The new World Health Organization classification of brain tumors depends on combining the histologic light microscopy features of central nervous system (CNS) tumors with canonical genetic alterations. This integrated diagnosis is redrawing the pedigree chart of brain tumors with rearrangement of tumor groups on the basis of geno-phenotypical behaviors into meaningful groups. Multiple radiogenomic studies provide a bridge between imaging features and tumor microenvironment. An overlap that can be integrated within the genophenotypical classification of CNS tumors for a better understanding of different clinically relevant entities.
Key points
- •
The new World Health Organization (WHO) classification has been recently released where integration of genotype and phenotype was done to achieve a more accurate and clinically relevant classification of different brain tumors.
- •
The new classification depends on combining the histologic light microscopy features of central nervous system tumors with key canonical genetic alterations.
- •
This new integrated diagnosis is redrawing the pedigree chart of brain tumors with rearrangement of tumor groups on basis of geno-phenotypical behaviors into clinically meaningful groups.
Introduction
Neoplasm of the central nervous system (CNS) represent a wide range of tumors. Each tumor is characterized by a set of unique features that are translated in the form of specific clinical presentations, different treatment approaches, and distinct outcomes. Therefore, accurate and precise classification and grading of brain tumors become essential steps in management of such tumors, which are expected to affect over 23,000 patients in 2016. Historically, brain tumor grading and classifications depended solely on their microscopic picture, where similarities with putative cells of origin and the degree of differentiation were the key classifiers. Tumors were grouped on the basis of light microscopic features in hematoxylin and eosin-stained sections, immunohistochemical detection of specific protein expression, or characteristic ultrastructure features.
Confining tumor classification to structural similarities was limiting, as sharing similar structural appearance did not fully reflect on clinical features or tumor behavior. For instance, astrocytic tumors that share similar microscopic features can behave distinctly from each other. Therefore, a more comprehensive classification is essential. In the effort to reach such clinically relevant classification, several studies in the past decade identified key genetic alterations that contribute to the tumorigenesis process of distinct tumors. These canonical genetic alterations were recently implemented in the new 2016 World Health Organization (WHO) classification of brain tumors.
The new WHO classification combined both the phenotypic and the genotypic key features for CNS tumor classification. Combining both features resulted in a more clinically relevant grouping of tumors, which would reflect on diagnostic accuracy with direct impact on management plans. This linking of both phenotypic and genotypic parameters is another step to an integrated efficient classification of brain tumors. However, it still faces multiple challenges, including:
- •
Economic challenges of availability of genotyping.
- •
Technical challenges involving the choice of genotyping techniques as well as standardization of such techniques to ensure universality of results.
- •
Communication challenges on how such integrated diagnoses should be reported.
Recently, many studies have focused on creating a bridge between the imaging gross appearance of CNS tumors and the underlying key genetic and molecular features. These radiogenomic correlation between the imaging phenotype and genetic profile of tumor can play a pivotal role in a more comprehensive tumor classification that integrates histologic appearance of the tumor with canonical genetic alterations in addition to imaging phenotypes of the tumor observed on different imaging modalities. In addition, an exponential amount of imaging features can be extracted to detect the trivial and minute voxel-by-voxel changes occurring in the context of CNS tumors. The term radiomics is used to describe such high throughput extraction of imaging features. These features can be correlated with clinical outcomes to create robust prognostic predictive markers and can be further correlated to genetic and epigenetic alterations occurring in different tumors. This exponential amount of data can be easily integrated into big data analytics for a more precise and accurate prediction of tumor type, grade, and more importantly its genetic features. Such advances in the field of medical imaging and imaging analytics are anticipated to become cornerstone of future classifications of brain tumors.
Introduction
Neoplasm of the central nervous system (CNS) represent a wide range of tumors. Each tumor is characterized by a set of unique features that are translated in the form of specific clinical presentations, different treatment approaches, and distinct outcomes. Therefore, accurate and precise classification and grading of brain tumors become essential steps in management of such tumors, which are expected to affect over 23,000 patients in 2016. Historically, brain tumor grading and classifications depended solely on their microscopic picture, where similarities with putative cells of origin and the degree of differentiation were the key classifiers. Tumors were grouped on the basis of light microscopic features in hematoxylin and eosin-stained sections, immunohistochemical detection of specific protein expression, or characteristic ultrastructure features.
Confining tumor classification to structural similarities was limiting, as sharing similar structural appearance did not fully reflect on clinical features or tumor behavior. For instance, astrocytic tumors that share similar microscopic features can behave distinctly from each other. Therefore, a more comprehensive classification is essential. In the effort to reach such clinically relevant classification, several studies in the past decade identified key genetic alterations that contribute to the tumorigenesis process of distinct tumors. These canonical genetic alterations were recently implemented in the new 2016 World Health Organization (WHO) classification of brain tumors.
The new WHO classification combined both the phenotypic and the genotypic key features for CNS tumor classification. Combining both features resulted in a more clinically relevant grouping of tumors, which would reflect on diagnostic accuracy with direct impact on management plans. This linking of both phenotypic and genotypic parameters is another step to an integrated efficient classification of brain tumors. However, it still faces multiple challenges, including:
- •
Economic challenges of availability of genotyping.
- •
Technical challenges involving the choice of genotyping techniques as well as standardization of such techniques to ensure universality of results.
- •
Communication challenges on how such integrated diagnoses should be reported.
Recently, many studies have focused on creating a bridge between the imaging gross appearance of CNS tumors and the underlying key genetic and molecular features. These radiogenomic correlation between the imaging phenotype and genetic profile of tumor can play a pivotal role in a more comprehensive tumor classification that integrates histologic appearance of the tumor with canonical genetic alterations in addition to imaging phenotypes of the tumor observed on different imaging modalities. In addition, an exponential amount of imaging features can be extracted to detect the trivial and minute voxel-by-voxel changes occurring in the context of CNS tumors. The term radiomics is used to describe such high throughput extraction of imaging features. These features can be correlated with clinical outcomes to create robust prognostic predictive markers and can be further correlated to genetic and epigenetic alterations occurring in different tumors. This exponential amount of data can be easily integrated into big data analytics for a more precise and accurate prediction of tumor type, grade, and more importantly its genetic features. Such advances in the field of medical imaging and imaging analytics are anticipated to become cornerstone of future classifications of brain tumors.
The new 2016 central nervous system tumor classification
Multiple studies have been deployed in the past decade to unveil and decode the genetic encryption of different CNS tumors. These studies have revealed sets of key genetic alterations that direct and harmonize the development of specific tumors and represent efficient prognostic biomarkers. Therefore, the new 2016 CNS tumor classification incorporated these canonical genetic alterations with light microscopy features to refine tumor grading and improve diagnostic precision. Such integration paved the way for a more clinically sound grouping of clinically analogous tumors, as tumors that share the similar genetic drivers are expected to follow a common, if not similar, pathway with overlapping treatment response and subsequently common patient outcome. Although it is still unclear if tumor grouping and classification can depend solely on the basis of genotypical features, the new integrated phenotypic–genotypic diagnostic parameters are reflected as a number of newly recognized entities, variants, and patterns that will be discussed in the following sections.
Diffuse gliomas
On previous classifications, all astrocytic tumors were believed to share the same behavioral hierarchy and growth patterns and were thus grouped together. However, this grouping was modified on basis of IDH1 and IDH2 mutations, key driver mutations noticed in all diffusely infiltrating gliomas, including those with oligodendroglial phenotypical appearance and those with astrocytic phenotypical appearance. Such integrated grouping reflects on the variable aspects of better care via incorporation of both phenotypic and genotypic features, eventually leading to proper categorization of tumors with similar prognostic biomarkers and shepherding therapeutic plans for biogenetically analogous entities.
According to the new classification, diffuse gliomas include WHO grade II and III astrocytic tumors; WHO grade II and III oligodendroglioma; the grade IV glioblastoma (GBM), and diffuse gliomas of childhood. This reformatted pedigree chart of tumor classification ( Fig. 1 ) clearly distinguishes diffuse gliomas from other gliomas that are characterized by a circumscribed pattern of growth, including pilocytic astrocytoma, pleomorphic xanthrocytoma, as well as the subependymal giant cell astrocytoma. This highlights the fact that sharing a common progenitor cannot be the sole grouping variable in CNS Tumors, as one can see that a diffusely growing grade III astrocytoma would share more clinically meaningful features with grade III oligodendroglioma than a more circumscribed pilocytic astrocytoma.
Diffuse astrocytomas and anaplastic astrocytomas
According to the new WHO classification, 3 categories of astrocytoma are adopted depending on the key driver mutation of the IDH family of genes ( IDH1 , IDH2): IDH – wild type, IDH -mutant and not otherwise specific (NOS). Most diffuse astrocytomas and anaplastic astrocytomas are expected to belong to the IDH -mutant category. According to the WHO guidelines, mutation in the IDH family of genes requires immunohistochemical (IHC) staining for the R132H IDH1 protein in addition to sequencing for IDH1 codon 132 and IDH2 codon 172. Diagnosis of IDH -wild-type astrocytoma requires either tests to come back negative or at least negative gene sequencing. These testing check-points are essential to ensure accurate diagnosis of the uncommon diffuse astrocytoma and anaplastic astrocytoma with IDH -wild type genetic annotation. In cases of unavailable Genetic testing or indeterminate testing results (eg, negative IHC staining for IDH with unavailable sequencing), WHO classification opts toward a diagnosis of diffuse astrocytoma or anaplastic astrocytoma NOS.
Currently, the WHO classification limits the variants of diffuse astrocytomas to gemistocytic astrocytoma, removing both the protoplasmic astrocytoma and fibrillary astrocytoma from its diagnostic glossary. In addition, gliomatosis cerebri is no longer considered a distinct entity in the new classification but rather a growth pattern seen across IDH mutant astrocytic and oligodendroglial tumors as well as the IDH wild-type GBM.
Glioblastoma
A schematic classification of glioblastoma was proposed previously, in which key genetic mutations in PDGFRA, IDH1, EGFR, and NF1 stratified GBM into clinically relevant subtypes. However, the new WHO classification addressed only the IDH family mutation in its classification of GBM. The grade IV lethal tumor was classified into a majority of IDH wild-type GBM, representing 90% of cases with a minority that falls under IDH -mutant GBM category ( Fig. 2 ). IDH wild-type GBM was mostly associated with an older age of incidence and a predilection toward de novo or primary GBM. On the other hand, IDH -mutant GBM was closely associated with younger age ( Fig. 3 ) and the diagnosis of secondary GBM that arises on top of previous lower-grade glioma.
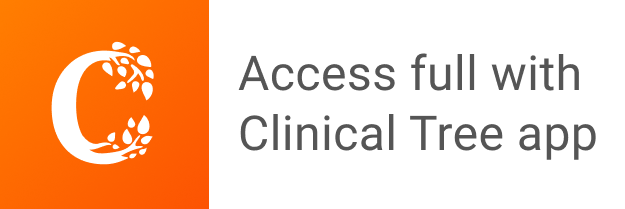