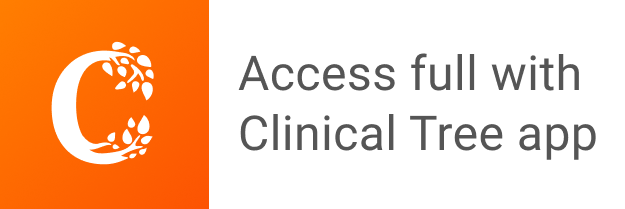