Fig. 8.1
Representation of the physical bases of the reconstruction of the diffusion tensor. In homogeneous tissues, the three eigenvalues have similar values, and the tensor is spherical. In tissues where barriers restrict water diffusion in a particular direction, the tensor is an ellipsoid, and the direction corresponding to the principal eigenvalue represents the direction of the fibres
The diffusion of water molecules in biological tissues can be measured using MR gradients and diffusion-weighted sequences. The acquisition technique consists of “tagging” the water molecules with a very short gradient. Tagged molecules acquire a magnetization and a phase that depend on their spatial position. The natural phenomenon of diffusion causes the displacement of these molecules to areas containing molecules with different magnetization and phase. The presence in a region of signals with different magnetization and phase results in an overall lower signal intensity, as the signal of the diffusing molecules reduces the one of local molecules. Therefore, increasing diffusion of the water molecules induces a reduction in tissue signal. The study of diffusion anisotropy uses the same image acquisition strategies as clinical diffusion studies. Indeed, water molecule diffusion data are actually generated as anisotropic data, because all acquisitions read diffusion along a given spatial direction, coinciding with the direction of the magnetic field gradient used. In clinical diffusion studies, the direction information is lost through the averaging of diffusion values along the three spatial axes; this simplifies the detection of pathological changes in the diffusion coefficient, which are independent of fibre direction.
In DTI studies, this information is retained, and the prevalence of diffusion along a direction, e.g. along a fibre bundle, can be expressed in terms of anisotropy. The degree of anisotropy can be quantified using the diffusion tensor [9–11]. A tensor is a complex mathematical entity [12]; when measured with MR, it may be represented in matrix form using data from diffusion-weighted images to obtain parameters like fractional anisotropy (FA) and the apparent diffusion coefficient (ADC), better known nowadays as mean diffusivity (MD) [13]. The diffusion tensor also contains much additional information. In particular, an algebraic procedure called diagonalization makes it possible to obtain for each image voxel three eigenvalues (λ1, λ2, λ3) representing the values of diffusion along three spatial directions (eigenvectors). If in a given voxel the three values are similar (λ1~λ2~λ3), as in all grey matter, the water diffuses in a similar manner in all directions and its diffusion in the voxel is called isotropic. If, by contrast, one of the three eigenvalues is much greater than the other two, as in white matter, water diffuses more easily along the direction corresponding to that eigenvalue, and its diffusion in the voxel is anisotropic.
It is worth noting that, unlike MR parameters such as relaxation times, those obtained from the diffusion tensor do not depend directly on the magnetic field and can thus be measured and directly compared between high- and low-field acquisitions. In practice, whereas T1 and T2, and thus the relevant images, change as a function of the magnetic field, water diffusion in a given space is the same at 1.5, 3.0 and even 7.0 T. Diffusion studies thus fully benefit from the greater signal/noise ratio (SNR) of high-field magnets.
8.2 Image Acquisition
Calculation of the diffusion tensor requires acquisition of a set of MR images using suitable diffusion-weighted sequences. Echo-planar sequences with different gradient directions and intensities are the more appropriate for these applications and are those most commonly used [1, 14, 15].
Diffusion weighting involves a general reduction in signal intensity, which increases the greater the diffusion of water. This magnifies the SNR problems shared by all MR acquisitions and makes it difficult to obtain images with very high spatial resolution. Currently, the best spatial resolution that can be achieved is rarely less than 1 mm, particularly along the slice-encoding direction, but the use of high magnetic fields (3.0 T or greater) and parallel imaging can further enhance resolution [16]. Diffusion weighting depends on gradient intensity, which is usually denominated b factor and is measured in s/mm2. In theory, increasing b values should be used to calculate MD; in practice, limitations in the gradients used in clinical practice, specific absorption rate and times of acquisition have led to the prevalent use of only two b values, one virtually null (no diffusion weighting) and the second high (1000 s/mm2 or greater). A b value slightly greater than 0 (~20) is used to remove the effects of large vessels. The minimum set of images to be acquired for a DTI study includes six different diffusion-weighted directions (with b = 1000 or greater) and a non-diffusion-weighted scan (b = 0). The minimum set may be acquired several times to improve the SNR, whereas acquisitions with different b values for each direction are unnecessary as well as inefficient in terms of SNR [11]. More accurate evaluation of the diffusion coefficient D from two acquisitions has been demonstrated using two values of b differing by ~1/D, which in the brain entails that b2–b1 < 1000–1500 s/mm2 [11, 17]. If more than two acquisitions are performed to optimize the SNR, the theory of error propagation states that it is more convenient to obtain multiple acquisitions at the two b values selected than to use a broader range of b values [11].
Acquisitions of b values in a range, rather than a pair (b = 0 and b = 1000), can however provide interesting information [18, 19]. Although a single MD value is usually assigned at each tissue voxel, most tissues are indeed made up of separate compartments, each bearing its distinct value of MD. Brain tissue comprises at least two compartments, a fast-diffusion intracellular compartment and a slower-diffusion extracellular compartment. The MD depends on the range of b values used, because low values (1000) are more sensitive to fast-diffusion components and thus to the structural features of the interstitium, than to those of axon fibres. Ideally, the different tissue compartments should be studied separately using several different b values and then fitted with a multi-exponential function. However, since slow-diffusion compartments can be studied only with high b values and favourable SNRs, this is difficult to achieve.
Simulation studies [20] confirmed the notion that higher b values allow the attainment of shaper angular diffusion profiles, which are more sensitive to the orientation of fibres. In particular, by increasing the b value from 1000 to 3000 s/mm2, the minimal resolvable angle between fibre bundles was reduced from about 45 to 30°, independently from the number of diffusion-encoding gradient orientations. Instead, increasing the b value to 5000 s/mm2 did not improve the diffusion model further.
The number of directions offering the best compromise between a reliably reconstructed tensor (multiple directions) and long acquisition times is still debated. In a given voxel, if the fibres are all oriented along the same axis, sampling of the angular diffusion space along a limited set of directions (at least six) is not a problem. However, large portions of white matter are characterized by complex fibre configurations [21], and for this reason the diffusion-weighted signal needs to be acquired along a larger number of unique orientations in order to obtain an accurate reconstruction of fibre orientation distribution functions. Even in the case of a single direction of the fibres within a voxel, a high number of sampling directions would be needed, since the orientation of this direction might change from voxel to voxel.
Besides increasing the precision (reproducibility) in reconstructing fibre orientation distribution functions, a higher number of unique sampling orientations will also reduce the extent to which the variance in an estimate of a given parameter depends on the orientation of the structure. For example, while from a mathematical standpoint at least six different directions plus a low b value acquisition are required in order to fit the diffusion tensor model, it has been shown that for a statistically rotationally invariant reconstruction (such that the variance in tensor-derived parameters is independent of the orientation of the tensor) at least 30 directions uniformly distributed over the sphere are needed [22]. Currently, most researchers use 6 to 90 different directions, with considerable differences in acquisition times and uncertain benefit. A sequence with 68 directions, b = 100 and 1000 s/mm2, and a cubic voxel of 2.3 mm lasts about 13 min, but actually takes much longer because image averaging to obtain an acceptable SNR requires multiple acquisitions. Here, a large role is played by acquisition conditions, particularly magnetic field intensity and the availability of parallel imaging to improve the SNR.
High-angular resolution techniques (HARDI; [23]), which require a much greater number of directions (even 252 or more), benefit from favourable conditions of field intensity and high coil sensitivity. A drawback of HARDI techniques is their requirement for a greater number of diffusion-encoded acquisitions compared to DTI, leading to an increase in acquisition time. In addition, these techniques often use a substantial amount of the acquisition sequence duration for the diffusion-encoding gradients, resulting in long repetition and scan times. For example, a typical 60-direction, 60-slice whole-brain Q-ball acquisition can take up to 10 min to complete the diffusion and slice encoding, while a 257-direction whole-brain diffusion spectrum imaging scan lasts as long as 45 min. The length of these acquisitions limits their utility in clinical and research studies. In order to overcome this limitation, several approaches have been developed, such as conventional accelerated 2D parallel imaging approaches and simultaneous multi-slice approaches for single-shot echo-planar imaging. With 2D parallel imaging [24], the number of phase-encoding steps can be decreased by a factor up to 4, thus significantly reducing image distortion and blurring. Despite allowing for an improved image quality, this reduction in echo train length does not translate to a significant reduction in acquisition time because of the large fixed diffusion-encoding time blocks [25]. On the other hand, multi-slice approaches can reduce scan time by a factor equal to the number of excited slices, which are diffusion-encoded with the same diffusion gradients, and readout simultaneously. Methods developed according to this approach include wideband imaging [26, 27], simultaneous image refocusing (SIR) [28, 29] and parallel image reconstruction-based multi-slice imaging [30–34]. Unfortunately, these techniques suffer from significant artefact and/or SNR loss: the wideband approach suffers from a large voxel tilting artefact, while the SIR technique necessarily lengthens the readout period of the echo-planar imaging, thus increasing echo time and susceptibility-induced image distortions. On the other hand, multi-slice imaging techniques based on parallel image reconstruction can lead to a large SNR penalty related to the g-factor, since the aliased slices are generally close to each other due to a comparatively small field of view (FOV) in the slice direction. Several attempts have been developed to solve these issues, such as the controlled aliasing in parallel imaging results in higher acceleration (CAIPIRINHA) technique [30] and the blipped-CAIPI method [34].
As mentioned above, the diffusion tensor basically provides two types of information: a quantitative estimate of diffusion anisotropy and the spatial orientation of fibres (Fig. 8.2). These data are interesting but “local”, i.e. they regard a single voxel. Tractography uses these microscopic data to obtain “global” information and reconstruct macroscopic fibre tracts.
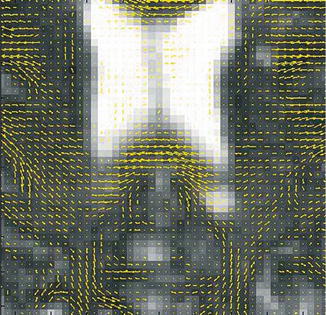
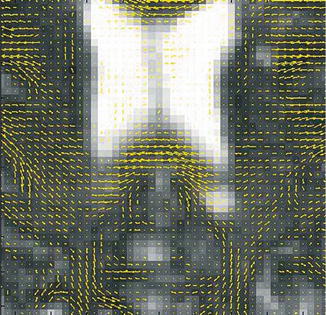
Fig. 8.2
Diffusion tensor. Projection in the image plane of the principal eigenvector. Vectors are represented by double-headed arrows because diffusion data provide the direction, not the orientation of diffusion
8.3 Fibre-Tracking Techniques
8.3.1 Line Propagation Algorithm: Deterministic Tractography
The voxel grid of an MR image may be compared to a chessboard: selecting a number of adjacent voxels that form a trajectory is like drawing a line on the chessboard (Fig. 8.3). The algorithm used to draw this trajectory in most fibre-tracking techniques involves selecting an initial point (seed point) that is highlighted on the image and then moving to the next nearest voxel, which in turn is highlighted, along the prevalent anisotropic direction, until a condition that halts this process arises (stopping criterion). The differences among these, line propagation, algorithms lie in the way in which the information contained in the voxels nearest to the one being examined with the algorithm (nearest neighbours approach) is used by the algorithm itself to draw the likeliest trajectories and minimize noise.
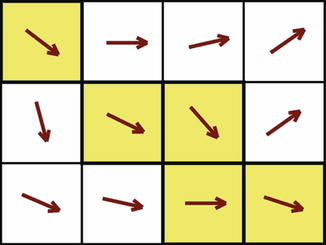
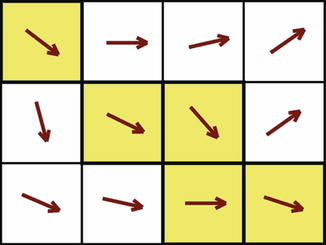
Fig. 8.3
Propagation of a fibre in a vector field. The yellow pixels represent the course of the reconstructed fibre
Since digital images are represented on discrete fields, the vector will often point to an area straddling at least two adjacent voxels, requiring a choice from one or more possible trajectories. In such cases, the selected tract will be a mere approximation of the information contained in the diffusion data, irrespective of the trajectory that has been selected.
The first researchers to reconstruct a white matter fibre tract successfully [35, 36] solved this problem using propagation in a continuous numerical field – where the coordinates can be expressed as decimal values – each time approximating the coordinates of the line to those of the nearest voxel. This simple but fairly rough method can be improved by applying a tract curvature threshold. Assuming that the course of a fibre tract exhibits only reasonably soft curves, whenever two possible trajectories present, the less curved one is selected, while sharp curves (e.g. >60°) are excluded. Line propagation algorithms may require vector interpolation (direction and eigenvalue) at the point of arrival of the previous step, which usually straddles two or more voxels and therefore does not directly correspond to a measured value. Interpolation is a mathematical operation that makes it possible to obtain the value of a point from those of surrounding points. In the simpler algorithms, the vectors corresponding to the neighbouring voxels are interpolated, while the more sophisticated ones directly interpolate the diffusion tensor and calculate a new vector [6]. Interpolation enables more uniform paths to be obtained with respect to the algorithms that do not employ them and is less sensitive to noise, although the additional calculations considerably increase computation time.
8.3.2 Global Algorithms
The algorithms of this class use a radically different approach. In fact, whereas the line propagation algorithms use only local information (i.e. the data contained in a voxel and in those nearest to it), these techniques employ the information in a global way by applying a mathematical function that reproduces the structural characteristics of the fibre tracts. For instance, the physical analogy used for the fast marching technique [37–39] is that of an ink drop falling on adsorbent tissue. The stain extends faster along the direction of the tissue fibres than perpendicular to them. Assuming a vector field indicating the directions in which the ink spreads, a speed function for front propagation can be defined on the basis of the fibres’ anisotropy value. This function reflects the fact that propagation is fastest along fibres and slowest perpendicular to them and makes it possible to calculate the “shape” of the stain from any point at any given time. Its contours may be compared to the isobars of meteorological charts and, in the case of a vector field of DTI data, they represent a sort of map of the likelihood of connection starting from a given point. Using this technique, the course of the fibres coincides with the faster route, hence its name.
Another physical analogy, well known in the field of numerical simulations as the “travelling salesman problem”, can help explain another class of methods. A travelling salesman needs to find the optimum route passing through all the towns where he will be calling. One solution is to define a function, e.g. petrol consumption or time, and find the route that minimizes it. Using DTI data, the function ensuring global energy minimization is related to paths along the direction of the field vectors, while those associated with greater energy expenditure are perpendicular to them [40]. Calculation of the value of the function for all possible trajectories makes it possible to identify the course that minimizes the energy function. However, methods like simulated annealing allow the solution to be found rapidly without calculating the energy for all the possible courses while minimizing the effect of noise.
Global methods have two main advantages. First, they can provide a semi-quantitative estimate of the level of connectivity between two points or regions. In fact, fibres like those shown in Fig. 8.4 provide a visual representation of the bundles, but not a “value” of the connection [41], for instance, between two activation areas shown on functional MR. Secondly, they are less affected by the typical limitations of line propagation algorithms (addressed below). However, the level of calculation required to implement them is often close to the computational capacity of current processors, and they are still in an early phase of development compared with the more common line propagation algorithms (Fig. 8.5).
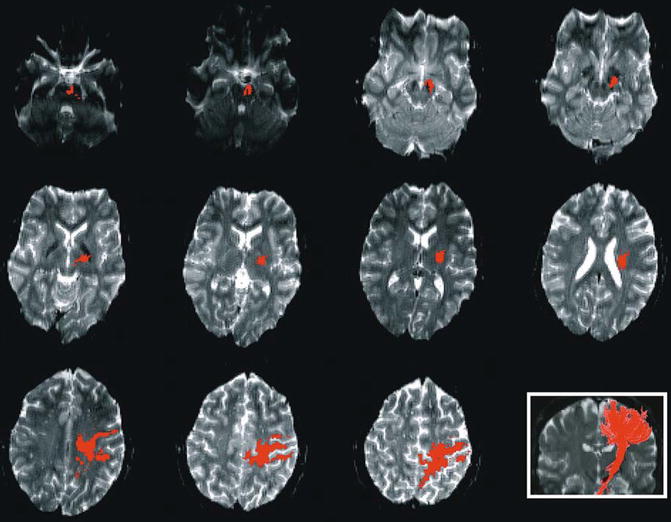
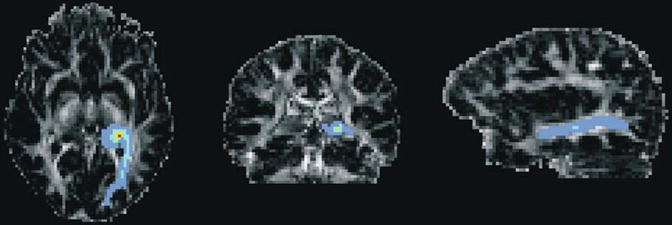
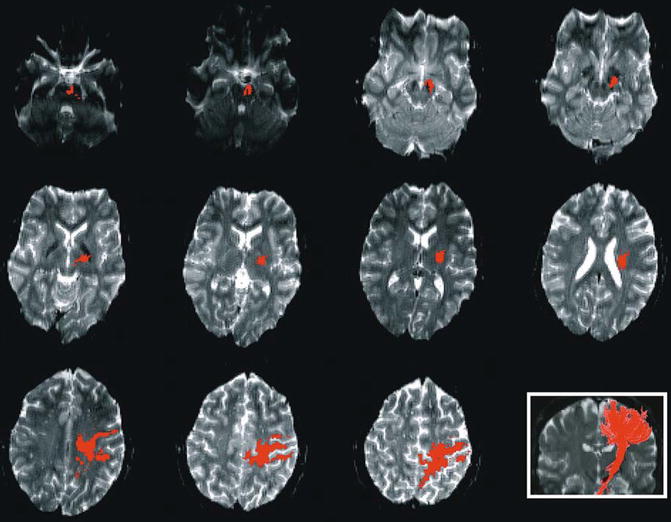
Fig. 8.4
Fibre reconstruction with a line propagation algorithm (left pyramidal tract in red), superimposed on axial T2-weighted images. Lower right corner: 3D reconstruction of the same tract overlaid on a coronal image
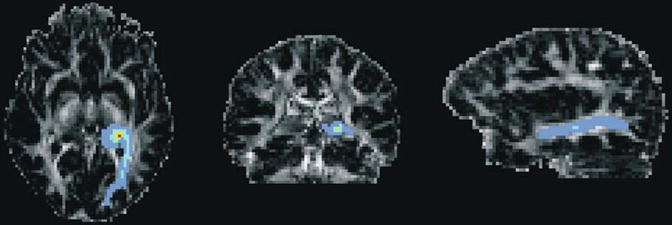
Fig. 8.5
Connectivity map generated using a Monte Carlo algorithm. Overlay on a fractional anisotropy map. Fractional anisotropy is derived from the diffusion tensor and represents white matter distribution. Colours represent the likelihood of connection with the seed point in the left lateral geniculate nucleus, according to an intensity scale
Halfway between deterministic (line propagation) and global algorithms are the Monte Carlo probabilistic methods [42–44]. With these techniques, thus named for their similarity to gambling, each time the tract is propagated from one voxel to the next, the various directions are given a probability value depending on the diffusion values measured. It is assumed that by repeating the line propagation a large number of times, the course that has been selected most often will correspond to the actual trajectory of the fibre.
To date, several deterministic (line propagation) and probabilistic tractography algorithms have been developed, some of which can be applied not only to DTI data but also on orientation distribution functions reconstructed on HARDI data. The most commonly used deterministic algorithm is the “fibre assignment by continuous tracking” (FACT; [36]), which is computationally leaner and requires minutes to perform whole-brain tractography for a single subject. For what concerns probabilistic tractography, the most commonly used approach relies on the combination of two steps [44, 45]: first, a Bayesian method for assessing the most appropriate number of fibre orientations at each voxel is performed; subsequently, probabilistic tractography through the complex orientation fields is carried out. The main advantage of this approach is that the Bayesian step allows the modelling of crossing fibres, which makes this approach more sensitive to secondary or subordinate pathways. Each voxel is modelled as an isotropic compartment (ball: “round” tensor with all eigenvalues equal) and one or several anisotropic compartments (sticks: “thin” tensors with only one non-zero eigenvalue).
However, the obtainable accuracy in identifying complex fibre configurations based on DTI data is limited [21], and HARDI approaches might be more suitable to this scope, even if their application in clinical routine is heavily limited by the long acquisition times.
8.3.3 Seed Point
A factor requiring careful consideration is the initial point of tract propagation, as this choice influences the relative effect of noise on the propagation itself. In the earliest approaches, a frequently adopted solution was to use a number of equidistant seed points arranged on a grid space. This reduced the variance connected with the arbitrary choice of the seed point. An acceptance criterion was applied to avoid selecting voxels not containing fibres. To date, the most frequently used condition is a minimum FA value ensuring the presence of a distinct fibre at the seed point. It is worth stressing that, since the directions identified in each voxel by the diffusion tensor do not have an orientation (see Fig. 8.2), a forward and a backward pathway, lying on the same straight line but running in opposite directions, are consistently generated at seed points.
More recently, because of the development of reliable brain atlases of cortical, subcortical and white matter structures, the definition of seed points has changed. In fact, by using these templates, cortical and subcortical region of interest can be automatically identified on the individual MR scan and used as seeds for tract reconstruction.
8.3.4 Stopping Criteria
All fibre-tracking algorithms that use a seed point require a stopping criterion to terminate the propagation process. The most intuitive criterion is the FA value itself; in grey matter FA is low (0.1–0.2 on a 0–1 scale), so the orientation of the principal eigenvector of the diffusion tensor is random and unrelated to that of the fibre tract. A useful stopping criterion may thus be an FA threshold (usually 0.2) below which the propagation is halted, preventing reconstruction of fibres that are not organized into bundles, like grey matter fibres. However, this criterion may also halt the elongation of a line in those white matter voxels, which, albeit containing fibre tracts, have a low FA because of the lack of a main direction (see below).
Another possible stopping criterion is the curvature of the reconstructed fibre tract. The method used to calculate the diffusion tensor assumes the absence in the voxels of sharp curves, in line with the fundamental hypothesis of the Gaussian nature of the diffusion process in all directions. A criterion halting tract propagation in the presence of sharp angles is thus useful, but is difficult to apply to the shorter and more tortuous tracts, where the low spatial resolution of the image does not enable reconstruction of the real course of the fibres.
8.3.5 Waypoints and Termination Points
When one is interested in using tractography to reconstruct a specific white matter tract, the definition of the seed region alone might not be sufficient. In fact, no tractography algorithm, to date, directly incorporates knowledge on the actual bundles known to connect different regions of the brain, and for this reason erroneous connections might be identified. To account for this limitation, there are two main approaches, both relying on the use of atlases. A frequently used procedure is that of defining not only the starting point (seed), but also a termination region, where the tract of interest is known to have one of its ends, and, eventually, one or more regions where the tract is known to pass (waypoints). These regions may be easily identified on existing MRI atlases (see Fig. 8.6, second row). If a deterministic or probabilistic streamline crosses the waypoints (eventually in a fixed order), it will be retained for the final reconstruction of the tract, otherwise it will be excluded. Of course, also one or more avoidance masks can be defined, implying that if a streamline reaches any of their voxels, it is rejected. Waypoints, avoidance and termination regions have been successfully used in order to create an atlas of the principal fibre bundles of the human brain [47].
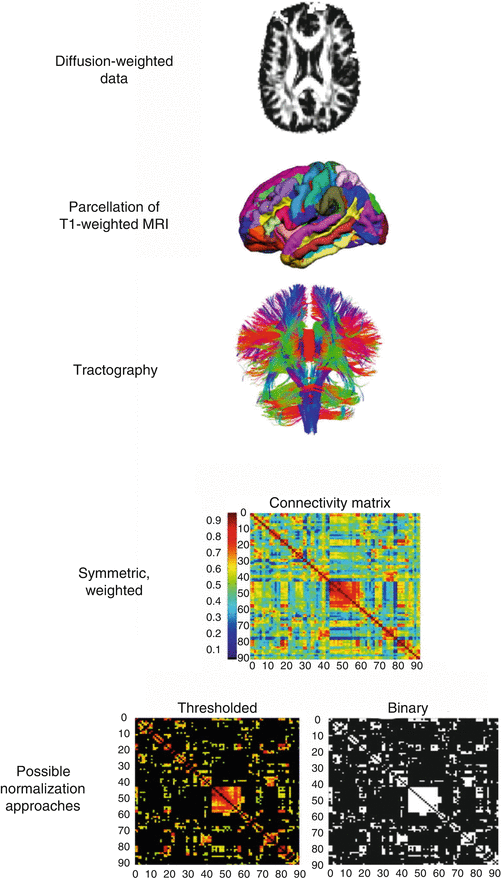
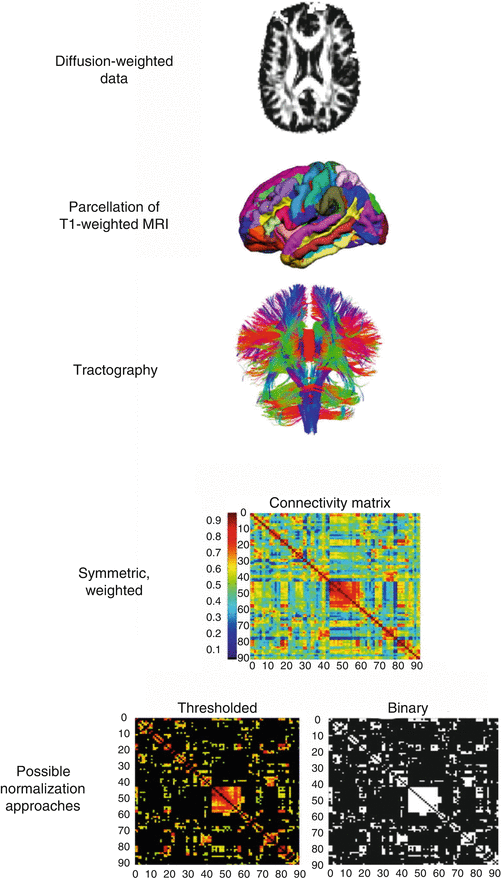
Fig. 8.6
Typical processing workflow for the reconstruction of whole-brain connectivity and network analysis (Adapted from Filippi et al. [46]). Diffusion-weighted data is used to perform tractography (first row). Atlas-based identification of cortical and subcortical brain regions on the individual brain (parcellation, second row) can be used to define seeds, targets, waypoints and exclusion masks for fibre tracking (third row). By using all the regions defined in the atlas to generate pairwise comparisons, a connectivity matrix is obtained in which the connection strength between all pairs of nodes is contained (fourth row). To obtain comparability across different subjects, a normalization step is required (bottom row): the matrix can be thresholded, to exclude weak connections, and or binarized, to exclude connection weights from the analysis
The second approach is mainly used in whole-brain tractography (see Fig. 8.6, third row), either deterministic or probabilistic, and consists in automatically assigning each tractography streamline to a specific white matter bundle. This labelling process relies on the correspondence of the anatomical position of the streamline with that of the white matter tracts in an atlas (which in turn could have been constructed using waypoints and termination masks).
8.3.6 Tractography-Based Metrics
The ultimate aim of tractography is, of course, to extract quantitative information regarding the brain’s structural connections. To this end, there are several metrics that can be extracted from tractography data.
If deterministic tractography is performed, the exact number of streamlines that connect two regions can be calculated and, for example, compared across groups. On the other hand, if probabilistic tractography is used, an analogue measure is calculated, at each voxel, as the fraction of retained streamlines passing through the voxels over all the streamlines generated from the seed region.
Any tractographic reconstruction, appropriately thresholded in order to exclude outliers and normalized by the total number of streamlines, can also be binarized and overlaid on other MRI sequences or maps in order to extract an average value of volume (e.g. from 3D T1-weighted scans) or diffusion metrics (e.g. FA and MD). However, after the development of white matter atlases, similar results can be achieved without performing tractography on the single subjects, but rather extracting the probabilistic map for the region of interest from the atlas itself and registering it on the subject’s image.
The most recently introduced method for exploiting tractography data is represented by the application of graph theory to reconstructed fibre bundles, which is known as structural connectomics [48, 49]: this theory considers pairwise brain connections (reconstructed using any fibre-tracking method) with the scope of modelling the brain as a complex network consisting of nodes (i.e. regions of the cortex and subcortical nuclei) connected by edges (i.e. the white matter bundles). There is a large number of theoretical metrics that can be extracted from graphs in order to quantify network organizational properties both at the global and the local. Here we briefly describe the metrics most frequently used in neuroimaging studies that exploit brain graph analysis [50]. The node degree is the number of edges connected to a given node. For each node, the clustering coefficient is defined as the ratio of the number of actual connections among the first-degree neighbours to the number of all possible connections. The clustering coefficient indicates the extent to which neighbouring nodes are interconnected to one another, thus reflecting the local efficiency of information transfer of a network. In order to assess the graph distance between nodes, the shortest path length is computed by counting the minimum number of edges needed to link any node pair. To measure the centrality of a node (i.e. its importance with respect to the entire network), node betweenness centrality is computed as the fraction of all shortest paths that contain the specific node. Similarly, edge betweenness centrality measures how influential any given edge is with respect to the entire network and is defined as the fraction of all shortest paths in the network that contain the considered edge. At the graph global level, instead, the characteristic path length is calculated as the average of all the shortest path lengths (i.e. across all node pairs), while the global efficiency, another frequently used graph metric, is the average of all the inverse shortest path lengths. Both characteristic path length and global efficiency quantify the global integration of the network, with the latter preferred if measuring topological distances in relatively disconnected graphs. Finally, any complex network in graph theory can be decomposed into modules. Each module is composed by a set of nodes whose connections with each other are much stronger than their connections to nodes in different modules. To quantify the community structure, the modularity metric can be used to assess how strongly nodes in a community interconnect compared to a random graph with the same number of nodes and edges (i.e. a graph where the edges occur at random). Thus, modularity is a statistical quantity related to the extent to which a network is decomposable into such clearly delineated modules.
Whenever applying graph analysis to tractography data, an important aspect to consider is that intrinsic network organizations are better assessed at a specific connection density, as this way it is less influenced by intersubject variability in the total number of reconstructed streamlines. If the appropriate density were not used, each subject’s raw connectivity matrix (containing, for each subject, the number of connections between any node pair, see Fig. 8.6, bottom rows) would show substantial differences in connections between regions of interest, based on slight variations in individual anatomy. To normalize the network density, connectivity matrices must be thresholded. The threshold is chosen so that a specified percentage of edges is preserved in the network. Furthermore, by imposing another threshold on edge weights (e.g. fibre density), only the strongest edges are preserved, reducing the likelihood of including spurious connections not supported by evidence [51]. Normalized networks allow for topological properties such as path length and clustering to be quantitatively compared across subjects. Binarization of edges aids this process by ensuring that in addition to the number of edges being identical across subjects, the sum of edge weights is also identical. In recent years, several studies used graph theory to explore the organization of brain circuits in healthy controls and different populations of patients with neurological and psychiatric disorders [46, 52–55].
8.4 Limitations of Tractography Techniques and Their Solutions
Also due to their recent introduction, tractography techniques suffer from a number of drawbacks.
8.4.1 Noise
Owing to the frequent need for compromising between acquisition time and image quality, the three-dimensional vector field that is obtained using DTI data may contain a high level of noise. Several researchers have tried to quantify the effects of noise on tensor and tract reconstruction [56–61]. Unlike what takes place in standard MR, the noise present in the reconstructed tensor is not directly perceived on images like those of Figs. 8.2 and 8.4, because it affects only the direction of the tracts. Consequently, though exhibiting a consistent distribution of directions, the vectors may indicate slightly different trajectories with respect to the actual anatomy. This type of noise considerably affects fibre tracking, and one of the main problems of line propagation algorithms is that such errors accumulate with increasing distance from the seed point [44]. Therefore, the greater this distance, the higher the risk of deviation of the reconstructed fibre towards an adjacent, unconnected fibre tract. This possibility should always be taken into account when analysing DTI reconstructions, especially of long fibre tracts. SNR optimization is essential to obviate this and other, conceptually related problems. Here, too, the intensity of the magnetic field used and the availability of parallel imaging play a large role.
8.4.2 Partial Volume
Different types of tissue may be found in a single voxel (partial volume effects), resulting in a reduction in the value of anisotropy [62]. Partial volume effects constitute a problem for fibre-tracking techniques. The problem may be accentuated in short fibre tracts and in those close to grey matter, where white matter tends to thin out and anisotropy to diminish. Addressing partial volume effects requires spatial resolution to be increased, thus reducing the SNR. As in the previous case, the solution lies in defining the noise level tolerated by the algorithm used and in adjusting the resolution and acquisition time of the MR image.
8.4.3 Ultrastructure and Complex Fibre Configurations
DTI provides information on fibre bundles, not on individual axon branches. In addition, the diffusion tensor is unable to model adequately voxels containing more than two axon populations with different directions [63]. For instance, if the relationship among the three eigenvalues of the diffusion tensor is of the type λ1 = λ2 > λ3, the FA may still be sufficiently high as to fail to halt a line propagation algorithm, even in the absence of a major direction with an eigenvalue greater than the other two. In this case, the plane defined by the two major eigenvectors contains several more or less equivalent directions, leading to error. This problem stems from the nature of the diffusion tensor itself, which being a mere second-order approximation of the diffusion process, cannot adequately represent complex situations like the one described. The tensor line technique partially obviates this problem by selecting, among the directions of the plane defined by the two main eigenvectors, the one minimizing the curvature of the trajectory according to the original direction [59].
These methods do not address the possibility that a dominant direction is not identified in a voxel due to crossing bundles giving rise to different directions. The inability to resolve a single direction within each voxel is a significant general limitation of DTI. In fact, in the millimetre scale of the MR voxel, voxels typically exhibit a number of fibre orientations. Common situations of intra-voxel heterogeneity of orientations may be due to the intersection of different white matter bundles or to the complex architecture of subcortical or junctional fibres. In the presence of fascicles with multiple directions within the same voxel, for example, due to crossing or divergence, DTI will estimate the prevalent direction, which does not necessarily correspond to any actual direction. For instance, if in a voxel a vertical bundle branches off a horizontal bundle, DTI will show the presence of a single direction corresponding to their diagonal, thus failing to represent either.
The standard diffusion tensor reconstruction technique using DTI data cannot resolve this problem. Indeed, even using several different diffusion-weighting directions to reconstruct the tensor, its mathematical nature prevents it from identifying the different directions when, for instance, two or three different bundles cross. The inability of the DTI technique to resolve fibres with multiple directions derives from the assumption of the Gaussian nature of the tensor model, because a Gaussian function has a single directional peak, preventing the recognition of multidirectional diffusion by the tensor model.
Methods capable of using all the diffusion information are HARDI techniques [23], which measure diffusion in several directions with an equal distribution in the three-dimensional space but do not calculate the diffusion tensor. These approaches to the resolution of multiple fibre directions in voxels are based on much more complex models of diffusion in nerve tissue (see Figs. 8.7 and 8.8).
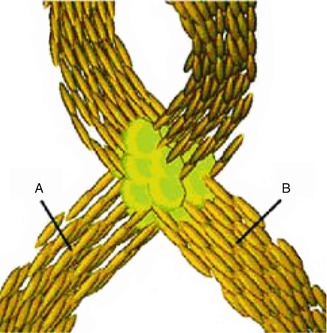
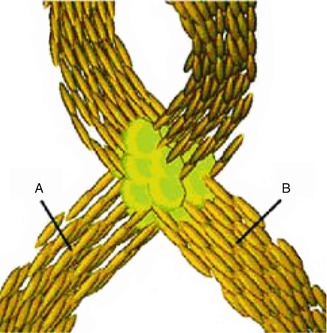
Fig. 8.7
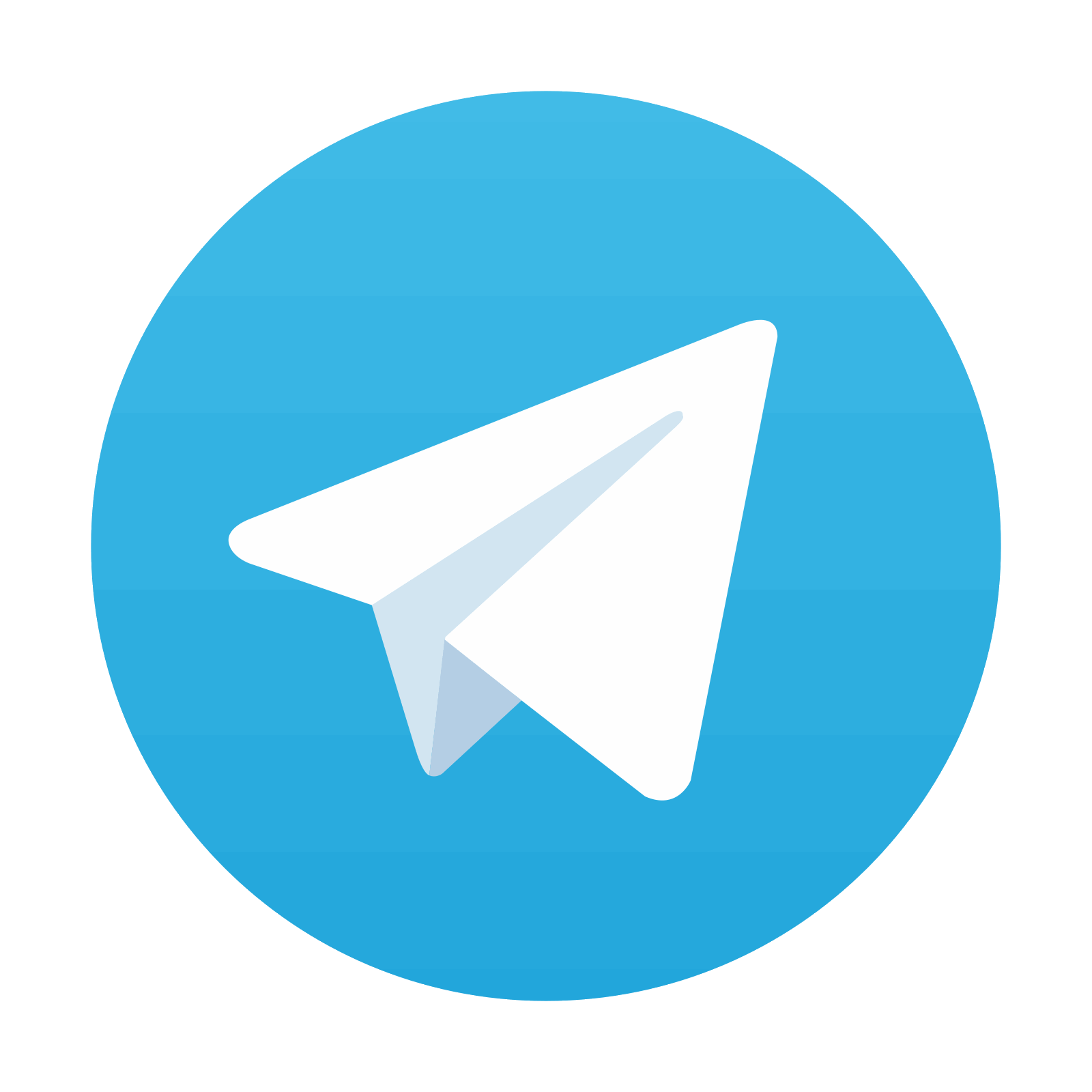
The crossing of two fibre bundles may result in a spherical diffusion tensor, erroneously indicating an absence of fibres
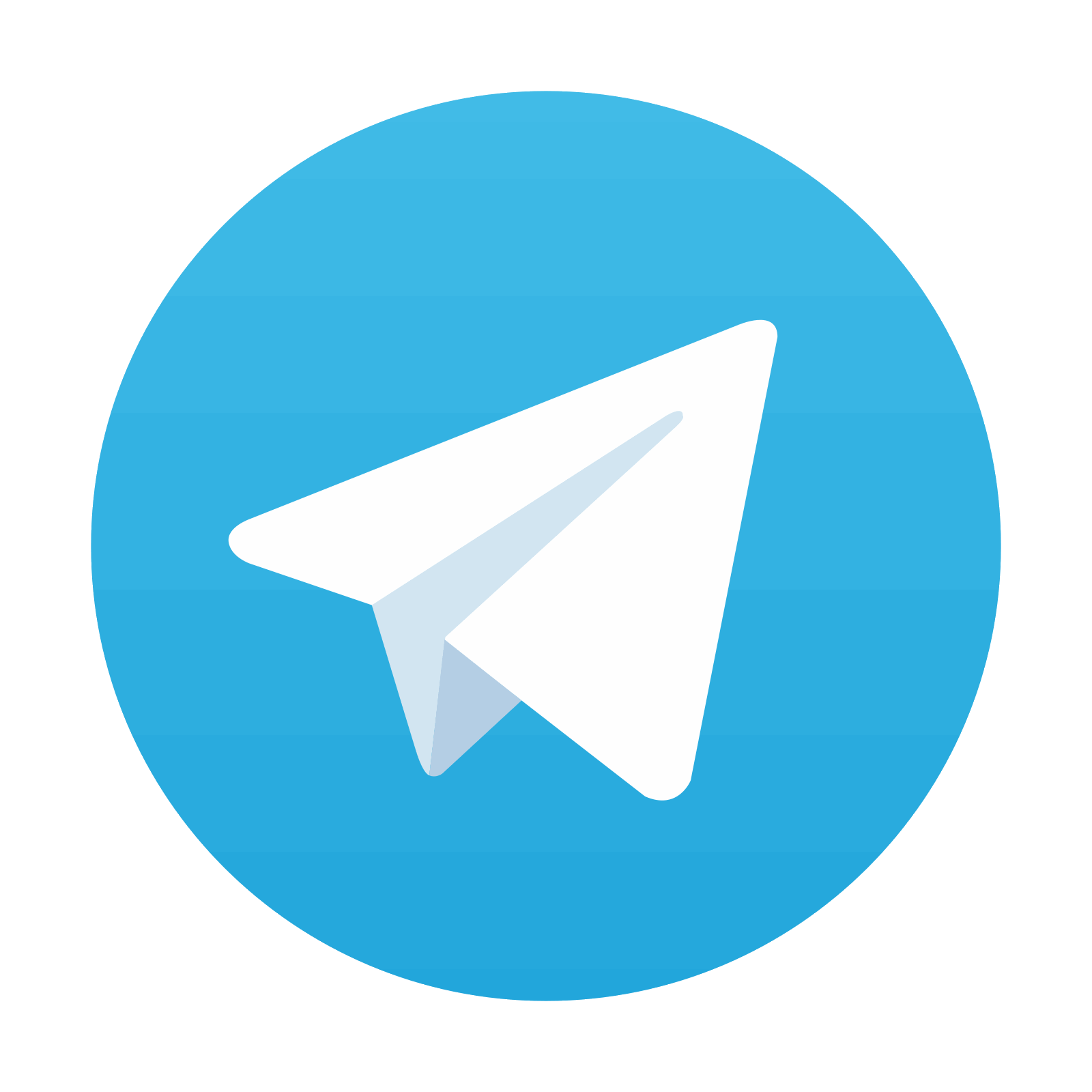
Stay updated, free articles. Join our Telegram channel

Full access? Get Clinical Tree
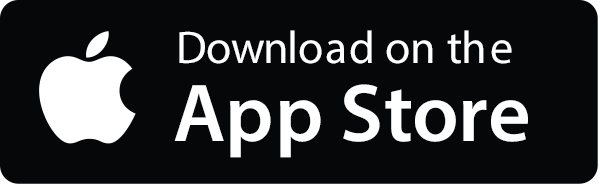

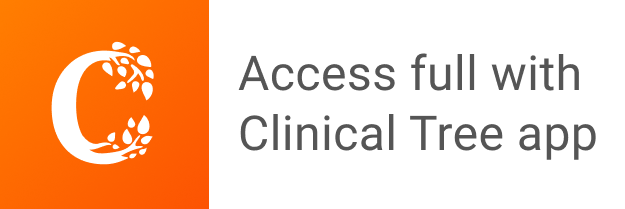