Fig. 4.1
Single-trial coupling of power amplitudes of the early auditory evoked EEG gamma-band response (GBR) and the corresponding BOLD activation (random effects analysis, uncorrected for multiple testing). GBR-specific BOLD activations can be seen in the left auditory cortex (gyrus temporalis superior, Brodmann area 41/22), the thalamus and the anterior cingulate cortex (Brodmann area 24). In the lower right corner, a glass brain view is provided demonstrating a 3D view of the three abovementioned clusters. Upper row: Talairach coordinates – x(left) = 0, x(middle) = −15, x(right) = −48. Lower row: y(left) = 32, z(middle) = 19. SAG sagittal, COR coronary, TRA transversal, A anterior, P posterior, R right, L left (Reprinted from Mulert et al. 2010. Copyright (2010), with permission from Elsevier)
Using EEG-fMRI, several studies also successfully tried to identify brain regions associated with changes in ongoing brain rhythms (Hanslmayr et al. 2011; Michels et al. 2012; Sadaghiani et al. 2010; Scheeringa et al. 2011). Therefore, in general, the spectral power in one or more specific frequency bands has been quantified for each of several short epochs of EEG. After convolving this power time series with a HRF, it is entered as a regressor in the fMRI GLM analysis.
The so-called joint ICA has been proposed as an alternative approach to the analysis techniques outlined above. This multivariate approach for the integration of EEG and fMRI measures tries to identify meaningful patterns in an EEG-fMRI joint data space considering EEG and fMRI signals together (Eichele et al. 2008; Kraut et al. 2003; Lei et al. 2010; Mangalathu-Arumana et al. 2012; Moosmann et al. 2008; Ostwald et al. 2012). EEG source localisation prior to the fusion of EEG and fMRI information may be another way of enriching the interpretation of EEG-fMRI patterns by separating the activity from different sources and additionally providing spatial information on the process of interest (Brookes et al. 2008). For example, Esposito et al. used the local source power trial-by-trial variation derived from EEG source localisation of the ERP of interest as an input for single-trial EEG-fMRI coupling (Esposito et al. 2009).
4.5.3 EEG-Informed fMRI and Functional Connectivity of Resting-State Networks
The functional connectivity between brain regions of intrinsic brain networks such as the default-mode network (DMN) is known to be increased. Patients with several neuropsychiatric diseases show alterations in resting-state networks, e.g. in Alzheimer’s disease (Franciotti et al. 2013). Studies using simultaneous EEG-fMRI measurements investigated resting-state activity with respect to BOLD correlates of EEG oscillations of specific frequencies such as alpha-oscillations (Goldman et al. 2002; Laufs et al. 2003; Moosmann et al. 2003). Beyond that, the EEG signal has also been related to the functional connectivity within and between networks in order to characterise network dynamics. For example, delta and beta oscillations explain 70 % of the DMN variance of functional connectivity (Hlinka et al. 2010). An increase of alpha power leads to a decrease of fMRI connectivity between the primary visual cortex and occipital regions as well as a decrease of the negative coupling between visual areas and regions of the DMN (Scheeringa et al. 2012). The strength of connectivity between DMN and the dorsal attention network (DAN) has been found to be inversely correlated with alpha power, whereas alpha power correlated with the spatial extent of anticorrelation between DMN and DAN (Chang et al. 2013).
FMRI patterns have also been related with complex patterns of EEG organisation such as so-called microstates. These are quasi-stable and unique topographic representations of the EEG reflecting the summation of concurrent neuronal activity across brain regions rather than being specific for a certain frequency band (Lehmann et al. 1987). Recently, the relationship between EEG microstates and BOLD resting-state networks has been investigated using simultaneous EEG-fMRI (Britz et al. 2010; Huang et al. 1996; Musso et al. 2010). Microstates are known to be altered in different psychiatric disorders such as schizophrenia (Lehmann et al. 2005).
The relationship between EEG coherence and fMRI connectivity patterns has been investigated by Wang et al. using the combination of fMRI and invasive electrophysiological recordings. This study revealed that the coherence in low frequencies (<20 Hz) predominantly contributes to fMRI connectivity patterns in the resting state in monkeys (Wang et al. 2012). However, especially high-frequency oscillations (30–100 Hz) seem to be tightly linked to the BOLD signal (Mukamel et al. 2005; Niessing et al. 2005). In order to integrate findings from EEG coherence and fMRI connectivity in humans in the future, the combination of simultaneous EEG-fMRI and EEG source analysis represents a promising approach.
4.5.4 EEG-fMRI and High-Frequency Oscillations
Scalp EEG activity includes oscillations of a variety of distinct frequency ranges each of which is supposed to represent the synchronisation of varying neuronal networks. The rhythmic activities in the resting or “spontaneous” EEG are usually divided into several frequency bands (delta, <4 Hz; theta, 4–8 Hz; alpha, 8–12 Hz; beta, 12–30 Hz; and gamma, >30 Hz) which are associated with different behavioural states, ranging from sleep to relaxation, heightened alertness and mental concentration (Lindsley 1952; Niedermeyer and Lopes Da Silva 2004; Nunez 1995). A comprehensive analysis of the different types of brain rhythms can be found in Buzsáki’s monography Rhythms of the Brain (Buzsaki 2006).
Several studies investigating the relationship between fMRI and special oscillatory EEG components reported especially high correlations between high- in comparison with low-frequency electric activity and BOLD signal. Recordings in cats revealed that correlations between LFPs and BOLD signal are especially high in the gamma-band frequency range (Niessing et al. 2005). Similar results were found in studies combining fMRI and intracranial EEG recordings in human epilepsy patients (Mukamel et al. 2005) showing also a predictive value of fMRI about the anatomical location of cross-condition gamma-band modulations (Brovelli et al. 2005; Lachaux et al. 2007). Foucher et al. reported a differential reactivity of event-related potentials (ERPs) and BOLD signal in response to the presentation of two kinds of rare events (i.e. target and novel stimulus in an oddball task) using combined measurement of EEG and fMRI. However, in accordance with fMRI results, target-related gamma oscillations were more intense than their novel-related counterparts (Foucher et al. 2003). The authors of this study propounded a physiological hypothesis underlying these findings: as opposed to ERPs reflecting synchronous activity of synapses within milliseconds (Baillet et al. 2001; Speckmann and Elger 1999) and similar to the BOLD signal, event-related gamma oscillations are less dependent on the small jitter of the neuronal response relative to a stimulus because they do not require to be time-locked to the stimulus that precisely (Tallon-Baudry and Bertrand 1999). Furthermore, ERPs reflect the synaptic input function of pyramidal cells only (Baillet et al. 2001; Speckmann and Elger 1999), whereas fMRI reflects the synaptic activity of all neural cells including inhibitory interneurons (Logothetis et al. 2001; Mathiesen et al. 2000; Matsuura and Kanno 2001) which are involved in the synchronisation of gamma oscillations (Traub et al. 1996), too. A third possible reason could be related to the assumption that ERPs might correspond to the simple phase resetting of ongoing cerebral activity (Makeig et al. 2002) which should not consume much energy (Foucher et al. 2003).
4.6 EEG-fMRI in Psychiatry
Using the EEG-informed fMRI approach, Mobascher et al. investigated the influence of nicotine on the EEG P300 component of the visual evoked potential elicited by an oddball-type task in patients with schizophrenia. The authors report a nicotine-associated increase in P300-informed fMRI activation in schizophrenia patients and healthy controls, mainly in the anterior cingulate cortex (ACC) and adjacent medial frontal cortex. The results of separate analyses of EEG and fMRI measures were reported to be largely unaffected by nicotine (see Fig. 4.2) (Mobascher et al. 2012). Musso et al. conducted an EEG-fMRI study on the human ketamine model of schizophrenia and report diminished P300 amplitudes (visual oddball task) and corresponding BOLD responses in the ketamine condition in cortical regions being involved in sensory processing and selective attention (Musso et al. 2011). The EEG-informed fMRI approach has recently also been used to describe the brain network involved in reward processing (Hauser et al. 2013; Plichta et al. 2013).
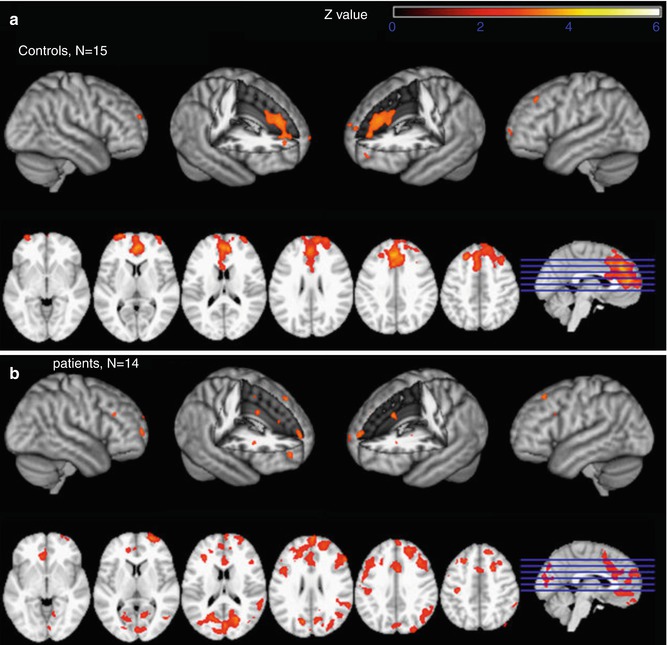
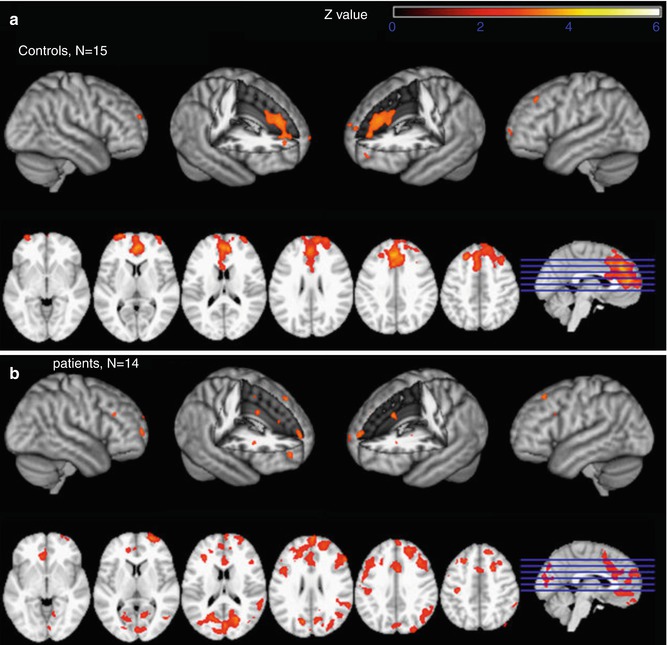
Fig. 4.2
Nicotine effects on EEG-informed fMRI BOLD activation specific for the EEG P300 component of the visual evoked potential elicited by a visual oddball task in habitual schizophrenia smokers (b) and healthy smoking controls (a). Nicotine (compared to placebo) induced an increase in P300-informed fMRI activation, mainly in the anterior cingulate cortex and adjacent medial frontal cortex (general linear model whole-brain analysis, cluster-corrected threshold Z = 2.3, p = 0.05). The results of separate analyses of EEG and fMRI measures were reported to be largely unaffected by nicotine (Reprinted from Mobascher et al. 2012. Copyright (2012), with permission from Elsevier)
With respect to ongoing brain rhythms in the resting state, Balsters et al. identified neuroanatomical origins of EEG measures with high sensitivity to the age-related cognitive decline and their manipulation by the acetylcholinesterase inhibitor donepezil. Investigating oscillatory patterns with an EEG-informed fMRI approach, the authors found drug-induced changes of delta, alpha and beta band power associated with activity differences within the hippocampus (delta, see Fig. 4.3), frontal-parietal network (alpha) and default-mode network (beta) (Balsters et al. 2011). In a study investigating resting-state networks with simultaneously recorded EEG-fMRI patients with a schizophrenia spectrum disorder showed lower EEG frequencies to be related to the default-mode and left-working-memory network compared to healthy controls (Razavi et al. 2013).
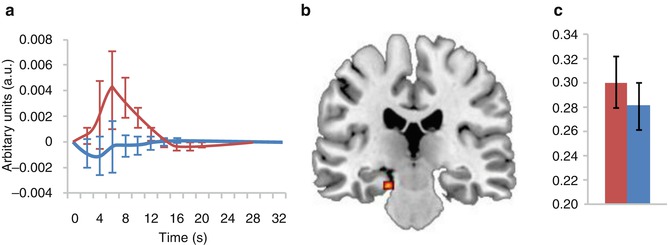
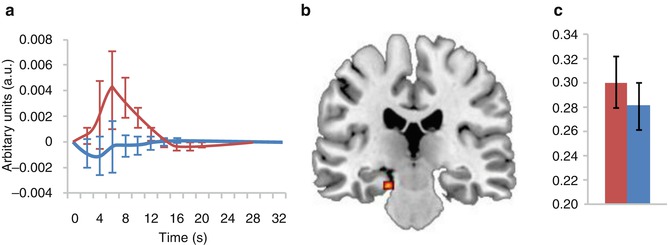
Fig. 4.3
Effects of acetylcholinesterase inhibitor donepezil on resting-state hippocampal delta (1.5–3 Hz) of healthy older participants assessed by means of EEG-informed fMRI: single-dose treatment with donepezil revealed an increase in EEG delta power and delta-specific activation of the hippocampus accompanied by a significantly worse performance on a working memory task. (a) Delta response function taken from the left hippocampus. (b) Hippocampal activation driven by differences in delta EEG power. (c) Relative delta band EEG power differences. In all graphs, red corresponds to donepezil and blue to placebo (Reprinted from Balsters et al. 2011)
Based on recent advances in EEG-fMRI, a first successful approach of real-time integration of simultaneous fMRI and EEG has been made. Thus, it was possible to perform a simultaneous multimodal real-time fMRI and EEG neurofeedback study: participants were able to simultaneously regulate their BOLD fMRI activation in the left amygdale and frontal EEG power asymmetry in the high beta band. These results indicate possible applications of the EEG-fMRI approach in enhanced cognitive therapeutic strategies for major neuropsychiatric disorders (Zotev et al. 2014).
4.7 Conclusion
Multimodal brain imaging using simultaneous measurement of EEG and fMRI is a powerful technique combining the information from EEG on neurophysiological coupling mechanisms in high temporal resolution with the superior spatial information on the involved network provided by fMRI. Therefore, EEG-fMRI can strongly contribute to the investigation of disturbances of connectivity in neuropsychiatric disorders. Both safety and signal quality issues can be addressed sufficiently today.
References
Allen PJ (2010) EEG instrumentation and safety. In: Mulert C, Lemieux L (eds) EEG-fMRI – physiological basis, technique, and applications. Springer, Berlin, pp 115–133
Allen PJ, Polizzi G, Krakow K, Fish DR, Lemieux L (1998) Identification of EEG events in the MR scanner: the problem of pulse artifact and a method for its subtraction. Neuroimage 8:229–239PubMed
Allen PJ, Josephs O, Turner R (2000) A method for removing imaging artifact from continuous EEG recorded during functional MRI. Neuroimage 12:230–239PubMed
Anami K, Mori T, Tanaka F, Kawagoe Y, Okamoto J, Yarita M, Ohnishi T, Yumoto M, Matsuda H, Saitoh O (2003) Stepping stone sampling for retrieving artifact-free electroencephalogram during functional magnetic resonance imaging. Neuroimage 19:281–295PubMed
Babiloni F, Babiloni C, Carducci F, Romani GL, Rossini PM, Angelone LM, Cincotti F (2003) Multimodal integration of high-resolution EEG and functional magnetic resonance imaging data: a simulation study. Neuroimage 19:1–15PubMed
Baillet S, Mosher JC, Leahy RM (2001) Electromagnetic brain mapping. IEEE Signal Proc Mag 18:14–30
Balsters JH, O’Connell RG, Martin MP, Galli A, Cassidy SM, Kilcullen SM, Delmonte S, Brennan S, Meaney JF, Fagan AJ, Bokde AL, Upton N, Lai R, Laruelle M, Lawlor B, Robertson IH (2011) Donepezil impairs memory in healthy older subjects: behavioural, EEG and simultaneous EEG/fMRI biomarkers. PLoS One 6:e24126PubMedCentralPubMed
Baumann SB, Noll DC (1999) A modified electrode cap for EEG recordings in MRI scanners. Clin Neurophysiol 110:2189–2193PubMed
Benar C, Aghakhani Y, Wang Y, Izenberg A, Al-Asmi A, Dubeau F, Gotman J (2003) Quality of EEG in simultaneous EEG-fMRI for epilepsy. Clin Neurophysiol 114:569–580PubMed
Benar CG, Schon D, Grimault S, Nazarian B, Burle B, Roth M, Badier JM, Marquis P, Liegeois-Chauvel C, Anton JL (2007) Single-trial analysis of oddball event-related potentials in simultaneous EEG-fMRI. Hum Brain Mapp 28:602–613PubMed
Bledowski C, Prvulovic D, Hoechstetter K, Scherg M, Wibral M, Goebel R, Linden DE (2004) Localizing P300 generators in visual target and distractor processing: a combined event-related potential and functional magnetic resonance imaging study. J Neurosci 24:9353–9360PubMed
Boynton GM, Engel SA, Glover GH, Heeger DJ (1996) Linear systems analysis of functional magnetic resonance imaging in human V1. J Neurosci 16:4207–4221PubMed
Britz J, Van De Ville D, Michel CM (2010) BOLD correlates of EEG topography reveal rapid resting-state network dynamics. Neuroimage 52:1162–1170PubMed
Brookes MJ, Mullinger KJ, Stevenson CM, Morris PG, Bowtell R (2008) Simultaneous EEG source localisation and artifact rejection during concurrent fMRI by means of spatial filtering. Neuroimage 40:1090–1104PubMed
Brovelli A, Lachaux JP, Kahane P, Boussaoud D (2005) High gamma frequency oscillatory activity dissociates attention from intention in the human premotor cortex. Neuroimage 28:154–164PubMed
Buzsaki G (2006) Rhythms of the brain. Oxford University Press, New York
Buzsaki G, Watson BO (2012) Brain rhythms and neural syntax: implications for efficient coding of cognitive content and neuropsychiatric disease. Dialogues Clin Neurosci 14:345–367PubMedCentralPubMed
Carlen M, Meletis K, Siegle JH, Cardin JA, Futai K, Vierling-Claassen D, Ruhlmann C, Jones SR, Deisseroth K, Sheng M, Moore CI, Tsai LH (2012) A critical role for NMDA receptors in parvalbumin interneurons for gamma rhythm induction and behavior. Mol Psychiatry 17:537–548PubMedCentralPubMed
Carmichael D (2010) Image quality issues. In: Mulert C, Lemieux L (eds) EEG-fMRI – physiological basis, technique, and applications. Springer, Berlin, pp 173–199
Chang C, Liu Z, Chen MC, Liu X, Duyn JH (2013) EEG correlates of time-varying BOLD functional connectivity. Neuroimage 72:227–236PubMedCentralPubMed
Colgin LL (2011) Oscillations and hippocampal-prefrontal synchrony. Curr Opin Neurobiol 21:467–474PubMedCentralPubMed
Davidson RJ, Jackson DC, Larson CL (2000) Human electroencephalography. In: Cacioppo JT, Tassinary LG, Bernston GG (eds) Handbook of psychophysiology. Cambridge University Press, Cambridge, UK, pp 27–56
de Munck JC, van Houdt PJ, Goncalves SI, van Wegen E, Ossenblok PP (2013) Novel artefact removal algorithms for co-registered EEG/fMRI based on selective averaging and subtraction. Neuroimage 64:407–415PubMed
Debener S, Ullsperger M, Siegel M, Fiehler K, von Cramon DY, Engel AK (2005) Trial-by-trial coupling of concurrent electroencephalogram and functional magnetic resonance imaging identifies the dynamics of performance monitoring. J Neurosci 25:11730–11737PubMed
Debener S, Strobel A, Sorger B, Peters J, Kranczioch C, Engel AK, Goebel R (2007) Improved quality of auditory event-related potentials recorded simultaneously with 3-T fMRI: removal of the ballistocardiogram artefact. Neuroimage 34:587–597PubMed
Debener S, Mullinger KJ, Niazy RK, Bowtell RW (2008) Properties of the ballistocardiogram artefact as revealed by EEG recordings at 1.5, 3 and 7T static magnetic field strength. Int J Psychophysiol 67:189–199PubMed
Debener S, Kranczioch C, Gutberlet I (2010) EEG Quality: origin and reduction of the EEG cardiac-related artefact. In: Mulert C, Lemieux L (eds) EEG-fMRI – physiological basis, technique, and applications. Springer, Berlin, pp 135–151
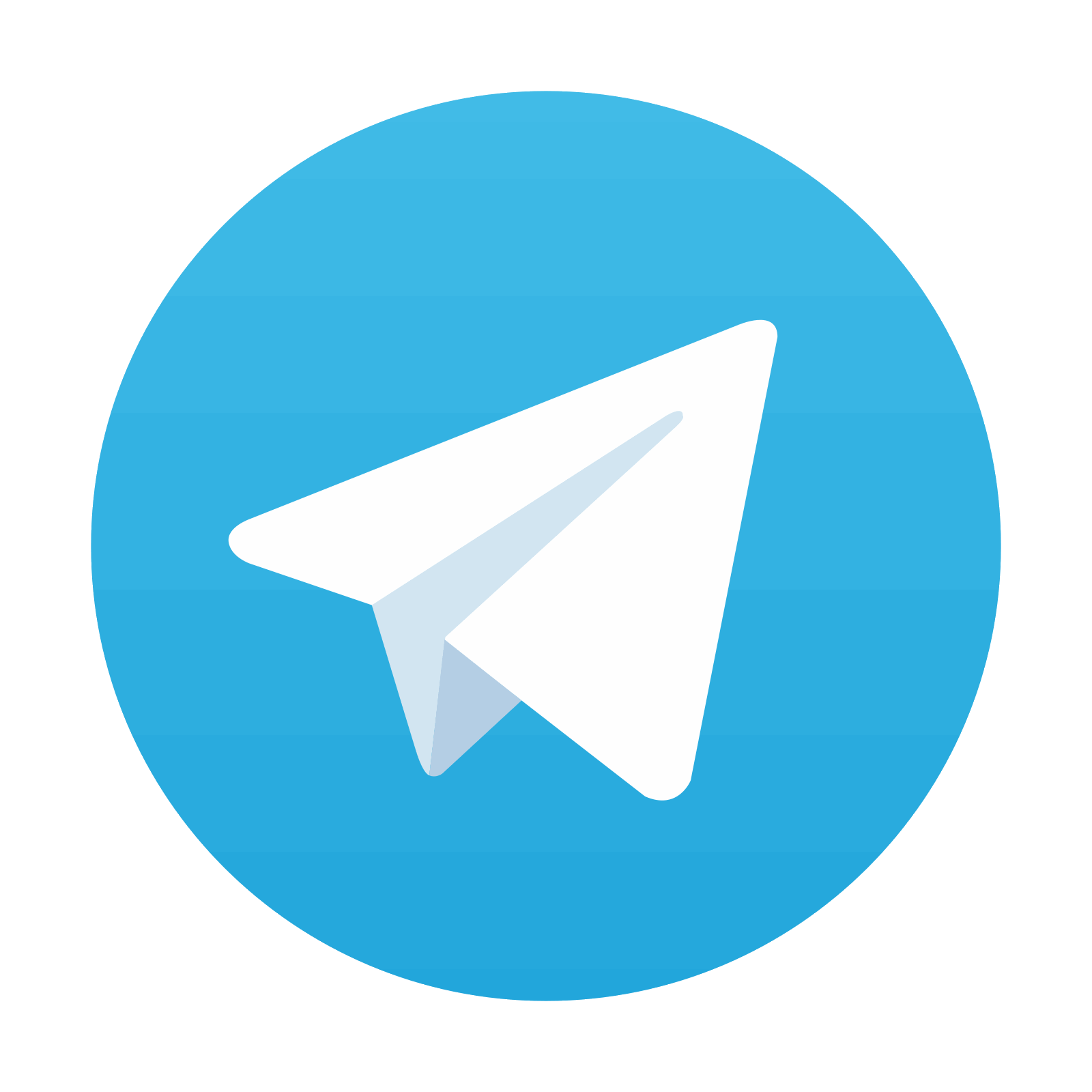
Stay updated, free articles. Join our Telegram channel

Full access? Get Clinical Tree
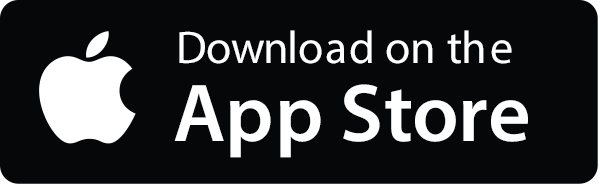
