Fig. 19.1
Density analysis of the proximal femur with QCT. (a) Coronal cross section where neck and trochanteric voxels have been highlighted in white. (b) Cortical (green contours) and trabecular (red voxels) compartments. The black vertical line indicates the femoral neck axis, while the black horizontal line divides the femoral neck and trochanteric subvolumes [1]
19.3.1.2 Bone Geometry
Bones break when applied forces overcome their strength. Bone resistance to fracture is dictated by its geometry, distribution of material properties, and magnitude and direction of the applied forces. Measures of bone geometry in the proximal femur include minimum femoral neck cross-sectional area, estimates of cortical bone volume, moments of inertia for strength estimation, and cortical bone thickness (Fig. 19.2) [1].
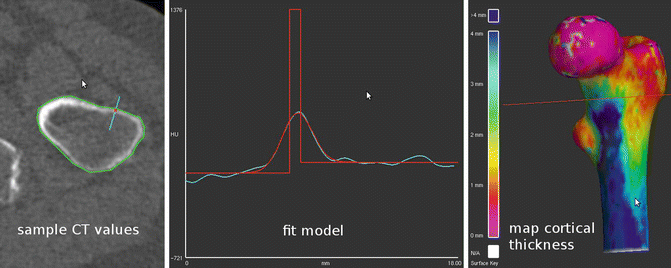
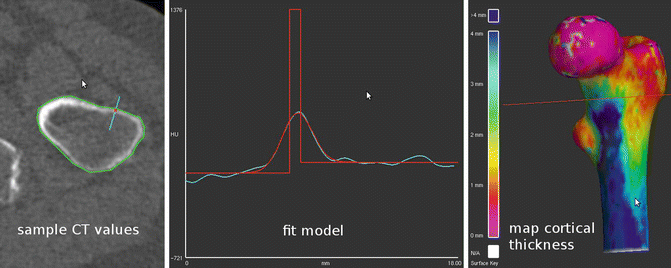
Fig. 19.2
Estimation of cortical bone thickness from QCTs of the proximal femur is performed in four steps: (1) bone segmentation (green contour), (2) sampling of CT values along lines perpendicular to the cortical bone (cyan), (3) model fitting, and (4) mapping of estimated thickness values to the femoral surfaces [25]
19.3.1.3 Finite Element Modeling (FEM)
FEM is a numerical engineering technique where the performance of a structure is studied under different loading conditions. In FEM, the complex 3D geometry and distribution of material properties play essential roles in the mathematical model. For this purpose, FEM subdivides the structure into many small elements that are referred as finite elements. Then material properties are assigned to each finite element and loading conditions are mathematically applied in the form of displacements or forces. The strength of the structure under specific loading conditions is then estimated based on material failure criteria, stress, and strain. Stress refers to an applied force or a system of forces that tends to strain or deform an object, while strain refers to a deformation produced by stress.
Studies have demonstrated that bone strength as measured on cadaveric bones can be best estimated by combining QCT imaging and subject-specific FEM [26–28]. Medical imaging has therefore recently incorporated FEM as a valuable in vivo tool to estimate material strength. Since QCT enables an accurate 3D representation of bone geometry as well as the derivation of material properties based on vBMD values, the use of QCT images of the proximal femur and spine has dramatically increased in recent years to estimate bone strength using FEM [29]. In studies involving the spine, uniform compression loading is the most common clinical loading condition [30], while in studies of the proximal femur, single-limb stance and a fall to the side with an impact on the posterolateral or lateral aspect of the greater trochanter are the most common loading conditions [31]. The most common parameter reported in FEM analyses is failure load (N). Figure 19.3 illustrates a subject-specific FEM example of the proximal femur derived from QCT.
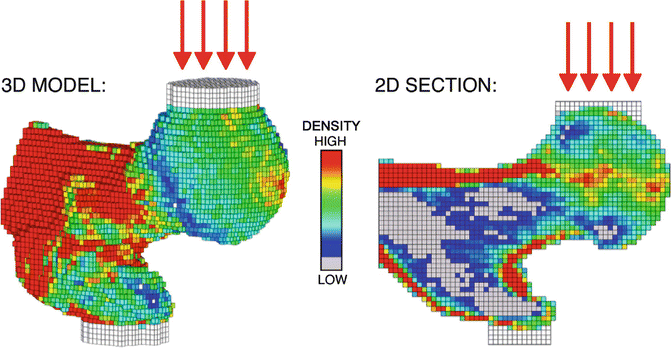
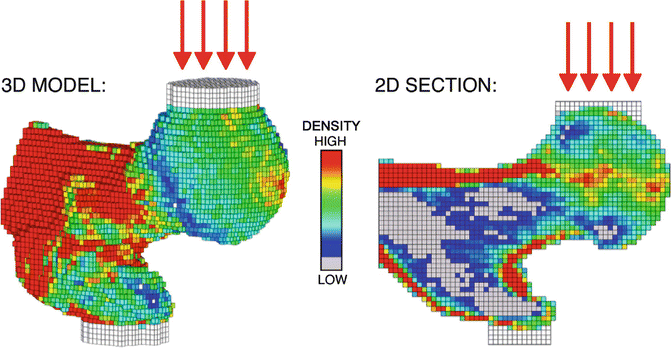
Fig. 19.3
Full 3D and coronal cross section of a FEM model of the proximal femur simulating a sideways fall. Each cube represents a single finite element with isotropic size 1.5 mm3 [32]
19.3.1.4 Muscle
While the majority of osteoporosis studies have been focused on bone assessment, there has been a recent interest toward surrogate imaging biomarkers of muscle strength. Smaller cross-sectional thigh muscle area—a measure of muscle size—and greater fat infiltration have been associated with increased risk of mobility loss in older men and women, suggesting that the association between low muscle mass and functional decline could be a function of muscle strength [33]. In QCT, inter- and intracellular adipose content of the muscles is primarily measured based on Hounsfield units. Lower Hounsfield units are indicative of higher fat infiltration [16]. Four muscle groups that are within the FOV of QCT acquisitions of the proximal femur are illustrated in Fig. 19.4.
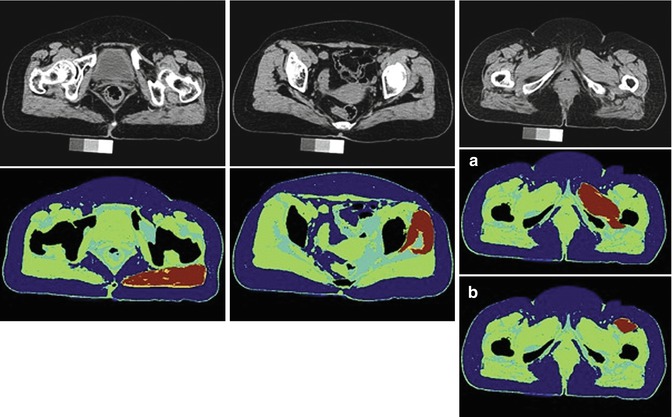
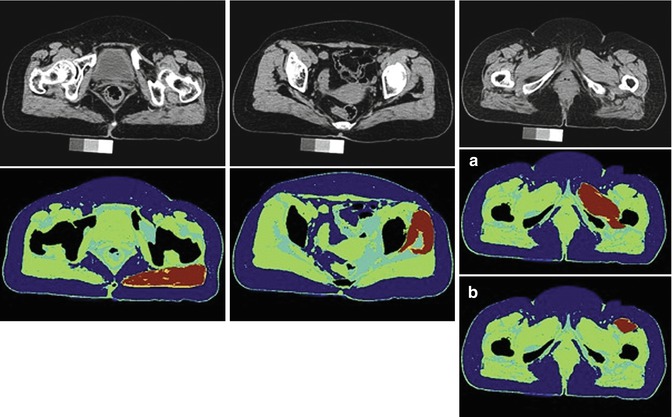
Fig. 19.4
Muscle groups commonly studied with QCTs of the proximal femur are highlighted in red. Left: hip extensors; middle: hip adductors; right-a: hip adductors; and right-b: hip flexors [1]
Image Analysis
Conventional vBMD analyses in both the spine and the proximal femur are based on summary statistics. In the spine, the lumbar level is the most studied in osteoporosis research. For most of the QCT analyses, the periosteal contours of the vertebral bodies and the proximal femora must be delineated. This process has been commonly accomplished automatically for the lumbar spine [34] and semi-automatically for the proximal femur [35]. However, recent advances in medical image segmentation algorithms have now enabled the automatic segmentation and multiparametric assessments of proximal femora with QCT [21]. In density analyses, once the outer bone surfaces have been identified, the cortical and trabecular bone compartments are separated and subdivided into subregions from which mean and standard deviation values of cortical and trabecular vBMD are computed. Because the femoral neck is a common fracture site, this anatomic region has been further subdivided into smaller regions with the aim of providing a better understanding of the association of the spatial distribution of vBMD and hip fracture [36, 37].
In conventional vBMD analyses, regions of interest are prescribed based on prior assumptions or hypotheses, and summary statistics discard relevant spatial information. For this reason, new population-based image analyses techniques previously developed for neuroimaging studies have been adapted to musculoskeletal applications. In particular, voxel-based morphometry (VBM) is a technique that enables the identification of bone regions where vBMD is significantly associated with a variable of interest [38, 39]. VBM is a data-driven technique that does not require specification of regions of interest and performs an automatic unbiased assessment of the whole structure. Another technique that makes no assumptions about the spatial association of vBMD with a variable of interest is statistical shape and intensity modeling. This technique is based on principal component analysis to describe each anatomical structure in terms of its associations with principal modes of shape and intensity [40].
Most of the studies have estimated cortical bone thickness in femoral neck cross sections or in the entire femoral neck region using thresholding or the 50 % relative threshold method and repored summary statistics, i.e., mean and standard deviation values. Since fractures usually initiate in the cortical bone, which has been observed to become thinner with aging, the assessment of cortical bone thickness has become quite relevant in recent years. Therefore, new methods have been developed toward not only the accurate [41, 42] and reproducible [21] quantification of cortical bone thickness but also toward the quantification of its spatial distribution across the entire proximal femur [21, 41, 42]. In addition, statistical parametric mapping (SPM) techniques similar to VBM now enable the spatial comparisons of cortical bone thickness and other cortical bone properties at corresponding anatomical locations between populations [21, 25, 41]. SPM thus allows the automatic identification of regions in the bone surface where cortical bone parameters, e.g., thickness or cortical vBMD, are significantly associated with a variable of interest.
Brain image analysis techniques investigating local differences in shape between two populations such as tensor-based morphometry (TBM) have also been adapted to musculoskeletal applications in order to study internal and external structural differences [43].
QCT-based subject-specific FEM is a complex engineering technique, which limits its widespread use even across research institutions. Most of the current FEM approaches are nonparametric and require significant amount of time. For this reason, a parametric FEM approach based on statistical shape and intensity modeling derived from clinical QCT data of proximal femora was recently proposed. From this model a 3D FEM of a new QCT scan can then be derived with high levels of accuracy [44].
Currently, image analyses of muscle size and composition in QCT studies of the proximal femur have not gone beyond summary statistics [16].
19.3.2 HR-pQCT
HR-pQCT is a relatively novel medical imaging modality aimed for the in vivo imaging of bone microstructure in the extremities, particularly the distal radius and distal tibia. Although the manufacturing company producing these devices (XtremeCT; Scanco Medical, Brüttisellen, Switzerland) has recently released the second generation of these scanners, which allow faster acquisition times, smaller voxel sizes [45] and enable imaging of the knee joint in certain populations, most of the studies in the literature have used first generation scanners and were based on the standard acquisition protocol provided by the manufacturer.
In the standard acquisition protocol, the extremity of a subject is immobilized using a carbon fiber cast that is fixed within the gantry of the scanner. Then an anterior–posterior projection is acquired which is used to plan the 3D acquisition. In the scout view, the operator manually places a reference line at the radial or tibial endplate, and the scanner acquires a slab of 9.02 mm consisting of 110 slices with isotropic voxel sizes of 82 μm. The slab is acquired at 9.5 and 22.5 mm proximal from the reference line and extends proximally for the radius and tibia, respectively. Scans take in the order of 3 min with an effective patient dose per scan in the order of 3 μSv. The standard protocol provided by the manufacturer also incorporates techniques to identify the periosteal and endosteal boundaries, thus enabling compartmental analyses [4].
19.3.2.1 vBMD
Similar to QCT acquisitions, a calibration phantom is scanned simultaneously with the patient in order to convert gray-scale attenuation values to equivalent concentrations of hydroxyapatite (HA). The phantom consists of five cylinders of HA-resin mixtures with the following concentrations: 0, 100, 200, 400, and 800 mgHA/cm3 [46]. Representative axial cross sections of acquisitions of the distal tibia and distal radius are shown in Fig. 19.5.
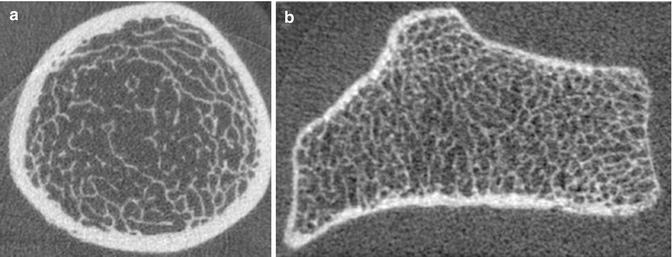
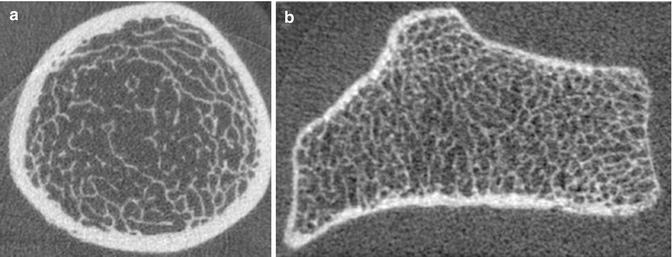
Fig. 19.5
Representative cross sections of HR-pQCT scans of the distal tibia (a) and distal radius (b) of postmenopausal women [47]
19.3.2.2 Trabecular Bone Microstructure
Trabecular bone structure is assessed based on binary images representing the trabecular bone network and with parameters equivalent to those of histomorphometry studies. The binary images are usually obtained with the software provided by the manufacturer using a Laplace–Hamming filter and a fixed threshold, although alternative approaches have also been developed. Common trabecular bone parameters include trabecular bone volume fraction (BV/TV), trabecular bone number (Tb.N; 1/mm), trabecular bone spacing (Tb.Sp; mm), and trabecular bone thickness (Tb.Th; mm). Trabecular bone number (Tb.N) is computed in three steps. In the first step, a mid-axis transformation is applied to extract trabecular bone ridges. In the second step, a distance transform method is used to create a map of distances between ridges. In the final step, the average of the distances over all interridge voxels is calculated and its inverse defined as Tb.N. Bone volume fraction is also computed directly; however, it is based on vBMD and the assumption that fully mineralized bone has a density of 1200 mgHA/cm3. Tb.Sp and Tb.Th are then derived from BV/TV and Tb.N using plate model assumptions [48].
19.3.2.3 Cortical Bone Structure
The most common cortical bone parameter assessed in HR-pQCT acquisitions is cortical bone thickness, which can be derived or measured directly. The derived measure of cortical bone thickness is based on the ratio of cortical bone volume and the outer bone surface, while the direct assessment is based on the distance transform of the cortical bone region.
A relatively new assessment of cortical bone structure is cortical porosity (Fig. 19.6). The algorithm provided by the manufacturer is based on the segmentation of cortical bone and the identification of cortical voids. Cortical porosity is then defined as the ratio of the volume of the cortical voids, i.e., intracortical pore volume (Ct.Po.V) and the sum of the mineralized and intracortical pore volume. Additional pore parameters include a cortical pore diameter and the distribution of cortical pore diameters [49].
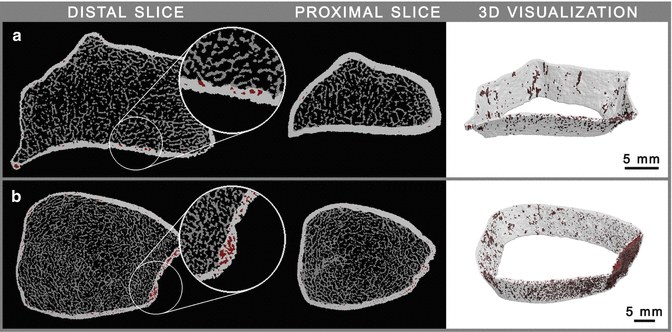
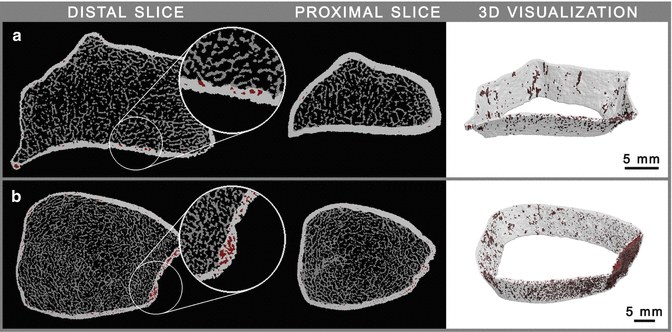
Fig. 19.6
Cross sections of a radius (a) and tibia (b) where the cortical bone was color-coded in white, trabecular bone in gray, and intracortical porosity in red. On the right side, 3D visualizations of the intracortical porosity (red) are shown with a semitransparent cortical bone [49]
19.3.2.4 Micro-finite Element Modeling (μFEM)
HR-pQCT also enables the estimation of bone strength through micro-finite element analysis. In these simulations, binary images representing the bone structure are converted into meshes of isotropic bone elements using voxel conversion techniques. Although material properties can be applied according to X-ray attenuation values in a similar way as in QCT, bone elements are commonly assigned homogeneous elastic moduli. An advantage of the small voxel sizes is that the cortical and trabecular bone compartments can be labeled as two different materials with identical material properties, thus facilitating compartmental analyses. The most common μFEM configuration for both anatomical sites is a uniaxial compression to 1 % strain. The most common imaging biomarkers of bone strength that are reported are bone stiffness (N/mm) and failure load (N) [50]. Figure 19.7 illustrates the loading conditions as well as representative boundary conditions and distributions of von Mises stresses for the tibia.
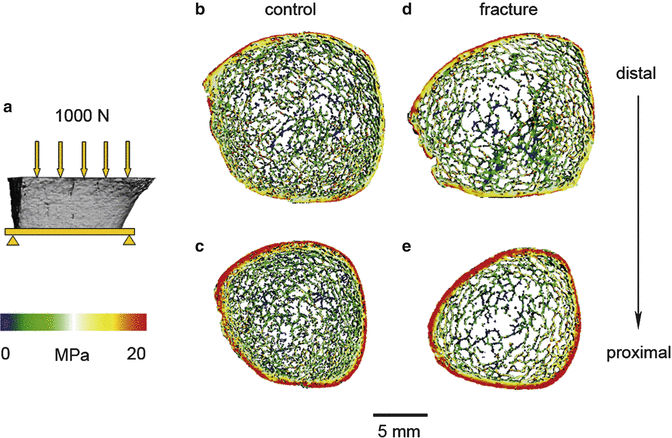
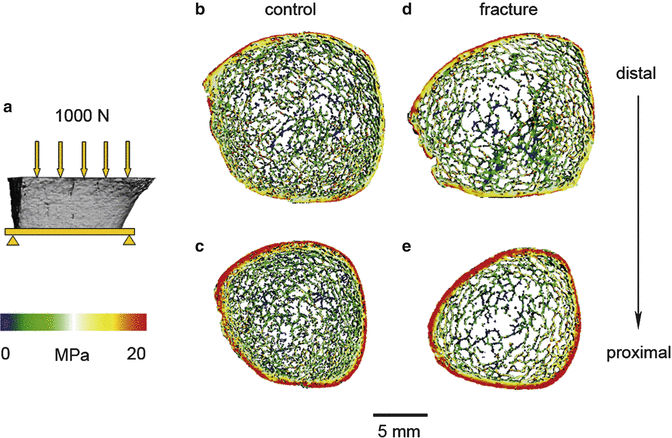
Fig. 19.7
Micro-finite element modeling of the distal tibia. (a) Axial compression with a 1000 N load applied to the first distal slice while the proximal surface was fully constrained. The boundary conditions and distributions of von Mises stresses for a control (b–c), and a fracture case (d–e) are shown where the level of stress has been color-coded, with red representing high stresses and blue low stresses [51]
Image Analysis
Most of the HR-pQCT studies have been based on summary statistics of cortical and trabecular bone parameters. However, some studies have subdivided the distal radius and distal tibia into subregions with the aim of incorporating some spatial information into the statistics to increase sensitivity in the analyses [52, 53]. Recent algorithms have also been developed to investigate the different cortical porosity phenotypes using 2D laminar analyses of cortical bone [54].
Initial indications of the adaptation of 3D data-driven techniques such as VBM, TBM and SPM to HR-pQCT have been observed in the literature [55]. These techniques will enable population-based comparisons to automatically identify regions where bone parameters are significantly associated with variables of interest.
19.4 Knee Osteoarthritis
Knee osteoarthritis is a condition characterized by pain, functional impairment, and biochemical and structural changes in articular tissue. The increasing elderly population and sports-related injuries have made knee osteoarthritis a leading cause of chronic disability. Despite this, knee osteoarthritis is still poorly understood and few therapeutic options are available. The current surrogate marker for demonstrating structural changes by regulatory agencies is joint space narrowing on weight-bearing radiographs. However, knee osteoarthritis is a disease of the whole joint, and in order to investigate the etiology of knee osteoarthritis, and consequently improve the development of disease modifying osteoarthritis drugs, investigation of all the relevant tissues involved in this disease should be performed.
19.4.1 MRI
MRI is the medical imaging modality of choice to perform studies of knee osteoarthritis [6]. MRI offers in vivo imaging characteristics that cannot be matched with current medical imaging technology such as non-ionizing radiation, tomographic capabilities along practically any orientation, high spatial resolution, high soft-tissue contrast, and unique capabilities to perform quantitative compositional and functional imaging. Furthermore, MRI is perhaps the medical imaging modality that moves faster technologically speaking, thus constantly offering new imaging and quantification approaches. MRI has therefore been extensively used in studies of knee osteoarthritis. While most of the studies have been focused on the morphology and composition of the knee cartilage, current studies are also looking into other joint tissues such as the bone, meniscus, and muscle, as well as into the hip joint.
19.4.1.1 Cartilage
Cartilage thinning and loss are common manifestations of knee osteoarthritis; therefore there has been substantial interest into the 3D morphological quantification of knee cartilage. Cartilage morphology has been primarily assessed in the form of volume and thickness using high-spatial-resolution images where cartilage signal is bright and bone signal is low [56]. This type of contrast can now be achieved with a variety of pulse sequences. While the majority of previous studies were done in the sagittal plane, current advances in MRI hardware and pulse sequence development allow the acquisition of high-spatial-resolution images with (quasi)-isotropic voxels thus enabling reformations into other planes without compromising image characteristics [57].
Unfortunately, morphological changes of cartilage are small and slow. In addition, recent studies have suggested that cartilage undergoes compositional changes prior to morphological events [5]. MRI has then been used to study cartilage composition based on the quantification of T2 (Fig. 19.8), T1ρ, and T1Gd relaxation times [7], which have been associated with different cartilage properties, therefore emerging as potential in vivo noninvasive imaging biomarkers for early detection of knee osteoarthritis. T2 relaxation times have been associated with tissue hydration and biochemical composition, specifically the integrity of the collagen matrix. T1ρ relaxation times describe the spin–lattice relaxation in the rotating frame and have been associated with changes in proteoglycan loss—a manifestation of early knee osteoarthritis. The rationale is that pulse sequences for T1ρ quantification were designed with the idea of providing biochemical information in the low-frequency regime (few hundred Hz to few kHz), thus interrogating the slow interactions in the extracellular matrix between motion-restricted water molecules, proteoglycan, and collagen. T1Gd refers to the T1 relaxation time of the tissue after intravenous injection of Gd-DTPA2−, which is a charged contrast agent that is absorbed in articular cartilage. This technique is known as delayed gadolinium-enhanced MRI of cartilage (dGEMRIC) and has been used to investigate the distribution and changes in glycosaminoglycan concentrations that occur early in knee osteoarthritis [58]. In order to calculate relaxation times, multiple MRI acquisitions are performed at different echo times (T2), spin-lock times (T1ρ), and inversion delay times (T1Gd). Then voxel-wise exponential fittings are performed according to the relaxation time in question, thus yielding maps depicting the spatial distribution of T2, T1ρ, or T1Gd. Other approaches to quantify cartilage composition and structure include gagCEST, sodium MRI, and diffusion tensor imaging [9, 59].
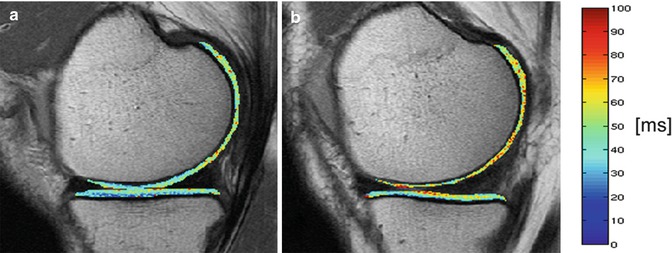
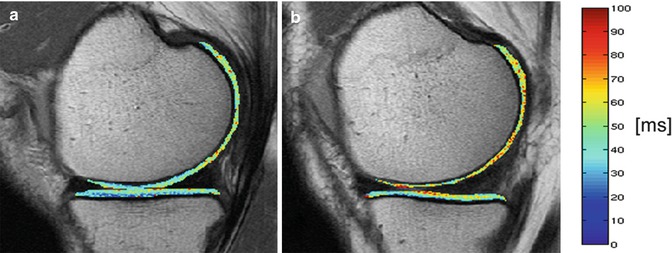
Fig. 19.8
Representative T2 relaxation time maps of the medial femur and medial tibia of a subject without knee pain (a) and a subject with knee pain (b) [60]
19.4.1.2 Meniscus
The relevance of the menisci in the biomechanics of the knee joint is clearly acknowledged. Therefore, it has been suggested that meniscal damage may be associated with onset and progression of knee osteoarthritis [61–63]. Because cartilage has been the dominant tissue investigated in knee osteoarthritis using MRI, studies focused on meniscal integrity took longer to materialize. Initial MRI assessments of meniscal integrity were morphometric in nature characterizing meniscal volume, regional thickness, and position [64]. However, the meniscal fibrocartilage is primarily composed of type I collagen and a small percentage of proteoglycans embedded in a dense collagen matrix. These characteristics have consequently stimulated studies of meniscal compositional integrity with MR relaxation times including T2 (Fig. 19.9), T1ρ [65], and T1Gd [66].
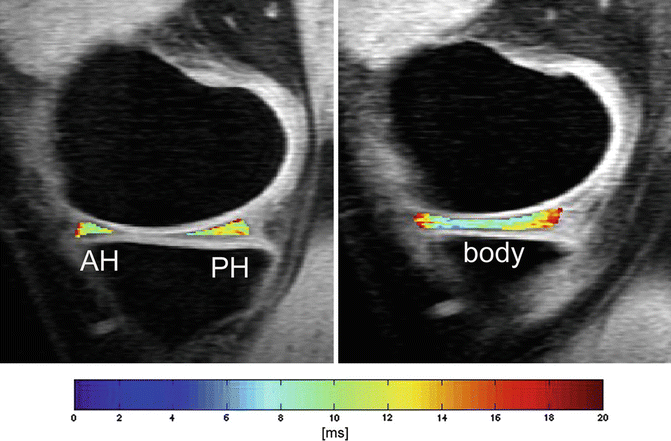
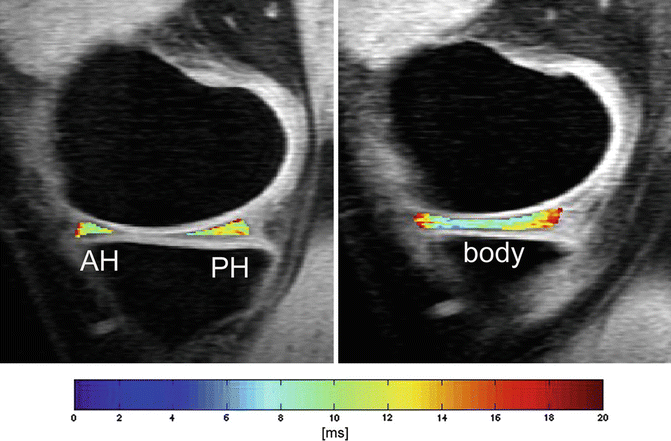
Fig. 19.9
Representative meniscal T2 relaxation time maps: (a) anterior horn (AH) and posterior horn (PH); (b) body of medial meniscus [67]
19.4.1.3 Trabecular Bone
In terms of bone quantification, MRI has been primarily used for trabecular bone assessment [68]. This is, however, a challenging task due to a trade-off between signal-to-noise ratio (SNR) and spatial resolution. The small dimensions of trabecular bone push the limits of clinical MRI scanners in order to obtain high-spatial-resolution images with high SNR at clinically feasible scan times.
Trabecular bone assessment with MRI has been primarily done in the distal radius, distal tibia, and calcaneus. The main reasons were not only due to their anatomical relevance in osteoporosis but also due to the trade-off mentioned above. These anatomical sites do not impose the same level of SNR limitations as deep body locations like the proximal femur, where radio frequency signals are attenuated by surrounding tissues such as fat and muscle. However, recent advances in MRI hardware, coil design, pulse sequences, and image analysis techniques have enabled the acquisition and analyses of high-spatial-resolution images depicting the trabecular bone microstructure of the proximal femur (Fig. 19.10) [69, 70], which is a site of utmost importance in studies of osteoporosis.
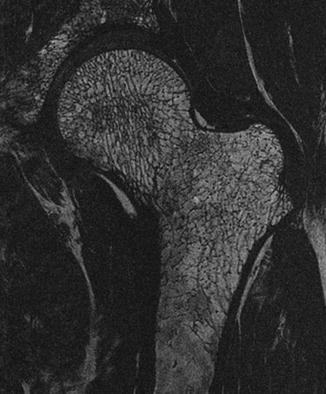
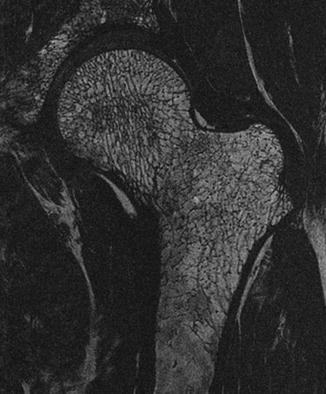
Fig. 19.10
Coronal cross section of an in vivo high-spatial-resolution MR image of the proximal femur with a spatial resolution of 234 × 234 × 500 μm3 [71]
Since clinical MRI is based on mobile protons and the bone is solid, then the bone exhibits very short T1 and T2 relaxation times thus yielding no signal. On the other hand, mobile protons in the fatty and water content of bone marrow yield a relatively strong signal. The result is therefore an “inverse” image depicting the trabecular bone micro-architecture. Several parameters have been developed with the aim of quantifying the trabecular bone micro-architecture using MRI. These parameters have been primarily divided into three classes according to the main aspect that they quantify: (1) scale, (2) topology, and (3) orientation also known as anisotropy.
19.4.1.4 Muscle
Similar to research involving menisci, muscle was largely ignored giving preference to studies of cartilage. However, it has been recently acknowledged that knee osteoarthritis is a pathology that goes beyond cartilage; it is a pathology that involves all the different tissues of the joint. For this reason, investigators have started to investigate the associations of muscle characteristics with knee osteoarthritis. In particular, investigators have looked into muscle cross-sectional area as well as into surrogate measures of fat infiltration [72], since fat infiltration has been observed in multiple chronic conditions such as diabetes and sarcopenia due to aging. For this purpose, investigators have primarily used T1-weighted images. However, T1-weighted images can only provide qualitative measures of fat content based on pure signal contrast. On the other hand, more advanced imaging techniques such as proton magnetic resonance spectroscopic imaging (1H MRSI) and chemical shift-based water-fat separation approaches can provide accurate measures of fat fraction content. 1H MRSI provides detailed spectral information at low spatial resolutions, while chemical shift-based water-fat separation approaches provide high-spatial-resolution fat fraction maps enabling voxel-wise analyses [73–75]. Figure 19.11 illustrates a representative fat infiltration muscle analysis in the thigh using chemical shift-based water-fat separation.
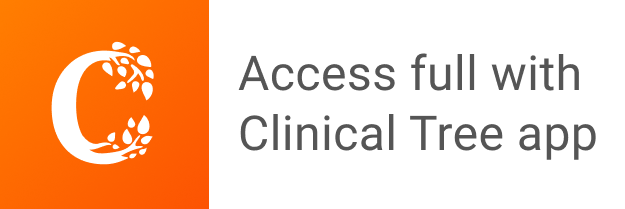