Fig. 20.1
Illustrative example of a synthetic spectrum signal showing the water (highest peak, on the left) and the multiple fat peaks. On a 3 Tesla MR, the main fat peak is located at a frequency shift of 420 Hz (1.46 ppm) relative to water peak. Fat proton density results from the sum of multiple fat peaks of the diverse chemical moieties of triglycerides. These multiple fat peaks should be included in the signal fitting algorithm

20.2.2 Chemical-Shift-Based MR Sequences
For several years, dual-echo chemical-shift (ChSh) gradient-echo (GRE) sequences have been widely used in clinical practice for the visual assessment of liver steatosis. For a given magnetic field strength, fat protons precess slower than water protons. Images are acquired with a first echo, when water and fat peaks are “out of phase” (OP), and then with a second echo, when the two peaks are “in phase” (IP). The echo time (TE) corresponding to the IP and OP images is dependent on the field strength: fat and water peaks are “in phase” every 4.6 ms (1.5 T MR) or 2.3 ms (3 T MR) and are “out of phase” at 2.3 ms (1.5 T MR) or 1.15 ms (3 T MR) and subsequent multiples [13]. On IP images, the liver signal intensity results from the water plus fat signals, while on OP images, the liver signal results from the difference between the water and fat signals. Therefore, steatosis can be recognized as a liver signal dropout on the OP image in comparison to the IP image (Fig. 20.2). Theoretically, the liver fat content could be estimated as:
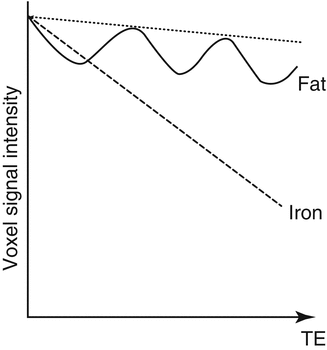
where SIP corresponds to liver/spleen signal intensity measured on the IP image and SOP represents the relative signal measured on the OP image [14]. Unfortunately, this easy approach cannot be used for precise fat quantification, because this estimated fat fraction is based on signal intensities of water and fat, which are subject to several biases, rather than on proton densities. For example, in patients with iron overload, due to the iron T2*-shortening effects, there is a decrease in liver signal intensity on second-echo IP images relative to the first-echo OP images (Fig. 20.2). Therefore, when fat and iron coexist, the decrease of liver signal in the second-echo (IP) image due to increased iron-related T2* decay results in less or no apparent liver signal change between the first- (OP) and second-echo (IP) images [15]. Consequently, liver fat content will be underestimated by the coexistence of iron, if the T2* decay is not taken into account. Since these chemical-shift sequences are acquired with at least two different echoes, some signal T2* decay occurs between different echoes, even in normal livers (Fig. 20.2).
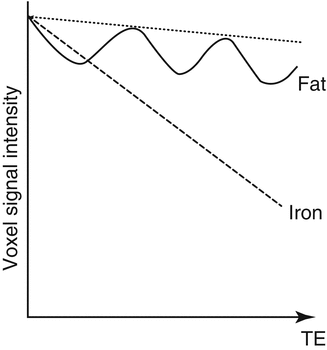
Fig. 20.2
Scheme illustrating the T2* decay in liver parenchyma without fat or iron overload (dot line), in livers with fat (solid line) and in livers with iron overload (dashed line)

Besides the T2* decay effect, the “signal” fat fraction is also influenced by other factors, such as the T1 relaxation, noise, eddy currents, and the spectral complexity of the fat spectrum [13]. Nevertheless, if all these confounding factors are corrected or minimized, the “signal” fat fraction becomes equivalent to the “proton density” fat fraction (PDFF). In the last decade, the development of multi-echo chemical-shift-encoded MR (MECSE-MR) sequences has provided an accurate tool for PDFF quantification. Nowadays, MR-estimated PDFF is being regarded as the best imaging biomarker for liver steatosis.
20.2.3 Multi-echo Chemical-Shift-Encoded MR (MECSE-MR) Sequences
20.2.3.1 Acquisition: MR Sequence, Biases of T2* effect and T1 (Flip angle)
MECSE-MR sequences are fast, spoiled gradient-recalled echo (SPGR) sequences performed with more than three echoes (usually between 6 and 12), acquiring images of the whole liver within one or few breath holds. The acquisition of multiple echoes allows to separate water and fat signals while simultaneously estimating the T2* decay.
For accurate liver fat quantification, the influence of T2* relaxation and T1 relaxation in the measurements of fat and water signals must be corrected or minimized [13]. If not taken into account, the effect of T2* decay confounds PDFF quantification, either in iron-overloaded or normal livers. Different approaches can be used to correct for the effect of T2* decay by incorporating the T2* in the post-processing fitting model, thereby correcting the T2* decay as part of the fitting, or by measuring the T2* of water and fat separately and then correcting for the effects of T2* [13]. Quite relevant, this T2* estimation also allows for the simultaneous quantification of iron deposits [14, 16–19], which is particularly important as iron overload and steatosis frequently coexist. These methods can assume a single T2* decay for water and fat, or they can perform independent T2* estimations of water and fat. Published reports are controversial about which of these strategies gives improved PDFF quantification [20, 21].
The different T1 relaxation times between water and fat (fat has a shorter T1 than water) may introduce a significant bias in fat fraction estimation. If the acquisition is T1 weighted, fat will have its signal relatively amplified as compared to water. The T1 bias can be reduced either by image acquisition with minimum T1 weighting or postacquisition processing to estimate and correct T1 effect. The T1 weighting can be minimized if a low flip angle is chosen in the gradient-echo sequence [22]. If using a 2D sequence with TR greater than 100 ms, the flip angle should be less than 10°, whereas using a 3D sequence, a flip angle of 2–5° should be preferred. The combination of low flip angle and TR must be chosen to optimize signal-to-noise ratio (close to Ernst angle) and contrast, because the signal-to-noise ratio (SNR) of an SPGR acquisition decreases rapidly at small flip angles [22]. For postacquisition correction, two or more acquisitions should be performed with different T1-weighting parameters, and the estimated T1 bias is then computationally corrected in the final PDFF quantification [23].
20.2.3.2 Imaging Processing and Analysis: Noise, Eddy Currents, and Fat Spectral Complexity
Two major approaches can be used in the imaging processing analysis, both being robust and accurate: the magnitude-based and the complex-based techniques [13]. The firsts discard phase information and only use the magnitude images. Thus, they cannot differentiate which is the dominant tissue component (fat or water) and are unable to quantify fat fraction beyond the 0–50 % spectrum. The complex-based methods use both magnitude and phase information from the different TEs and, in contrast to magnitude-based methods, allow for fat quantification between the range of 0 and 100 %.
Noise is particularly relevant for magnitude-based post-processing methods: after performing the magnitude operation, when the phase information is discarded, the signal intensities of areas with lower fat content will be increased by noise, introducing a bias in PDFF calculation. This effect is more significant at low fat fractions, and it can be avoided using phase constrained or magnitude discrimination methods [22].
Eddy currents may result into phase errors on images acquired at different echo times, such as complex images acquired with these MECSE-MR sequences, leading to bias in PDFF quantification. Eddy currents affect post-processing methods that use phase information (complex methods), whereas magnitude-based methods (which discard phase information) are relatively insensitive to its effects. Hybrid and mixed magnitude/complex fitting methods have been proposed to correct for eddy currents, particularly at 1.5 T acquisitions [22, 24, 25].
Whether magnitude or complex data are used, the acquired liver MR signal is modeled for fat-water separation. The fat signal has at least six distinct frequency components, with different amplitudes (Fig. 20.1). In contrast to dual-echo chemical-shift GRE sequences, which only take into account the frequency of the main fat spectral peak, multipeak reconstruction models consider the complexity of fat spectrum by modeling the fat signal as a weighted sum of all fat frequency components. Multipeak methods provide higher accuracy and should be performed for precise measuring of liver fat [18, 26–29]. Because it is impractical to determine every fat peak for each individual liver, pre-calibrated multipeak fat spectral methods have been proposed, in which the resonance frequencies and relative amplitudes of each fat peak are known a priori and based on MR spectroscopy-derived measurements [30–32]. Although no specific multipeak spectral model was shown to be superior to the rest [32], further research is needed to define the influence of the number, location, and fitting of the fat peaks in PDFF quantification with different multipeak reconstruction models.
Parametric maps can be obtained if the post-processing models are applied in a voxel-wise approach, demonstrating the quantity and distribution of PDFF data throughout the liver parenchyma. These parametric maps are particularly useful because of the nonhomogeneous distribution of fat deposits throughout the liver parenchyma (Fig. 20.3, Fig. 20.4). There are some commercial products available to measure PDFF with T1-independent and T2*-corrected MECSE-MR, like IDEAL IQ (General Electric®) and mDIXON QUANT (Philips®).
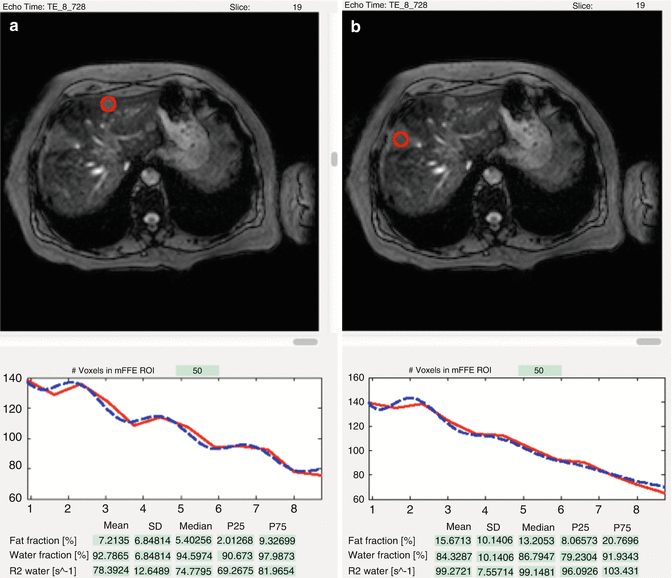
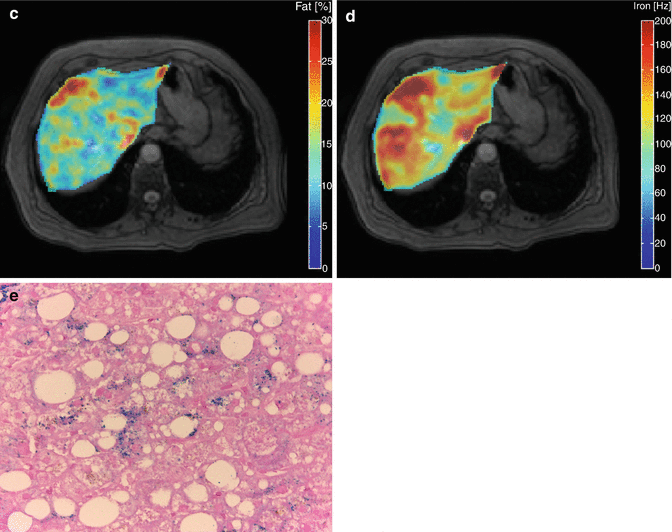
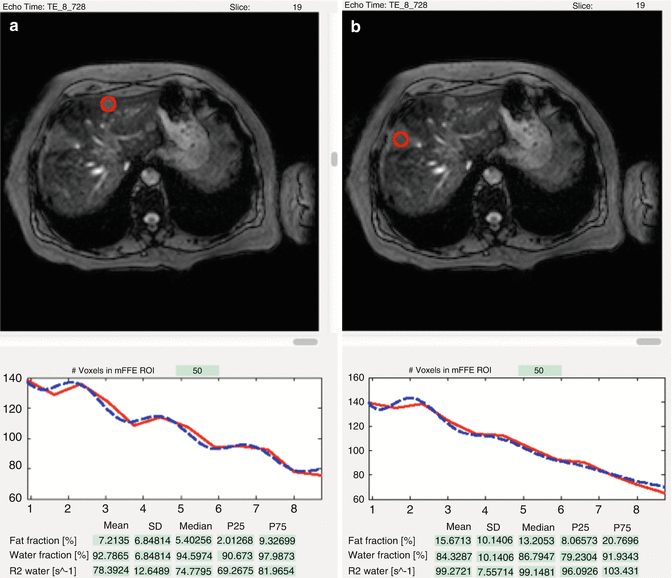
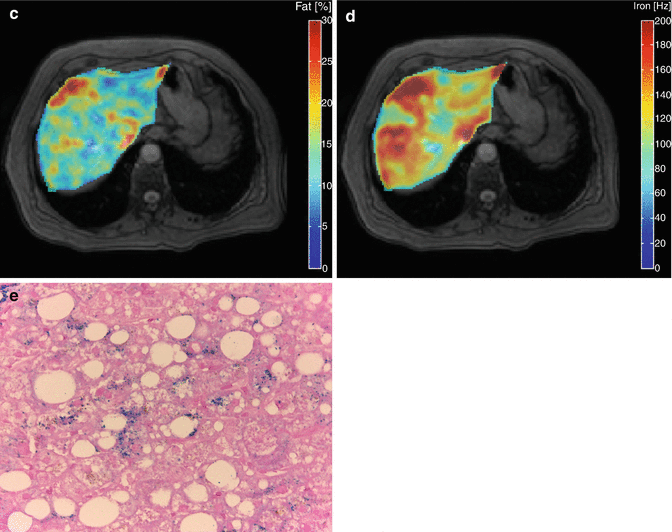
Fig. 20.3
PDFF and R2* quantification with a MECSE-MR sequence. (a) and (b) represent magnitude images used for fat and iron quantification, and the respective signal decay curve calculated with QLiver® software. Images show a heterogeneous and patchy distribution of fat and iron deposits throughout the liver parenchyma. (a) A ROI area in IVa liver segment determined a PDFF=5.4% and R2*=74 s-1. (b) Another ROI placed over the VIII liver segment measured a PDFF=13.2% and R2*=99 s-1. Parametric maps of PDFF (c) and iron related-R2* (d) measurements are particularly useful to demonstrate the heterogeneous distribution of fat and iron deposits throughout the liver parenchyma. (e) Liver biopsy (after hematoxylin-eosin and Perls’ Prussian staining, 400x magnification) demonstrating coexistence of steatosis and iron deposits in liver parenchyma
At their own institutions, the authors are using a 2D MECSE-MR sequence in a 3-T MR scanner, with 12 echoes (TEs = 0.99, 1.69, 2.39, 3.09, 3.79, 4.49, 5.19, 5.89, 6.59, 7.29, 7.99, 8.69; TR = 10 ms, echo spacing = 0.7 ms) and a flip angle of 10° [14]. The whole liver is covered under end-expiratory phase single breath-hold acquisition with 34 axial slices (voxel dimensions, 3 × 3 mm; slice thickness, 7 mm; 0.3 mm gap; reconstruction voxel size, 2 × 2 mm; field of view, 375 × 302 mm; parallel imaging effective acceleration factor, 1.8; bandwidth, 2433 Hz per pixel). Acquired images are exported as raw data to PDFF and R2*(=1/T2*) quantification, using QLiver software (QUIBIM®, Valencia, Spain) (Fig. 20.3, Fig. 20.4).
The complex phase information estimates the resonance peak of the larger component (water or fat), to generate frequency distribution maps. Then, a joint fit between water signal, fat signal, R2* of water, and R2* of fat is performed. A multipeak reconstruction model for fat quantification is used as proposed by Yu et al. (75 %, 420 Hz; 17 %, 318 Hz; 8 %, −94 Hz) [30]. Finally, the pixel PDFF is calculated as the ratio between the normalized fat proton density and the total (fat and water) proton density (PDFF = PDfat/[PDfat + PDwater]). In addition to T2* correction, the R2* (=1/T2*) of water is used to estimate the iron content.
20.2.4 Proof of Principle
These MECSE-MR imaging sequences were shown to be robust and accurate for PDFF quantification in several studies, either using MRS measurements [17, 29, 33, 34] or liver biopsy [18, 19, 26, 27, 35, 36] as reference standards. Also, PDFF measurements were shown to be repeatable [37–39, 41] and reproducible across different MR scanner platforms at 1.5- and 3-T scanners [42]. Longitudinal hepatic PDFF changes greater than 1.6–1.8 % are likely to represent real changes rather than noise or measurement imprecision [39, 40].
When interpreting PDFF measurements as a biomarker of steatosis, it should be remembered that although PDFF and histological estimated steatosis percentages are highly correlated, they are not equivalent: histologic evaluation measures the percentage of hepatocytes with macrovesicules of fat, whereas PDFF measures the amount of fat protons within the hepatocytes. Recently, PDFF threshold values have been proposed either to diagnose liver steatosis or to distinguish between different histologic grades. PDFF threshold values to diagnose hepatic steatosis range from 2.9 to 7.53 % [18, 26–28, 43, 44].
20.2.5 Proof of Efficacy and Effectiveness
PDFF quantification corrected for the main confounding factors (T1 bias, effect of T2* relaxation, fat spectral complexity, noise bias, and eddy currents) is currently being accepted as the best available imaging biomarker of liver steatosis [45]. MECSE-MR sequences were considered to be more precise than liver biopsy for therapy monitoring in patients with NASH [13, 46]. Other potential applications for these sequences are the living liver donors’ evaluation and the pre- and postoperative assessment of patients undergoing bariatric surgery.
Nevertheless, most of the validation studies were conducted in patients with NAFLD and, consequently, could be biased to higher liver fat content. Distinct PDFF thresholds to diagnose hepatic steatosis and to discriminate different histologic grades have been reported in different studies. Therefore, proposed PDFF thresholds still require validation in large cohorts of patients with different clinical scenarios of diffuse liver diseases, before they become standardized and widely accepted. Moreover, the best strategy regarding the number of echoes, the curve fitting, or the multipeak fat modeling has yet to be defined.
20.3 Imaging Biomarkers of Liver Iron: MR Sequences, Measurements, and Biases
In contrast with fat quantification, MR imaging does not quantify iron directly but, instead, depicts the paramagnetic effect of iron on the neighborhood protons. Iron accelerates the T2 relaxation and mainly the T2* signal decay, resulting in a recognizable signal loss on T2- and T2*-weighted images, which is proportional to the iron content.
The MR imaging methods developed for liver iron quantification can be divided into signal intensity ratio (SIR) methods and relaxometry methods. All of these methods need calibration as they perform measurements (signal intensity ratios or relaxation times or rates) from MR images, which are compared to chemically determined LIC values from liver biopsies (the gold standard), to generate empirical calibration curves [8].
20.3.1 Signal Intensity Ratio (SIR) Methods
In SIR methods, the signal intensity (SI) of the liver is compared to the SI of a reference tissue that does not accumulate iron, usually the skeletal muscle. The most widely used SIR method [47] is performed with a body coil, acquiring five IP GRE sequences, with constant TR but with different flip angles and increasing TE. On each sequence, three ROIs are placed within the right hepatic lobe and two ROIs within the paraspinal muscles to quantify liver and muscle SI. In a free online worksheet provided by the University of Rennes (http://www.radio.univ-rennes1.fr/Sources/FR/HemoCalc15.html), the LIC (μmol Fe/g) is estimated from the mean SI of each ROI. This computer-based algorithm was validated in 149 patients and provides accurate LIC measurements, over a range from 3 to 375 μmol Fe/g dry weight. However, in a subsequent multicenter study with 171 patients, which has compared LIC estimated by MR and LIC chemically quantified, the diagnostic accuracy was 61.4 %, with a tendency to overestimate overload [48]. The differences between the MR-estimated LIC and the biopsy LIC may be important in clinical practice. Nevertheless, this model is very useful to rule out disease, due to its low tendency to underestimate LIC: hemochromatosis is excluded at a cutoff point of less than 60 μmol Fe/g, with a negative predictive value of 100 % [48].
Alústiza et al. [49] proposed a mathematic model for estimating LIC using only two sequences (T2-* and PD-weighted sequences) and, therefore, reducing the acquisition time required for liver iron MR quantification as compared to the original Gandon’s method. A free online worksheet for LIC quantification has been provided by the Sociedad Española de Diagnóstico por Imagen del Abdómen (SEDIA) (http://www.sedia.es/sedia_investiga/proyectos2007/calculo_hierro/calculoFE.php). In patients suspected of having hemochromatosis, an MR-estimated LIC >79 μmol/g has a positive predictive value of 100 %, whereas an estimated LIC <20 μmol/g has a negative predicted value of 100 %.
In spite of being widely available, these SIR methods have some important limitations. Firstly, they saturate with very high iron overload, with an upper limit value of LIC in the range of 350 μmol Fe/g. Many patients with transfusional hemochromatosis have LIC values higher than this range. Secondly, SI ratios may be confounded by coexisting hepatic steatosis and/or muscle fatty infiltration. Also, these protocols are not compatible with phased-array coils, and, quite relevant, they are not calibrated for 3-T machines. Finally, SIR methods are influenced by the sequence TR, TE, flip angle, and body habitus [8].
20.3.2 Relaxometry Methods
Relaxometry techniques measure relaxation time constants after acquiring series of images with increasing TEs, usually more than six echoes, and can be performed with surface coils. The liver SI is modeled as a function of TE, and signal decay constants are then calculated [8]. The T2 or T2* values (measured in ms) are calculated, depending on whether a spin-echo-based or a gradient-echo-based sequence is performed, respectively. The rates of signal decay R2 (=1/T2) or R2*(=1/T2*) may be used instead and are usually presented in s−1. The underlying proof of concept is that liver T2 and T2* are related to liver iron concentrations: the greater the liver iron concentration, the higher the relaxation rates (R2 or R2*) and the lower the relaxation times (T2 or T2*) [50].
20.3.2.1 R2 Relaxometry
The most known R2 relaxometry method [51] was validated in over 100 patients, with LIC values ranging from 0.3 to 42.7 mg Fe/g dry weight (5–747 μmol Fe/g). Liver R2 had a curvilinear relationship with LIC, with a correlation coefficient of 0.98. This technique is currently available as a commercial service (“FerriScan®”), which has been approved by the Food and Drug Administration (FDA) in the USA. After calibration, MR images are acquired with five T2-weighted sequences, during free breathing, and are then forwarded for centralized image data analysis and R2 measurements. This method has shown a low inter-exam variability and good inter-machine reproducibility [52, 53]. Wood et al. [54] have reproduced the same calibration curve obtained by St. Pierre et al., using different imaging parameters, and a different fitting model (a monoexponential decay model rather than a biexponential decay model). In a recent multicenter validation study, the calibration curve appeared independent of the patient age, stage of liver fibrosis, grade of necroinflammation, and use of chelation therapy. However, the limits of agreement between R2 LIC and biopsy LIC were very broad (between 74 and −71 %) [53].
Using R2 relaxometry, new MR methods have been proposed for separately quantifying the two principal forms of tissue storage iron [55–57]: the dispersed, soluble ferritin iron, which is rapidly mobilized, and the aggregated, insoluble hemosiderin iron, which acts as a long-term reserve. If the MR signal is obtained with varying echo spacing, the signal analysis can be decomposed into the two different forms of iron storage because ferritin iron has a monoexponential decay and the hemosiderin iron has a non-monoexponential. This method could improve the monitoring of iron-reducing therapies, since ferritin is in equilibrium with the cytosolic iron pool that changes rapidly with iron chelation.
20.3.2.2 R2* Relaxometry
R2* relaxometry methods usually are performed with SPGR multi-echo sequences, with increasing TEs. The liver R2* is calculated from the fitting of the rate of exponential signal decay, on either a voxel-by-voxel basis or averaging the measured signal within a ROI [58]. Ideally, the first echo should be as short as possible (1 ms or less), and the echo spacing should be short enough (approximately 1 ms or less), to guarantee that the signal decay is captured. This is particularly relevant in severe iron-overloaded livers: if the first TE is too long, most of the MR signal will have irreversibly disappeared by the first image acquisition [52]. A high number of echoes may result in improved R2* estimation (reduced standard deviation) in cases of low R2* values (low iron content), but not for severe iron-overloaded livers (R2* higher than 1000 s−1), where most of the signal has fully decayed by a TE of 3–4 ms [59]. Finally, the last echo time of 10–15 ms is usually sufficient [60].
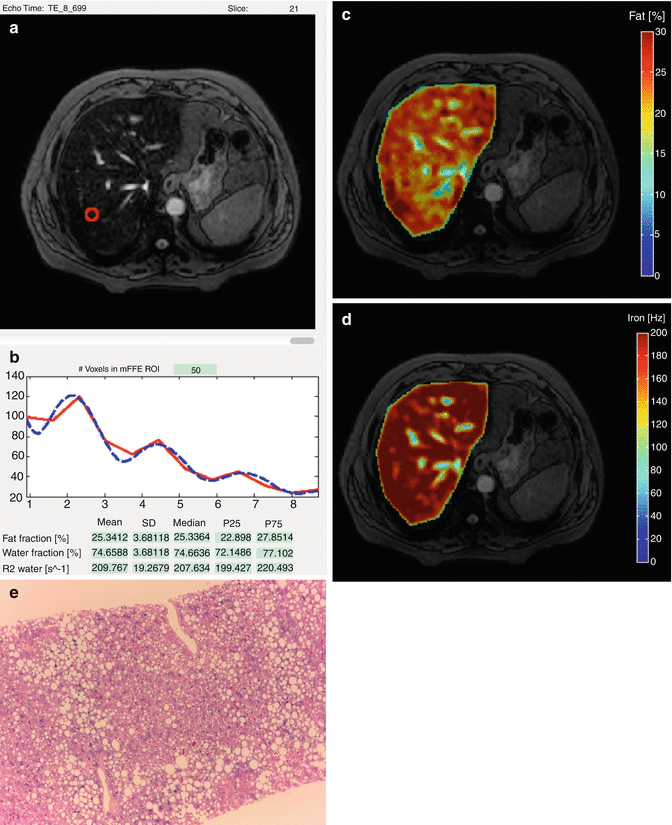
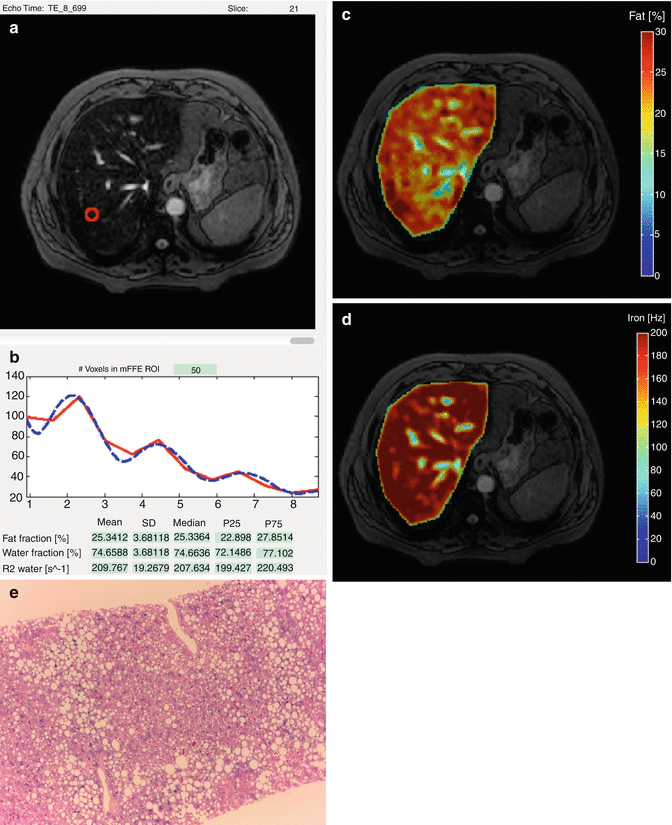
Fig. 20.4
PDFF and R2* quantification with a MECSE-MR sequence. (a) magnitude images used for fat and iron quantification, and the respective signal decay curve calculated with QLiver® software. A ROI area in the VII liver segment determined a PDFF=25% and R2*=208 s-1. (b) Parametric maps of PDFF (c) and iron related-R2* (d) measurements are particularly useful to demonstrate the distribution of fat and iron deposits throughout the liver parenchyma. (e) Liver biopsy (after Perls’ Prussian staining, 100x magnification) demonstrating severe steatosis (grade 3/3) and iron overload (grade 3/4). The MECSE-MR measurements were well related with liver biopsy
The most used R2* relaxometry method [54] was calibrated in 21 patients (23 liver biopsies), in a 1.5-T equipment, using a single breath-hold multi-echo GRE sequence with 17 TE, stepped at 0.25-ms intervals from 0.8 to 4.8 ms. Data were fitted to a monoexponential decay curve with constant offset on a pixel-by-pixel basis, obtaining liver R2* parametric maps. A linear relationship between R2-* and biopsy-based LIC (mg Fe/g) was derived, with a correlation coefficient of 0.97. Other authors have derived different calibration curves to transform R2* values into LIC values (mg Fe/g or μmol Fe/g), most probably due to differences in methodologies (Fig. 20.5) [61, 62]. R2* relaxometry methods have shown a good reproducibility across different scanners and centers, as also a good interobserver reproducibility [58, 63]. For image analysis, a CE-marked and FDA-approved software is commercially available (Thalassaemia Tools – https://www.cmrtools.com).
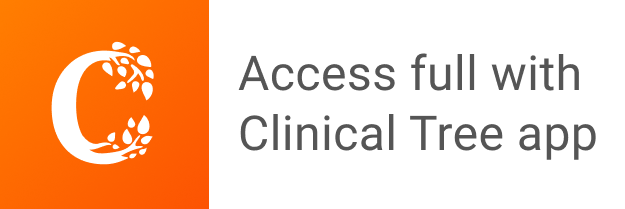