Fig. 11.1
The first recorded radiology report in 1896 by William Morton, MD
The report is a document in which the imaging modality used, the radiological findings and the diagnosis should be compulsorily incorporated. Moreover, the findings must be written in a concise but complete way and should answer the questions that originated the exploration. The methodology and style of writing the radiology report relies on the professional experience of the physician and his/her abilities in taking findings and correlating them with other clinical information, e.g. laboratory data or anatomical pathology data. This means that this methodology requires writing resources besides technical knowledge, as the traditional report, i.e. in free text, does not present any structure. This form of reporting is learned through the period of medical residencies.
Most hospitals decide to divide the report in sections: patient data, characteristics of examination and indications for the exploration, comparison with other studies (optional), findings and conclusions. In this sense, the Radiology Society of North America (RSNA) established an initial consensus regarding the content of the report (Table 11.1) [17].
Table 11.1
Components of the radiology report
Section | Contents |
---|---|
Administrative information | Imaging facility |
Referring provider | |
Date of exploration | |
Time of exploration | |
Patient identification | Name |
Identifier (e.g. social security number) | |
Birthdate | |
Gender | |
Clinical data | Medical history |
Risk factors | |
Allergies | |
Reason for exam, including clinical need | |
Imaging modality | Time of image acquisition |
Image equipment | |
Image acquisition parameters | |
Contrast materials and other drugs administered | |
Radiation dose (depends on modality) | |
Summary or impression | Key observations |
Inferences | |
Conclusions, including any recommendations | |
Signature | The date and time of electronic signature for each responsible provider, including attestation statement for physicians supervising trainees, if applicable |
However, this way of reporting is subjective and in some cases does not answer the clinical question or does not have an impact in improving patient care. Furthermore, there are many discrepancies between reports in clinical routine, even with the same professional and the same diagnosed disease. Jeffrey Sobel analysed 822 reports in 1996 and found that the radiologist used 14 terms for describing interstitial oedema/infiltration and 23 terms for the presence of an abnormality [2]. With the objective of solving this critical situation, Armas R.R. proposed in 1998 that an effective report should not contain abbreviations or neologisms [3]. The main drawback of the traditional way of reporting is that the radiologist is prone to fall in a stream-of-consciousness writing. That is, the physician makes a customization of the organization and content of the report for each case. This inherent variability in unstructured radiology reporting generates reports with different degrees of completeness and effectiveness. Armas enumerated the main properties of the report: clear, correct, concise, complete, consistent and confidence focused. Lafortune M. marked the steps to transform the radiology report in a clear and structured document in 1998 [4]:
The report should be useful to the requesting doctor and to the patient.
The report must answer the clinical reason for the benefit of the patient.
The text must be readable, comprehensible, brief and concise.
The report should avoid unnecessary long sentences and prolific language.
The report should consistently focus on important features of the case.
Since 1996 the scientific and radiological community has made an effort to structure the radiology report. Structuring the report may lead to a quicker diagnosis, improving the communication between radiologists and between radiologists and clinicians, increasing report completeness and effectiveness, reducing costs, and raising the satisfaction levels of the clinicians, and most importantly, the report will consistently focus on important features of the study case [5]. Studies in the last decade show that the radiologist and the clinician prefer structured reporting systems [6–9].
There are many initiatives to promote structured reporting, among which the project of the European Society of Radiology (ESR) and the RSNA (www.radreport.org) stands out. These institutions created a library with more than 200 report templates in English and approximately 50 templates translated into other languages. The templates try to serve as samples of “best practice” to lead radiologists through the process of report generation [17]. Moreover, each template includes metadata about the author, title, subject, brief description and date. The ESR and RSNA mapped the information in templates in standardized biomedical ontologies. The best example to illustrate structured reports is the Breast Imaging Reporting and Data System (BI-RADS), as the Food and Drug Administration of the United States (FDA) requires this system to be used for all mammography reports. This report has helped reduce variability in diagnostics and improved transparency in the communication between radiologists and clinicians [19].
Modern speech recognition software has popularized structured radiology thanks to its automation features [10–12]. Several speech recognition software packages allow creating fields that can contain text by default and/or that can contain a choice of possibilities for the radiologist to select [10]. This technology provides the chance to implement structured reports in radiology departments [12–15]. However, nowadays, there are few radiology departments where the structured report is the standard in clinical routine. In the words of Reiner, “adoption to date has been tepid” [16]. The strengths and weaknesses of structured reports will be assessed in depth in this chapter. In addition, we analyse which initiatives carry out this project.
Due to advances in technology, the way radiologists report and their work environment have changed in a drastic manner. Since the introduction of digital radiology terms like picture archiving and communication system (PACS) or radiology information system (RIS) have gained much importance in daily routine. Although this chapter does not intend to explain the information systems of a hospital in depth, we will briefly describe the different information systems that radiologists use.
The radiology information system (RIS) allows to maximize the resources available to carry out the examinations, and it also facilitates the introduction of patient data, the exploration scheduling and the patient care control and helps identify the professionals and radiologists who perform the exploration. The RIS also allows the interconnection with the digital radiology system and has to be flexible enough to connect to the hospital information system (HIS) and to the image storage system (PACS).
The PACS manages radiological images after they are acquired by any of its supported medical imaging machine types. It has two main functions: storing the images and sending them to the required workstation. The functional unit of a PACS is the study, which consists of one or more series, each formed by one or more images. The possibility of communication between all the devices that form a PACS is made possible by the standardization of products. Digital Imaging and Communications in Medicine (DICOM) is the standard in medical imaging [19]. This protocol defines the services that each equipment or device is able to implement, independently of the manufacturer. The most important feature of the PACS is the interaction and integration with the RIS. The integration between health information systems is achieved through information exchange protocols, for example, the well-known Health Level 7 (HL7) protocol [20].
Using this technology, engineers dedicated to healthcare ICT created the DICOM Structured Reporting (DICOM-SR). DICOM-SR is defined by how it is constructed more than by what it contains [21]. In this way, DICOM-SR structures data hierarchically into a tree of nodes. Each node has a concept name with a value. The concept name is coded from standard medical terminologies such as the Systematized Nomenclature of Medicine (SNOMED), Radiology Lexicon (RadLex) or Logical Observation Identifiers Names and Codes (LOINC), and its code is unique and language independent. Finally, the values of a node may be one of several types: text, numeric, concept coded or reference to other DICOM objects.
DICOM-SR can be simple documents, without the need to present the content into a human-readable form. Each document encodes only the content, but does not define how it has to be presented. However, it forces a restriction: the content must be unambiguous [22]. For this reason, DICOM-SR may be compared to Extensible Markup Language (XML). The XML files contain tags with meaning but without any form of presentation. So, following this parallelism, if the XML files need some presentation tool like Cascading Style Sheets (CSS), the DICOM-SR also needs an application to make its content legible.
With the objective of reducing variability, DICOM-SR includes the Information Object Definitions (IODs). Their function is similar to Document Type Definition (DTD) in XML files: to create “well-formed” documents. IODs specify the valid combinations of atomic components.
The benefits of using DICOM-SR are listed here:
Better communication with the clinician
More precise coding of the diagnosis, minimizing the number of refused reports
Less typing time
Automatic coding and language independent
Consistent and complete information
Reports stored next to the study images
References to regions of interest (ROI) (organs, tissues, etc.)
Automatic references to previous versions of the document
No need for dictation of patient data
Nowadays the DICOM Structured Report is still in research phase. Only the Radiation Dose Structured Report (DSR) is used in hospitals in daily routine to control the radiation dose administration in patients. Recently, Rosa Medina Garcia et al. published a paper detailing a system to diagnose breast cancer through the DICOM Structured Report [23]. The main reason for the lack of implementation of structured reports is the absence of support for these files in current PACS systems.
On the other hand, a new need arises due to the integration of imaging biomarkers in clinical routine. The final result should be able to be seen and reviewed on the available information systems by radiologists and clinicians [24]. Current PACS systems do not integrate a DICOM-SR viewer, so the extra information added in DICOM files can’t be exploited by the users. Moreover, there is no way of performing data mining on imaging biomarkers. A possible solution for this issue is the utilization of a framework like JasperReports or Crystal Reports that allow to create flexible reports [25, 26]. These frameworks provide a feature for creating customized templates, where the input data may be the imaging biomarkers extracted from a study. Supported input types include XML files, databases (e.g. MySQL, Oracle or PostgreSQL), JavaBeans or comma-separated values (CSV) files.
Thanks to these frameworks, a user with programming knowledge is able to automatically create documents in report form using JAVA or C# libraries. This way, the radiologist is able to review the extracted imaging biomarkers in a result report that can improve the final diagnostic, thanks to the added quantitative information. The final report will be human-readable and stored in the PACS system as a DICOM file associated to the corresponding study. In this chapter we will introduce a possible solution to create imaging biomarker reports.
11.2 Data Mining on an Unstructured Radiology Report
Radiology reports in free text are highly variable, so if we try to create a database to exploit the information provided in unstructured reports, the end result will probably be negligible and frustrating. A possible solution is based on the application of natural language processing techniques to perform an extraction of the information included in an unstructured report.
Radiology reports present a particular lexicon, such that the identification of specific terms in the text is of great importance to improve the performance of information recovery systems [25]. An important issue related with a radiology report is that the negation of medical terms is often used to indicate the absence of a certain disease or injury. In fact, in a radiology report more than half of the terms used are negated, i.e. the radiologist uses the negation to indicate a healthy condition of the tissue. In this context, standard techniques of natural language processing fail to recover the required information in an efficient manner. Research groups work with negation recognition systems to solve this difficulty in other types of text with a similar problem than the one found in radiology reports [26]. Another important need for the natural language system is to assign weights to the most critical and important words in the report, using a dictionary of typical terms. This dictionary is restricted to the terms used by radiologists.
Using these techniques, we can develop systems with an efficient processing engine and report indexing. Natural language systems allow to manage the high number of radiology reports generated daily by a hospital without affecting the system performance. Additionally, to the indexing process, the extraction of statistical information and analysis results should also be included in order to perform a quality control of the data and the structured reports introduced into the system.
A prototype system has been implemented at La Fe Polytechnics and University Hospital in Valencia. This solution uses the extracted RIS reports as inputs. The reports are analysed by the system and stored it in a customized database. To visualize the results, a web application with a similar interface as Google search is used. This application allows searching by simple words or by concatenated words in the reports. The results show a list of reports that contains the terms of the search. Recursively, the user can indicate the most significant reports to refine the search. The final result list can be downloaded as a CSV file.
However, natural language processing suffers from limitations when performing data mining on radiology reports. There are various reasons. First, as we indicated above, there is the issue of negation [28, 29]. This is a problem that has been widely studied, but it is not a trivial one. Scientific literature indicates that a 90 % of sensitivity and specificity can be achieved [27]. Unfortunately, these rates are insufficient in clinical care. Second, natural language processing depends on the nature of the data. And third, natural language processing requires a synonym vocabulary to search efficiently.
11.3 Structured Report
According to Weiss and Langlotz, there are three structured report types [10]. The first model is written in free text, but it is split into sections; nowadays this is the most widely used model. The second type is modelled with templates with a highlighted format; a sample is proposed by Naik et al. [6]. The third type of report uses a standardized language or lexicons, such as RadLex does. This type of report is possible thanks to technologies such as DICOM. Apart from these types, the RSNA promotes their recommended best practices to adapt the structured report to each individual or to each centre [17, 30]. In this section of the chapter, we focus on the DICOM structured report and on the template-dependent structured report.
The structured report offers many benefits. First, high quality and accuracy (a critical point) as it reduces ambiguity and variability in terms with multiple definitions [13]. Second, a structured report is able to help research, quality improvement and clinical decision support. Third, immediate information accessibility, automation and exchange between centres in order to optimize teleradiology. Fourth, the structured report facilitates the communication between radiologist and clinician, due to the fact that the clinician is able to distinguish essential from secondary information [45]. Fifth, thanks to a systemised approach, the structured report prevents discovering only one lesion and omitting the rest. It helps improving the general view of patient care.
Step by step, the structured report is gaining acceptance in clinical routine, but we can’t turn a blind eye to the principal obstacles that prevent its definite implantation. The main problem is resistance to change. The time and energy required to learn and a positive attitude towards change are essential, but not always found, especially in radiologists of advanced age [13]. A higher accuracy is usually an indicator of structured reporting, but authors have questioned this assertion [37]. In fact, the largest study on structured reporting found that it suffered from a lower accuracy compared to the traditional report [7]. However, this might be partly due to limitations of the reporting system used. Another potential problem is the negative impact in the radiologist workflow. The creation of a structured report requires an increased visual attention, so the radiologist has less time to spend seeing and analysing the study images, which may disrupt the diagnosis of lesions or pathologies [10]. Furthermore, it may be difficult to summarize or provide an overview when facing complex diseases, due to the fact that structured radiology reports are split into several sections.
An important drawback of the actual structured report systems, from the point of view of the radiologist, is that it is more laborious to generate a structured report than a traditional one, leaving less time for the radiologist to examine the study images, and leading to a lower efficiency. In this sense, Sistrom and Honeyman-Buck demonstrated that the structured report and the report with free text show similar efficiency and accuracy rates for transmitting case-specific interpretative content [31]. An important aspect to keep in mind is that radiologists and clinicians must be involved in the creation of templates for structured reports [32]. Therefore, the structured reporting systems are not yet widely available in PACS or radiologist’s workstations.
Some authors reported that the use of checklists may improve the accuracy of the report when using templates [33]. Anyway, there are different solutions with a different degree of restriction to create structured report systems using templates [33–35]. However, some authors indicate that the restriction level of a template depends on the diagnostic of the reported disease [36, 37]. One critical concern that radiologists have about structured reports is that they may result in a vast data increase in the information systems. However, due to all information being coded into unique fields, the reports can be indexed. This way the computational load can be reduced. On the other hand, information generated from unstructured reports does not allow any form of indexing, which implies an excessive computational load for the information system servers.
The American College of Radiology (ACR) has promoted a system over the past few decades for indexing the images and cases collected by radiologists. The ACR Index presents attractive features for image indexing with the purpose of using them as teaching materials. First, this index offers anatomic and pathologic identifiers using decimal numbers. In a very simple way, the numbers before the decimal point indicate the anatomic location, and the numbers after the decimal point indicate the pathologic entity. Second, the ACR Index is human-readable. Each digit denotes a specific term in the taxonomy. Third, the code scheme employed by the ACR Index ensures that teaching materials are not modified by radiologists or institutions. Due to the Internet and its capabilities, the teaching materials have now an online version. Lexicons like the Systematized Nomenclature of Medicine-Clinical Terms (SNOMED-CT) can be used to organize the information in electronic medical records with a more extensive amount of terms. The ACR Index contains only a few thousand unique terms, offering much less detail than other terminology systems [38].
The RSNA generated the Medical Imaging Resource Community (MIRC) project, offering an online tool for the creation of electronic teaching files and other forms of image libraries. Moreover, the user can annotate information to the images. This way, a need arises for a more complete and computerized index than the original ACR Index. RadLex was developed by the RSNA to fill these gaps, with the purpose of creating a complete terminology on medical imaging. RadLex’ main goal is to create a terminology that can be used to annotate, index and retrieve content from MIRC. To avoid useless efforts, the RSNA and the College of American Pathologists agree to use SNOMED-CT terms as a starting point for the lexicon. Furthermore, various standards organizations, such as DICOM or Integrating the Healthcare Enterprise (IHE) have participated in the RadLex project [22, 39].
RadLex includes the anatomic and pathologic codes available in the ACR Index. In addition, it also integrates other types of terms: equipment, procedures and imaging techniques used in image acquisition, difficulty of image interpretation and image quality. Other important advantage of RadLex is the possibility of updating with new concepts, including other popular medical lexicons, such as SNOMED-CT, International Classification of Diseases (ICD)-9, Current Procedural Terminology (CPT) or BrainInfo. Nowadays, RadLex includes more than 68,000 English terms, which are available in a variety of forms: table format (downloaded directly to an Excel file), Ontology Web Language (OWL) files and as a database. RadLex terms also are available online or using the MIRC authoring tool. The RadLex project must be continuously updated to guarantee that new concepts are incorporated and to maintain the cross-links with other vocabularies.
All the information included in a report cannot be captured by a standardized lexicon. Radiologists need a narrative text to express unusual elements, to integrate a large and complex set of observations or to describe parts of the image that may not be relevant to patient care at that moment, but that should still be documented. Therefore, this is a feature that should be integrated into any reporting software. In addition to potential improvements in the quality of care, the use of lexicons has other practical advantages. The lexicons allow us to avoid different interpretations and ambiguities, since each code referring to a concept has a specific meaning for a particular coding. Hence, coding is essential for a subsequent exploitation, avoiding ambiguous interpretations that can lead to confusion. Yi Hong et al. analysed the frequency use of RadLex terms employed by radiologists when using radiology reports based on templates [44]. About 2,509 unique reporting elements were extracted from a list of 70 reports, and they were afterwards matched with RadLex. The results indicated that there was a 41 % of perfect correlation and a 26 % of partial correlation and that 33 % of the terms were uncorrelated to RadLex. Using multidimensional scaling analysis (MDS), it was discovered that 13 % of the 33 % that represented the unmatched terms were combinations of two or more RadLex terms. So, it was demonstrated that a significant overlap exists between terms of structured reports using templates and the RadLex.
DICOM is the adopted standard, and it is used in most radiology departments in hospitals. Due to the growth in new medicine areas, DICOM has expanded its standard to meet the new needs created. One of the latest additions to the DICOM standard is including structured reports using DICOM-SR. This type of DICOM file allows adding semantic information to medical images.
DICOM-SR can be used in areas with high heterogeneity and with very different data types. For this reason, a generic specification for structured reports is highly complex. The information in a structured report requires a pattern to describe exhaustively the casuistry of the radiology report. This point is fundamental: the structure of the report must be subject to the final user needs. For example, the radiologist detects and evaluates findings on medical images, and the traumatologist assesses the need of a surgical intervention. In addition to structuring, the unification of terms that defines the information in a report can be different and complex.
The coding of concepts can help improve the structure of reports. In this sense, there are many different codes and tools that meet different needs. The most widely used ones are the ICD-9 and ICD-10 (International Classification of Diseases), which are lexicons to code diagnosis and procedures; the Systematized Nomenclature of Medicine (SNOMED); the Logical Observation Identifier Names and Codes (LOINC), a database created with the purpose of facilitating the exchange and development of a result pool; and RadLex, explained previously in detail. The great diversity of lexicons adds to the complexity of unifying concepts in a standard way in order to generate the information from a DICOM tag value.
The DICOM standard includes a code to generate structured reports in the medical field. DICOM Structured Reporting (DICOM-SR) allows the integration of the most important lexicons in medical imaging, and also it allows the inclusion of customized lexicons [22]. DICOM-SR itself establishes the guidelines to follow when preparing a DICOM structured document, and it allows a standard coding of information on the report in DICOM format (sets of “Data Elements” that match a given IOD).
DICOM-SR is able to structure semantic information. The representation of a DICOM-SR will be defined by the IODs; in DICOM-SR there are only three specified types of IODs that define a structured document: Basic Text, Enhanced SR and Comprehensive SR. DICOM-SR objects include a tree structure, which represent the structured report information as “Data Elements”. This tree structure is known as the “SR Document Content” and contains the definition of the structured report. The “SR Document Content” has a tree structure in which each node keeps a relationship with its parent node and has a defined data type, as shown in Fig. 11.2. This figure shows an “SR Document Content” that describes the discovery of a round malignant tumour of 1.5 cm.
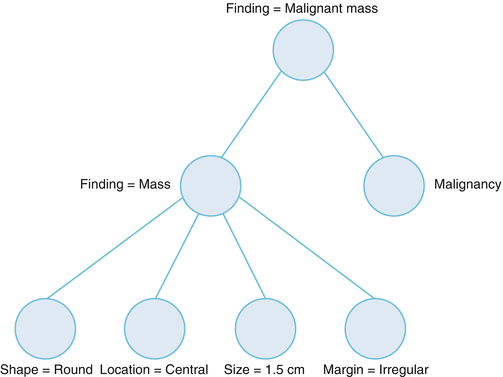
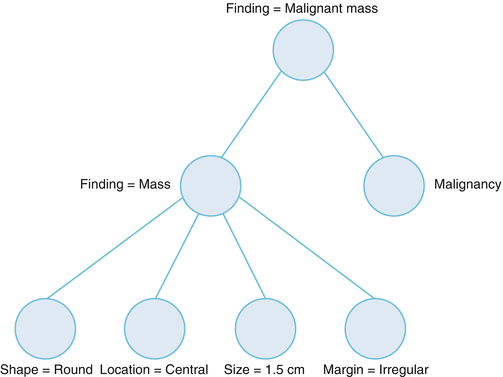
Fig. 11.2
General structure in a content tree of DICOM-SR
Moreover, the “SR Document Content” can have different structures depending on the use of DICOM-SR. There are templates for DICOM-SR that define the needed structures and constraints, such as lexicons included or the relationship between nodes. DICOM-SR also offers the possibility of validating the structures determined by these templates, as it is done with XML schemas. The templates are defined in the DICOM standard. These templates are in the process of specification and are not yet completed. At present, there are works underway to migrate DICOM-SR documents to XML and also for generating XML schemas from DICOM-SR templates [40, 41].
Therefore, DICOM-SR is a useful tool to generate structured reports because it fits perfectly into the DICOM world. It allows generation, processing and validation of these reports in a simple manner using current XML-based technologies. A structured DICOM-SR document can be encoded into XML documents, so that the way of handling and using of these documents will not be different from any XML document.
The Health Level Seven (HL7) standard is also included in its standard natively, using a structured document management called Clinical Data Architecture (CDA) [20, 42]. This format was incorporated in 1997 to structure the semantic content of clinical documents. All content of a CDA is defined through the coding of information using the XML standard. However, DICOM-SR is a complementary approach to structured documents for HL7, because currently HL7 has not proposed a rigorous structure with CDAs, i.e. it does not include templates for defining a structured report.
Another important aspect to consider is measurements taken by a radiologist from medical images using the PACS viewer. These annotations and measurements provide critical information to support the observations in the report. With the purpose of integrating them into DICOM-SR and to avoid expressions like “5 cm mass found, best seen in image 65”, the National Cancer Institute’s Cancer Biomedical Informatics Grid (caBIG) launched an initiative called Annotation and Image Markup (AIM) [43]. With AIM, the radiologist is able to specify what type of information he has captured when making an annotation or drawing a shape. AIM saves the position of the regions of interest (ROIs), geometry properties, anatomic entities, image observations and calculations. AIM provides a means to add this information to DICOM-SR or XML files, so it can be included directly or indirectly in structured reports.
The Breast Imaging Reporting and Data System (BI-RADS) is the best example of structured report radiology. BI-RADS includes a limited range of breast diseases, which is a good practice, and it is well suited for structured reporting. BI-RADS has five editions: 1993, 1995, 1998, 2003 and 2013 [46–50]. There are specific guides for mammography, ultrasound and magnetic resonance. The most important improvement is a better clarity and consistency in reporting, improving patient care and clinical practice. The Institute of Medicine in its 2005 report recognized that BI-RADS assessment provides an important tool for diagnosing mammography [50]. Moreover, the standardized language aids in the education of resident radiologists, also offering a more consistent practice routine [51]. The structure of BI-RADS is designed for coherent and rational examination of mammographic findings. These properties facilitate the resident training. Basset et al. indicated that 98 % of radiology residents of the United States and Canada used BI-RADS in their mammographic reports [52]. Scientific papers show that BI-RADS training can decrease variability and improve performance in spite of interobserver variability due to heterogeneity of recommendations and disease categories [52–58]. Mammography research has increased thanks to the structured report for mammography evaluation proposed by BI-RADS [18].
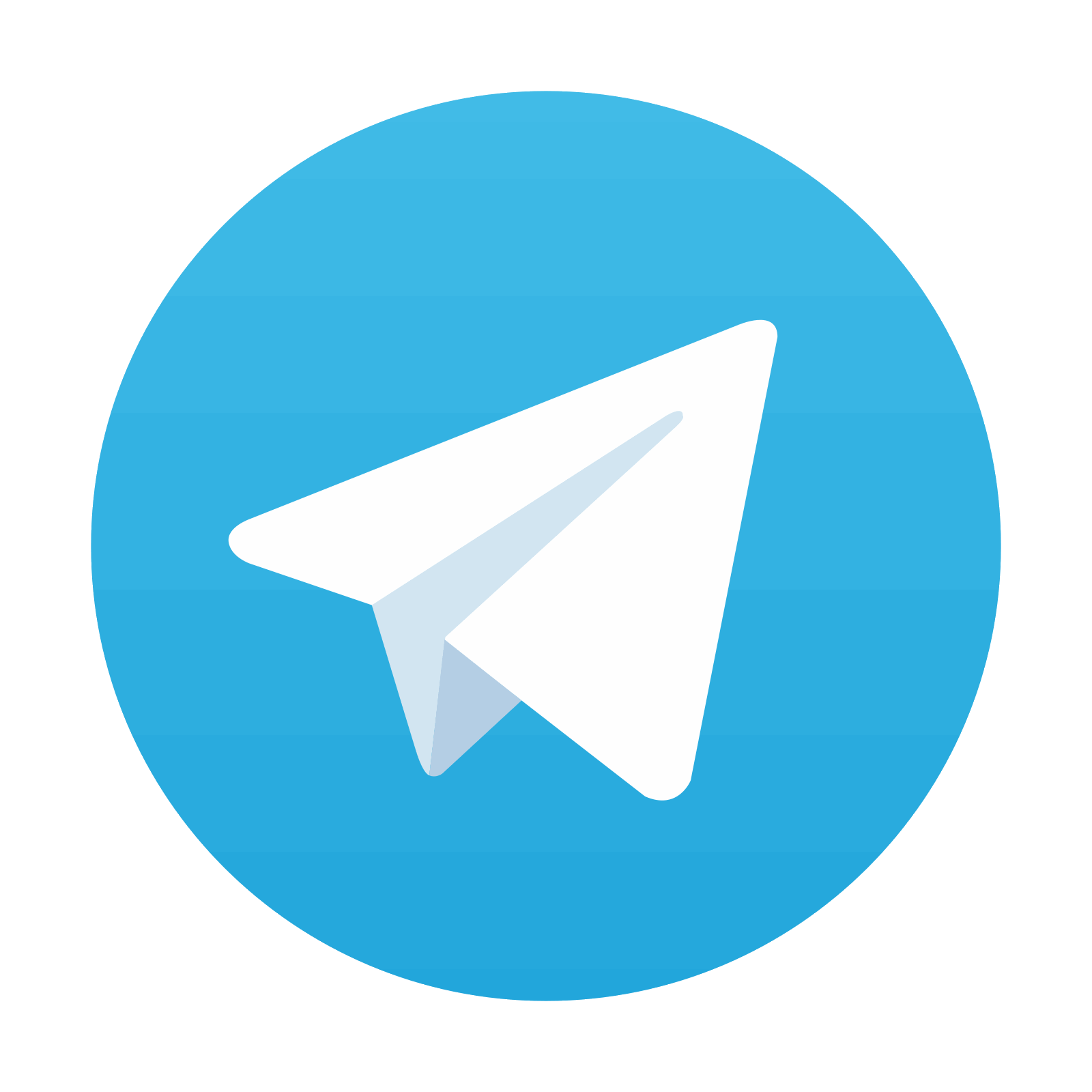
Stay updated, free articles. Join our Telegram channel

Full access? Get Clinical Tree
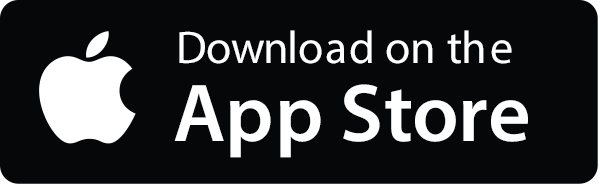
